Bioinformatic identification and analysis of immune-related chromatin regulatory genes as potential biomarkers in idiopathic pulmonary fibrosis
Introduction
Idiopathic pulmonary fibrosis (IPF) is a chronic, progressive, and fibrotic interstitial lung disease with an extremely poor prognosis, having a median survival of only 2–3 months after diagnosis (1). Only two drugs have been approved by the US Food and Drug Administration (FDA) for treatment of IPF (pirfenidone and nintedanib), but neither can prevent or reverse the disease progression, only slow down the deterioration in lung function. Their common side effects include nausea, fatigue, and diarrhea, which are often difficult to tolerate. In selected IPF patients, lung transplantation may be the only treatment option (2-4). At present, the pathogenesis of IPF is still unclear but has been well elucidated from the perspective of epigenetics (5). Chromatin regulators (CRs) are indispensable upstream regulatory factors of epigenetics and can modulate the underlying genes of cancer and other diseases (6). According to their modulation mode, CRs can be divided into three categories: DNA methylators, histone modifiers, and chromatin remodelers (7). Current studies have reported that CRs can affect the progress of IPF by modulating the proliferation and differentiation of alveolar myofibroblasts and regulating apoptosis (8-10).
DNA methylation is a chemical modification of the DNA structure. With S-adenosyl methionine as the methyl donor, it is mediated by DNA methyltransferases to complete the covalent bonding with specific bases in the DNA sequence. In eukaryotes, DNA methylation mainly exists in CpG islands in gene promoter regions and exons, primarily involving cytosine nucleotides, and the expression and silencing of its methylation can regulate the expressions of related genes (11). DNA methylation is associated with the occurrence of IPF, and DNA methylation in IPF may affect the expressions of specific genes in the lungs of IPF patients (12,13). In addition, it can regulate IPF-related transforming growth factor-beta (TGF-β) and Wnt signaling pathways (14). Through methylation array data analysis, Evans et al. (14) found that the transcriptional regulator gene c8orf4 was downregulated in fibrotic fibroblasts, and its three CpG islands in the promoter region were hypermethylated. The knockdown of C8orf4 in lung fibroblasts by small interfering RNA revealed that the levels of cyclooxygenase-2 (COX-2) and prostaglandin E2 (PGE2) were downregulated. Therefore, it is believed that downregulation of COX-2 and PGE2 levels in lung fibroblasts is related to the hypermethylation of C8orf4, which leads to fiber growth in the lungs. DNA methylation can also lead to hyperproliferation of fibroblasts and an altered differentiation phenotype of airway macrophages (15).
Histones are a family of basic proteins that associate with DNA in the nucleus of eukaryotic cells. There are five histone types, including H1, H2A, H2B, H3, and H4. Histones assemble to form a nucleosome together with DNA, thereby packing it tight inside the nucleus. Covalent modifications (phosphorylation, methylation, acetylation, and ubiquitination) at the amino terminus of core histones in nucleosomes play an important role in gene regulation, and most of the histone modifications associated with IPF are acetylation, methylation, and ubiquitination (16-18).
Chromatin remodeling refers to the weakening of nucleosome-DNA binding and aggregation between adjacent nucleosomes through physical ATP-dependent chromatin modifications or chemical covalent modifications. Different chromatin remodeling factors can affect epithelial-mesenchymal transition (EMT) processes in multiple organs (19). Obrien et al. exposed non-Brg1 (Brahma-related gene 1) mutant wild-type cell line H441 and Brg1-mutant cell line A549 to TGF-β and found that both cell lines showed a myofibroblast-like phenotype; however, H411 had more obvious upregulation of epithelial cell markers (e.g., E-cadherin) and downregulation of fibroblast markers (e.g., vimentin). In addition, the upregulation of TGF-β target genes (such as PAl-1 and JUN) was more pronounced in H441 than in A549. Therefore, the chromatin remodeling protein Brg1 mediates the EMT process in IPF by affecting the TGF-β signaling pathway (20).
Thus, CRs are closely related to the occurrence of IPF. The recent decades have witnessed dramatic advances in research on the immune system. It has been found that immune dysregulation is a driver of IPF (21). M2 macrophages, Th17 cells, CD8+ T cells, and possibly regulatory T-cells (Tregs) promote fibrosis, while Th1 and TRM CD4+ T cells appear to be protective. Targeted immunomodulators show promise as IPF treatment (22).
Epigenetic dysregulation is an important feature of immune escape in tumorigenesis, and CRs have cell-intrinsic effects on the immune sensitivity of cancer cells (23). Because the immune response is closely related to CRs, there are no functional studies of immune-related CRs in IPF. The aim of this study is to screen immune-related CRs as biomarkers of IPF for diagnosis, treatment and prognostic assessment of IPF. It also provides a basis for further studies on the function of CRs in IPF. We present the following article in accordance with the TRIPOD reporting checklist (available at https://atm.amegroups.com/article/view/10.21037/atm-22-3700/rc).
Methods
Data sources
All the data were downloaded from the Gene Expression Omnibus (GEO) database (https://www.ncbi.nlm.nih.gov/geo/). Data on normal and IPF tissues were collected from four datasets (GSE53845, GSE17978, GSE24206, and GSE70866) on May 1, 2022. Three (GSE53845, GSE17978, and GSE24206) were combined into an integrated dataset to be used as the training dataset, and GSE70866 was used as a validation dataset. Data from the three datasets were merged and normalized using the “SVA” R package. The study was conducted in accordance with the Declaration of Helsinki (as revised in 2013).
