Identification and validation of a 9-gene signature for the prognosis of ovarian cancer by integrated bioinformatical analysis
Introduction
Ovarian cancer (OC) is by far the most aggressive malignancy among female reproductive carcinomas, and it has been reported that there are approximately 300,000 new cases per year globally (1). Due to its uncertain etiology and a lack of efficient early detection biomarkers, approximately 70% of OC individuals are already at an advanced stage with widespread metastases at the time of diagnosis (2). Even though some genetic risk factors like breast cancer 1 (BRCA1) and breast cancer 2 (BRCA2) are identified in OC (3), the development of new predictive biomarkers is important for the understanding of OC. Despite some advances in the past few decades, the long-term survival rate of individuals with OC remains dismal (4). Thus, it is vital to identify novel biomarkers for the prognosis and early diagnosis to elevate the survival rates of OC patients.
Recently, various biomarkers have been identified as having a significant effect on oncogenicity and as having the potential to be used to treat OC. In-vitro and in-vivo research has shown that the silencing of mitochondrial elongation factor 2 greatly inhibits OC cell growth and metastasis by blocking epithelial-to-mesenchymal transition, and causing cell death, and thus is considered a viable prognostic indicator of OC (5). Carbohydrate antigen 25 (CA125) was detected as a biomarker for OC with the specificity of 78% (95% CI: 76–80%) (6). Moreover, circulating tumor DNA is a promising biomarker with an estimated sensitivity of 70% and specificity of 90% for quantitative analysis in OC (7). Lung adenocarcinoma (LUAD) has been shown to be associated with a 9-gene signature that is independently linked to the disease and substantially correlated with immunological infiltration (8). This signature might provide direction for LUAD patients’ prognoses and molecular-targeted treatment (8). Due to the unsatisfied clinical utility, it is urgent to identify more effective biomarkers to improve the survival of OC.
We built a prognostic risk model comprising protein phosphatase, Mg2+/Mn2+ dependent 1K (PPM1K), protein phosphatase 1 catalytic subunit alpha (PPP1CA), exostosin glycosyltransferase 1 (EXT1), RAB GTPase activating protein 1 like (RABGAP1L), mitotic arrest deficient 2 like 1 (MAD2L1), xeroderma pigmentosum complementation group C (XPC), Egl-9 family hypoxia inducible factor 3 (EGLN3), cyclin D1 binding protein 1 (CCNDBP1), and zinc finger protein 25 (ZNF25) functions as innovative biomarkers, and validated the prognostic and predictive significance of the model in providing molecular evidence of OC. We present the following article in accordance with the TRIPOD reporting checklist (available at https://atm.amegroups.com/article/view/10.21037/atm-22-3752/rc).
Methods
Data sets and data acquisition
The Cancer Genome Atlas (TCGA) (https://portal.gdc.cancer.gov/repository) provided us with the ribonucleic acid sequencing (RNA-seq) data of 379 OC patients and the relevant clinical characteristics. We also acquired 4 microarray data sets GSE105437 (9), GSE14407 (10), GSE54388 (11), GSE69428 (12), and a immunotherapy cohort (IMvigor210) (13) from the Gene Expression Omnibus (GEO) database. GSE26193 was used as a training cohort while GSE18520 was treated as a validation cohort and TCGA as an external validation cohort for clinicopathological features. The study was conducted in accordance with the Declaration of Helsinki (as revised in 2013).
DEGs
When comparing the expression profiling data between the OC and adjacent normal samples, the “limma” R program was employed as a screening tool. A |log 2-fold change (FC)| >1 and an adjusted P value <0.05 were considered significant.
WGCNA and key module identification
A WGCNA was conducted to identify the co-expressed gene modules and examine the links between the gene networks and the clinical characteristics (14). The WGCNA was conducted using the “WGCNA” function in the R platform to develop a matrix establishing the module-trait correlations between the prognostic genes and the grade based on the β value, and the Pearson correlation test was used (soft-thresholding value).
