The non-coding RNA (ncRNA)-mediated high expression of polycomb group factor 1 (PCGF1) is a prognostic biomarker and is correlated with tumor immunity infiltration in liver hepatocellular carcinoma
Introduction
Liver hepatocellular carcinoma (LIHC) is common worldwide and has a poor prognosis, which places a huge burden on the global public health system, especially in China (1,2). In 2018, there were about 841,300 newly diagnosed liver cancer cases and 782,000 deaths, accounting for nearly 4.7% of all new cancer patients and 8.2% of all cancer deaths (3). LIHC accounts for more than 75% of new liver cancer cases and over 50% of those cases occur in China (4,5).
The leading risk factors for LIHC vary and include hepatitis B virus (HBV), hepatitis C virus (HCV), food contaminated with aflatoxin, long-term smoking, alcohol abuse, type 2 diabetes, and obesity (3,5). The influence of these major risk factors varies by region. In China and Eastern Africa, chronic HBV infection and frequent aflatoxin exposure are the main causes of LIHC, while in Japan and Egypt, HCV infection is the main cause of LIHC (1-3).
The curative treatment regimens for LIHC include surgery and radiofrequency ablation (5-7), but these are only applicable to patients with early-stage LIHC. Indeed, curative surgery is not suitable for advanced LIHC patients, for whom the standard of care includes transarterial chemoembolization, radiotherapy, and systemic therapy, such as chemotherapy, targeted therapy, and new immunotherapeutic agents (8-11). In recent decades, due to the implementation of a comprehensive multidisciplinary approach, the survival rate of LIHC patients has improved. However, the survival rate of LIHC is still lower than expected, as some patients show multicentric recurrence after curative treatment (5,11,12). Thus, novel therapeutic targets and promising prognostic biomarkers need to be identified for LIHC.
Polycomb group factor 1 (PCGF1), which is also known as nervous system polycomb1 (NSPc1), was first identified in 2001 (13,14). PCGF1 is a novel mammalian polycomb gene, belongs to the polycomb group (PcG) protein family, and plays a significant role in the development of the nervous system (15). The PcG protein family forms multiprotein complexes, which are classified as polycomb repressive complex 1 (PRC1) and polycomb repressive complex 2 (PRC2) (16-18). These complexes function by suppressing gene transcription through epigenetic remodeling and were originally identified in drosophila melanogaster (16-18). Among the various PCGF proteins, PRC1 is typically involved in canonical polycomb repressive complex 1 (cPRC1) and non-canonical polycomb repressive complex 1 (ncPRC1) (16,19). As one of the members of the PRC1, PCGF1 is a protein coding gene and is overexpressed in the developing nervous system (15). The RING finger domain of PCGF1 is 93% homologous to Bmi1 (PCGF4) (13,18,20). Thus, PCGF1 may have similar functions to Bmil, as it shares the same domain architecture (21).
Previous studies on PCGF1 have focused on the developing nervous system and stem cell function (22). The abnormal expression of PCGF1 has been implicated in cancer stem cell phenotypes and has been shown to promote tumorigenesis in various types of cancers (21,22). PCGF1 was shown to be overexpressed in colorectal cancer (CRC) and was linked to cancer progression and a poor prognosis (14). In CRC, PCGF1 contributes to stem cell enrichment and induces the activation of stem cell biomarkers (14). Research has shown that the downregulation of PCGF1 in gliomas leads to the inactivation of the c-myc signaling pathway and reduces cell proliferation (15). PCGF1 expression is increased in several cancer types (14) and regulates the ability of stem cell self-renewal by targeting retinol dehydrogenase 16 (RDH16) in glioma cells (21); however, to date, no studies have explored its prognostic and diagnostic value and the regulatory network of PCGF1 in LIHC. Additionally, the expression, prognosis, and mechanism of PCGF1, and the role of tumor immune infiltration in LIHC remain unclear.
In this research, we performed PCGF1 expression and survival analyses in human cancer. We also investigated the regulation of micro ribonucleic acids (miRNAs) and long non-coding RNAs (lncRNAs) related to PCGF1 in LIHC. Finally, we explored the value of PCGF1 in immune cells. In summary, we found links between non-coding RNA (ncRNA) and the upregulation of PCGF1, which may serve as a biomarker and regulate immune cell infiltration in LIHC. We present the following article in accordance with the TRIPOD reporting checklist (available at https://atm.amegroups.com/article/view/10.21037/atm-22-3862/rc).
Methods
RNA-seq data source availability and ethics statement
PCGF1 expression levels in 20 cancers were identified in The Cancer Genome Atlas (TCGA) database (https://portal.gdc.cancer.gov/). The RNA sequencing (RNA-seq) transcriptomic data [level 3 HTSeq—fragments per kilobase per Million (FPKM)] were converted to transcripts per million reads (TPM) format and log2 values for the study (23). Additionally, clinical information was retained, and duplicate samples were removed. Subsequently, we also extracted the PCGF1 data from the University of California Santa Cruz (UCSC) XEUC database (https://xenabrowser.net/datapages/), RNA-seq data in TPM format from TCGA, and genotype-tissue expression (GTEx) data uniformly processed by the Toil process (24). The RNA-seq data in the TPM format of TCGA cancer samples and the corresponding normal tissues of the GTEX were extracted, and the expression levels of the samples after log2 transformation were compared. The miRNA data for LIHC were also extracted from TCGA. According to the median expression level of PCGF1, all the data were divided into high and low expression groups. The study was conducted in accordance with the Declaration of Helsinki (as revised in 2013).
Survival analysis of PCGF1 in cancers
A Kaplan-Meier (KM) analysis was conducted to determine the prognostic value of the PCGF1 characteristics and survival. We also extracted the survival data and clinical information of cancer patients from an article for subsequent analysis (25).
StarBase database analysis for candidate miRNA and lncRNA prediction
The StarBase database (http://starbase.sysu.edu.cn/) is widely used to determine target-miRNA interactions (26). We used it to analyze the correlations between ncRNA and gene expression in LIHC. We first predicted the upstream binding miRNAs of PCGF1 and confirmed the candidate miRNA-PCGF1 to predict the potential lncRNAs that might bind to miRNA-pcGF1. We also examined the correlations between lncRNA, miRNA, and PCGF1 expression in LIHC. Additionally, TCGA data were used to test the relationship between the expression levels and clinical outcomes using the R tool (version 3.6.3).
Correlations with PCGF1 expression and its clinical value in LIHC
Wilcoxon rank-sum tests and a logistic analysis were conducted to analyze the correlations between clinicopathological features and PCGF1 expression (27). The receiver operating characteristic (ROC) curve showed that PCGF1 had a good predictive value. Cox regression modeling was carried out to identify prognostic factors. Using the “rms” package of R (version 3.6.3) and a multivariate Cox model, a nomogram for predicting 1-, 3- and 5-year survival was established. The calibration curve was established, and the prediction probability of the nomogram was estimated. The consistency index (C-index) was calculated to evaluate the prediction accuracy of the nomogram.