Identification of CRs and screening of differentially expressed genes (DEGs)
We identified 870 CR-related genes from the appendix of a literature review (24). We used the “Limma” R package to screen DEGs (n=169), with |log2 Fold Change| >1 and adjusted P value <0.05 as the cut-off values. Volcano plots and heat maps of the DEGs were drawn. Gene Ontology (GO) and Kyoto Encyclopedia of Genes and Genomes (KEGG) pathway enrichment analyses of the DEGs were performed with the “clusterProfiler” package. A protein-protein interaction (PPI) network of DEGs was constructed and visualized by the STRING database (with a minimum required interaction score of 0.9) and Cytoscape 3.8.2 software, respectively.
Identification of hub genes on WGCNA
Weighted gene coexpression network analysis (WGCNA) of all genes in IPF and normal tissues was performed using the “WGCNA” R package. Low-expression genes were filtered, and samples were clustered to remove outliers as abnormal values. An adjacency matrix was constructed to map the association between the graph nodes. The pickSoftThreshold function was used to select the optimal value for the weighting parameters of adjacent functions, with a soft threshold β=3 (scale-free R2=0.9). The modules of related genes were constructed based on hierarchical clustering of the dissimilarity measure (1-TOM) of the topological overlap matrix (TOM). The minimum number of genes in modules was set to 60 and the threshold for merging similar modules was set to 0.25. The coexpression module is a collection of genes with high topological overlap similarity, and genes in the same module often have a higher degree of coexpression. Module eigengene is defined as the first principal component of the expression matrix of the corresponding module and is used to describe the expression pattern of the module in each sample. The key modules (yellow, green, and brown) were screened for analysis. The inclusive criterion for identifying eigengenes (n=390) in the key modules was as follows: module membership (MM) >0.8 and gene significance (GS) >0.5. MM refers to the correlation coefficient between genes and module eigengenes, which is used to describe the reliability of a gene belonging to a module. GS values reflect the correlation between gene expression levels.
Construction of a diagnostic model based on CRs
Four genes (PADI4, IGFBP7, GADD45A, and SETBP1) were obtained by overlapping and intersecting the DEGs and eigengenes. The “ggstatsplot” package was used to perform Spearman correlation analysis on diagnostic markers and infiltrating immune cells and the “ggplot2” package was used to visualize the results, which showed that PADI4, IGFBP7, and GADD45A correlated with immunity. A logistic regression model was constructed with PADI4, IGFBP7, and GADD45A as joint predictors. The sample information of normal and IPF tissues in the GSE53845, GSE17978, and GSE24206 datasets was extracted as the training sets, and the receiver operating characteristic (ROC) curves were drawn using the “ROC” R package. Area under the ROC curve (AUC) was used to assess the accuracy of the regression model for differential diagnosis. GSE70866 was used as the validation dataset. Nomograms were drawn for various outcomes with the “nomogram()” function in R.
Analysis of immune activity
Single-sample gene set enrichment analysis (ssGSEA) was performed using the “gsva” package to calculate the score of infiltrating immune cells and to assess immune function. Heatmaps of the expressions of immune cells and immune function in each sample were drawn using the “pheatmap” package. Heatmaps reflecting the correlations between immune cells and immune function were drawn using the “corrplot” package. The “vioplot” package was used to visualize and compare the levels of immune cells in IPF and normal tissues.
Statistical analysis
R software (version 4.2.0) was used for statistical analysis. The R packages applied in each step were downloaded from the Bioconductor website (https://bioconductor.org/). All reported P values are two-tailed. P<0.05 was set as the threshold for statistical significance.
Results
Identification of CR DEGs between normal and IPF tissues
Among the three GEO datasets (GSE53845, GSE17978, and GSE24206) selected, GSE53845 contained 8 normal lung tissue samples and 40 IPF tissue samples, GSE17978 contained 20 normal tissue samples and 38 IPF tissue samples, and GSE24206 contained 6 normal lung tissue samples and 17 IPF tissue samples. By comparing the expression levels of CR-related genes in the 34 normal tissues and 95 IPF tissues, we screened 169 CR DEGs (P<0.05, |log2FC| ≥0.2), of which 88 genes were upregulated and 81 were downregulated. Volcano plots and heat maps of these DEGs were drawn (Figures 1,2). To further analyze the interactions among the CR DEGs, a PPI network with 169 nodes and 194 edges was constructed using the highest stringent minimum required interaction score of 0.9 by STRING (Figure 3). KEGG enrichment analysis (Figure 4) showed that DEGs were mainly enriched in the cell cycle, p53 signaling pathway, viral life cycle - HIV-1, Notch signaling pathway, transcription coregulator activity, and other signaling pathways. GO enrichment analysis, which depicts three complementary biological concepts including biological process (BP), molecular function (MF) and cellular component (CC), showed the top 5 enriched terms. BP enrichment showed that DEGs were mainly involved in histone modification, chromatin organization, chromatin remodeling, peptidyl-lysine modification, and peptidyl-lysine acetylation; GO:0018393 internal peptidyl-lysine acetylation; the most enriched CC included SWI/SNF superfamily-type complex, ATPase complex, histone deacetylase complex, histone acetyltransferase complex, and histone methyltransferase complex; and the top 5 enriched MF terms included histone binding, transcriptional coregulator activity, nucleosome binding, modification-dependent protein binding, and transcriptional coactivator activity (Figure 5).