Functional enrichment analysis
To determine the biological roles of the key genes in the modules, we used the “clusterProfiler” R program to conduct Gene Ontology (GO) functional annotations (15) and a Kyoto Encyclopedia of Genes and Genomes (KEGG) pathway enrichment analysis using the key genes in the modules (16). The cutoff value for the adjusted P value in relation to the false discovery rate was set at 0.05.
Establishment of a prognostic model for OC
To select the prognostic genes from the DEGs, we performed multivariate and univariate Cox regression analyses. A LASSO regression analysis was employed to examine the genes that had been chosen for further investigation. To choose the prognostic genes, the LASSO Cox regression technique was employed in conjunction with the “glmnet” program to determine the variable coefficients. To determine each sample’s risk score, we used the following equation: risk score = (coefficient mRNA1 × expression of mRNA1) + (coefficient mRNA2 × expression of mRNA2) + … + (coefficient mRNAn × expression mRNAn). The threshold level was determined by taking the median risk score for all the specimens. The specimens in the training and validation cohorts were classified into high- and low-risk groups based on the threshold value.
Nomogram
To discover the independent predicting variables, the “rms” tool in the R program was used to create the nomogram and calibrate curves. With the help of a receiver operating characteristic curve analysis, we successfully verified the specificity and sensitivity of the nomogram in terms of overall survival (OS) prediction.
Relationship of gene expression with immune cell infiltration
The Spearman’s correlation test was used to examine the possible link between the hub genes’ expression levels and the levels of the 6 tumor-infiltrating immune cells (TIICs). The data were acquired from the Tumor Immune Estimation Resource (TIMER; https://cistrome.shinyapps.io/timer/). A P value <0.05, when corrected for tumor purity, was considered significant.
Prediction of chemotherapeutic drugs
To evaluate the different sensitivities to chemotherapeutic agents for the high and low long non-coding ribonucleic acid (lncRNA) score subgroups, the pRRophetic algorithm was used to predict the 50% inhibiting concentration (IC50) value of the 138 drugs based on the Cancer Cell Line Encyclopedia (17).
Statistical analysis
All the computational and statistical studies were undertaken using the R program (version 4.0.3). The Kaplan-Meier technique was employed to conduct a survival analysis of the data. Statistically significant differences were set at two-sided P<0.05.
Results
Identification of DEGs and mapping to chromosomal locations
Following the examination of the GEO database, we identified 4 potentially useful microarray data sets (i.e., GSE105437, GSE14407, GSE54388, and GSE69428), which were included in our research. Table 1 summarizes the most essential features of the GEO data sets that were included. A total of 46 OC and 33 normal tissues were included in our study. Based on the findings recorded from the Robust Rank Analysis (RRA) analysis with an adjusted P value <0.05 and a |log 2-FC| >1, we identified 499 DEGs that were significant, of which 196 were upregulated and 303 were downregulated (see Figure 1A). S100 Calcium Binding Protein 2 (S100A2) was the top-ranking gene among all those that were upregulated (P=9.50E-10, adjusted P=2.06E-05), while OGN (P=8.07E-10, adjusted P=1.75E-05) was the most considerably downregulated gene in the OC samples. Further, the top 20 upregulated and downregulated DEGs were shown on a heatmap (see Figure 1B). To illustrate the expression profiles of these genes and their corresponding chromosome sites, the 50 most upregulated and downregulated genes were selected (see Figure 1C). The top 5 upmodulated genes (i.e., S100A2, RRM2, PTH2R, KLK6, and MELK) were distributed in chromosomes 1, 2, 2, 19, and 9. While, the top 5 downregulated genes (i.e., OGN, TCEAL2, ALDH1A1, NDNF, and BCHE) were distributed in chromosomes 9, X, 9, 4, and 3.
