Model development to predict central lymph node metastasis in cN0 papillary thyroid microcarcinoma by machine learning
Introduction
Thyroid cancer is the most pervasive malignant endocrine carcinoma worldwide, the incidence of which has significantly increased over the past decades (1-3). This surge is mainly due to the rising incidence of papillary thyroid cancer (PTC) (4), particularly papillary thyroid microcarcinomas (PTMC), which are defined as thyroid cancers of 1 cm or less in size (5). Although PTMC is viewed as an indolent tumor, central lymph node metastasis (CLNM) is significantly detected in PTMC patients (6,7).
According to the American Association of Endocrine Surgeons guidelines, therapeutic central compartment LN dissection (CLND) is recommended during initial thyroidectomy for PTMC patients with a clinical or image-detected nodal disease. However, implementing prophylactic CLND for low-risk PTMC remains controversial (8). The efficacy of preoperative neck ultrasound (US) to detect CLNM is limited due to the overlying thyroid gland (9). For PTMC, whether prophylactic CLND is performed during thyroidectomy often depends on the patient’s situation and the surgeon’s expertise. It is important to avoid overtreatment of prophylactic CLND because of surgical complications, including hypoparathyroidism and recurrent laryngeal nerve injury. On the other hand, simple thyroidectomy may contribute to disease recurrence (10), and second surgery is very difficult for cervical lymph node dissection. In recent years, some studies on the influencing factors of lymph node metastasis (LNM) have not excluded factors that have indications for lymph node resection, such as lateral LNM (7,11-14). Moreover, the conclusions of these studies are inconsistent. Therefore, it is clinically crucial to establish a reliable prediction model and identify CLNM before surgery for those patients who do not have strong surgical indications for lymphadenectomy.
Machine learning (ML) was created in 1959 by Arthur Samuel, whose main purpose was to introduce algorithms that take in input data, capitalize on computer analysis to predict output values within an acceptable range of accuracy, identify trends and patterns within the data, and ultimately learn from previous experience (15). ML in medicine can conduce to more accurate diagnostic algorithms and individual patient treatment (15-17). Hence, for better prediction of CLNM and individualized treatment in cN0 PTMC patients, this study was designed to investigate the influencing factors of CLNM and develop promising prediction models using ML algorithms. We present the following article in accordance with the TRIPOD reporting checklist (available at https://atm.amegroups.com/article/view/10.21037/atm-22-3594/rc).
Methods
Patients
This retrospective study was conducted in accordance with the Declaration of Helsinki (as revised in 2013). The study was approved by the ethics committee board of Xijing Hospital (XJLL-KY-20220428) and individual consent for this retrospective analysis was waived. A total of 1,209 PTMC patients who underwent initial thyroidectomy at the Department of Endocrine Surgery between January 2014 and December 2018 were enrolled. PTMC is diagnosed by preoperative ultrasonography and fine needle aspiration. Inclusion criteria were patients with preoperative diagnosis of PTMC. The exclusion criteria were as follows: Incomplete medical information, other types of thyroid carcinomas, absence of CLND, and evidence of central compartment LNM detected by preoperative US. Finally, 1,121 patients were included in the study.
Surgical strategy
In our institution, surgical decisions are made according to the American Thyroid Association guidelines. Lobectomy plus isthmusectomy with ipsilateral CLND was performed for patients with unilateral lobe PTC, and total thyroidectomy with bilateral CLND was performed for patients with bilateral PTC or PTC in the isthmus. A total of thyroidectomy plus ipsilateral CLND was performed for patients with extrathyroidal extension (ETE) tumor or multifocal tumors limited to a unilateral lobe.