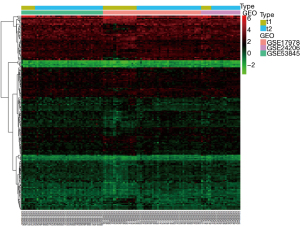

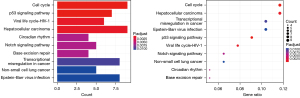
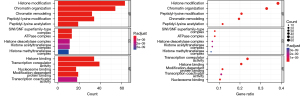
Gene coexpression network and identification of key modules and hub genes
After data preprocessing, a gene expression matrix (14,725 genes) was generated after the three databases (GSE53845, GSE17978, and GSE24206) were merged. The missing values of the samples were checked, and cluster analysis was performed to remove outliers and abnormal samples. The modules of related of genes in modules were constructed based on hierarchical clustering of the dissimilarity measure (1-TOM) of the TOM. The minimum number of genes in modules was set to 60 and the threshold for merging similar modules was set to 0.25. A total of 9 modules were yielded. Genes in the same module had high connectivity and similar functions (Figure 6). Each module was assigned a unique color identifier and an expression profile summarized by module eigengenes (MEs) (Figure 7). The ME of the brown modules negatively correlated with IPF (r=−0.52, P=2−10), and the MEs of the green modules (r=0.41, P=2–6) and yellow modules (r=0.75, P=4−24) positively correlated with IPF. The brown, green, and yellow modules were selected as key modules for further analyses. Phenotypic data, and correlation graphs were used to show the correlation between the MM and GS of IPF-related modules (Figures 8-10). Genes with MM >0.8 and GS >0.5 in the three modules were selected, and the eligible genes in the three key modules were merged into the eigengenes of IPF (n=390).
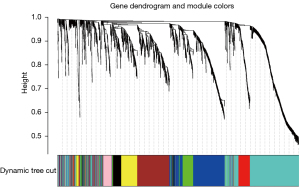
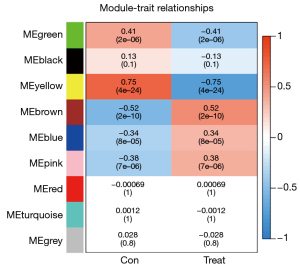
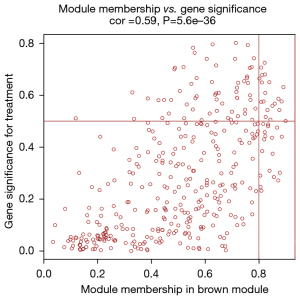
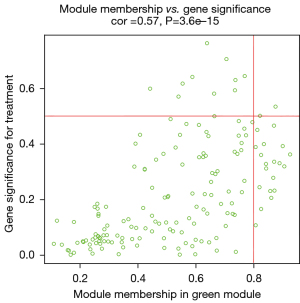
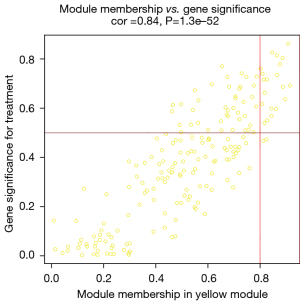
Identification and validation of immune-related CR genes
Four hub genes (PADI4, IGFBP7, GADD45A, and SETBP1) were obtained by overlapping and intersecting the DEGs and eigengenes screened by WGCNA (Figure 11). The relationships of these four hub genes with immune cells and immune function were analyzed using the “ggcorrplot” R package, which showed that PADI4, IGFBP7, and GADD45A correlated with immunity (Figure 12). Analysis of the potential correlation between relevant biomarkers and infiltrating immune cells showed that: GADD45A positively correlated with APC_co_stimulation, CCR, parainflammation, and Treg; IGFBP7 negatively correlated with APC_co_inhibition and positively correlated with Mast_cells; and PADI4 negatively correlated with HLA, Macrophages, and Mast_cells and positively correlated with Neutrophils. A logistic regression model was constructed based on the three immHub genes (PADI4, IGFBP7 and GADD45A), with whether the tissue was IPF as the dependent variable and the levels of PADI4, IGFBP7 and GADD45A as the dependent variables. PADI4, IGFBP7, and GADD45A were divided into high- and low-expression groups according to their median values. The levels of PADI4 [odds ratio (OR) =0.2498; 95% confidence interval (CI): 0.092–0.629], IGFBP7 (OR =2.7784; 95% CI: 1.055–7.736), and GADD45A (OR =3.0641; 95% CI: 1.18–8.435) correlated with the occurrence of IPF (all P<0.05). According to the prediction formula [P=1/1+exp(-Z)] of the regression model, the prediction formula of our current study was: P=1/{1 + exp[–(1.022 × IGFBP7 – 1.387 × PADI4 + 1.120 × GADD45A)]. For the training dataset, the AUC was used as the assessment parameter, which was 0.771. Nomograms were drawn for various outcomes (Figures 13,14).