Table 1
Series accession ID | Country | Number of samples | Platform ID | |
---|---|---|---|---|
Tumor | Normal | |||
GSE105437 | South Korea | 10 | 5 | GPL570 |
GSE14407 | United States | 12 | 12 | GPL570 |
GSE54388 | United States | 16 | 6 | GPL570 |
GSE69428 | China | 10 | 10 | GPL570 |
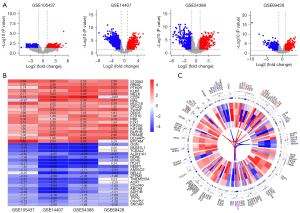
Analysis of the enrichment of DEGs
The biological roles of DEGs were investigated using GO annotations. Mitotic nuclear division, mitotic sister chromatid segregation, and sister chromatid segregation were the biological processes (BPs) that were the most enriched (see Figure 2A). In relation to the cellular components (CCs), the spindle, chromosome, centromeric region, and midbody were the most enriched (see Figure 2B). In relation to the molecular functions (MFs), the DEGs enrichment was predominantly found in microtubule binding, tubulin binding, and heparin-binding (see Figure 2C). Additionally, the enriched KEGG pathways included the cell cycle, retinol metabolism, and protein 53 signaling pathways (see Figure 2D).
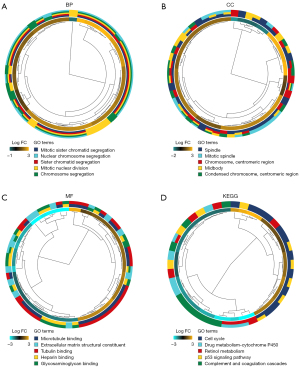
WGCNA and identification of critical modules
To identify which major modules were strongly linked to the clinical characteristics of OC, a WGCNA was conducted of the GSE26193 data set, which included the DEGs (see Figure 3A). Following the establishment of the soft-threshold power as 4 (scale-free R2=0.95 slope =−2.36; see Figure 3B,3C), 30 modules were obtained from the co-expression network after integrating similar modules based on a cutoff height of 0.25 (see Figure 3D). The green, green-yellow, and turquoise modules were found to be substantially linked to the clinical features, as shown by a heatmap of the module-trait associations (see Figure 3E). The module relevance of these 3 modules was greater than that of other modules, indicating that there was a significant link to of the modules the grade (see Figure 3F).
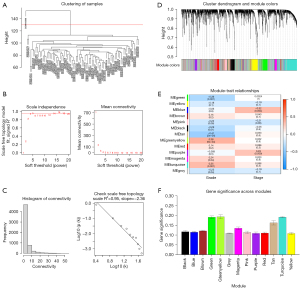
Validation of hub genes
To detect the hub genes from the modules, we analyzed the members from the 3 modules. Figure 4A shows the P values and the relationship between the gene significance values and module membership. The univariable and multivariable Cox regression analyses revealed that PPM1K, PPP1CA, EXT1, RABGAP1L, MAD2L1, XPC, EGLN3, CCNDBP1, and ZNF25 might have prognostic value (see Figure 4B,4C). The expression levels of CCNDBP1, PPM1K, RABGAP1L, and ZNF25 were considerably lower in the OC samples than the normal tissue samples, while EGLN3, MAD2L1, PPP1CA were much lower in the normal tissue samples than the OC samples (see Figure 4D). Further, immunohistochemical staining data acquired from the Human Protein Atlas (HPA) database confirmed the consistent protein expression levels of the 7 hub genes, excluding EGLN3 and EXT1, which were not stained in the HPA data (see Figure 4E).
