Development and validation of the diagnostic accuracy of artificial intelligence-assisted ultrasound in the classification of splenic trauma
Introduction
Due to its fragile texture and abundant blood supply, the spleen is the most vulnerable organ in abdominal trauma. Splenic injury accounts for about 13–25% of abdominal trauma (1). The early symptoms of splenic trauma are not obvious in some patients, but splenic trauma is often accompanied by serious organ problems. If patients are not diagnosed and treated in a timely manner, their lives may be endangered (2-4). Presently, preliminary screening by imaging is the main way to quickly evaluate splenic injury. Imaging examination methods mainly include computed tomography (CT) and ultrasound (US) examinations. CT has a number of advantages, including that it has a high coincidence rate and is not affected by gas interference or patient breathing during the examination. However, CT examinations also have certain limitations. As CT is radioactive, children and pregnant women have an increased risk of exposure to radiation. Additionally, a certain rate of adverse reactions occurs in enhanced CT.
US has become an important examination method for splenic trauma. It can be applied to rapid injury assessments in pre-hospital or emergency rooms and can be used as an evaluation method for post-traumatic treatment. It can also be repeatedly performed to observe the recovery of organs (5,6). US can reveal whether there is organ damage by displaying the continuity of the organ capsule, the presence of subcapsular hematoma, and changes to the echo of the parenchyma. Additionally, US can also indicate whether there is organ damage through some indirect signs, such as retroperitoneal hematoma and peritoneal effusion. Color doppler US can detect abnormal blood supply in the lesions, which improves the detection rate of parenchymal organ injury. However, US examinations are operator dependent and cannot detect some mild traumas. It has been reported in the literature that the sensitivity of conventional US in the diagnosis of parenchymal organ injury is only about 41% (7,8).
Contrast-enhanced ultrasound (CEUS) can detect parenchymal organ injury and active bleeding from multiple abdominal injuries, improve the accuracy of ultrasonic examinations of splenic trauma, provides a more reliable evaluation method for parenchymal organ injury, and makes up for the shortcomings of conventional US (9). Studies have shown that CEUS is as accurate as CT in the detection and staging of traumatic splenic injury (10-12). However, CEUS is an invasive examination, and the contrast agent is expensive; thus, it has some limitations.
As a leading technology for the future, artificial intelligence (AI) is increasingly being applied to all fields. As a popular analysis method of machine learning, deep learning can represent massive data by constructing multi-layer artificial neural networks. Rapid improvement of graphics processing capacity has made the development of more advanced algorithm possible. Such algorithms have a stable and powerful image analysis ability (13,14). Thus, they have been widely used in the diagnosis and recognition of ultrasonic images of various organs, and have been proven to have high accuracy (15-17).
However, deep learning requires a large amount of image data. To solve this problem, we applied the method of transfer learning. The performance of pig spleen in ultrasound is similar to that of human, and the performance of spleen trauma in conventional ultrasound and contrast-enhanced ultrasound is also similar to that of human spleen trauma (18). Therefore, we learn the low-level ultrasonic damage characteristics by building animal models. On the premise that the low-level ultrasonic damage characteristics are unchanged, we migrate the animal models, retrain with a small number of human spleen ultrasonic images, and learn the high-level human spleen damage characteristics, so as to achieve the effect of building a human spleen damage identification model. Although there was no migration from animal to human model in the past studies, However, there are examples of using natural image modeling to migrate medical images (19), using data modeling of other organs or modalities to migrate to lung segmentation models (20), and using 2D image modeling to migrate to 3D images (21). Therefore, we assume that animal models can be established first by collecting a large number of animal spleen trauma images and fine-tuning through human data, so as to make up for the lack of human data. At present, there is no report on the AI-assisted US diagnosis of splenic trauma. This study combined animal experiments and clinical US image data, and applied a deep-learning method to establish a CEUS splenic trauma classification model. We present the following article in accordance with the TRIPOD reporting checklist (available at https://atm.amegroups.com/article/view/10.21037/atm-22-3767/rc).
Methods
Experimental animals
The animal experiments were performed under a project license (No. 2021KY033-KS001) granted by the Medical Ethics Committee of PLA General Hospital, in compliance with National Laboratory Animal Management Regulations and guidelines for the care and use of animals.