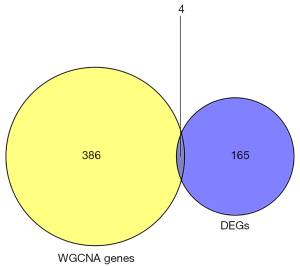
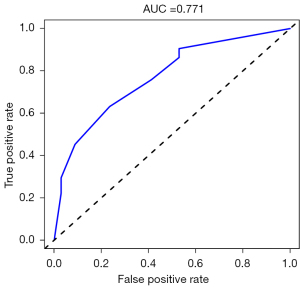
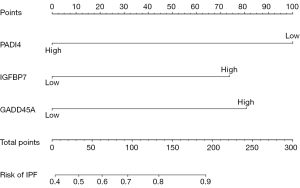
Validating model performance in the validation dataset. In the validation dataset, the AUC was 0.759. Nomograms are shown in Figures 15,16.
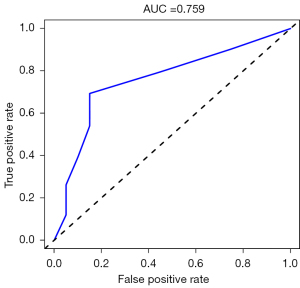
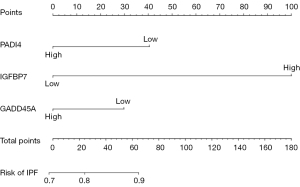
The ROC is used to determine the differential diagnostic accuracy of the i.e. logistic regression model, with the closer the AUC value to 1 the more effective the model is. We believe that models with AUC between 0.5 and 0.6 have low diagnostic accuracy, models with AUC between 0.6 and 0.7 have some diagnostic accuracy, models with AUC between 0.7 and 0.8 have high accuracy, and models with AUC >0.9 have excellent diagnostic performance.
The AUCs of the models ranged from 0.7 to 0.8 in the training and validation datasets, indicating that the model had good predictive ability.
Immune activities of IPF and normal tissues
Heatmaps were constructed to display the expressions of immune cells and immune function in each sample (Figure 17). Analyses of the enrichment scores of 16 immune cells in IPF and normal tissues and the activities of 13 immune function showed that, among intestinal mucosal mast cells (IMMCs), B_cells, CD8+_T_cells, DCs, iDCs, Macrophages, Mast_cells, T_helper_cells Tfh, and Th2_cells were highly expressed in IPF whereas NK_cells and Neutrophils were lowly expressed in IPF. In IMMF, APC_co_stimulation, Check-point, HLA, Inflammation-promoting, MHC_class_I, Parainflammation, T_cell_co-inhibition, and T_cell_co-stimulation were all highly expressed in IPF tissues (Figure 18). Analysis for the potential correlations between immune cells and immune function showed that immune cell Tumor Infiltrating lymphocytes positively correlated with B_cells (r=0.75) and Neutrophils negatively correlated with Mast_cells (r=–0.38). Analysis for the potential correlations among immune function showed that Check-point positively correlated with T_cell_co-stimulation (r=0.88) and Type_II_IFN_Response negatively correlated with HLA (r=–0.06) (Figure 19).
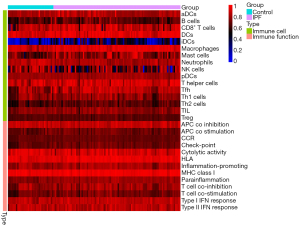
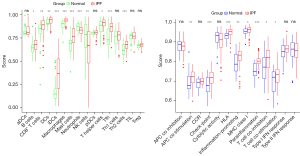
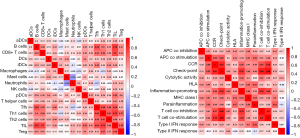
Prediction of model-related gene therapy drugs
Through the Enrichr website (https://maayanlab.cloud/Enrichr/), some small-molecule compounds that may be used in the treatment of IPF were obtained, and the top 10 drugs are listed (Table 1).
Table 1
Rank | Name | P value | Genes |
---|---|---|---|
1 | Dimethyl sulfoxide CTD 00005842 | 3.18E-04 | GADD45A; PADI4 |
2 | Dronabinol CTD 00006853 | 0.001192927 | GADD45A; IGFBP7 |
3 | Primaquine PC3 UP | 0.001389158 | IGFBP7; PADI4 |
4 | Scopolamine PC3 UP | 0.001519268 | IGFBP7; PADI4 |
5 | Pregnenolone PC3 UP | 0.001532594 | IGFBP7; PADI4 |
6 | Etoposide CTD 00005948 | 0.00156616 | GADD45A; IGFBP7 |
7 | PTAQUILOSIDE CTD 00001981 | 0.001649143 | GADD45A |
8 | Lead dinitrate CTD 00000898 | 0.001649143 | GADD45A |
9 | Streptonigrin CTD 00006785 | 0.001649143 | PADI4 |
10 | IPRODIONE CTD 00001594 | 0.001649143 | GADD45A |
Discussion
CRs are a class of enzymes with special functional structures that can recognize, form, and maintain epigenetic states in a cell-environment-dependent manner. CRs are indispensable upstream regulators of epigenetics, and their abnormal expressions are closely related to various BPs. Research has shown that immune dysregulation is the main driver of IPF pathogenesis, and CRs can help tumor immune escape. We constructed a logistic regression model based on three immune-associated CR genes (PADI4, IGFBP7, and GADD45A). The AUC was 0.771 in the training dataset and 0.759 in the validation dataset, indicating our model had good predictive power. The three genes are potential biomarkers in IPF.