Data collection
Clinical characteristics collected from clinical and pathological records included gender, age, family history of cancer, US characteristics, thyroid function test, and clinicopathological features. A family history of cancer was defined as any cancer occurring among the first- and second-degree relatives. US information included maximal tumor size, microcalcification, irregular shape, and ill-defined margin, while thyroid function test results included free tetraiodothyronine (FT4), free triiodothyronine (FT3), tetraiodothyronine (T4), triiodothyronine (T3), and thyroid-stimulating hormone (TSH). Clinicopathological features included Hashimoto thyroiditis (HT), bilateral lesions, multifocal/solitary lesions, capsule invasion, primary tumor ETE, and BRAFV600E mutation. Cervical US and thyroid function test were performed within one month before the surgery, and clinicopathological features were collected from intraoperative frozen section and postoperative pathological results. All patients were diagnosed with PTMC pre-operatively by fine-needle aspiration biopsy or intraoperatively using the frozen section procedure.
Statistical analyses
R software version 4.0.5 (R Foundation for Statistical Computing, Vienna, Austria) was used for statistical analyses. The Shapiro-Wilk test was used to test the normal distribution of quantitative data, and for non-normally distributed data, the median with interquartile range (IQR) was applied for expression. The student’s t-test was used to analyze quantitative parameters and the Pearson’s chi-square test was used to analyze discrete parameters. Multivariate logistic regression (LR) analysis with stepwise selection was used to assess the independent risk factors of CLNM.
We randomly divided the data into the test set (70%) and validation set (30%), which were used for model establishment and evaluation, respectively. Six types of ML algorithms models with all variables were developed in our study: LR, multivariate adaptive regression splines (MARS), decision tree (DT), random forest (RF), extreme gradient boosting (XGBoost), and neural network (NNET). We then calculated the area under the receiver operating characteristic (AUROC), sensitivity, specificity, accuracy, positive predictive value (PPV), and negative predictive value (NPV) of ML algorithms in the validation set. Relative importance ranking list was generated for each ML algorithm, and for the best-performing model, we calculated the error of different numbers of variables by ten cross validation to find the optimal number of variables. A two-sided P value <0.05 was considered statistically significant.
Results
Demographics features
The clinicopathological characteristics of 1,121 PTMC patients are shown in Table 1. Among them, 878 were female (78.3%), and 243 were male (21.7%), and the patients’ age at the time of initial treatment ranged from 16 to 73 years (median age 45 years; IQR: 37–51 years). The median tumor size was 0.7 (IQR: 0.5–0.8) cm, and 122 patients (10.9%) had a family history of cancer. Microcalcification was observed in 543 patients (48.4%) and an irregular tumor shape was detected in 819 (73.1%). A total of 798 patients (71.2%) had an ill-defined margin.