In total, 20 Bama miniature pigs, male and female, weighing 15–25 kg, were anesthetized by intramuscular injection with 50 mg/mL of Shutai 50 (tiletamine hydrochloride 125 mg and zolazepam hydrochloride 125 mg) (0.1 mL/kg). After being anesthetized, each animal was fixed on the operating table, the vein access was established in the ear marginal vein, and the abdominal skin was prepared. Heart rate, blood oxygen, and other indicators were monitored. We examed the injuries in the condition of gray scale. Then we collected normal-spleen images in different sections before establishing the trauma model. About 100 images were collected for each organ, and a total of 2,225 spleen images were collected. After the image acquisition, the spleen was impacted by external force to simulate blunt organ injury and establish a splenic trauma model. A total of 49 splenic trauma foci were established.
We used the Mindray M9 portable ultrasonic diagnostic instrument (Mindray Company, China), which has a convex array probe C5-1s, and a probe frequency of 1–5 MHz, and the large ultrasonic diagnostic instrument, Philip EPIQ 7 (Philips, Netherlands), which has a convex array probe C5-1, and a probe frequency of 1–5 MHz. After the establishment of the wound lesions, US contrast agent was administered. The contrast agent used was the SonoVue contrast agent (Bracco, Italy). Normal saline (5 mL) was dissolved in each contrast agent and shaken. The probe was placed in the abdomen, and at the trauma site, the parameters of the ultrasonic instrument were adjusted, and the same parameters were applied to the same trauma focus. The contrast medium (1 mL) was injected into the spleen through the ear vein, followed by normal saline (5 mL). In the arterial phase, the damaged part was observed, the size of the wound was measured, and the dynamic angiography images were collected. The ultrasonic instrument was adjusted to the gray-scale condition, the wound location shown by the contrast agent was explored, its location, boundary, echo, and other ultrasonic manifestations were observed, the size of the wound was measured, and the scope of the wound was determined according to the results of the CEUS. About 100 gray-scale images were collected from different angles and sections of each trauma focus, and 4,280 trauma images were collected from the spleens.
Clinical image collection
The study was conducted in accordance with the Declaration of Helsinki (as revised in 2013). The study was approved by ethics committee of PLA General Hospital (No. S2020-323-01) and informed consent was taken from all the patients. Ultrasonic images of splenic trauma from PLA General Hospital and Beijing Chaoyang Emergency Rescue Center were collected retrospectively (2015.4.10–2021.8.18). All injuries were diagnosed by CEUS, enhanced CT or surgery. A total of 548 images of 149 patients with splenic trauma were collected. Additionally, 652 images of 652 normal spleen patients were collected. After excluding the poor-quality images, 505 spleen-trauma images and 559 normal-spleen images remained. A small number of images (125 images, including 58 spleen-trauma images and 67 normal-spleen images) were randomly selected as the training set. The remaining images were divided into test set 1 and test set 2. The 274 images (133 spleen-trauma images and 141 normal-spleen images) collected at the 1st and 4th Medical Centers of PLA General Hospital were used as test set 1, and the 665 images (314 spleen-trauma images and 351 normal-spleen images) collected by the Beijing Chaoyang Emergency Rescue Center were used as test set 2.
Image annotation
Image annotation was undertaken to establish the classification model, and the normal spleen and spleen-trauma images were sketched with sketching software. For the normal-spleen images, we only needed to draw the outline of the spleen and the edge of the spleen as accurately as possible along the spleen envelope. For the spleen-trauma images, we needed to draw the outline of the spleen and trauma at the same time. The outline of the wound was based on the location and scope of the wound as determined by the results of the CEUS or enhanced CT.
US model building
US static images were collected in JEPG format. Each image was resized to 224×224 pixels. The MobileNet V2 structure was used to construct the US model. The animal CV models were trained by 3-fold cross validation (CV) in the animal training cohorts and animal validation cohorts. Next, some human data were used to fine tune the animal CV models. The whole model was constructed by averaging the prediction ability of the 3 fine-tuned models.