Enrichment analysis and gene set enrichment analysis (GSEA)
Differentially expressed genes (DEGs) with a |log fold change (FC)| value >1.5 were identified using R language-related software DESeq2 (version 1.26.0) (27), and an adjusted P value less than 0.05 was set as the cut-off value for DEG identification. The “ClusterProfiler” software package was used to conduct the Gene Ontology (GO) enrichment and Kyoto Encyclopedia of Genes and Genomes (KEGG) pathway analyses, and “ggplot2” software package was used to visualize the results (28). A GSEA (29) was conducted using R package ClusterProfiler (Version 3.14.3) (28) to confirm the significant functions and pathways (30). The terms with an adjusted P value less than 0.05 and a false discovery rate (FDR) value less than 0.25 were selected.
Immune infiltration analysis in LIHC
A single-sample GSEA (ssGSEA) was performed with the GSVA package (version 1.34.0) (31), and the infiltration of 24 immune cells was estimated according to the inferred immune characteristics (32). The correlations between PCGF1 expression and these immune cells were evaluated by calculating the Pearson’s correlation coefficients. The enrichment scores of the immune cells in the related samples were compared using the Wilcoxon rank sum test. A P value less than 0.05 was considered statistically significant.
Statistical analysis
R Statistics Software Package (version 3.6.3) was used for the statistical analysis. In the PCGF1 expression analysis, the Wilcoxon rank-sum test was used to compare the expression levels of PCGF1 in the tumor and normal tissues. The PCGF1 expression levels were defined as high or low based on the median value. The relationship between PCGF1 and clinicopathology was analyzed using the Wilcoxon rank-sum test and a logistic regression analysis. We conducted a Cox regression analysis to confirm the effects of the clinicopathology variables on survival time. A P value less than 0.05 was considered statistically significant.
Results
Expression levels of PCGF1 in cancers
We used TCGA data to investigate the expression levels of PCGF1 and its predictive value in different cancers. As Figure 1A shows, PCGF1 was more upregulated in tumor tissues than normal tissues, including LIHC, bladder urothelial carcinoma, kidney renal clear cell carcinoma (KIRC), cholangiocarcinoma, stomach adenocarcinoma (STAD), colon adenocarcinoma (COAD), head and neck squamous cell carcinoma, breast invasive carcinoma (BRCA), kidney renal papillary cell carcinoma (KIRC), lung adenocarcinoma (LUAD), thyroid carcinoma (THCA), lung squamous cell carcinoma (LUSC), pheochromocytoma and paraganglioma (PCPG), prostate adenocarcinoma (PRAD), rectum adenocarcinoma (READ), and uterine corpus endometrial carcinoma (UCEC) (P<0.05), and was only downregulated in kidney chromophobe (KICH). However, no significant difference in PCGF1 expression was observed in cervical squamous cell carcinoma and endocervical adenocarcinoma (CESC), and pancreatic adenocarcinoma (PAAD) (P>0.05; see Figure 1A). Further, we extracted the PCGF1 data from the UCSC XENA database (http://xena.ucsc.edu/) and found that PCGF1 was also significantly more upregulated in LIHC, COAD READ, STAD, PAAD, UCEC, LUSC, PRAD, ESCA, KIRC, BRCA, CESC, and LUAD compared to TCGA and the GTEx corresponding normal controls (P<0.05; see Figure 1B-1M). To sum up, we found that PCGF1 was overexpressed in several cancers, and it may be a key driver of carcinogenesis in cancer.

The survival analysis data of PCGF1 in cancer patients
To explore the predictive value of PCGF1, a KM plotter analysis was conducted of different cancers, mainly using TCGA data sets. Overall survival (OS), the progress-free interval (PFI), and disease-specific survival (DSS) were examined. As Figure 2A-2F show, the high expression of PCGF1 was associated with a poor prognosis in terms of OS, PFI, and disease-free survival (DFS) in LIHC (P<0.05; see Figure 2A-2C) and COAD-READ (P<0.05; see Figure 2D-2F). Next, we divided the COAD-READ cases into COAD and READ and explored the relationship between PCGF1 expression and prognosis. The OS analysis showed that the high expression of PCGF1 was associated with a poor prognosis in COAD patients (P=0.027; see Figure 2G). However, PCGF1 had no significant effect on prognosis in READ patients (P=0.053; see Figure 2H). Similar results were also observed in other cancers (P>0.05; see Figure S1A-S1J). These data suggest that PCGF1 is related to a poor prognosis and can be used as a biomarker of LIHC.
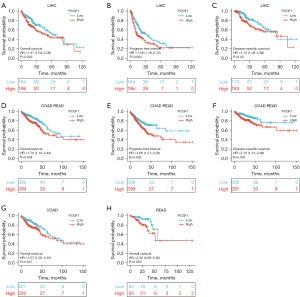
Predicted upstream miRNAs of PCGF1 in LIHC
There is increasing evidence that ncRNAs are actively involved in gene expression regulation (33). Thus, we sought to determine whether PCGF1 was also regulated by ncRNAs. First, we used the StarBase database to predict the upstream miRNAs of PCGF1. A total of 16 possible upstream miRNAs were predicted to potentially target PCGF1. For better visualization, a miRNA-PCGF1 regulatory network was created using Cytoscape software (see Figure 3A). As a negative regulator of gene expression, miRNA plays an important role in many biological processes. As Figure 3B shows, among all the upstream miRNAs, hsa-miR-22-3p was negatively correlated with PCGF1, and hsa-miR-23c was positively correlated with PCGF1 in LIHC. Subsequently, we evaluated the expression and prognostic value of hsa-miR-22-3p in LIHC using TCGA data, which was more overexpressed in normal tissues than LIHC tissues (P<0.05; see Figure 3C) and linked to unfavorable DSS (P=0.002; see Figure 3D), the PFI (P=0.001; see Figure 3E), and OS (P<0.001; see Figure 3F). Thus, upstream hsa-miR-22-3p appears to act as tumor suppressive miRNA, and the hsa-miR-22-3p-PCGF1 axis may be the potential pathway in LIHC.
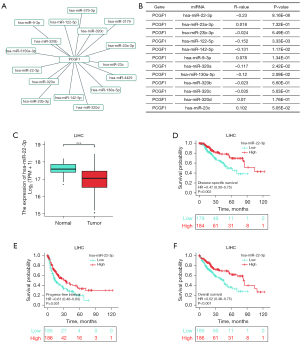
Upstream lncRNAs of hsa-miR-22-3p in LIHC
LncRNAs act as competitive endogenous RNAs (ceRNAs) and interact with messenger RNAs (mRNAs) via the miRNA binding sites. Next, to ascertain whether hsa-miR-22-3p was modulated by some lncRNAs, we used the StarBase database to examine the upstream lncRNAs of hsa-miR-22-3p. The regulatory network of lncRNA and hsa-miR-22-3p is shown in Figure 4. We used TCGA data sets to examine the expression levels and predictive values of selected lncRNAs. Only 4 lncRNAs (i.e., LINC01184, AC016405.3, BX284668., and MIR4435-2HG) were significantly higher in LIHC (P<0.001; see Figure 5A) and were correlated with a poorer OS (P<0.05; see Figure 5B-5E). Additionally, overexpressed MIR4435-2HG indicated poor DSS (P=0.011; see Figure 5F), and high levels of LINC01184 (P=0.02; see Figure 5G) and AC016405.3 (P=0.035; see Figure 5H) were linked to a poor PFI in LIHC. Taken together, based on the classical sponge theory/competitive ceRNA hypothesis, these results indicate that lncRNA levels are inversely linked to miRNA levels and positively linked to mRNA levels. We used the StarBase database to examine the correlation between 4 lncRNAs and hsa-miR-22-3p or PCGF1 expression in LIHC (see Table 1). We found that AC016405.3, BX284668.6 and MIR4435-2HG downregulate hsa-miR-22-3p, and increase the expression of PCGF1 in LIHC.