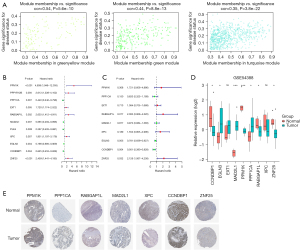
Establishment of a prognostic risk model
A regression analysis using the LASSO was used to predict the therapeutic outcomes of the 9 hub genes in OC patients with high accuracy. The study was conducted using the expression patterns of the 9 hub genes from the GEO training cohort. The coefficients produced by the LASSO technique were used to compute the risk scores of the training (GSE26193) and validation (GSE18520) cohorts, and in this case, the following formula was used: (risk score = 0.54302496 × PPM1K − 0.4778786 × PPP1CA + 0.08080111 × EXT1 + 0.6944571 × RABGAP1L − 0.0278971 × MAD2L1 + 0.43948467 × XPC − 0.2467983 × EGLN3 − 0.5788344 × CCNDBP1 + 0.75443752 × ZNF25). Based on the median risk score, patients were categorized into the following 2 groups: the low- and high-risk groups. Figure 5A,5B show the distribution of the risk scores, OS, and the expression patterns of the 9 hub genes across the training and validation groups. An examination of the Kaplan-Meier survival data derived from this model demonstrated that patients belonging to the low-risk group had significantly longer survival times than those belonging to the high-risk group (see Figure 5C). This was consistent with TCGA results (see Figure S1).
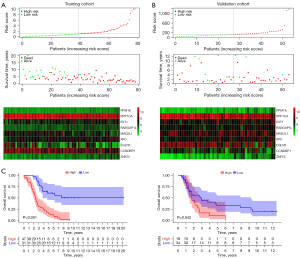
Nomogram construction
We conducted univariate and multivariate Cox regression analyses of the grade, stage, and risk score to examine whether the newly developed immune risk score model was independent of other clinicopathologic factors (univariate: HR =1.853, P<0.001; multivariate: HR =1.698, P=0.003, see Figure 6A,6B). The findings from the 2 analyses demonstrated that the risk score was an independent prognostic predictor for OC. A nomogram was created to help us illustrate our model, and we discovered that the grade, risk score, and stage of OC patients may all be used to predict their survival (see Figure 6C).
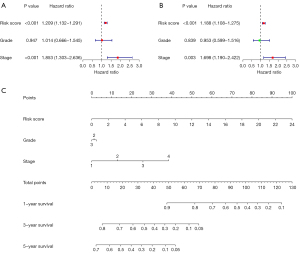
Relationship between hub genes and immune cell infiltration
The TIMER analysis indicated that the expression of PPM1K was linked to dendritic cells, cluster of differentiation (CD)8+ T cells, macrophages, and neutrophil cells. PPP1CA was shown to be closely linked to dendritic cells, B cells, macrophages, and neutrophils. MAD2L1 expression was linked to dendritic cells, macrophages, and neutrophils. XPC expression was linked to dendritic cells and B cells. EGLN3 expression was linked to macrophages, neutrophils, and dendritic cells. There was a substantial association between CCNDBP1 expression and dendritic cells, B cells, CD8+ T cells, and neutrophils. ZNF25 was only correlated with macrophages. No significant link was found between EXT1 and RABGAP1L expression (see Figure 7).
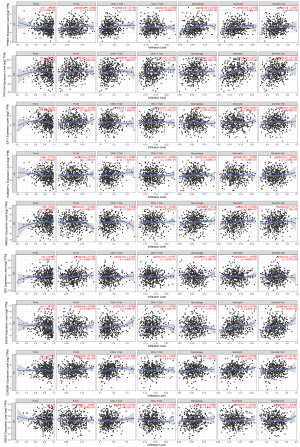
The risk score predicted immunotherapeutic benefits
To examine the possible mechanisms of the lncRNA score in OC, we determined the enriched pathways between the high- and low-risk score groups using KEGG. In the low-risk score group, immune-related pathways were enriched, including antigen processing and the presentation and intestinal immune network for immunoglobulin A (IgA) production (see Figure 8A). Moreover, homologous recombination, the cell cycle, and mismatch repair were also highly enriched in the low-risk score group. Additionally, the risk score was strongly associated with repair like mismatch repair, nucleotide excision repair, and deoxyribonucleic acid (DNA) damage repair (see Figure 8B).