Table 1
Variables | Total (n=1,121) | CLNM(–) (n=745) | CLNM(+) (n=376) | P | Statistic |
---|---|---|---|---|---|
Gender, n (%) | <0.001 | 25.659 | |||
Female | 878 (78.3) | 617 (82.8) | 261 (69.4) | ||
Male | 243 (21.7) | 128 (17.2) | 115 (30.6) | ||
Age, median (IQR) | 45.0 (37.0, 51.0) | 46.0 (39.0, 52.0) | 42.0 (34.0, 49.0) | <0.001 | 34.821 |
Family history of cancer, n (%) | 0.6 | 0.275 | |||
No | 999 (89.1) | 667 (89.5) | 332 (88.3) | ||
Yes | 122 (10.9) | 78 (10.5) | 44 (11.7) | ||
Tumor size (cm), median (IQR) | 0.7 (0.5, 0.8) | 0.7 (0.5, 0.8) | 0.7 (0.6, 0.8) | <0.001 | 15.388 |
Microcalcification, n (%) | 0.007 | 7.317 | |||
No | 578 (51.6) | 406 (54.5) | 172 (45.7) | ||
Yes | 543 (48.4) | 339 (45.5) | 204 (54.3) | ||
Irregular shape, n (%) | 0.494 | 0.468 | |||
No | 302 (26.9) | 206 (27.7) | 96 (25.5) | ||
Yes | 819 (73.1) | 539 (72.3) | 280 (74.5) | ||
Margin, n (%) | 0.098 | 2.735 | |||
Smooth | 323 (28.8) | 227 (30.5) | 96 (25.5) | ||
Ill-defined | 798 (71.2) | 518 (69.5) | 280 (74.5) | ||
FT4 (pmol/L), median (IQR) | 16.5 (15.1, 18.1) | 16.5 (15.0, 18.0) | 16.5 (15.1, 18.2) | 0.39 | 0.739 |
FT3 (pmol/L), median (IQR) | 4.8 (4.4, 5.2) | 4.8 (4.4, 5.1) | 4.8 (4.5, 5.3) | <0.001 | 12.327 |
T4 (nmol/L), median (IQR) | 104.8 (93.0, 117.1) | 104.3 (92.8, 116.5) | 105.4 (93.5, 117.7) | 0.552 | 0.355 |
T3 (nmol/L), median (IQR) | 1.8 (1.6, 2.0) | 1.8 (1.6, 2.0) | 1.8 (1.6, 2.0) | 0.336 | 0.926 |
TSH (μIU/mL), median (IQR) | 2.2 (1.5, 3.2) | 2.2 (1.5, 3.2) | 2.2 (1.6, 3.2) | 0.637 | 0.222 |
Hashimoto thyroiditis, n (%) | 0.662 | 0.191 | |||
No | 951 (84.8) | 635 (85.2) | 316 (84.0) | ||
Yes | 170 (15.2) | 110 (14.8) | 60 (16.0) | ||
Bilateral lesions, n (%) | 0.103 | 2.655 | |||
No | 811 (72.3) | 551 (74.0) | 260 (69.1) | ||
Yes | 310 (27.7) | 194 (26.0) | 116 (30.9) | ||
Multifocal lesions, n (%) | 0.005 | 7.766 | |||
No | 674 (60.1) | 470 (63.1) | 204 (54.3) | ||
Yes | 447 (39.9) | 275 (36.9) | 172 (45.7) | ||
Capsule invasion, n (%) | 0.241 | 1.375 | |||
No | 1,055 (94.1) | 706 (94.8) | 349 (92.8) | ||
Yes | 66 (5.9) | 39 (5.2) | 27 (7.2) | ||
ETE, n (%) | 0.088 | 2.91 | |||
No | 1,068 (95.3) | 716 (96.1) | 352 (93.6) | ||
Yes | 53 (4.7) | 29 (3.9) | 24 (6.4) | ||
BRAF mutation, n (%) | 0.442 | 0.592 | |||
No | 322 (28.7) | 220 (29.5) | 102 (27.1) | ||
Yes | 799 (71.3) | 525 (70.5) | 274 (72.9) |
IQR, interquartile range; FT4, free tetraiodothyronine; FT3, free triiodothyronine; T4, tetraiodothyronine; T3, triiodothyronine; TSH, thyroid stimulating hormone; ETE, extrathyroidal extension; CLNM, central lymph node metastasis.
The median of FT4, FT3, T4, T3, and TSH were 16.5 (IQR: 15.1–18.1) pmol/L, 4.8 (IQR: 4.4–5.2) pmol/L, 104.8 (IQR: 93.0–117.1) nmol/L, 1.8 (IQR: 1.6–2.0) nmol/L, and 2.2 (IQR: 1.5–3.2) µIU/mL, respectively. The number of patients with HT was 170 (15.2%), and 310 (27.7%) had bilateral lesions, and 447 (39.9%) had multifocal lesions. Capsule invasion was present in 66 cases (5.9%), and primary tumor ETE was observed in 53 (4.7%). BRAFV600E mutation was detected in 799 patients (71.3%).