Model construction
The static model was a structure of MobileNet V2 (Appendix 1). MobileNet V2 was used to extract the spatial features (see Figure 1).
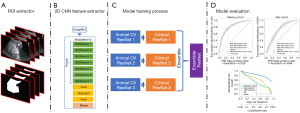
MobileNet V2
The structure of MobileNet V2 (22) is listed in Table S1, and had 7 bottleneck stages. All of the bottleneck stages were constructed by a 2-dimensional convolution layer, batch normalization layer, ReLu activation layer, and depth-wise convolution layer (see Figure 1).
Image evaluation
In this study, 2 experienced doctors (with 10 and 12 years of US examination experience respectively) performed blind evaluations of 382 splenic injury images from clinical test set 2 and classified each image as traumatic or non-invasive. We then compared the US doctors’ image recognition results to those of the CEUS model to evaluate the performance of the model.
Statistical analysis
We used t-tests for the continuous data and χ2-tests for the categorical data to compare the baseline characteristics between test cohorts 1 and 2. A receiver operating characteristic (ROC) curve analysis was conducted to examine the prediction performance of the model. In the CV process, the validation cohorts were used to evaluate the performance of the animal model. Test cohorts 1 and 2 were used to verify the predictive efficacy of the model. Statistical analyses were undertaken using R software (version 3.3.1; R 21 Foundation for Statistical Computing, Vienna, Austria). All the statistical tests were 2-sided, and the statistical significance level was set at P<0.05. The AUC, sensitivity, specificity, negative predictive value, and positive predictive value were used to evaluate the diagnostic performance in diagnosis of spleen trauma.
Results
Results of the animal model
We used 3-fold CVs to establish the animal models. A total of 5,087 images (3,275 spleen-trauma images and 1,812 normal-spleen images) were selected for the 1st training set, and a total of 1,079 images (666 spleen-trauma images and 413 normal-spleen images) were selected for the validation set). Next, 5,549 images (3,532 spleen-trauma images and 2,017 normal-spleen images) were selected for the 2nd training set, and 617 images (409 spleen-trauma images and 208 normal-spleen images) were selected for the validation set. Finally, 5,541 images (3,515 spleen-trauma images and 2,026 normal-spleen images) were selected for the 3rd-fold CV training set, and 625 images (426 spleen-trauma images and 199 normal-spleen images) were selected for the validation set. The areas under the curve (AUCs) of the 3rd-fold model were 0.81 for the training set and 0.70 for the test set (see Table 1, Figure 2).
Table 1
Data | Cohort | AUC | Accuracy | Sensitivity | Specificity | PPV | NPV |
---|---|---|---|---|---|---|---|
Fold 1 | Training cohort | 0.86 (0.86–0.87) | 0.77 (0.77–0.78) | 0.76 (0.74–0.76) | 0.81 (0.80–0.82) | 0.88 (0.87–0.88) | 0.65 (0.63–0.66) |
Test cohort | 0.70 (0.68–0.71) | 0.65 (0.64–0.67) | 0.66 (0.64–0.73) | 0.63 (0.55–0.66) | 0.74 (0.72–0.76) | 0.54 (0.52–0.57) | |
Fold 2 | Training cohort | 0.79 (0.79–0.80) | 0.72 (0.71–0.73) | 0.70 (0.69–0.75) | 0.75 (0.70–0.76) | 0.83 (0.81–0.84) | 0.58 (0.58–0.62) |
Test cohort | 0.74 (0.72–0.76) | 0.72 (0.70–0.74 | 0.74 (0.71–0.81) | 0.67 (0.59–0.71) | 0.81 (0.79–0.84) | 0.57 (0.54–0.63) | |
Fold 3 | Training cohort | 0.81 (0.81–0.82) | 0.73 (0.73–0.76) | 0.71 (0.69–0.78) | 0.77 (0.71–0.80) | 0.84 (0.82–0.86) | 0.61 (0.59–0.65) |
Test cohort | 0.70 (0.67–0.72) | 0.79 (0.78–0.81) | 0.93 (0.92–0.97) | 0.48 (0.43–0.51) | 0.79 (0.77–0.81) | 0.77 (0.74–0.87) |
Data in parentheses are 95% CIs. AUC, area under the curve; PPV, positive predictive value; NPV, negative predictive value.