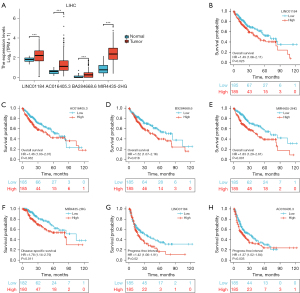
Table 1
miRNA/PCGF1 | LncRNA | R value | P value |
---|---|---|---|
miRNA | |||
hsa-miR-22-3p | LINC01184 | 0.003 | 9.55E–01 |
hsa-miR-22-3p | AC016405.3 | −0.323 | 1.89E–10 |
hsa-miR-22-3p | BX284668.6 | −0.186 | 3.26E–04 |
hsa-miR-22-3p | MIR4435-2HG | −0.168 | 1.16E–03 |
Gene | |||
PCGF1 | LINC01184 | 0.073 | 1.57E–01 |
PCGF1 | AC016405.3 | 0.291 | 9.59E–09 |
PCGF1 | BX284668.6 | 0.224 | 1.20E–05 |
PCGF1 | MIR4435-2HG | 0.3 | 3.08E–09 |
lncRNA, long non-coding RNA; miRNA, micro ribonucleic acid; PCGF1, polycomb group factor 1; LIHC, liver hepatocellular carcinoma.
Correlation between PCGF1 expression and clinical characteristics in LIHC patients
To investigate the relationship between PCGF1 expression and the clinical features of LIHC, we compared the clinical features of 371 patients with LIHC and divided them into high and low PCGF1 expression groups (see Table 2). As Table 2 and Figure 6A-6E show, the high expression of PCGF1 is closely related to tumor (T) stage (T1 vs. T2, T3, & T4, P<0.001), pathologic stage (Stage I vs. Stages II, III, & IV, P=0.001), vascular invasion (no vs. yes, P=0.012), histologic grade (G1 & G2 vs. G3 & G4, P=0.002), and alpha fetoprotein (AFP) level (≤400 vs. >400 ng/mL, P=0.045). Additionally, a statistically significant correlation was found with node (N) stage, metastasis (M) stage, Child-Pugh grade, age, gender, residual tumor, and race in PCGF1-high (P>0.05, see Figure S2A-2G). To further confirm that the expression of PCGF1 was associated with the poor prognosis of LIHC patients, we performed a logistic regression analysis (see Table 3). High PCGF1 expression levels were positively correlated with pathologic stage [Stages I, II, & IV vs. Stage I, OR: 2.239, 95% confidence interval (CI): 1.461–3.451, P=0.001], histologic grade (G3 & G4 vs. G1 & G2, OR: 1.606, 95% CI: 1.047–2.473, P=0.030), AFP level (>400 vs. ≤400 ng/mL, OR: 1.878, 95% CI: 1.070–3.345, P=0.030), and vascular invasion (yes vs. no, OR: 1.731, 95% CI: 1.085–2.777, P=0.022).
Table 2
Characteristics | Low expression of PCGF1 (N=185) | High expression of PCGF1 (N=186) | P value |
---|---|---|---|
Age, median (IQR) | 61.5 (51, 69) | 61 (52, 68) | 0.759 |
Age, n (%) | 0.914 | ||
≤60 | 87 (23.5) | 90 (24.3) | |
>60 | 97 (26.2) | 96 (25.9) | |
Gender, n (%) | 0.130 | ||
Female | 53 (14.3) | 68 (18.3) | |
Male | 132 (35.6) | 118 (31.8) | |
Race, n (%) | 0.204 | ||
Asian | 73 (20.3) | 85 (23.7) | |
Black or African American | 7 (1.9) | 10 (2.8) | |
White | 101 (28.1) | 83 (23.1) | |
T stage, n (%) | 0.001 | ||
T1 | 109 (29.6) | 72 (19.6) | |
T2 | 35 (9.5) | 59 (16.0) | |
T3 | 34 (9.2) | 46 (12.5) | |
T4 | 5 (1.4) | 8 (2.2) | |
N stage, n (%) | 0.622 | ||
N0 | 125 (48.8) | 127 (49.6) | |
N1 | 1 (0.4) | 3 (1.2) | |
M stage, n (%) | 1.000 | ||
M0 | 133 (49.3) | 133 (49.3) | |
M1 | 2 (0.7) | 2 (0.7) | |
Pathologic stage, n (%) | 0.001 | ||
Stage I | 102 (29.4) | 69 (19.9) | |
Stage II | 34 (9.8) | 52 (15) | |
Stage III | 33 (9.5) | 52 (15.0) | |
Stage IV | 3 (0.9) | 2 (0.6) | |
Residual tumor, n (%) | 0.044 | ||
R0 | 166 (48.5) | 158 (46.2) | |
R1 | 4 (1.2) | 13 (3.8) | |
R2 | 1 (0.3) | 0 (0) | |
Histologic grade, n (%) | 0.057 | ||
G1 | 35 (9.6) | 20 (5.5) | |
G2 | 91 (24.9) | 86 (23.5) | |
G3 | 51 (13.9) | 71 (19.4) | |
G4 | 6 (1.6) | 6 (1.6) | |
AFP (ng/mL), n (%) | 0.040 | ||
≤400 | 115 (41.4) | 98 (35.3) | |
>400 | 25 (9.0) | 40 (14.4) | |
Child-Pugh grade, n (%) | 0.497 | ||
A | 112 (46.9) | 105 (43.9) | |
B | 9 (3.8) | 12 (5.0) | |
C | 1 (0.4) | 0 (0) | |
Vascular invasion, n (%) | 0.029 | ||
No | 115 (36.5) | 91 (28.9) | |
Yes | 46 (14.6) | 63 (20.0) |
PCGF1, polycomb group factor 1; LIHC, liver hepatocellular carcinoma; IQR, interquartile range; AFP, alpha fetoprotein.
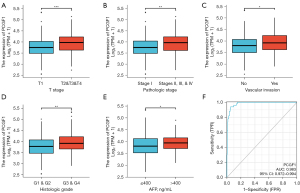
Table 3
Characteristics | Total (N) | OR | P value |
---|---|---|---|
T stage (T3 & T4 vs. T1 & T2) | 368 | 1.522 (0.949–2.459) | 0.083 |
N stage (N1 vs. N0) | 256 | 2.953 (0.372–60.132) | 0.351 |
M stage (M1 vs. M0) | 270 | 1.000 (0.119–8.434) | 1.000 |
Pathologic stage (Stages II, III, & IV vs. Stage I) | 347 | 2.239 (1.461–3.451) | <0.001 |
Gender (male vs. female) | 371 | 0.697 (0.449–1.077) | 0.105 |
Race (Black or African American & White vs. Asian) | 359 | 0.740 (0.486–1.122) | 0.157 |
Age (>60 vs. ≤60) | 370 | 0.957 (0.636–1.439) | 0.832 |
Residual tumor (R1 & R2 vs. R0) | 342 | 2.732 (1.005–8.675) | 0.062 |
Histologic grade (G3 & G4 vs. G1 & G2) | 366 | 1.606 (1.047–2.473) | 0.030 |
AFP (ng/mL) (>400 vs. ≤400) | 278 | 1.878 (1.070–3.345) | 0.030 |
Child-Pugh grade (B & C vs. A) | 239 | 1.280 (0.530–3.152) | 0.583 |
Vascular invasion (yes vs. no) | 315 | 1.731 (1.085–2.777) | 0.022 |
PCGF1, polycomb group factor 1; OR, odds ratio; AFP, alpha fetoprotein.