Univariate and multivariate analysis
Univariable analysis (Table 2) showed gender, age, tumor size, microcalcification, FT3, and multifocality were all significantly associated with the occurrence of CLNM in PTMC patients (P<0.05). In multivariate logistic regression analyses (Table 2), seven variables (gender, age, family history of cancer, tumor size, ill-defined margin, multifocal lesions, primary tumor ETE) were selected using stepwise selection. The results showed that male gender (OR =2.06, 95% CI: 1.53–2.79, P<0.001), tumor size (OR =3.58, 95% CI: 1.86–6.88), multifocal lesions (OR =1.56, 95% CI: 1.19–2.03, P=0.001), and ETE (OR =1.85, 95% CI: 1.03–3.31, P=0.039) were independent positive predictors of CLNM, while older age (OR =0.96, 95% CI: 0.94–0.97, P<0.001) was a negative predictor.
Table 2
Variable | Univariate analysis | Multivariate analysis | |||
---|---|---|---|---|---|
Crude OR 95% CI | Crude P value | Adj OR 95% CI | Adj P value | ||
Gender (Male) | 2.12 (1.59–2.84) | <0.001 | 2.06 (1.53–2.79) | <0.001 | |
Age | 0.96 (0.95–0.97) | <0.001 | 0.96 (0.94–0.97) | <0.001 | |
Family history of cancer (+/–) | 1.13 (0.77–1.68) | 0.532 | 1.45 (0.96–2.19) | 0.081 | |
Tumor size (cm) | 3.4 (1.83–6.32) | <0.001 | 3.58 (1.86–6.88) | <0.001 | |
Microcalcification (+/–) | 1.42 (1.11–1.82) | 0.006 | |||
Irregular shape (+/–) | 1.11 (0.84–1.48) | 0.45 | |||
Margin (Ill-defined/smooth) | 1.28 (0.97–1.69) | 0.085 | 1.27 (0.94–1.7) | 0.115 | |
FT4 (pmol/L) | 1.03 (0.98–1.08) | 0.295 | |||
FT3 (pmol/L) | 1.47 (1.21–1.8) | <0.001 | |||
T4 (nmol/L) | 1 (0.99–1.01) | 0.941 | |||
T3 (nmol/L) | 1.4 (0.97–2.02) | 0.07 | |||
TSH (μIU/mL) | 1.02 (0.94–1.09) | 0.667 | |||
Hashimoto thyroiditis (+/–) | 1.1 (0.78–1.54) | 0.599 | |||
Bilateral lesions (+/–) | 1.27 (0.96–1.67) | 0.089 | |||
Multifocal lesions (+/–) | 1.44 (1.12–1.85) | 0.004 | 1.56 (1.19–2.03) | 0.001 | |
Capsule invasion (+/–) | 1.4 (0.84–2.33) | 0.193 | |||
ETE (+/–) | 1.68 (0.97–2.93) | 0.066 | 1.85 (1.03–3.31) | 0.039 | |
BRAF mutation (+/–) | 1.13 (0.85–1.48) | 0.401 |
FT4, free tetraiodothyronine; FT3, free triiodothyronine; T4, tetraiodothyronine; T3, triiodothyronine; TSH, thyroid stimulating hormone; ETE, extrathyroidal extension; CLNM, central lymph node metastasis.
Predictive performance of ML algorithms
All 18 variables were used in CLNM predictive models based on six ML algorithms models, whose prediction performance is shown in Figure 1 and Table 3. The RF model demonstrated the most potential for predicting CLNM in PTMC patients, showing the highest AUROC (0.794, 95% CI: 0.744–0.844) and highest accuracy (0.772). Threshold values of AUC in RF model is 0.423 and the sensitivity and specificity of this model were 0.564 and 0.872. Consequently, the RF model was selected as the prediction model in this study.