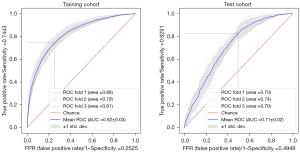
Clinical model establishment
To evaluate the performance of the proposed model, the ROC and DCA curves were plotted (see Figure 3). The ROC curve results showed that the performance of test cohorts 1 (AUC =0.84) and 2 (AUC =0.90) was similar. Based on the DCA curves, while the threshold was <0.8, the proposed model performed better on test cohort 2 than test cohort 1. As Table 2 shows, the test cohort 2 had higher sensitivity (0.82 vs. 0.71, P<0.01) and higher specificity (0.88 vs. 0.81, P<0.01) than test cohort 1.
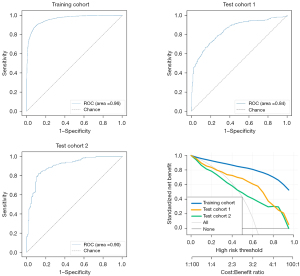
Table 2
Data | AUC | Accuracy | Sensitivity | Specificity | PPV | NPV |
---|---|---|---|---|---|---|
Animal cohort | 0.96 (0.96–0.96) | 0.89 (0.89–0.90) | 0.89 (0.87–0.90) | 0.91 (0.89–0.93) | 0.94 (0.94–0.96) | 0.82 (0.80–0.84) |
Test cohort 1 | 0.84 (0.83–0.86) | 0.76 (0.75–0.78) | 0.71 (0.65–0.87) | 0.81 (0.65–0.87) | 0.77 (0.69–0.83) | 0.76 (0.73–0.85) |
Test cohort 2 | 0.90 (0.89–0.92) | 0.85 (0.83–0.87) | 0.82 (0.78–0.87) | 0.88 (0.83–0.92) | 0.87 (0.82–0.90) | 0.84 (0.81–0.87) |
Data in parentheses are 95% CIs. AUC, area under the curve; PPV, positive predictive value; NPV, negative predictive value.
Doctor’s US recognition results
Next, 2 doctors examined all the normal and abnormal images and divided the recognition results into “traumatic” and “non-invasive”. Taking the final modeling results from test cohort 2 as the standard for convolutional neural network (CNN) recognition, Figure 3 shows the ROC curves of the images recognized by the CNN model and doctors. The accuracy of image recognition by doctor 1 was 0.70 (95% CI: 0.66–0.73), the sensitivity was 0.62 (95% CI: 0.56–0.67), the specificity was 0.78 (95% CI: 0.73–0.83), the positive predictive value was 0.76 (95% CI: 0.71–0.81), and the negative predictive value was 0.64 (95% CI: 0.60–0.69). While the accuracy of image recognition by doctor 2 was 0.74 (95% CI: 0.70–0.77), the sensitivity was 0.66 (95% CI: 0.61–0.71), the specificity was 0.82 (95% CI: 0.78–0.86), the positive predictive value is 0.80 (95% CI: 0.75–0.85), and the negative predictive value was 0.68 (95% CI: 0.63–0.73). The diagnostic sensitivity of the deep-learning model was higher than that of doctor 1 (0.82 vs. 0.62, P<0.001) and doctor 2 (0.82 vs. 0.66, P<0.001), and the specificity was higher than that of doctor 1 (0.88 vs. 0.78, P=0.001) and doctor 2 (0.88 vs. 0.82, P=0.03). The final modeling results for the deep-learning test set 2 are shown in Figure 4 and Table 3.