The diagnostic and prognostic value of PCGF1 expression in LIHC
To better understand the clinical benefits of PCGF1 evaluations, a ROC curve analysis was performed to determine its prognostic value. The area under the curve (AUC) of PCGF1 for identifying tumors from normal tissues was 0.983 (95% CI: 0.972–0.994). Thus, PCGF1 has a high value in terms of its diagnostic sensitivity and specificity (see Figure 6F). Additionally, the univariate Cox analysis showed that the high expression of PCGF1 was related to a decrease in OS [hazards ratio (HR): 1.692, P=0.006], DSS (HR: 1.756, P=0.022), and the PFI (HR: 1.683, P=0.001) (see Table 4).
Table 4
Characteristics | HR (95% CI) for OS | HR (95% CI) for DSS | HR (95% CI) for PFI | |||||||||||
---|---|---|---|---|---|---|---|---|---|---|---|---|---|---|
Univariate analysis |
P value | Multivariate analysis | P value | Univariate analysis | P value | Multivariate analysis | P value | Univariate analysis | P value | Multivariate analysis | P value | |||
PCGF1 | 1.692 (1.166–2.455) |
0.006 | 1.696 (1.063–2.704) |
0.027 | 1.756 (1.085–2.842) |
0.022 | 2.139 (1.107–4.131) |
0.024 | 1.683 (1.228–2.307) |
0.001 | 1.512 (1.031–2.219) |
0.034 | ||
T stage (T1 & T2 & T3 vs. T4) | 3.681 (1.912–7.085) |
<0.001 | 2.489 (0.920–6.735) |
0.073 | 6.012 (2.839–12.732) |
< 0.001 | 3.656 (1.077–12.414) |
0.038 | 2.747 (1.443–5.230) |
0.002 | 1.921 (0.788–4.684) |
0.151 | ||
N stage (N0 vs. N1) | 2.004 (0.491–8.181) |
0.333 | 3.562 (0.858–14.785) |
0.080 | 1.896 (0.442–8.135) |
0.389 | 1.385 (0.342–5.611) |
0.648 | ||||||
M stage (M0 vs. M1) | 4.032 (1.267–12.831) |
0.018 | 1.331 (0.311–5.697) |
0.700 | 5.102 (1.230–21.161) |
0.025 | 0.905 (0.149–5.503) |
0.913 | 3.442 (1.080–10.967) |
0.037 | 1.776 (0.474–6.653) |
0.394 | ||
Pathologic stage (Stage I vs. Stages II, III, & IV) | 2.074 (1.418–3.032) |
<0.001 | 2.154 (1.340–3.462) |
0.002 | 2.887 (1.705–4.888) |
<0.001 | 2.705 (1.393–5.253) |
0.003 | 2.310 (1.683–3.170) |
<0.001 | 1.775 (1.225–2.574) |
0.002 | ||
Histologic grade (G1 vs. G2, G3, & G4) | 1.216 (0.738–2.004) |
0.443 | 1.231 (0.649–2.335) |
0.524 | 1.211 (0.798–1.838) |
0.369 | ||||||||
Residual tumor (R0 vs. R1 & R2) | 1.571 (0.795–3.104) |
0.194 | 1.640 (0.711–3.782) |
0.246 | 1.515 (0.841–2.730) |
0.167 | ||||||||
AFP (ng/mL) (≤400 vs. >400) | 1.056 (0.646–1.727) |
0.827 | 0.849 (0.442–1.634) |
0.625 | 1.056 (0.705–1.581) |
0.793 | ||||||||
Age (≤60 vs. >60) | 1.248 (0.880–1.768) |
0.214 | 0.880 (0.566–1.370) |
0.573 | 0.952 (0.710–1.275) |
0.740 | ||||||||
Gender (female vs. male) | 0.816 (0.573–1.163) |
0.260 | 0.840 (0.533–1.323) |
0.452 | 0.973 (0.713–1.327) |
0.861 | ||||||||
Race (Asian vs. Black or African American & White) | 1.316 (0.909–1.906) |
0.146 | 1.469 (0.908–2.377) |
0.117 | 1.225 (0.905–1.658) |
0.188 |
OS, overall survival; DSS, disease-specific survival; PFI, progress-free interval; LIHC, liver hepatocellular carcinoma; HR, hazards ratio; CI, confidence interval; PCGF1, polycomb group factor 1; AFP, alpha fetoprotein.
To further examine the survival-related factors, we used a Cox proportional-risk regression model for the multivariate analysis. High PCGF1 expression remained an independent factor associated with poor OS (HR: 1.696, P=0.027), DSS (HR: 2.139, P=0.024), and the PFI (HR: 1.512, P=0.034). The analysis also showed that pathologic stage (Stage I vs. Stages II, III, & IV) had predictive advantages for OS (HR: 2.154, P=0.002), and the PFI (HR: 1.775, P=0.002) in LIHC patients. T stage (T1, T2, & T3 vs. T4) (HR: 3.656, P=0.038) and pathologic stage (Stage I vs. Stages II, III, & IV) (HR: 2.705, P=0.003) also showed the similar trend in DSS.
Next, as Figure 7A-7F show, a nomogram was constructed to predict the 1-, 3-, and 5-year OS, PFI, and DSS in TCGA-LIHC. Pathological stage and PCGF1 were incorporated into the nomogram to predict OS (C-index: 0.624) and the PFI (C-index: 0.656). A DSS predictive nomogram was established by T stage, pathological stage and PCGF1, and the C-index was 0.714. A calibration curve was drawn to verify the effectiveness of the nomogram.
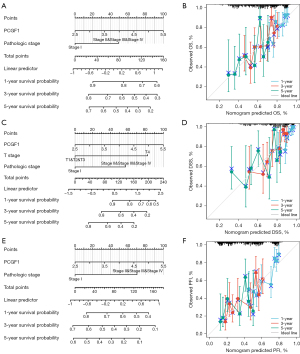
Identification of the high and low PCGF1-related DEGs in LIHC
To study the differential expression of PCGF1 between the high and low expression groups, we first identified 532 DEGs based on a |log2 FC| value >1.5 and an adjusted P value <0.05. Of the 532 DEGs, 422 were upregulated and 120 were downregulated (see Figure 8A). The top 20 genes correlated with PCGF1 are presented in a heatmap (see Figure 8B).

Functional annotation and predicted signaling pathways of PCGF1-associated DEGs
To predict the functional enrichment implications of PCGF1 in LIHC, the ClusterProfile software package was used to analyze the enrichment of GO and KEGG pathways. GO analysis was performed based on an adjusted P value less than 0.05 and Q value less than 0.2 to divide them into a biological process (BP) group (229 items), cellular composition (CC) group (44 items), and a molecular function (MF) group (49 items) (see Figure 8C). The KEGG pathways were mainly involved in some oncogenic signaling pathways, such as the Ras and PI3K-AKT signaling pathways (see Figure 8C).
