Nicotinic acid supplementation contributes to the amelioration of Alzheimer’s disease in mouse models
Introduction
Alzheimer’s disease (AD) is a degenerative disease of the central nervous system (CNS) characterized by progressive cognitive dysfunction, and it is also the most common cause of dementia. Epidemiological surveys have shown that approximately 47 million people worldwide suffer from dementia. This is estimated to increase until 2050 (1). The high prevalence rate of AD and the low rate of medical visits have brought a huge economic burden to society and families. There was still a lack of effective treatments for AD, and the early diagnosis and intervention in the pre-clinical stage are essential (2-5).
In recent years, a series of clinical trials that have been carried out to eliminate or reduce amyloid deposits have not significantly improved the cognitive function of AD patients, and some have even resulted in adverse events (6). Non-pharmacological interventions for AD have attracted the attention of an increasing number of researchers (7-10). Nutritional intervention is a critical component of nonpharmacological treatment (11-15). Dietary composition and nutritional components are associated with the onset of AD (16-18). Currently, the ketogenic and Mediterranean diets are the most extensively explored dietary structures (19-22). The ketogenic diet may raise the concentration of ketone bodies in the plasma, therefore correcting the dysregulated energy metabolism and boosting glucose metabolism in the brain. Phillips et al. conducted a randomized trial to investigate the impact of a ketogenic diet in patients with uniform diagnoses of AD. The research showed high rates of retention, adherence, and safety in applying a 12-week modified ketogenic diet to AD patients. Patients on the ketogenic diet improved their mean within-individual AD Cooperative Study-Activities of Daily Living (ADCS-ADL) and Quality of Life in AD (QOL-AD) scores, compared to those on a usual diet supplemented with low-fat healthy-eating guidelines; the ACE-III score rose somewhat, but not substantially. Cardiovascular risk factor changes were mostly beneficial, and side effects were minor (19). Neth et al. investigated the effects of the ketogenic diet and the American Heart Association-recommended diet on cerebrospinal fluid (CSF) fluid biomarkers: neuroimaging indications and cognitive performance in 20 AD risk categories. The findings indicated that individuals on the ketogenic diet had increased levels of ketone bodies in their cerebrospinal fluid. Aβ42 levels rose, but tau levels fell. Additionally, the higher levels of cerebral perfusion and brain ketone bodies suggest that the ketogenic diet may have a protective impact on cognitive performance in persons at risk of AD (23).
The Mediterranean diet emphasizes the consumption of vegetables, fruits, legumes, and whole grains while minimizing red meat consumption. Olive oil is recommended, as are fewer processed meals. Long-term Mediterranean diet adherence has been found to significantly minimize the risk of cognitive impairment. A total of 51,529 American males aged 40–75 years were enrolled in a large study. A long follow-up period revealed that adhering to the Mediterranean diet may help reduce subjective memory loss. While Norwitz et al. summarized the limitations of the current major human randomized crossover trial, which revealed that interaction among genes, environment and lifestyle including diet style are associated with AD (24).
Along with dietary structure, functional foods with specialized nutrients and specific nutritional and healthcare activities (25-27), such as plant polyphenols, flavonoids, ginsenosides, oligopeptides, spermidine, and vitamins, may also boost cognitive performance. Unsaturated fatty acids, for example, have anti-Aβ aggregation, anti-aging, anti-oxidative damage, and anti-inflammatory properties (28,29).
Niacin is a critical vitamin (PP), occurring as nicotinamide (NAM) (pyridine-3-carboxyamide) and nicotinic acid (NA) (pyridine-3-carboxylic acid). Niacin is mostly found in food as a coenzyme. It is the precursor for nicotinamide adenine dinucleotide (NAD) and nicotinamide adenine dinucleotide phosphate (NADP). Niacin is present in a wide variety of foods, including animal and plant items. In tissues, niacin is transformed into NAD and NADP, which are involved in a wide variety of cellular functions. NAM is formed in the body when adenine, ribose, and phosphoric acid combine to produce coenzyme I and coenzyme II, and it works as an electron transporter and hydrogen transmitter in biological redox processes. Although data on niacin deficiency in human disease are few, the clinical signs, which include dermatitis, diarrhea, dementia, and mortality, are well known. Recent research has shown that niacin is beneficial in the prevention and treatment of aging, cancer, metabolic illnesses, depression, and schizophrenia. By supplementing with niacin, cognitive impairment caused by niacin deficiency may be improved. This shifts our perspective away from niacin as a vitamin and toward its potential as a medicinal agent (30,31).