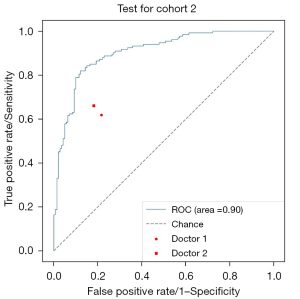
Table 3
Data | Accuracy | Sensitivity | Specificity | PPV | NPV |
---|---|---|---|---|---|
Test cohort 2 | 0.85 (0.83–0.87) | 0.82 (0.78–0.87) | 0.88 (0.83–0.92) | 0.87 (0.82–0.90) | 0.84 (0.81–0.87) |
Doctor 1 | 0.70 (0.66–0.73) | 0.62 (0.56–0.67) | 0.78 (0.73–0.83) | 0.76 (0.71–0.81) | 0.64 (0.60–0.69) |
Doctor 2 | 0.74 (0.70–0.77) | 0.66 (0.61–0.71) | 0.82 (0.78–0.86) | 0.80 (0.75–0.85) | 0.68 (0.63–0.73) |
Data in parentheses are 95% CIs. CNN, convolutional neural network; PPV, positive predictive value; NPV, negative predictive value.
Discussion
As an important imaging method for the injury evaluation of patients with splenic trauma, US plays a very important role in the diagnosis of trauma. In pre-hospital treatment, it can help save lives, as it enables the injuries of patients to be evaluated and medical interventions to be conducted quickly and accurately.
Focused assessment with sonography for trauma (FAST), which is a bedside assessment tool for patients with abdominal trauma, is widely used in clinical practice. This method is mainly used for the assessment of intraperitoneal hemorrhages caused by injuries to the liver, spleen or other organs in patients with trauma. It has high sensitivity to intraperitoneal hemorrhage and can indirectly assess abdominal organ injuries (23). In the diagnosis of abdominal trauma, AI can be used to diagnose organ trauma by FAST (24,25). However, in FAST, it is often difficult to distinguish between peritoneal effusion caused by abdominal organ injury and peritoneal effusion caused by other factors (e.g., tumor or liver cirrhosis), and it is impossible to determine which organ has trauma.
Conventional US diagnosis of organ trauma is not only operator dependent, but also often miss minor traumas. The sensitivity of US diagnosis is relatively low. Thus, as an important examination method of abdominal trauma, it is urgent to improve the sensitivity of ultrasonic diagnosis of visceral trauma. However, there is no relevant report on evaluations of the presence or absence of trauma foci in organs. In this study, AI was used to identify traumatic lesions that are difficult to identify by conventional gray-scale US through feature extraction, internal echo changes of parenchymal organs, and subtle texture changes of images. Its diagnosis accuracy was significantly higher than that of the US doctors.
The establishment of the AI model required a large number of ultrasonic images, but ultrasonic images of splenic trauma are difficult to collect. Thus, we first established an animal model of splenic trauma, and found that the performance of image features of the pig spleen under US was similar to that of human. In the process of establishing the model, to establish different degrees of trauma, we used different forces to impact the spleen area. Thus, some trauma foci were difficult to identify in gray-scale US images, and the trauma lesions needed to be identified by CEUS. Part of the trauma included splenic rupture. In US images, splenic trauma can be classified as hypoechoic, isoechoic, or hyperechoic echogenicity, heterogeneous echogenicity, or other. To simulate different levels of splenic trauma to the greatest extent possible, we obtained a large number of animal spleen-trauma images and established an AI model of splenic trauma through deep learning. For the human spleen-trauma images, we used a multi-center method to gather images collected by different machines and doctors to increase the number of images. Next, we fine-tuned the animal model. To evaluate the performance of this model, we established a test cohort 1 and an independent test cohort 2. We proposed a transfer-learning model with a MobileNet V2 structure and a pre-trained ImageNet weight to predict the splenic trauma from the US images. Transfer learning can not only transfer the information in the original model established by a large amount of data to less data, but can also shorten the training time and achieve higher performance. The transfer-learning time is shorter than the original learning process time required to build the source model (26,27). The model can learn higher features by pre-trained weight. Our results showed that the model was able to predict splenic trauma from US images even in different center cohorts.
This study had some limitations. First, due to the morphological difference in the spleens of different patients, it was necessary to crop the spleen region for further analysis. This will become more automatized in further research. Second, the model was constructed using animal US images, and more clinical images are needed for further correction. Finally, more data from different center cohorts are needed for further study.