Related signaling pathways of PXGF1 based on GSEA
To further quantify the role of PCGF1 in tumorigenesis and progression, a GSEA was performed based on a p adjusted value <0.05 and a FDR <0.25 in the Molecular Signatures Database (MSigDB) [c2.all.v7.0.symbols.gmt (Curated)]. The GSEA analysis showed that the PCGF1-associated DEGs were significantly enriched in cancer pathways (see Figure 9A-9F), such as the PI3K-AKT signaling pathway, the Wingless/Integrated (WNT) signaling pathway, forkhead box O (FOXO)-mediated transcription, selective autophagy, second gap-mitosis (G2-M) checkpoints, and cell cycle checkpoints.
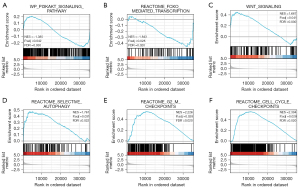
Role of PCGF1 in immune cells based on the ssGSEA
Tumor-infiltrating lymphocytes (TILs) are considered independent predictors of adverse OS in cancer patients (34,35). The relationship between PCGF1 expression and infiltration levels in 24 immune cell subtypes in the LIHC microenvironment was studied by a Wilcoxon rank-sum test. The enrichment score of the T cells, B cells, a cluster of differentiation 8 (CD8) T cells, cytotoxic cells, dendritic cells (DCs), iDCs, macrophages, mast cells, neutrophils, natural killer (NK) CD56dim cells, NK cells, pDCs, T central memory (Tcm), T effector memory (Tem), T gamma delta (Tgd), T helper cell 1 (Th1) cells, and regulatory T cells (Tregs) were enriched in the low PCGF1 group (P<0.05; see Figure 10A). Next, we conducted a Spearman analysis to explore the relationship between PCGF1 and the infiltration levels of TILs. We observed that PCGF1 expression was negatively associated with mast cells (r=–0.276; P<0.001), DCs (r=–0.273; P<0.001), B cells (r=–0.277; P<0.001), neutrophils (r=–0.282; P<0.001), and cytotoxic cells (r=–0.289; P<0.001) (see Figure 10B-10G). Further, we conducted a GSEA to quantify the immune-related-associated signaling pathways of PCGF1. PCGF1-high expression was significantly enriched in the interleukin (IL)-12, IL-4 and IL-13, and IL-10 signaling pathways, CD22 mediated B cell receptor (BCR) regulation, neutrophil pathways, and interferon-gamma pathways (see Figure 11A-11F). In summary, PCGF1 appears to play a role in the regulation of the tumor immune response.
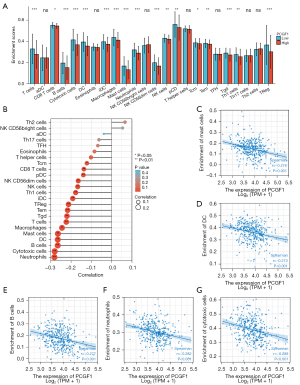
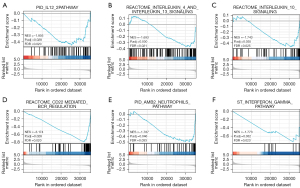
Discussion
LIHC is a major aggressive cancer of the digestive system worldwide, which is common in patients with chronic liver disease and cirrhosis (36). There have been some marked improvements in the management of LIHC since 2015, especially in the rapidly evolving field of systemic therapies, such as radiofrequency ablation, microwave ablation, cryoablation, and immunotherapy (37-40). With the development of a comprehensive treatment mode, the quality of life and OS of LIHC patients have been significantly improved. However, a useful predictive or prognostic LIHC biomarker has yet to be identified for use in daily practice, especially in immunotherapy (41).
PCGF1 is a member of the PCG protein family and plays an important role in the development of the mammalian nervous system. Studies have confirmed that PCGF1 plays an important role in the self-renewal of embryonic stem cells and is expressed in many tumors (13,21). The molecular mechanisms of PCGF1 in LIHC progression remain speculative and require further investigation. In our previous research, we found that PCGF1 was overexpressed in glioma, and is a prognostic biomarker related to tumor immunity (42). In this study, we conducted a comprehensive expression and survival analysis based on TCGA data and found that PCGF1 is highly expressed in several cancers. The KM (OS, DSS, and the PFI) analyses showed that high PCGF1 expression was associated with poor survival in LIHC. Ji et al. found that PCGF1 is upregulated in CRC and is associated with decreased OS rates (14). We also described the upregulation of PCGF1 in CRC tissues. In summary, our observations suggest that PCGF1 functions as a candidate oncogene and is unlikely to be limited to LIHC and CRC.
There is accumulating evidence that various ncRNAs play key roles in the pathogenesis of human cancer (43). It is increasingly recognized that ncRNAs interact with each other via a ceRNA network and crosstalk to regulate gene expression (44). MiRNAs are widely expressed in human cells and can regulate gene expression as negative regulators (45). Thus, we used the online StarBase tool to explore the upstream regulatory miRNAs of PCGF1. Ultimately, a total of 16 miRNAs were identified as upstream regulatory oncogenic miRNAs of PCGF1 in LIHC. These miRNAs appear to work as tumor suppressor miRNAs.
We also performed expression validation and survival analyses and found a high negative correlation between hsa-miR-22-3p and PCGF1 in LIHC. We found ample evidence that hsa-miR-22-3p plays a key role in the development of various tumor cells (46,47). Chen et al. (48) showed that hsa-miR-22-3p acts as a tumor suppressor that inhibits cell proliferation and induces cell cycle arrest and apoptosis by targeting specificity protein 1 (SP1) in LIHC. Further, catalpol negatively regulates MTA3 through hsa-mir-22-3p in LIHC cells to achieve an antitumor effect (49).
We also used the StarBase database to examine the upstream lncRNAs of the hsa-miR-22-3p/PCGF1 axis in LIHC. Under the ceRNA network and crosstalk hypothesis, upstream lncRNAs should be overexpressed in LIHC and act as oncogenic lncRNAs. Based on the expression, survival, and correlation analyses, only AC016405.3, BX284668.6, and MIR4435-2HG were identified as potential regulators of the hsa-miR-22-3p-PCGF1 axis and as functioning as oncogenes in LIHC.
In BRCA, AC016405.3 acts as an oncogenic lncRNA by targeting hsa-miR-22-3p to regulate ERBB3 (50). However, Ren et al. (51) reported that AC016405.3 is downregulated in glioblastoma and targets miR-19a-5p to regulate the expression of Ten-Eleven Translocation 2 (TET2) and inhibit cell proliferation and metastasis. BX284668.6 is highly expressed in TCGA-LIHC and is associated with a poor prognosis, but the function and mechanism of BX284668.6 in cancers are unknown. This needs to be explored further.