PADI4 is a member of the peptidyl arginine deiminase (PAD) family. Depending on PAD enzyme activity, PADI4 can modify the guanidine group of arginine into the ureido group of citrulline. Although PADI4 plays an important role in human innate immunity, NETosis is a special pathway of neutrophil death. When an extracellular pathogen invades, neutrophils release a network of linear fibers called neutrophil elastase traps (NETs) into the extracellular environment to trap pathogens. The PADI4 enzyme predominates in neutrophils and initiates the process of NETs by unfolding and decondensing nuclear chromatin via citrullination of histones. Subsequently, the PADI4 co-expanded NETs enter the extracellular high-calcium space to complete the bactericidal process by citrullinating extracellular proteins such as fibrin (25,26).
PADI4 was first reported by the genome-wide association study as a disease susceptibility gene for non-MHC rheumatoid arthritis (RA). PADI4 is currently considered to be an important in the pathogenesis of RA (27). Studies have shown that PADI4 interferes with fibrosis in several organs, including the lung, by affecting the release of NETs. Suzuki et al. found that the expression of NETs in PADI4 knockout mice was suppressed, and the level of tumor necrosis factor-α showed a downward trend. PADI4 deficiency prevented a reduction in the number of alveolar epithelial cells and pulmonary vascular endothelial cells and an increase in ACTA2-positive mesenchymal cells and S100A4-positive fibroblasts in the lungs. Thus, PADI4 deficiency can ameliorate bleomycin-induced NET formation and pulmonary fibrosis (28). In this study, PADI4 gene expression was negatively regulated and suppressed in IPF patients. Recent studies have shown that nintedanib suppressed PADI4 expression in mice. Nintedanib was administered to mice models of RA-related interstitial lung disease (RA-ILD). Changes in PADI4 expression after nintedanib administration were suppressed in the RA-ILD lung. However, similar results have been shown after treatment with nintedanib for galectin-3, which is expected to be the next anti-fibrotic target (29,30).
Insulin-like growth factor-binding protein 7 (IGFBP7) is a glycoprotein with a similar structure to insulin. It localizes to the cell surface and interacts with proteoglycans to promote cell adhesion to fibronectin and cohesin (31,32). A study has shown that IGFBP7 can intervene in the immune system through the IGF pathway, and deletion of IGFBP7 leads to downregulation of a set of interferon (IFN)-γ-regulated genes that regulate antigen presentation, which manifests as inhibition of antigen presentation and the decreased infiltration of CD8+ and CD4+ T cells and natural killer cells in tumor tissue (33).
IGFBP7 can promote various BPs related to fibrotic diseases in different organs. For example, it is involved in the occurrence and development of kidney and liver fibrosis (34,35). TGF-β enhances the expression of IGFBP7 by stimulating renal tubular epithelial cells, and its silencing reduces the number of TGF-β-induced α-SMA-positive cells (35). Likewise, IGFBP7 has been shown to promote hepatic stellate cell activation, favor the development of a myofibroblast phenotype, and promote the production of extracellular matrix proteins (34). Extracellular vesicles (EVs) are important players in intercellular communication. Velázquez-Enríquez et al. revealed key proteins in the EV cargo associated with IPF in vitro through proteomic analysis and found that the IGFBP7 level in EVs was higher in IPF tissue than in normal tissue (36), which was consistent with the findings of Hsu et al. (37).
Growth arrest and DNA-damage-inducible alpha (GADD45A) is a member of the GADD family and highly conserved. The GADD45A protein is widely expressed in normal tissues (38). R-loops are DNA-RNA hybrids enriched at CpG islands (CGIs) that can regulate chromatin states. GADD45A binds directly to the R-loops and mediates local DNA demethylation by recruiting TET1. GADD45A is considered to be an epigenetic R-loop reader (39). As an immune cell, T cells play an important role in the pathogenesis of IPF. There is increasing evidence that Th1/Th2 imbalance is associated with the inflammatory phase in pulmonary fibrosis. IFN-γ and interleukin (IL)-12 secreted by Th1 cells have anti-fibrotic effects, while IL-4, IL-5, and IL-13 secreted by Th2 cells are involved in pulmonary fibrosis. It has been proposed that Th1 response is protective whereas Th2 response causes damage (40). In addition, Tregs are also involved in the pathogenesis of IPF. Boveda-Ruiz et al. found that Tregs can increase TGF-β1 release and collagen deposition in the early stage of pulmonary fibrosis, proving that Tregs have a pro-fibrotic effect in this stage (41); however, Tregs in the late stage have anti-fibrotic effects. Xiong et al. showed that Treg depletion attenuated pulmonary fibrosis by inducing a Th17 response and shifting the Th1/Th2 cytokine balance into Th1 dominance (42). Therefore, immune cells play an auxiliary role in the pathogenesis of IPF, and some immune cells can regulate IPF in a bidirectional manner (43).