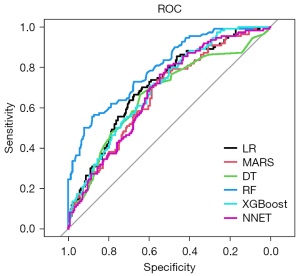
Table 3
Methods | AUROC (95% CI) | Sensitivity | Specificity | Accuracy | PPV | NPV |
---|---|---|---|---|---|---|
LR | 0.709 (0.650–0.765) | 0.664 | 0.674 | 0.671 | 0.497 | 0.805 |
MARS | 0.668 (0.608–0.728) | 0.773 | 0.520 | 0.602 | 0.438 | 0.825 |
DT | 0.664 (0.601–0.728) | 0.664 | 0.643 | 0.650 | 0.474 | 0.798 |
RF | 0.794 (0.744–0.844) | 0.564 | 0.872 | 0.772 | 0.681 | 0.805 |
XGBoost | 0.699 (0.642–0.756) | 0.700 | 0.595 | 0.629 | 0.456 | 0.804 |
NNET | 0.678 (0.619–0.736) | 0.791 | 0.515 | 0.605 | 0.442 | 0.836 |
LR, logistic regression; MARS, multivariate adaptive regression splines; DT, decision tree; RF, random forest; XGBoost, extreme gradient boosting; NNET, neural network; AUROC, area under the receiver operating characteristic; PPV, positive predictive value; NPV, negative predictive value.
Variable importance
The relative importance of the ten most important predictors of the six models is summarized in Figure 2. Notably, age, tumor size, gender, and multifocality were ranked in the top 10 of all six models. The errors of RF reached the lowest when all 18 variables were introduced (Figure 3).
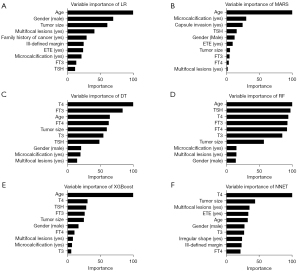
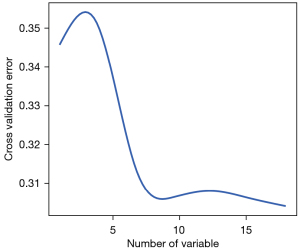
Web-based calculator
An online calculator based on the RF model was established to predict a patient’s risk of CLNM by simply entering clinical variables (Figure 4).
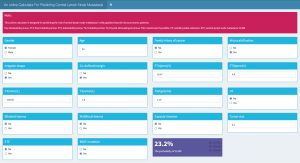
Discussion
The incidence of PTMC has increased rapidly in the last few decades (5). According to previous studies, CLNM is an important risk factor in the recurrence of PTMC but is rarely identified clinically (18-20). The study conducted by Qu et al. demonstrated CLNM prevalence ranged between 17.8% and 64.1% (6), while in this study, the CLNM incidence in cN0 PTMC was 33.5% (376/1,121). Although it is widely accepted that CLND should be performed in patients with positive clinical CLNM, for PTMC patients without preoperative and intraoperative suspected CLNM, the need for CLND remains controversial (21,22) and mostly relies on the surgeon’s experience. Several studies have aimed to recognize predictive factors associated with CLNM in cN0 PTMC patients, but the results were inconsistent (23-25). Although ML algorithms are gradually being used for clinical decisions, few studies utilized them to establish a CLNM prediction model (16,17). Therefore, besides univariate and multivariate analysis, we developed and internally validated multiple models for predicting CLNM in cN0 PTMC patients using ML algorithms.
Herein, a retrospective cohort of 1,121 PTMC patients was assessed. A total of 18 variables, including clinicopathological features and US characteristics, were collected for analysis and constructing prediction models, and multivariate analysis showed tumor size, male, young age, multifocality, and ETE were highly predictive of CLNM. This is similar to several previous studies (11,26), while it was not completely consistent with some reports. Siddiqui’s et al. revealed that male and tumor size were not related to CLNM (27), while in Yang et al.’s study, age had no significant correlation with CLNM (28). Microcalcification, FT3, and T3 correlated with CLNM in the univariate analysis but had no significance after cofounding adjustment in multivariate analysis. Recent research verified BRAFV600E mutation was associated with CLNM (23,28,29), but Kim et al. reported it was not an indicator of CLNM (30). In our study, no significant correlation was seen between BRAF mutation and CLNM.