MIR4435-2HG enhances the development of LIHC (52,53). For example, Shen et al. (53) reported that MIR4435-2HG is involved in tumor proliferation and metastasis through the miR-22-3P/YWHAZ axis, which is related to a poor prognosis and overexpression. Based on these results, AC016405.3, BX284668.6, and MIR4435-2HG/the hsa-miR-22-3p/PCGF1 axis were used to construct a ceRNA network axis in LIHC.
Previous studies have shown that high PCGF1 expression is related to clinicopathological features and a poor prognosis (14,42). Additionally, similar results were observed in relation to LIHC, including in relation to the pathologic stage, histologic grade, AFP level, and vascular invasion. PCGF1 may be more predictive in early and late periods of cancer. In addition, for a biomarker to be successful, it must have appropriate detection accuracy. In recent years, while we have been keen to study novel biomarkers in serum or tissue biomarkers, their utility in clinical practice has been limited, except for AFP (41). Thus, we need to constantly search for biomarkers that can be used in the clinical diagnosis and prediction of LIHC. PCGF1 showed a promising diagnostic value for LIHC and had an AUC of 0.983 in the ROC analyses. We also constructed a predictive nomogram that included PCGF1 expression, T stage, and pathologic stage to determine the OS, DSS, and PFI of LIHC patients. Physicians can use this nomogram to identify high-risk patients. Thus, PCGF1 has the potential as a diagnostic and prognostic indicator of LIHC.
PcG complexes are involved in diverse BPs, including cell stages, cellular signaling and cancer, and positively regulate gene transcription, and modify non-histone substrates (54). PCGF4 (BMI1) is an important oncogene in CRC, which can downregulate the expression of cyclin-dependent kinase inhibitor 2A (CDKN2A) (55). Additionally, the gene product of PCGF2 (Mel-18) is very similar to that of PCGF4 in structure and is also a member of the PCG family (42). In gastric cancer, the expression of PCGF4 and PCGF2 were negatively correlated. Unlike PCGF4, which is an oncogene, PCGF2 acts as a tumor suppresser (56,57). Conversely, PCGF6 acts as a master regulator to Nanog homeobox (Nanog), octamer-binding transcription factor 4 (Oct4), and SRY-box transcription factor 2 (Sox2) expression in embryonic stem cells (58). Yan et al. reported that PCGF1 function is strongly and mechanistically associated with tumorigenesis and progression, and the c-Myc signaling pathway takes part in regulating cell proliferation in glioblastoma (15). We speculated that the abnormal expression of PCGF1 interferes with the transmission of various signal pathways related to tumorigenesis and progression. However, the role of PCGF1 in the occurrence and development of LIHC requires further study.
We then conducted a bioinformatics analysis to confirm the biological function and mechanisms of PCGF1 in LIHC. We found that PCGF1 was mainly involved in ion channel activity, gated channel activity, the immunoglobulin complex, the extracellular matrix, and neuron-to-neuron synapses. The KEGG analysis and the additional GSEA showed that PCGF1 expression was associated with the Ras, PI3K-AKT, FOXO, and WNT signaling pathways, autophagy, and the cell cycle. The literature demonstrated that these pathways play a pivotal role in tumorigenesis and progression, including proliferation, stemness, neovascularization, apoptosis, and epithelial-mesenchymal transition (59-63). Based on our findings, we hypothesized that PCGF1 functions as a proto-oncogene by promoting invasiveness and metastasis in LIHC; however, the detailed mechanisms should be tested in other experiments.
The tumor microenvironment (TME) is a complex ecology in which immune cells are critical components. In the TME, immune cells can have divergent effects on tumorigenesis (e.g., anti-tumorigenic and pro-tumorigenic effects) according to the environment and tumor type (64). During cancer development and progression, the variation of TME components controlled by the host immune system can affect the immunophenotype and disease progression (64,65). In our previous study, we found that the expression of PCGF1 in glioma was positively correlated with Th2 cells, and negatively correlated with T follicular helper (TFH) enrichment, traditional Chinese medicine, and Tgd (42). However, our LIHC data demonstrated that PCGF1 expression was negatively correlated with mast cells, DCs, B cells, neutrophils, and cytotoxic cells. Further, several immune-related pathways were confirmed by GSEA, such as the IL-12, IL-4, IL-13, and IL-10 signaling pathways, CD22-mediated BCR regulation, neutrophil pathways, and interferon-gamma pathways. The function of B cells in the TME can predict the poor prognosis of cancer, and the production of cytokines promotes immune suppressive phenotypes in tumor aggression (64). Neutrophils can release VEGF to promote tumor angiogenesis in the peritumoral stroma of LIHC (66). DCs harbor the potential to recognize, capture, and present antigens to T cells, which serve as immune system sentinels. However, the TME can combine with DCs to block its function and promote tumor progression (64,65).
Above all, we speculate that PCGF1 is a biomarker for predicting immune cell infiltration and a potential therapeutic target in LIHC. We identified the main mechanism of PCGF1 in LIHC; however, this study still had some limitations. First, it only used TCGA data, which may indicate bias. Second, to confirm the credibility of the results, our hypothesis needs to be further validated by experiments.
Conclusions
In summary, our research showed that PCGF1 is upregulated in multiple cancers and is a diagnostic and prognostic biomarker in LIHC. We further identified the upstream regulatory network of PCGF1, and used lncRNAs (i.e., AC016405.3, BX284668.6, and MIR4435-2HG) to construct a hsa-miR-22-3p/PCGF1 network. Further, our research suggests that PCGF1 may play a crucial role in immune infiltration and act as an oncogene in LIHC.
Acknowledgments
The authors appreciate the academic support from the AME Hepatocellular Carcinoma Collaborative Group.
Funding: The study was supported by 2021 Shandong Medical Association Clinical Research Fund—Qilu Special Project (No. YXH2022ZX02206).
Footnote
Reporting Checklist: The authors have completed the TRIPOD reporting checklist. Available at https://atm.amegroups.com/article/view/10.21037/atm-22-3862/rc
Conflicts of Interest: All authors have completed the ICMJE uniform disclosure form (available at https://atm.amegroups.com/article/view/10.21037/atm-22-3862/coif). The authors have no conflicts of interest to declare.
Ethical Statement: The authors are accountable for all aspects of the work in ensuring that questions related to the accuracy or integrity of any part of the work are appropriately investigated and resolved. The study was conducted in accordance with the Declaration of Helsinki (as revised in 2013).
Open Access Statement: This is an Open Access article distributed in accordance with the Creative Commons Attribution-NonCommercial-NoDerivs 4.0 International License (CC BY-NC-ND 4.0), which permits the non-commercial replication and distribution of the article with the strict proviso that no changes or edits are made and the original work is properly cited (including links to both the formal publication through the relevant DOI and the license). See: https://creativecommons.org/licenses/by-nc-nd/4.0/.