GADD45A plays essential roles in controlling differentiation and activating T cells in the immune system; in particular, GADD45A can inhibit p38 kinase activation downstream of T-cell receptors. Salvador et al. found that GADD45A was a negative regulator of T cell proliferation induced by T-cell antigen receptor-mediated activation and that lack of GADD45A led to the development of a lupus-like syndrome; in addition, the counts of leukocytes and lymphocytes in the peripheral blood of GADD45A-deficient mice decreased (44,45).
Although the pathogenic mechanisms of IPF are still unclear, more studies have shown that immune activation is linked to its pathogenesis. In our current study, comparisons of the enrichment scores of 16 immune cells in IPF and normal tissues and the activities of 13 immune function from the ssGSEA showed that, among IMMCs, B_cells, CD8+_T_cells, DCs, iDCs, Macrophages, Mast_cells, T_helper_cells Tfh, and Th2_cells were highly expressed in IPF whereas NK_cells and Neutrophils were lowly expressed in IPF. The expression of PADI4 was downregulated in IPF tissues and showed a positive correlation with the level of neutrophils. Because PADI4 can prevent tissue fibrosis caused by excessive release of NETS, it is believed that PADI4 plays a negative regulatory role in the occurrence of IPF. IGFBP7 was highly expressed in IPF and negatively correlated with APC_co_inhibition and positively correlated with Mast_cells. It has been demonstrated that IGFBP7 is involved in BPs (e.g., cell adhesion), the pathogenesis of IPF, and regulation of the antigen presentation system, which is consistent with the results of our current study. Mathew et al. assessed the extent of pulmonary fibrosis in mice by lung collagen content and histology and found a significant increase in pulmonary fibrotic changes in GADD45a(-/-) mice at 2 weeks after bleomycin treatment (46). In our study, GADD45A was highly expressed in IPF tissues and positively correlated with APC_co_stimulation, CCR, parainflammation, and Treg. Thus, GADD45A plays an essential role in controlling differentiation and activating T cells in the immune system. However, due to the complex role of T cells in pulmonary fibrosis, the relevance of GADD45A to IPF needs to be further investigated.
To sum up, our study showed that IPF is closely related to CRs and immune dysregulation. Analysis based on models constructed from three immune-related CR genes showed that high expression of PADI4 negatively correlated with the occurrence of IPF, and high expressions of IGFBP7 and GADD45A positively associated with the pathogenesis of IPF. The AUC was 0.771 in the training dataset and 0.759 in the validation dataset, suggesting that PADI4, IGFBP7, and GADD45A are potential biomarkers of IPF for predicting risk. This study will help to clarify the pathogenic mechanism of IPF and provide a reference for future targeted therapies.
Our study still had some limitations, and the results of this study have yet to be validated by relevant in vivo and in vitro experiments. Our future studies will continue to focus on the roles of genes such as PADI4, IGFBP7, and GADD45A in IPF.
Acknowledgments
The authors appreciate the academic support from the AME Respiratory Medicine Collaborative Group.
Funding: This work was supported by General Project of Natural Science Foundation of Shandong Province (No. ZR2021MF118; Research on Interpretable Deep Learning Methods for Electronic Health Records); Shandong Provincial People’s Government Taishan Scholarship Construction Program (No. TS201712096); and Shandong Province Traditional Chinese Medicine Science and Technology Program (No. 2020Z06; Feiweikang granules regulating EMT process and intervening in pulmonary fibrosis matrix remodeling: A research based on TGF-β1/notch1 pathway).
Footnote
Reporting Checklist: The authors have completed the TRIPOD reporting checklist. Available at https://atm.amegroups.com/article/view/10.21037/atm-22-3700/rc
Conflicts of Interest: All authors have completed the ICMJE uniform disclosure form (available at https://atm.amegroups.com/article/view/10.21037/atm-22-3700/coif). The authors have no conflicts of interest to declare.
Ethical Statement: The authors are accountable for all aspects of the work in ensuring that questions related to the accuracy or integrity of any part of the work are appropriately investigated and resolved. The study was conducted in accordance with the Declaration of Helsinki (as revised in 2013).
Open Access Statement: This is an Open Access article distributed in accordance with the Creative Commons Attribution-NonCommercial-NoDerivs 4.0 International License (CC BY-NC-ND 4.0), which permits the non-commercial replication and distribution of the article with the strict proviso that no changes or edits are made and the original work is properly cited (including links to both the formal publication through the relevant DOI and the license). See: https://creativecommons.org/licenses/by-nc-nd/4.0/.