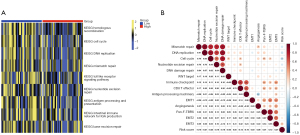
We explored the predictive significance of the risk score in relation to responsiveness to immune checkpoint blockade (ICB) treatment in 2 immunotherapy groups. The expression of programmed death-ligand 1 (PD-L1) was high in the high-risk score patients (see Figure 9A). Patients receiving anti-PD-L1 treatment with high-risk scores had a more favorable prognosis than in the low-risk score group (IMvigor210; see Figure 9B). Patients with high-risk scores had remarkable therapeutic benefits and showed enhanced immune responsiveness to the PD-L1 blockade (see Figure 9C,9D). Further, patients who had a combined high-risk score and high neoantigen load benefited significantly in terms of survival (see Figure 9E). In IMvigor210, the high-risk scores were significantly associated with the inflamed immune phenotype, and the checkpoint inhibitors exerted an anti-tumor effect in this phenotype (see Figure 9F). Thus, the risk score was shown to be significantly correlated with tumor immune phenotypes and was useful in predicting the responses of patients to anti-PD-L1 immunotherapy.
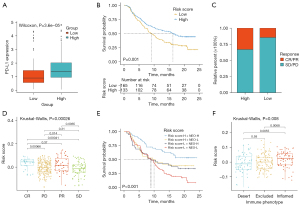
The risk score predicted patients’ sensitivity to chemotherapeutic drugs
To evaluate the value of the risk score in predicting patients’ responses to drugs, the IC50 values of 138 drugs were calculated (see Figure 10A). We found that the high-risk score patients had a greater sensitivity to midostaurin (see Figure 10B), Nutlin.3a (see Figure 10C), PD.173074 (see Figure 10D), and NVP.BEZ235 (see Figure 10E). Thus, the risk score appeared to be a predictive biological marker for medications against OC.
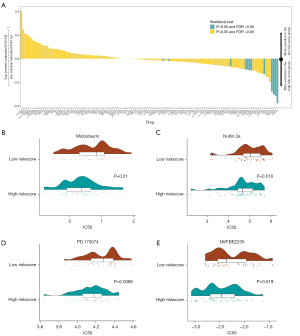
Discussion
We discovered 499 DEGs between the OC and neighboring normal tissues in our investigation. Further, we performed a WGCNA, and 9 hub genes were chosen by multivariate and univariate Cox regression analyses and LASSO. A prognostic risk model, which was considered to be an independent indicator, was also strongly linked to the infiltration of immune cells. Thus, a prognostic risk model comprising PPM1K, PPP1CA, EXT1, RABGAP1L, MAD2L1, XPC, EGLN3, CCNDBP1, and ZNF25 may be used as an innovative biological marker with prognostic and predictive significance for OC.
To identify the OC-related hub genes, we aggregated the expression patterns from 4 GEO data sets. S100A2 was the most highly expressed gene in the OC tissues, which is consistent with a similar finding that S100A2 overexpression increases glucose metabolism and proliferation in colorectal cancer (18). Additionally, OGN, which was the most lowly expressed gene in OC, has been shown to be a tumor inhibitor in bladder cancer (19). We discovered that DEGs are linked to cell proliferation after examining their expression levels in the GO and KEGG pathways. The WGCNA was used to identify the co-expression modules linked to the clinical characteristics.