In summary, establishing an AI splenic trauma model through transfer learning could significantly improve the ultrasonic diagnostic sensitivity of splenic trauma. Such a model could help clinicians make clear judgments for patients with splenic trauma, especially pre-hospital patients. When enhanced CT and other examinations cannot be carried out, routine US examination and AI-assisted diagnosis can be used to quickly evaluate injuries, which is conductive to the clinical adoption of a reasonable treatment plan and is of great significance to improve the survival rate of patients. This method is convenient and non-invasive and can be applied to pre-hospital diagnoses, post-treatment injury evaluations, and regular follow-ups. We still need to collect additional clinical data from more centers and adjust the original model to increase the accuracy of the diagnoses.
Acknowledgments
Funding: The study was supported by Clinical Research Support Fund of PLA General Hospital (No. ZH19021) and the Major Project of Military Logistical Support Department (No. ALB19J001).
Footnote
Reporting Checklist: The authors have completed the TRIPOD reporting checklist. Available at https://atm.amegroups.com/article/view/10.21037/atm-22-3767/rc
Data Sharing Statement: Available at https://atm.amegroups.com/article/view/10.21037/atm-22-3767/dss
Conflicts of Interest: All authors have completed the ICMJE uniform disclosure form (available at https://atm.amegroups.com/article/view/10.21037/atm-22-3767/coif). HL is from Beijing Mindray Medical Instrument Co., Ltd. The other authors have no conflicts of interest to declare.
Ethical Statement: The authors are accountable for all aspects of the work in ensuring that questions related to the accuracy or integrity of any part of the work are appropriately investigated and resolved. The animal experiments were performed under a project license (No. 2021KY033-KS001) granted by the Medical Ethics Committee of PLA General Hospital, in compliance with National Laboratory Animal Management Regulations and guidelines for the care and use of animals. The study was conducted in accordance with the Declaration of Helsinki (as revised in 2013). The study was approved by ethics committee of PLA General Hospital (No. S2020-323-01) and informed consent was taken from all the patients.
Open Access Statement: This is an Open Access article distributed in accordance with the Creative Commons Attribution-NonCommercial-NoDerivs 4.0 International License (CC BY-NC-ND 4.0), which permits the non-commercial replication and distribution of the article with the strict proviso that no changes or edits are made and the original work is properly cited (including links to both the formal publication through the relevant DOI and the license). See: https://creativecommons.org/licenses/by-nc-nd/4.0/.
References
- Tagliati C, Argalia G, Polonara G, et al. Contrast-enhanced ultrasound in delayed splenic vascular injury and active extravasation diagnosis. Radiol Med 2019;124:170-5. [Crossref] [PubMed]
- Uranues S, Kilic YA. Injuries to the Spleen. Eur J Trauma Emerg Surg 2008;34:355. [Crossref] [PubMed]
- McKechnie PS, Kerslake DA, Parks RW. Time to CT and Surgery for HPB Trauma in Scotland Prior to the Introduction of Major Trauma Centres. World J Surg 2017;41:1796-800. [Crossref] [PubMed]
- Pothmann CEM, Sprengel K, Alkadhi H, et al. Abdominal injuries in polytraumatized adults: Systematic review. Unfallchirurg 2018;121:159-73. [Crossref] [PubMed]
- Richards JR, McGahan JP. Focused Assessment with Sonography in Trauma (FAST) in 2017: What Radiologists Can Learn. Radiology 2017;283:30-48. [Crossref] [PubMed]
- Doody O, Lyburn D, Geoghegan T, et al. Blunt trauma to the spleen: ultrasonographic findings. Clin Radiol 2005;60:968-76. [Crossref] [PubMed]