Compared with previous studies predicting the risk of CLNM in PTMC (7,11), our work focused on cN0 PTMC patients. Although ML in medicine has gradually become a hot topic in recent years (31), to the best of our knowledge, this is the first study to develop a prediction model based on ML algorithms for real-time risk evaluation of CLNM in cN0 PTMC patients. Herein, we used six different ML algorithms to establish CLNM prediction models and show the importance of variables in them. Although the importance of variables in different models was not completely consistent, age, tumor size gender, and multifocal lesions were always highly ranked. Some variables, such as FT4, T3, and T4, showed no significance in univariate or multivariate analysis but occupied important positions in some ML models. According to AUROC values (0.794) and the accuracy (0.772) of the models, RF was selected as the best predictive model, and has achieved better performance than traditional methods used by previous studies (23,32,33). Furthermore, to make this ML-based model easier to use, we built an online application based on it, and clinicians can now promote personalized surgical treatment by calculating the risks for each patient.
There are several limitations to this study. Firstly, our work is a single-center retrospective observational study. Hence the effectiveness of the models has only been verified internally, and a potential selection bias exists. Secondly, some variables in the models are based on frozen biopsy and postoperative pathological diagnosis, which might restrict the ability to detect CLNM before surgery. From our point of view, pathological results are more stable and consistent than US results. The clinicopathology features in our model may be collected by fine-needle aspiration and frozen sections before or during surgery in the future. Thirdly, our study lacks long follow-up data to verify the prognosis of the patients. Finally, calculated according to the cut-off value we gave, the RF model has high accuracy but low sensitivity. However, this model still has high potential clinical application value. Obviously, when the accuracy is certain, the sensitivity and accuracy cannot reach a high level at the same time. Although this model has low sensitivity, it has a high PPV. Therefore, considering that surgery may cause damage to nerves or the thoracic duct, for surgical teams lacking nerve detectors, after evaluated by this predictive model, patients with higher predictive values were offered prophylactic lymphadenectomy. In patients with low levels, the surgeon can make an intraoperative decision whether to prophylactically remove the lymph nodes. Despite these shortcomings, our study is the first to apply ML algorithms to CLNM prediction in cN0 PTMC patients and may offer a basis for future clinical treatment.
Conclusions
This study developed and validated ML algorithms for individualized risk prediction of CLNM in cN0 PTMC patients. Our ML-based prediction model and its accompanying online risk calculator can provide clinicians and researchers with a simple tool to make precise surgical decisions. In the future, we aim to incorporate multiple biological variables, including imaging, molecular, genetic, and immune microenvironment data, to further improve the model’s performance.
Acknowledgments
Funding: None.
Footnote
Reporting Checklist: The authors have completed the TRIPOD reporting checklist. Available at https://atm.amegroups.com/article/view/10.21037/atm-22-3594/rc
Data Sharing Statement: Available at https://atm.amegroups.com/article/view/10.21037/atm-22-3594/dss
Conflicts of Interest: All authors have completed the ICMJE uniform disclosure form (available at https://atm.amegroups.com/article/view/10.21037/atm-22-3594/coif). The authors have no conflicts of interest to declare.
Ethical Statement: The authors are accountable for all aspects of the work in ensuring that questions related to the accuracy or integrity of any part of the work are appropriately investigated and resolved. This retrospective study was conducted in accordance with the Declaration of Helsinki (as revised in 2013). The study was approved by the ethics committee board of Xijing Hospital (XJLL-KY-20220428) and individual consent for this retrospective analysis was waived.
Open Access Statement: This is an Open Access article distributed in accordance with the Creative Commons Attribution-NonCommercial-NoDerivs 4.0 International License (CC BY-NC-ND 4.0), which permits the non-commercial replication and distribution of the article with the strict proviso that no changes or edits are made and the original work is properly cited (including links to both the formal publication through the relevant DOI and the license). See: https://creativecommons.org/licenses/by-nc-nd/4.0/.