There are three main biosynthetic pathways for NAD+, kynurenine pathway (KP), Preiss-Handler pathway and salvage pathway. The KP is one of the de novo biosynthetic pathways for NAD+, and mammalian cells can generate NAD+ from dietary tryptophan (Trp) by the KP (32). The Preiss-Handler pathway can convert dietary NA to nicotinate mononucleotide (NAMN) by nicotinic acid phosphoribosyl transferase (NAPRT) (33-35). In the salvage pathway, NAD+ is recycled from NAM, NA, nicotinamide riboside (NR) and nicotinamide mononucleotide (NMN) in the salvage pathway to maintain the cellular NAD+ levels. Current researches focused more on the salvage pathway, NMN and NR. Research on the Preiss-Handler pathway is still lacking. Maybe, NA supplementation is safer and more accessible. Therefore, we conducted this research to identify the role of NA supplementation in AD (36,37).
Weighted co-expression network analysis (WGCNA) is a systems biology technique that is valuable for the description of the connection of gene expression in microarray datasets, which may be used to investigate disease causes and treatment targets. We downloaded the microarray data (GSE135999) from the Gene Expression Omnibus (GEO) database for NAD supplementation of the APP/PS1 AD mice model. Then, using WGCNA, we created co-expression networks to discover the gene network modules associated with niacin supplementation and AD. Additionally, using the cluster Profiler and DOSE packages, Gene Ontology (GO) and pathway enrichment analyses were conducted on the common genes found in the niacin supplement and AD-linked modules. Finally, we used the Search Tool for the Retrieval of Interacting Genes (STRING) database to design a network of protein-protein interactions (PPIs) and identified the network’s hub genes. Simultaneously, behavioral studies were conducted to verify the model. We present the following article in accordance with the ARRIVE reporting checklist (available at https://atm.amegroups.com/article/view/10.21037/atm-22-1148/rc).
Methods
Data gathering and pre-processing
The data for this investigation were obtained from the NCBI’s GEO database, entry number GSE135999 [Hou Y, Lautrup S, Wang Y, Cordonnier S, Wei Y, Yang B, Mattson M, Croteau D, and Bohr V (August 18, 2021), GEO Accession viewer; https://www.ncbi.nlm.nih.gov/geo/query/acc.cgi?acc=GSE135999]. The platform was Agilent-074809 SurePrint G3 Mouse GE v2 8×60 K Microarray. A total of 24 wild-type (WT) and 24 APP/PS1 AD mice are included in the GSE135999 dataset. WT and APP/PS1 AD mice (n=5–7 per group) between 7 and 12 months of age were given either NR (12 mM) or nothing (CTR) in their drinking water. Hippocampi and cortical tissues were then taken for RNA extraction and microarray analysis.
The R software (version 3.5.3) and Bioconductor packages were used to handle the raw expression data, create expression matrices, and match probes to their respective gene symbols. The normalized data were downloaded and the expression matrix was calculated, as well as data filtering. R software was used to search for differential genes using the student’s t-test statistical approach and the following selection condition: t-test P value of 0.05.
Constructing a weighted gene co-expression network and detecting modules
We generated unsigned co-expression networks in R using the WGCNA algorithms. The WGCNA package’s blockwise modules function was used to identify co-expression modules in a one-step network design technique (38,39). Prior to the building of the co-expression networks, the flash-Clust program in R language was used to conduct a hierarchical clustering analysis of the data using a suitable threshold value for detecting and excluding outliers. Pearson’s correlations were calculated to generate a matrix of gene correlations among the dataset’s 11,330 chosen genes.
According to the scale-free topology requirement, a soft thresholding power was selected for the adjacency matrix, which was generated using the WGCNA’s pickSoft Threshold function (40). We chose an adjacency cutoff value of 0.8. Then, using the adjacency matrix, the topological overlap matrix (TOM) was generated. A dissimilarity matrix was utilized in conjunction with a dynamic tree-cutting method to identify gene modules [gene sets with a high degree of topological overlap (TO)] (41). To generate modules of reasonable size, the minimum number of genes was set to 1,000, and a cutline was determined in order to combine modules with comparable expression patterns.