Based on the univariate, multivariate Cox regression, and LASSO analyses, 9 key genes (i.e., PPM1K, PPP1CA, EXT1, RABGAP1L, MAD2L1, XPC, EGLN3, CCNDBP1, and ZNF25) were found to be correlated with the prognosis of OC. PPM1K has not been closely studied in OC. Research has shown that a PPM1K deficiency results in a significant reduction in MEIS1/p21 signaling, which reduces hematopoietic stem cells’ glycolysis and quiescence, and the deletion of PPM1K greatly prolongs survival in a mouse leukemia model (20). Congruent with our findings, research has shown that PPP1CA is expressed at a higher level in esophageal squamous cell carcinoma samples than adjoining normal samples (21). Previous research has shown that EXT1 is downregulated in acute lymphoblastic leukemia (22), but the levels of EXT1 did not differ in our study between the OC and normal tissues. In breast cancer cells and mouse fibroblasts, RABGAP1L has been shown to be an important aspect for conformational specific integrin trafficking and to delineate the function of Rabgap1 in β1-integrin-induced cell migration (23). The overexpression of MAD2L1 has been discovered in diverse malignancies, such as lung (24), breast (25), and gastric cancers (26). XPC has been identified as an essential protein that recognizes DNA damage and performs an integral function in the repair of nucleotide excision and the modulation of cell growth and viability in non-small cell lung cancer (27). EGLN3 regulates tumor cell apoptosis and proliferation in glioma (28). When tested on dedifferentiated liposarcoma cells, CCNDBP1 was shown to greatly reduce cancer cell clone creation, and the proliferative, migratory, and invasive capacities of cells (29). ZNF25 performs a critical function in the differentiation of human bone marrow stromal or mesenchymal stem cells (hMSCs) to osteoblasts (30), but it is not well studied in cancers. Thus, the 9 hub genes identified perform important roles in the modulation of biological activities in cells.
Tumor microenvironments and immune cell infiltration are becoming more popular topics of research (31-34). The 9 genes identified in the present research are linked to neutrophils, B cells, CD8+ T cells, macrophages, and dendritic cells, and these genes may have promising applications in immunotherapies. Notably, in lung cancer, TIICs are thought to be major drivers of both patients’ prognoses and their responsiveness to immunotherapeutic treatments. However, the possible mechanisms of the biomarkers and immune cells remain to be explored.
The KEGG analysis indicated that the immune-related pathways were enriched. Notably, antigen processing and presentation and the intestinal immune network for IgA production were enriched in the low-risk score group. We also explored the predictive value of the risk score in relation to patients’ responses to immunotherapy. The PD-L1 blockade proved to have more therapeutic advantages and produce more immune responses in patients with high-risk scores. Further, the combination of a high-risk score and a high neoantigen burden served as a significant predictor of survival. Notably, higher-risk scores were closely associated with an inflamed immune phenotype, which provided evidence that high-risk scores were beneficial for immunotherapy. A combination of the results from the immunotherapy cohorts strongly supported the supposition that the risk score is a predictor of the immunotherapeutic response in OC patients. Additionally, potential chemotherapeutic drugs were predicted based on the risk score, indicating that the risk score is a meaningful tool for evaluating the drug sensitivity of OC patients.
Conclusions
In summary, we established a Riskscore system to identify the OC patients who are eligible for immunotherapy and predict their sensitivity to chemotherapeutic drugs. However, it should be noted that further investigations on the role and mechanism of this 9-gene signature in the progression of OC need to be conducted.
Acknowledgments
Funding: None.
Footnote
Reporting Checklist: The authors have completed the TRIPOD reporting checklist. Available at https://atm.amegroups.com/article/view/10.21037/atm-22-3752/rc
Conflicts of Interest: All authors have completed the ICMJE uniform disclosure form (available at https://atm.amegroups.com/article/view/10.21037/atm-22-3752/coif). The authors have no conflicts of interest to declare.
Ethical Statement: The authors are accountable for all aspects of the work in ensuring that questions related to the accuracy or integrity of any part of the work are appropriately investigated and resolved. The study was conducted in accordance with the Declaration of Helsinki (as revised in 2013).
Open Access Statement: This is an Open Access article distributed in accordance with the Creative Commons Attribution-NonCommercial-NoDerivs 4.0 International License (CC BY-NC-ND 4.0), which permits the non-commercial replication and distribution of the article with the strict proviso that no changes or edits are made and the original work is properly cited (including links to both the formal publication through the relevant DOI and the license). See: https://creativecommons.org/licenses/by-nc-nd/4.0/.