References
- Bray F, Ferlay J, Soerjomataram I, et al. Global cancer statistics 2018: GLOBOCAN estimates of incidence and mortality worldwide for 36 cancers in 185 countries. CA Cancer J Clin 2018;68:394-424. [Crossref] [PubMed]
- Deng Y, Li H, Wang M, et al. Global Burden of Thyroid Cancer From 1990 to 2017. JAMA Netw Open 2020;3:e208759. [Crossref] [PubMed]
- Zhai M, Zhang D, Long J, et al. The global burden of thyroid cancer and its attributable risk factor in 195 countries and territories: A systematic analysis for the Global Burden of Disease Study. Cancer Med 2021;10:4542-54. [Crossref] [PubMed]
- La Vecchia C, Malvezzi M, Bosetti C, et al. Thyroid cancer mortality and incidence: a global overview. Int J Cancer 2015;136:2187-95. [Crossref] [PubMed]
- Brito JP, Hay ID. Management of Papillary Thyroid Microcarcinoma. Endocrinol Metab Clin North Am 2019;48:199-213. [Crossref] [PubMed]
- Qu N, Zhang L, Ji QH, et al. Risk Factors for Central Compartment Lymph Node Metastasis in Papillary Thyroid Microcarcinoma: A Meta-Analysis. World J Surg 2015;39:2459-70. [Crossref] [PubMed]
- Wang Y, Guan Q, Xiang J. Nomogram for predicting central lymph node metastasis in papillary thyroid microcarcinoma: A retrospective cohort study of 8668 patients. Int J Surg 2018;55:98-102. [Crossref] [PubMed]
- Patel KN, Yip L, Lubitz CC, et al. The American Association of Endocrine Surgeons Guidelines for the Definitive Surgical Management of Thyroid Disease in Adults. Ann Surg 2020;271:e21-93. [Crossref] [PubMed]
- Hwang HS, Orloff LA. Efficacy of preoperative neck ultrasound in the detection of cervical lymph node metastasis from thyroid cancer. Laryngoscope 2011;121:487-91. [Crossref] [PubMed]
- Miller JE, Al-Attar NC, Brown OH, et al. Location and Causation of Residual Lymph Node Metastasis After Surgical Treatment of Regionally Advanced Differentiated Thyroid Cancer. Thyroid 2018;28:593-600. [Crossref] [PubMed]
- Sun J, Jiang Q, Wang X, et al. Nomogram for Preoperative Estimation of Cervical Lymph Node Metastasis Risk in Papillary Thyroid Microcarcinoma. Front Endocrinol (Lausanne) 2021;12:613974. [Crossref] [PubMed]
- Zhang Q, Wang Z, Meng X, et al. Predictors for central lymph node metastases in CN0 papillary thyroid microcarcinoma (mPTC): A retrospective analysis of 1304 cases. Asian J Surg 2019;42:571-6. [Crossref] [PubMed]
- Zhang L, Wei WJ, Ji QH, et al. Risk factors for neck nodal metastasis in papillary thyroid microcarcinoma: a study of 1066 patients. J Clin Endocrinol Metab 2012;97:1250-7. [Crossref] [PubMed]
- Lin DZ, Qu N, Shi RL, et al. Risk prediction and clinical model building for lymph node metastasis in papillary thyroid microcarcinoma. Onco Targets Ther 2016;9:5307-16. [Crossref] [PubMed]
- Handelman GS, Kok HK, Chandra RV, et al. eDoctor: machine learning and the future of medicine. J Intern Med 2018;284:603-19. [Crossref] [PubMed]
- Wu Y, Rao K, Liu J, et al. Machine Learning Algorithms for the Prediction of Central Lymph Node Metastasis in Patients With Papillary Thyroid Cancer. Front Endocrinol (Lausanne) 2020;11:577537. [Crossref] [PubMed]
- Zhu J, Zheng J, Li L, et al. Application of Machine Learning Algorithms to Predict Central Lymph Node Metastasis in T1-T2, Non-invasive, and Clinically Node Negative Papillary Thyroid Carcinoma. Front Med (Lausanne) 2021;8:635771. [Crossref] [PubMed]