We assessed the connection between module eigengenes (MEs) and clinical characteristics and looked for the most significant links between AD and WT, the hippocampus and cortex, and treatment with NR or CTR. MEs were calculated using the first principal component, which was chosen since it was thought to be representative of module gene expression patterns. For each module, we defined module membership (MM) as the correlation between the gene expression profile and ME, and gene significance (GS) as the size of the relationship between the gene and clinical features. Genes with a high MM were assigned to modules, and modules with a high GS and a P value of 0.05 were shown to be significantly linked with the clinical features in this study.
To validate the stability of WGCNA, we calculated the module’s preservation using the module preservation function (42). Prior to the module preservation analysis, the GSE135999 dataset’s outlier samples were eliminated. Statistics of the preservation Zsummary of >10 indicate a high probability of preservation in the module’s test dataset. 2< Zsummary <10 indicates that there are insufficient data to moderate evidence for module preservation. Zsummary <2 shows that there is no indication of module preservation. The preservation median rank is inversely proportional to the module’s preservation. Then, genes associated with AD and NR preservation modules were identified as promising candidates for additional research (43).
Analysis of functional enrichment in the co-expression modules
To further investigate the biological functions of the shared genes in the selected AD and NR modules with a high correlation, we used the clusterProfiler and DOSE packages in R to perform GO term enrichment analysis and Kyoto Encyclopedia of Genes and Genomes (KEGG) pathway analysis (44,45). Statistical significance was defined as a P value less than 0.05.
Identifying and validating hub genes in the key modules
Genes with substantial connections to clinical characteristics and a high degree of network connectivity were referred to informally as intramodular hub genes of the modules. We identified common genes in the AD and NR modules for further investigation in this work. Then, we used the online STRING database 11.5 (https://www.string-db.org/); all of the common genes from the chosen relevant modules were further studied to create the network using a PPI analysis. The PPI network was constructed using a composite score greater than 0.4 and displayed using Cytoscape3.9.0 (46). The node degree was used to search for common genes in the networks, and the genes with the greatest contacts were referred to as hub genes.
In vivo experiments
We carried out validation of the dietary supplementation of niacin in AD transgenic mice. We selected 6 WT and 12 APP/PS1 AD mice, all of the mice are 8 months old. The 12 AD mice were treated with niacin acid supplement diet or normal food. The APP/PS1 double-transgenic mice were purchased from Guangdong Medical Laboratory Animal Center. They were created by integrating a human/murine APP construct with the Swedish double mutation and the exon-9-deleted PSEN1 mutant (APPswe + PSEN1/dE9). APP/PS1 and their littermates (WT) were used in this study. WT C57BL/6 mice were purchased from the Animal Center of Guangdong Medical Laboratories. All mice were maintained in a pathogen-free environment. All animal trials were authorized by the Animal Ethics Committee at Xiangya Hospital, Central South University. Mice of the same sex were kept four to a cage on a 12 h light/dark cycle with free access to food and water. All in vivo tests were conducted on matched sexes and ages. The experimental conditions were allocated randomly to the mice. The sample sizes were determined mostly via experience with comparable sorts of investigations. All animal studies were carried out during the daytime. Experiments were performed under a project license (No. 2020XY-GLMC030211) granted by the Institutional Review Board of the Animal Ethics Committee at Xiangya Hospital, Central South University, in compliance with the rules of the National Science and Technology Commission of the People’s Republic of China on the care and use of animals.
Mice were divided into different groups in a blinded manner by QL. The mice were treated with or without niacin acid from 8 to 14 months of age, half of the mice received approximately 360 mg/kg/day of niacin acid (Sigma-Aldrich, St. Louis, MO, USA) dissolved in drinking water or vehicle (water). Water consumption was monitored every four days, and the niacin content in the water was adjusted accordingly. At all times, food was available ad libitum. The following summarizes the macronutrient makeup of tailored typical diets on a per-calorie basis: 10% protein, 13% fat, and 77% carbs.