References
- Kim YS, Shin SW. Hepatocellular Carcinoma. N Engl J Med 2019;381:e2. [Crossref] [PubMed]
- Llovet JM, Kelley RK, Villanueva A, et al. Hepatocellular carcinoma. Nat Rev Dis Primers 2021;7:6. [Crossref] [PubMed]
- Bray F, Ferlay J, Soerjomataram I, et al. Global cancer statistics 2018: GLOBOCAN estimates of incidence and mortality worldwide for 36 cancers in 185 countries. CA Cancer J Clin 2018;68:394-424. [Crossref] [PubMed]
- Feng RM, Zong YN, Cao SM, et al. Current cancer situation in China: good or bad news from the 2018 Global Cancer Statistics? Cancer Commun (Lond) 2019;39:22. [Crossref] [PubMed]
- Torimura T, Iwamoto H. Treatment and the prognosis of hepatocellular carcinoma in Asia. Liver Int 2022;42:2042-54. [Crossref] [PubMed]
- Chen L, Wang Z, Song S, et al. The Efficacy of Radiotherapy in the Treatment of Hepatocellular Carcinoma with Distant Organ Metastasis. J Oncol 2021;2021:5190611. [Crossref] [PubMed]
- Roberts HJ, Wo JY. Stereotactic body radiation therapy for primary liver tumors: An effective liver-directed therapy in the toolbox. Cancer 2022;128:956-65. [Crossref] [PubMed]
- Fasano R, Shadbad MA, Brunetti O, et al. Immunotherapy for Hepatocellular Carcinoma: New Prospects for the Cancer Therapy. Life (Basel) 2021;11:1355. [Crossref] [PubMed]
- Liu JKH, Irvine AF, Jones RL, et al. Immunotherapies for hepatocellular carcinoma. Cancer Med 2022;11:571-91. [Crossref] [PubMed]
- Liu M, Xu M, Tang T. Association between chemotherapy and prognostic factors of survival in hepatocellular carcinoma: a SEER population-based cohort study. Sci Rep 2021;11:23754. [Crossref] [PubMed]
- Makary MS, Ramsell S, Miller E, et al. Hepatocellular carcinoma locoregional therapies: Outcomes and future horizons. World J Gastroenterol 2021;27:7462-79. [Crossref] [PubMed]
- Lou W, Wang W, Chen J, et al. ncRNAs-mediated high expression of SEMA3F correlates with poor prognosis and tumor immune infiltration of hepatocellular carcinoma. Mol Ther Nucleic Acids 2021;24:845-55. [Crossref] [PubMed]
- Nunes M, Blanc I, Maes J, et al. NSPc1, a novel mammalian Polycomb gene, is expressed in neural crest-derived structures of the peripheral nervous system. Mech Dev 2001;102:219-22. [Crossref] [PubMed]
- Ji G, Zhou W, Du J, et al. PCGF1 promotes epigenetic activation of stemness markers and colorectal cancer stem cell enrichment. Cell Death Dis 2021;12:633. [Crossref] [PubMed]
- Yan R, Cui F, Dong L, et al. Repression of PCGF1 Decreases the Proliferation of Glioblastoma Cells in Association with Inactivation of c-Myc Signaling Pathway. Onco Targets Ther 2020;13:253-61. [Crossref] [PubMed]
- Wang W, Qin JJ, Voruganti SPolycomb Group, et al. (PcG) Proteins and Human Cancers: Multifaceted Functions and Therapeutic Implications. Med Res Rev 2015;35:1220-67. [Crossref] [PubMed]
- Margueron R, Reinberg D. The Polycomb complex PRC2 and its mark in life. Nature 2011;469:343-9. [Crossref] [PubMed]
- Richly H, Aloia L, Di Croce L. Roles of the Polycomb group proteins in stem cells and cancer. Cell Death Dis 2011;2:e204. [Crossref] [PubMed]
- Schuettengruber B, Cavalli G. Recruitment of polycomb group complexes and their role in the dynamic regulation of cell fate choice. Development 2009;136:3531-42. [Crossref] [PubMed]
- Gong Y, Wang X, Liu J, et al. NSPc1, a mainly nuclear localized protein of novel PcG family members, has a transcription repression activity related to its PKC phosphorylation site at S183. FEBS Lett 2005;579:115-21. [Crossref] [PubMed]
- Hu PS, Xia QS, Wu F, et al. NSPc1 promotes cancer stem cell self-renewal by repressing the synthesis of all-trans retinoic acid via targeting RDH16 in malignant glioma. Oncogene 2017;36:4706-18. [Crossref] [PubMed]
- Rajasekhar VK, Begemann M. Concise review: roles of polycomb group proteins in development and disease: a stem cell perspective. Stem Cells 2007;25:2498-510. [Crossref] [PubMed]
- Huang W, Zhang Y, Xu Y, et al. Comprehensive analysis of the expression of sodium/potassium-ATPase alpha subunits and prognosis of ovarian serous cystadenocarcinoma. Cancer Cell Int 2020;20:309. [Crossref] [PubMed]
- Vivian J, Rao AA, Nothaft FA, et al. Toil enables reproducible, open source, big biomedical data analyses. Nat Biotechnol 2017;35:314-6. [Crossref] [PubMed]
- Liu J, Lichtenberg T, Hoadley KA, et al. An Integrated TCGA Pan-Cancer Clinical Data Resource to Drive High-Quality Survival Outcome Analytics. Cell 2018;173:400-416.e11. [Crossref] [PubMed]
- Li JH, Liu S, Zhou H, et al. starBase v2.0: decoding miRNA-ceRNA, miRNA-ncRNA and protein-RNA interaction networks from large-scale CLIP-Seq data. Nucleic Acids Res 2014;42:D92-7. [Crossref] [PubMed]
- Love MI, Huber W, Anders S. Moderated estimation of fold change and dispersion for RNA-seq data with DESeq2. Genome Biol 2014;15:550. [Crossref] [PubMed]
- Yu G, Wang LG, Han Y, et al. clusterProfiler: an R package for comparing biological themes among gene clusters. OMICS 2012;16:284-7. [Crossref] [PubMed]
- Subramanian A, Tamayo P, Mootha VK, et al. Gene set enrichment analysis: a knowledge-based approach for interpreting genome-wide expression profiles. Proc Natl Acad Sci U S A 2005;102:15545-50. [Crossref] [PubMed]
- Zhao K, Yi Y, Ma Z, et al. INHBA is a Prognostic Biomarker and Correlated With Immune Cell Infiltration in Cervical Cancer. Front Genet 2022;12:705512. [Crossref] [PubMed]
- Hänzelmann S, Castelo R, Guinney J. GSVA: gene set variation analysis for microarray and RNA-seq data. BMC Bioinformatics 2013;14:7. [Crossref] [PubMed]
- Bindea G, Mlecnik B, Tosolini M, et al. Spatiotemporal dynamics of intratumoral immune cells reveal the immune landscape in human cancer. Immunity 2013;39:782-95. [Crossref] [PubMed]
- Buntz A, Killian T, Schmid D, et al. Quantitative fluorescence imaging determines the absolute number of locked nucleic acid oligonucleotides needed for suppression of target gene expression. Nucleic Acids Res 2019;47:953-69. [Crossref] [PubMed]
- Azimi F, Scolyer RA, Rumcheva P, et al. Tumor-infiltrating lymphocyte grade is an independent predictor of sentinel lymph node status and survival in patients with cutaneous melanoma. J Clin Oncol 2012;30:2678-83. [Crossref] [PubMed]