References
- Raghu G, Collard HR, Egan JJ, et al. An official ATS/ERS/JRS/ALAT statement: idiopathic pulmonary fibrosis: evidence-based guidelines for diagnosis and management. Am J Respir Crit Care Med 2011;183:788-824. [Crossref] [PubMed]
- King TE Jr, Bradford WZ, Castro-Bernardini S, et al. A phase 3 trial of pirfenidone in patients with idiopathic pulmonary fibrosis. N Engl J Med 2014;370:2083-92. [Crossref] [PubMed]
- Richeldi L, du Bois RM, Raghu G, et al. Efficacy and safety of nintedanib in idiopathic pulmonary fibrosis. N Engl J Med 2014;370:2071-82. [Crossref] [PubMed]
- Travis WD, Costabel U, Hansell DM, et al. An official American Thoracic Society/European Respiratory Society statement: Update of the international multidisciplinary classification of the idiopathic interstitial pneumonias. Am J Respir Crit Care Med 2013;188:733-48. [Crossref] [PubMed]
- Wolters PJ, Collard HR, Jones KD. Pathogenesis of idiopathic pulmonary fibrosis. Annu Rev Pathol 2014;9:157-79. [Crossref] [PubMed]
- Wang GG. Chromatin-based modulations underlying gene regulation and pathogenesis. FASEB J 2022;36: [Crossref]
- Medvedeva YA, Lennartsson A, Ehsani R, et al. EpiFactors: a comprehensive database of human epigenetic factors and complexes. Database (Oxford) 2015;2015:bav067. [Crossref] [PubMed]
- Zhang X, Liu H, Zhou JQ, et al. Modulation of H4K16Ac levels reduces pro-fibrotic gene expression and mitigates lung fibrosis in aged mice. Theranostics 2022;12:530-41. [Crossref] [PubMed]
- He H, Chen J, Zhao J, et al. PRMT7 targets of Foxm1 controls alveolar myofibroblast proliferation and differentiation during alveologenesis. Cell Death Dis 2021;12:841. [Crossref] [PubMed]
- Wang Q, Xie ZL, Wu Q, et al. Role of various imbalances centered on alveolar epithelial cell/fibroblast apoptosis imbalance in the pathogenesis of idiopathic pulmonary fibrosis. Chin Med J (Engl) 2021;134:261-74. [Crossref] [PubMed]
- Dor Y, Cedar H. Principles of DNA methylation and their implications for biology and medicine. Lancet 2018;392:777-86. [Crossref] [PubMed]
- Yang IV, Pedersen BS, Rabinovich E, et al. Relationship of DNA methylation and gene expression in idiopathic pulmonary fibrosis. Am J Respir Crit Care Med 2014;190:1263-72. [Crossref] [PubMed]
- Sanders YY, Ambalavanan N, Halloran B, et al. Altered DNA methylation profile in idiopathic pulmonary fibrosis. Am J Respir Crit Care Med 2012;186:525-35. [Crossref] [PubMed]
- Evans IC, Barnes JL, Garner IM, et al. Epigenetic regulation of cyclooxygenase-2 by methylation of c8orf4 in pulmonary fibrosis. Clin Sci (Lond) 2016;130:575-86. [Crossref] [PubMed]
- McErlean P, Bell CG, Hewitt RJ, et al. DNA Methylome Alterations Are Associated with Airway Macrophage Differentiation and Phenotype during Lung Fibrosis. Am J Respir Crit Care Med 2021;204:954-66. [Crossref] [PubMed]
- Li M, Zheng Y, Yuan H, et al. Effects of dynamic changes in histone acetylation and deacetylase activity on pulmonary fibrosis. Int Immunopharmacol 2017;52:272-80. [Crossref] [PubMed]
- Krämer OH, Zhu P, Ostendorff HP, et al. The histone deacetylase inhibitor valproic acid selectively induces proteasomal degradation of HDAC2. EMBO J 2003;22:3411-20. [Crossref] [PubMed]
- Coward WR, Watts K, Feghali-Bostwick CA, et al. Defective histone acetylation is responsible for the diminished expression of cyclooxygenase 2 in idiopathic pulmonary fibrosis. Mol Cell Biol 2009;29:4325-39. [Crossref] [PubMed]
- Dong W, Kong M, Zhu Y, et al. Activation of TWIST Transcription by Chromatin Remodeling Protein BRG1 Contributes to Liver Fibrosis in Mice. Front Cell Dev Biol 2020;8:340. [Crossref] [PubMed]
- Obrien CM, Cao YX, Gochuico BR, et al. Chromatin remodeling in the regulation of gene expression in idiopathic pulmonary fibrosis. Am J Respir Crit Care Med 2009;179:A1886.