- Valentino M, Serra C, Zironi G, et al. Blunt abdominal trauma: emergency contrast-enhanced sonography for detection of solid organ injuries. AJR Am J Roentgenol 2006;186:1361-7. [Crossref] [PubMed]
- Poletti PA, Kinkel K, Vermeulen B, et al. Blunt abdominal trauma: should US be used to detect both free fluid and organ injuries? Radiology 2003;227:95-103. [Crossref] [PubMed]
- Jaspers N, Holzapfel B, Kasper P. Abdominal ultrasound in emergency and critical care medicine. Med Klin Intensivmed Notfmed 2019;114:509-18. [Crossref] [PubMed]
- Miele V, Piccolo CL, Galluzzo M, et al. Contrast-enhanced ultrasound (CEUS) in blunt abdominal trauma. Br J Radiol 2016;89:20150823. [Crossref] [PubMed]
- Di Serafino M, Iacobellis F, Schillirò ML, et al. The Technique and Advantages of Contrast-Enhanced Ultrasound in the Diagnosis and Follow-Up of Traumatic Abdomen Solid Organ Injuries. Diagnostics (Basel) 2022;12:435. [Crossref] [PubMed]
- Tagliati C, Argalia G, Graziani B, et al. Contrast-enhanced ultrasound in the evaluation of splenic injury healing time and grade. Radiol Med 2019;124:163-9. [Crossref] [PubMed]
- Akkus Z, Cai J, Boonrod A, et al. A Survey of Deep-Learning Applications in Ultrasound: Artificial Intelligence-Powered Ultrasound for Improving Clinical Workflow. J Am Coll Radiol 2019;16:1318-28. [Crossref] [PubMed]
- Xiao T, Liu L, Li K, et al. Comparison of Transferred Deep Neural Networks in Ultrasonic Breast Masses Discrimination. Biomed Res Int 2018;2018:4605191. [Crossref] [PubMed]
- Zhou H, Jin Y, Dai L, et al. Differential Diagnosis of Benign and Malignant Thyroid Nodules Using Deep Learning Radiomics of Thyroid Ultrasound Images. Eur J Radiol 2020;127:108992. [Crossref] [PubMed]
- Li J, Bu Y, Lu S, et al. Development of a Deep Learning-Based Model for Diagnosing Breast Nodules With Ultrasound. J Ultrasound Med 2021;40:513-20. [Crossref] [PubMed]
- Zhu YC, AlZoubi A, Jassim S, et al. A generic deep learning framework to classify thyroid and breast lesions in ultrasound images. Ultrasonics 2021;110:106300. [Crossref] [PubMed]
- Feng C, Wang L, Huang S, et al. Application of Contrast-Enhanced Real-time 3-Dimensional Ultrasound in Solid Abdominal Organ Trauma. J Ultrasound Med 2020;39:869-74. [Crossref] [PubMed]
- Raghu M, Zhang C, Kleinberg J, et al. Transfusion: Understanding transfer learning for medical imaging. In: Advances in neural information processing systems. 2019:3347-57.
- Chen S, Ma K, Zheng Y. Med3d: Transfer learning for 3d medical image analysis. arXiv preprint arXiv:1904.00625. 2019.
- Taleb A, Loetzsch W, Danz N, et al. 3D Self-Supervised Methods for Medical Imaging. arXiv preprint arXiv:2006.03829. 2020.
- Sandler M, Howard A, Zhu M, et al. Mobilenetv2: Inverted residuals and linear bottlenecks. Proceedings of the IEEE conference on computer vision and pattern recognition; 2018:4510-20.
- Abu-Zidan FM, Shalak HS, Alhaddad MA. A diagnostic negative ultrasound finding in blunt abdominal trauma. Turk J Emerg Med 2018;18:125-7. [Crossref] [PubMed]
- Cheng CY, Chiu IM, Hsu MY, et al. Deep Learning Assisted Detection of Abdominal Free Fluid in Morison's Pouch During Focused Assessment With Sonography in Trauma. Front Med (Lausanne) 2021;8:707437. [Crossref] [PubMed]
- Lin Z, Li Z, Cao P, et al. Deep learning for emergency ascites diagnosis using ultrasonography images. J Appl Clin Med Phys 2022;23:e13695. [Crossref] [PubMed]
- Mori M, Ariji Y, Katsumata A, et al. A deep transfer learning approach for the detection and diagnosis of maxillary sinusitis on panoramic radiographs. Odontology 2021;109:941-8. [Crossref] [PubMed]
- Zhou H, Wang K, Tian J. Online Transfer Learning for Differential Diagnosis of Benign and Malignant Thyroid Nodules With Ultrasound Images. IEEE Trans Biomed Eng 2020;67:2773-80. [Crossref] [PubMed]
(English Language Editor: L. Huleatt)