References
- Siegel RL, Miller KD, Jemal A. Cancer statistics, 2020. CA Cancer J Clin 2020;70:7-30. [Crossref] [PubMed]
- Koppenol WH, Bounds PL, Dang CV. Otto Warburg's contributions to current concepts of cancer metabolism. Nat Rev Cancer 2011;11:325-37. [Crossref] [PubMed]
- Paul A, Paul S. The breast cancer susceptibility genes (BRCA) in breast and ovarian cancers. Front Biosci (Landmark Ed) 2014;19:605-18. [Crossref] [PubMed]
- Coleman RL, Monk BJ, Sood AK, et al. Latest research and treatment of advanced-stage epithelial ovarian cancer. Nat Rev Clin Oncol 2013;10:211-24. [Crossref] [PubMed]
- Zhao S, Zhang X, Shi Y, et al. MIEF2 over-expression promotes tumor growth and metastasis through reprogramming of glucose metabolism in ovarian cancer. J Exp Clin Cancer Res 2020;39:286. [Crossref] [PubMed]
- Ferraro S, Braga F, Lanzoni M, et al. Serum human epididymis protein 4 vs carbohydrate antigen 125 for ovarian cancer diagnosis: a systematic review. J Clin Pathol 2013;66:273-81. [Crossref] [PubMed]
- Zhou J, Gong G, Tan H, et al. Urinary microRNA-30a-5p is a potential biomarker for ovarian serous adenocarcinoma. Oncol Rep 2015;33:2915-23. [Crossref] [PubMed]
- Zhong H, Wang J, Zhu Y, et al. Comprehensive Analysis of a Nine-Gene Signature Related to Tumor Microenvironment in Lung Adenocarcinoma. Front Cell Dev Biol 2021;9:700607. [Crossref] [PubMed]
- Noh K, Mangala LS, Han HD, et al. Differential Effects of EGFL6 on Tumor versus Wound Angiogenesis. Cell Rep 2017;21:2785-95. [Crossref] [PubMed]
- Bowen NJ, Walker LD, Matyunina LV, et al. Gene expression profiling supports the hypothesis that human ovarian surface epithelia are multipotent and capable of serving as ovarian cancer initiating cells. BMC Med Genomics 2009;2:71. [Crossref] [PubMed]
- Yeung TL, Leung CS, Wong KK, et al. ELF3 is a negative regulator of epithelial-mesenchymal transition in ovarian cancer cells. Oncotarget 2017;8:16951-63. [Crossref] [PubMed]
- Yamamoto Y, Ning G, Howitt BE, et al. In vitro and in vivo correlates of physiological and neoplastic human Fallopian tube stem cells. J Pathol 2016;238:519-30. [Crossref] [PubMed]
- Mariathasan S, Turley SJ, Nickles D, et al. TGFβ attenuates tumour response to PD-L1 blockade by contributing to exclusion of T cells. Nature 2018;554:544-8. [Crossref] [PubMed]
- Langfelder P, Horvath S. WGCNA: an R package for weighted correlation network analysis. BMC Bioinformatics 2008;9:559. [Crossref] [PubMed]
- Harris MA, Clark J, Ireland A, et al. The Gene Ontology (GO) database and informatics resource. Nucleic Acids Res 2004;32:D258-61. [Crossref] [PubMed]
- Kanehisa M, Sato Y, Kawashima M, et al. KEGG as a reference resource for gene and protein annotation. Nucleic Acids Res 2016;44:D457-62. [Crossref] [PubMed]
- Geeleher P, Cox NJ, Huang RS. Clinical drug response can be predicted using baseline gene expression levels and in vitro drug sensitivity in cell lines. Genome Biol 2014;15:R47. [Crossref] [PubMed]
- Li C, Chen Q, Zhou Y, et al. S100A2 promotes glycolysis and proliferation via GLUT1 regulation in colorectal cancer. FASEB J 2020;34:13333-44. [Crossref] [PubMed]
- Liang X, Gao J, Wang Q, et al. ECRG4 Represses Cell Proliferation and Invasiveness via NFIC/OGN/NF-κB Signaling Pathway in Bladder Cancer. Front Genet 2020;11:846. [Crossref] [PubMed]