- O'Dwyer DN, Armstrong ME, Trujillo G, et al. The Toll-like receptor 3 L412F polymorphism and disease progression in idiopathic pulmonary fibrosis. Am J Respir Crit Care Med 2013;188:1442-50. [Crossref] [PubMed]
- Shenderov K, Collins SL, Powell JD, et al. Immune dysregulation as a driver of idiopathic pulmonary fibrosis. J Clin Invest 2021;131:143226. [Crossref] [PubMed]
- Griffin GK, Wu J, Iracheta-Vellve A, et al. Epigenetic silencing by SETDB1 suppresses tumour intrinsic immunogenicity. Nature 2021;595:309-14. [Crossref] [PubMed]
- Lu J, Xu J, Li J, et al. FACER: comprehensive molecular and functional characterization of epigenetic chromatin regulators. Nucleic Acids Res 2018;46:10019-33. [Crossref] [PubMed]
- Sørensen OE, Borregaard N. Neutrophil extracellular traps - the dark side of neutrophils. J Clin Invest 2016;126:1612-20. [Crossref] [PubMed]
- Abi Abdallah DS, Denkers EY. Neutrophils cast extracellular traps in response to protozoan parasites. Front Immunol 2012;3:382. [Crossref] [PubMed]
- Suzuki A, Yamada R, Chang X, et al. Functional haplotypes of PADI4, encoding citrullinating enzyme peptidylarginine deiminase 4, are associated with rheumatoid arthritis. Nat Genet 2003;34:395-402. [Crossref] [PubMed]
- Suzuki M, Ikari J, Anazawa R, et al. PAD4 Deficiency Improves Bleomycin-induced Neutrophil Extracellular Traps and Fibrosis in Mouse Lung. Am J Respir Cell Mol Biol 2020;63:806-18. [Crossref] [PubMed]
- Eyre S, Bowes J, Diogo D, et al. High-density genetic mapping identifies new susceptibility loci for rheumatoid arthritis. Nat Genet 2012;44:1336-40. [Crossref] [PubMed]
- Miura Y, Ohkubo H, Niimi A, et al. Suppression of epithelial abnormalities by nintedanib in induced-rheumatoid arthritis-associated interstitial lung disease mouse model. ERJ Open Res 2021;7:e00345-2021. [Crossref] [PubMed]
- Ahmed S, Yamamoto K, Sato Y, et al. Proteolytic processing of IGFBP-related protein-1 (TAF/angiomodulin/mac25) modulates its biological activity. Biochem Biophys Res Commun 2003;310:612-8. [Crossref] [PubMed]
- Sato Y, Chen Z, Miyazaki K. Strong suppression of tumor growth by insulin-like growth factor-binding protein-related protein 1/tumor-derived cell adhesion factor/mac25. Cancer Sci 2007;98:1055-63. [Crossref] [PubMed]
- Akiel M, Guo C, Li X, et al. IGFBP7 Deletion Promotes Hepatocellular Carcinoma. Cancer Res 2017;77:4014-25. [Crossref] [PubMed]
- Liu LX, Huang S, Zhang QQ, et al. Insulin-like growth factor binding protein-7 induces activation and transdifferentiation of hepatic stellate cells in vitro. World J Gastroenterol 2009;15:3246-53. [Crossref] [PubMed]
- Watanabe J, Takiyama Y, Honjyo J, et al. Role of IGFBP7 in Diabetic Nephropathy: TGF-β1 Induces IGFBP7 via Smad2/4 in Human Renal Proximal Tubular Epithelial Cells. PLoS One 2016;11:e0150897. [Crossref] [PubMed]
- Velázquez-Enríquez JM, Santos-Álvarez JC, Ramírez-Hernández AA, et al. Proteomic Analysis Reveals Key Proteins in Extracellular Vesicles Cargo Associated with Idiopathic Pulmonary Fibrosis In Vitro. Biomedicines 2021;9:1058. [Crossref] [PubMed]
- Hsu E, Shi H, Jordan RM, et al. Lung tissues in patients with systemic sclerosis have gene expression patterns unique to pulmonary fibrosis and pulmonary hypertension. Arthritis Rheum 2011;63:783-94. [Crossref] [PubMed]
- Pietrasik S, Zajac G, Morawiec J, et al. Interplay between BRCA1 and GADD45A and Its Potential for Nucleotide Excision Repair in Breast Cancer Pathogenesis. Int J Mol Sci 2020;21:870. [Crossref] [PubMed]
- Arab K, Karaulanov E, Musheev M, et al. GADD45A binds R-loops and recruits TET1 to CpG island promoters. Nat Genet 2019;51:217-23. [Crossref] [PubMed]
- Hambly N, Shimbori C, Kolb M. Molecular classification of idiopathic pulmonary fibrosis: personalized medicine, genetics and biomarkers. Respirology 2015;20:1010-22. [Crossref] [PubMed]
- Boveda-Ruiz D, D'Alessandro-Gabazza CN, Toda M, et al. Differential role of regulatory T cells in early and late stages of pulmonary fibrosis. Immunobiology 2013;218:245-54. [Crossref] [PubMed]
- Xiong S, Guo R, Yang Z, et al. Treg depletion attenuates irradiation-induced pulmonary fibrosis by reducing fibrocyte accumulation, inducing Th17 response, and shifting IFN-γ, IL-12/IL-4, IL-5 balance. Immunobiology 2015;220:1284-91. [Crossref] [PubMed]
- Kotsianidis I, Nakou E, Bouchliou I, et al. Global impairment of CD4+CD25+FOXP3+ regulatory T cells in idiopathic pulmonary fibrosis. Am J Respir Crit Care Med 2009;179:1121-30. [Crossref] [PubMed]
- Salvador JM, Hollander MC, Nguyen AT, et al. Mice lacking the p53-effector gene Gadd45a develop a lupus-like syndrome. Immunity 2002;16:499-508. [Crossref] [PubMed]
- Salvador JM, Mittelstadt PR, Belova GI, et al. The autoimmune suppressor Gadd45alpha inhibits the T cell alternative p38 activation pathway. Nat Immunol 2005;6:396-402. [Crossref] [PubMed]
- Mathew B, Takekoshi D, Sammani S, et al. Role of GADD45a in murine models of radiation- and bleomycin-induced lung injury. Am J Physiol Lung Cell Mol Physiol 2015;309:L1420-9. [Crossref] [PubMed]
(English Language Editor: K. Brown)