- Miyawaki T, Wakuda K, Kenmotsu H, et al. Proposing synchronous oligometastatic non-small-cell lung cancer based on progression after first-line systemic therapy. Cancer Sci 2021;112:359-68. [Crossref] [PubMed]
- Tao J, Xu E, Zhao Y, et al. Modeling a human hepatocellular carcinoma subset in mice through coexpression of met and point-mutant beta-catenin. Hepatology 2016;64:1587-605. [Crossref] [PubMed]
- Llovet JM, Kelley RK, Villanueva A, et al. Hepatocellular carcinoma. Nat Rev Dis Primers 2021;7:6. [Crossref] [PubMed]
- Rajyaguru DJ, Borgert AJ, Smith AL, et al. Radiofrequency Ablation Versus Stereotactic Body Radiotherapy for Localized Hepatocellular Carcinoma in Nonsurgically Managed Patients: Analysis of the National Cancer Database. J Clin Oncol 2018;36:600-8. [Crossref] [PubMed]
- Yang JD, Hainaut P, Gores GJ, et al. A global view of hepatocellular carcinoma: trends, risk, prevention and management. Nat Rev Gastroenterol Hepatol 2019;16:589-604. [Crossref] [PubMed]
- Sangro B, Sarobe P, Hervás-Stubbs S, et al. Advances in immunotherapy for hepatocellular carcinoma. Nat Rev Gastroenterol Hepatol 2021;18:525-43. [Crossref] [PubMed]
- Piñero F, Dirchwolf M, Pessôa MG. Biomarkers in Hepatocellular Carcinoma: Diagnosis, Prognosis and Treatment Response Assessment. Cells 2020;9:1370. [Crossref] [PubMed]
- Xie J, Qiao L, Deng G, et al. PCGF1 is a prognostic biomarker and correlates with tumor immunity in gliomas. Ann Transl Med 2022;10:227. [Crossref] [PubMed]
- Mowel WK, McCright SJ, Kotzin JJ, et al. Group 1 Innate Lymphoid Cell Lineage Identity Is Determined by a cis-Regulatory Element Marked by a Long Non-coding RNA. Immunity 2017;47:435-449.e8. [Crossref] [PubMed]
- Chiu HS, Martínez MR, Komissarova EV, et al. The number of titrated microRNA species dictates ceRNA regulation. Nucleic Acids Res 2018;46:4354-69. [Crossref] [PubMed]
- Yuan Q, Yu H, Chen J, et al. Antitumor Effect of miR-1294/Pyruvate Kinase M2 Signaling Cascade in Osteosarcoma Cells. Onco Targets Ther 2020;13:1637-47. [Crossref] [PubMed]
- Xiong J, Du Q, Liang Z. Tumor-suppressive microRNA-22 inhibits the transcription of E-box-containing c-Myc target genes by silencing c-Myc binding protein. Oncogene 2010;29:4980-8. [Crossref] [PubMed]
- Xu D, Takeshita F, Hino Y, et al. miR-22 represses cancer progression by inducing cellular senescence. J Cell Biol 2011;193:409-24. [Crossref] [PubMed]
- Chen J, Wu FX, Luo HL, et al. Berberine upregulates miR-22-3p to suppress hepatocellular carcinoma cell proliferation by targeting Sp1. Am J Transl Res 2016;8:4932-41. [PubMed]
- Zhao L, Wang Y, Liu Q. Catalpol inhibits cell proliferation, invasion and migration through regulating miR-22-3p/MTA3 signalling in hepatocellular carcinoma. Exp Mol Pathol 2019;109:51-60. [Crossref] [PubMed]
- Wei M, Wang J, He Q, et al. AC016405.3 functions as an oncogenic long non-coding RNA by regulating ERBB3 via sponging miR-22-3p in breast cancer. J Clin Lab Anal 2021;35:e23952. [Crossref] [PubMed]
- Ren S, Xu Y. AC016405.3, a novel long noncoding RNA, acts as a tumor suppressor through modulation of TET2 by microRNA-19a-5p sponging in glioblastoma. Cancer Sci 2019;110:1621-32. [Crossref] [PubMed]
- Kong Q, Liang C, Jin Y, et al. The lncRNA MIR4435-2HG is upregulated in hepatocellular carcinoma and promotes cancer cell proliferation by upregulating miRNA-487a. Cell Mol Biol Lett 2019;24:26. [Crossref] [PubMed]
- Shen X, Ding Y, Lu F, et al. Long noncoding RNA MIR4435-2HG promotes hepatocellular carcinoma proliferation and metastasis through the miR-22-3p/YWHAZ axis. Am J Transl Res 2020;12:6381-94. [PubMed]
- Chan HL, Morey L. Emerging Roles for Polycomb-Group Proteins in Stem Cells and Cancer. Trends Biochem Sci 2019;44:688-700. [Crossref] [PubMed]
- Bracken AP, Kleine-Kohlbrecher D, Dietrich N, et al. The Polycomb group proteins bind throughout the INK4A-ARF locus and are disassociated in senescent cells. Genes Dev 2007;21:525-30. [Crossref] [PubMed]
- Lu YW, Li J, Guo WJ. Expression and clinicopathological significance of Mel-18 and Bmi-1 mRNA in gastric carcinoma. J Exp Clin Cancer Res 2010;29:143. [Crossref] [PubMed]
- Zhang XW, Sheng YP, Li Q, et al. BMI1 and Mel-18 oppositely regulate carcinogenesis and progression of gastric cancer. Mol Cancer 2010;9:40. [Crossref] [PubMed]
- Yang CS, Chang KY, Dang J, et al. Polycomb Group Protein Pcgf6 Acts as a Master Regulator to Maintain Embryonic Stem Cell Identity. Sci Rep 2016;6:26899. [Crossref] [PubMed]
- Katoh M, Igarashi M, Fukuda H, et al. Cancer genetics and genomics of human FOX family genes. Cancer Lett 2013;328:198-206. [Crossref] [PubMed]
- Behrooz AB, Syahir A. Could We Address the Interplay Between CD133, Wnt/beta-Catenin, and TERT Signaling Pathways as a Potential Target for Glioblastoma Therapy? Front Oncol 2021;11:642719. [Crossref] [PubMed]
- Gurzu S, Kobori L, Fodor D, et al. Epithelial Mesenchymal and Endothelial Mesenchymal Transitions in Hepatocellular Carcinoma: A Review. Biomed Res Int 2019;2019:2962580. [Crossref] [PubMed]
- Zhang HF, Gao X, Wang X, et al. The mechanisms of renin-angiotensin system in hepatocellular carcinoma: From the perspective of liver fibrosis, HCC cell proliferation, metastasis and angiogenesis, and corresponding protection measures. Biomed Pharmacother 2021;141:111868. [Crossref] [PubMed]
- Zhou Y, Li Y, Xu S, et al. Eukaryotic elongation factor 2 kinase promotes angiogenesis in hepatocellular carcinoma via PI3K/Akt and STAT3. Int J Cancer 2020;146:1383-95. [Crossref] [PubMed]
- Anderson NM, Simon MC. The tumor microenvironment. Curr Biol 2020;30:R921-5. [Crossref] [PubMed]
- Arneth B. Tumor Microenvironment. Medicina (Kaunas) 2019;56:15. [Crossref] [PubMed]
- Schobert IT, Savic LJ, Chapiro J, et al. Neutrophil-to-lymphocyte and platelet-to-lymphocyte ratios as predictors of tumor response in hepatocellular carcinoma after DEB-TACE. Eur Radiol 2020;30:5663-73. [Crossref] [PubMed]
(English Language Editor: L. Huleatt)