- Liu X, Zhang F, Zhang Y, et al. PPM1K Regulates Hematopoiesis and Leukemogenesis through CDC20-Mediated Ubiquitination of MEIS1 and p21. Cell Rep 2018;23:1461-75. [Crossref] [PubMed]
- Zhu Y, Qi X, Yu C, et al. Identification of prothymosin alpha (PTMA) as a biomarker for esophageal squamous cell carcinoma (ESCC) by label-free quantitative proteomics and Quantitative Dot Blot (QDB). Clin Proteomics 2019;16:12. [Crossref] [PubMed]
- Liu NW, Huang X, Liu S, et al. EXT1, Regulated by MiR-665, Promotes Cell Apoptosis via ERK1/2 Signaling Pathway in Acute Lymphoblastic Leukemia. Med Sci Monit 2019;25:6491-503. [Crossref] [PubMed]
- Samarelli AV, Ziegler T, Meves A, et al. Rabgap1 promotes recycling of active β1 integrins to support effective cell migration. J Cell Sci 2020;133:jcs243683. [Crossref] [PubMed]
- Guo Y, Zhang X, Yang M, et al. Functional evaluation of missense variations in the human MAD1L1 and MAD2L1 genes and their impact on susceptibility to lung cancer. J Med Genet 2010;47:616-22. [Crossref] [PubMed]
- Sun Q, Zhang X, Liu T, et al. Increased expression of mitotic arrest deficient-like 1 (MAD1L1) is associated with poor prognosis and insensitive to Taxol treatment in breast cancer. Breast Cancer Res Treat 2013;140:323-30. [Crossref] [PubMed]
- Wang Y, Wang F, He J, et al. miR-30a-3p Targets MAD2L1 and Regulates Proliferation of Gastric Cancer Cells. Onco Targets Ther 2019;12:11313-24. [Crossref] [PubMed]
- Chen JC, Ko JC, Taso YC, et al. Downregulation of Xeroderma Pigmentosum Complementation Group C Expression by 17-Allylamino-17-Demethoxygeldanamycin Enhances Bevacizumab-Induced Cytotoxicity in Human Lung Cancer Cells. Pharmacology 2021;106:154-68. [Crossref] [PubMed]
- Mao K, You C, Lei D, et al. Potential regulation of glioma through the induction of apoptosis signaling via Egl-9 family hypoxia-inducible factor 3. Oncol Lett 2017;13:893-7. [Crossref] [PubMed]
- Yang L, Wu Z, Sun W, et al. CCNDBP1, a Prognostic Marker Regulated by DNA Methylation, Inhibits Aggressive Behavior in Dedifferentiated Liposarcoma via Repressing Epithelial Mesenchymal Transition. Front Oncol 2021;11:687012. [Crossref] [PubMed]
- Twine NA, Harkness L, Kassem M, et al. Transcription factor ZNF25 is associated with osteoblast differentiation of human skeletal stem cells. BMC Genomics 2016;17:872. [Crossref] [PubMed]
- Olalekan S, Xie B, Back R, et al. Characterizing the tumor microenvironment of metastatic ovarian cancer by single-cell transcriptomics. Cell Rep 2021;35:109165. [Crossref] [PubMed]
- Hornburg M, Desbois M, Lu S, et al. Single-cell dissection of cellular components and interactions shaping the tumor immune phenotypes in ovarian cancer. Cancer Cell 2021;39:928-944.e6. [Crossref] [PubMed]
- Zhang QF, Li J, Jiang K, et al. CDK4/6 inhibition promotes immune infiltration in ovarian cancer and synergizes with PD-1 blockade in a B cell-dependent manner. Theranostics 2020;10:10619-33. [Crossref] [PubMed]
- Baci D, Bosi A, Gallazzi M, et al. The Ovarian Cancer Tumor Immune Microenvironment (TIME) as Target for Therapy: A Focus on Innate Immunity Cells as Therapeutic Effectors. Int J Mol Sci 2020;21:3125. [Crossref] [PubMed]
(English Language Editor: L. Huleatt)