- Wada N, Duh QY, Sugino K, et al. Lymph node metastasis from 259 papillary thyroid microcarcinomas: frequency, pattern of occurrence and recurrence, and optimal strategy for neck dissection. Ann Surg 2003;237:399-407. [Crossref] [PubMed]
- Chow SM, Law SC, Chan JK, et al. Papillary microcarcinoma of the thyroid-Prognostic significance of lymph node metastasis and multifocality. Cancer 2003;98:31-40. [Crossref] [PubMed]
- Hay ID, Hutchinson ME, Gonzalez-Losada T, et al. Papillary thyroid microcarcinoma: a study of 900 cases observed in a 60-year period. Surgery 2008;144:980-7; discussion 987-8. [Crossref] [PubMed]
- Agcaoglu O, Sengun B, Ozoran E, et al. Should we perform routine prophylactic central neck dissection in patients with thyroid papillary microcarcinoma? Ann Ital Chir 2018;89:485-8. [PubMed]
- Chang YW, Kim HS, Kim HY, et al. Should central lymph node dissection be considered for all papillary thyroid microcarcinoma? Asian J Surg 2016;39:197-201. [Crossref] [PubMed]
- Chen BD, Zhang Z, Wang KK, et al. A multivariable model of BRAFV600E and ultrasonographic features for predicting the risk of central lymph node metastasis in cN0 papillary thyroid microcarcinoma. Cancer Manag Res 2019;11:7211-7. [Crossref] [PubMed]
- Liu LS, Liang J, Li JH, et al. The incidence and risk factors for central lymph node metastasis in cN0 papillary thyroid microcarcinoma: a meta-analysis. Eur Arch Otorhinolaryngol 2017;274:1327-38. [Crossref] [PubMed]
- Yan B, Hou Y, Chen D, et al. Risk factors for contralateral central lymph node metastasis in unilateral cN0 papillary thyroid carcinoma: A meta-analysis. Int J Surg 2018;59:90-8. [Crossref] [PubMed]
- Yu X, Song X, Sun W, et al. Independent Risk Factors Predicting Central Lymph Node Metastasis in Papillary Thyroid Microcarcinoma. Horm Metab Res 2017;49:201-7. [Crossref] [PubMed]
- Siddiqui S, White MG, Antic T, et al. Clinical and Pathologic Predictors of Lymph Node Metastasis and Recurrence in Papillary Thyroid Microcarcinoma. Thyroid 2016;26:807-15. [Crossref] [PubMed]
- Yang Y, Chen C, Chen Z, et al. Prediction of central compartment lymph node metastasis in papillary thyroid microcarcinoma. Clin Endocrinol (Oxf) 2014;81:282-8. [Crossref] [PubMed]
- Lang BH, Chai YJ, Cowling BJ, et al. Is BRAFV600E mutation a marker for central nodal metastasis in small papillary thyroid carcinoma? Endocr Relat Cancer 2014;21:285-95. [Crossref] [PubMed]
- Kim SK, Woo JW, Lee JH, et al. Role of BRAF V600E mutation as an indicator of the extent of thyroidectomy and lymph node dissection in conventional papillary thyroid carcinoma. Surgery 2015;158:1500-11. [Crossref] [PubMed]
- Deo RC. Machine Learning in Medicine. Circulation 2015;132:1920-30. [Crossref] [PubMed]
- Huang C, Cong S, Shang S, et al. Web-Based Ultrasonic Nomogram Predicts Preoperative Central Lymph Node Metastasis of cN0 Papillary Thyroid Microcarcinoma. Front Endocrinol (Lausanne) 2021;12:734900. [Crossref] [PubMed]
- Feng JW, Ye J, Wu WX, et al. Management of cN0 papillary thyroid microcarcinoma patients according to risk-scoring model for central lymph node metastasis and predictors of recurrence. J Endocrinol Invest 2020;43:1807-17. [Crossref] [PubMed]