From 14 months old, their learning and spatial memory were conducted using a Morris water maze (47). Spatial learning and memory were evaluated in a Morris water maze (120 cm in circumference, 50 cm in height, and 30 cm in depth) full of white water (22 ℃) and accompanied by distant additional maze stimuli. The day before training, the animals were handled to acclimate them to the water labyrinth pool; this day is referred to as day 0. Mice were next introduced to water and swimming on a familiarization day, during which they were required to locate a visible platform in the pool. To assess visuomotor impairments on day 0, mice were given four trials (60 s cutoff) in the Morris water maze to locate a visible platform indicated by a cue. The animals were required to identify the submerged platform utilizing distal additional maze cues after the training sessions (days 1–5). They were trained for five consecutive days with four trials each day (60 s cutoff). To improve spatial learning, mice were introduced in a randomized daily sequence from four separate beginning positions. An automated video tracking system recorded the speed, latency, and distance required to reach the platform, as well as the swim route for each trial (SuperMaze, Shanghai XR). All the mice were fed for further study.
Statistical analysis
SPSS Statistics 24 and GraphPad Prism 8.0 were used for statistical analysis. The data shown are the mean ± standard error of mean (SEM), with P<0.05 considered statistically significant. Group differences were analyzed with a one-way analysis of variance (ANOVA), followed by a Bonferroni multiple comparisons test for multiple groups.
Results
Data filtering and preprocessing
The data of GSE135999 were selected and divided into two groups according to AD and WT. There were 24 samples from the AD group and 24 from the normal group. We obtained the GEO standardized data series matrix. Then, we searched for differentially expressed genes (DEGs) using the R language and the t-test (Student’s t-test) statistical approach, with a P value of 0.05 as the selection criteria. There were 59,305 genes in the sample; following data cleaning, we retained 11,330 genes for further analysis. By analyzing the microarray findings from the GSE135999 dataset, we discovered 5,222 up-regulated and 6,108 down-regulated genes. Volcano plots were drawn to show the DEGs in Figure S1.
WGCNA construction and key module
We found 13 modules in various hues using unsupervised WGCNA, which identifies groupings (called modules) of co-expressed genes across a population of data (Figure 1A). Each module consists of a collection of genes that have a high degree of co-expression, as indicated by a high TO. Modules are discovered using a measure of dissimilarity (i.e., 1 TO) and hierarchical clustering. Genes inside a module have much more co-expression (either positive or negative) than genes outside the module (48).
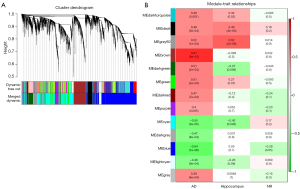
To determine whether the modules were substantially connected with the clinical characteristics, we determined the Pearson’s correlation coefficient between each module and each phenotype (Figure 1B). In addition, we also identified the relationship between the modules and the relationship between the modules and samples (Figure S2). Correlations between MEs and each phenotype AD-WT, hippocampus–cortex, and treatment with NR-CTR were evaluated to determine which modules are significantly associated with clinical features.
As seen in Figure 1B, there were nine modules that were substantially linked with AD in the first column. The results indicate that the dark turquoise, black, grey60, brown, dark green, green, dark red, purple, and grey modules were positively correlated with AD, whereas the cyan, dark grey, blue, and light cyan modules were negatively correlated with AD, indicating that genes in the positively related module were predominantly over-expressed, whereas genes in the negatively related module were predominantly under-expressed. According to the calculation method settings, we assigned AD to 1 and WT to 0. If it is positive in the AD, this means that it is invisible in the WT, and vice versa.
In the second column, there were five modules that were substantially associated with various brain regions (hippocampus-cortex). The results indicate that the dark turquoise, black, and grey60 modules were positively correlated with the hippocampus, whereas the dark green and cyan modules were negatively correlated with the hippocampus, indicating that genes in the positively related module were predominantly over-expressed, whereas genes in the negatively related module were predominantly under-expressed. According to the calculation method settings, we assigned the hippocampus to 1 and the cortex to 0. If it is positive in the hippocampus, this means that it is negative in the cortex, and vice versa.
In the third column, there was one module significantly correlated with NR. The results indicate that the blue module was negatively correlated with NR, meaning that genes in the negatively related module were mostly under-expressed. According to the calculation method settings, we assigned the NR to 1 and the CTR to 0. If it is positive in the NR, this means that it is invisible in the CTR, and vice versa. Considering that the module screening came from the screening of differential genes between AD and WT, this means that the gene modules that are lowly expressed in the third column are negatively correlated with the onset of AD.
We picked three modules (brown, grey60, and blue) for further research based on their clinical significance.
Analysis of function enrichment in the key modules
We focused on the effect of niacin in AD, so we chose the MEblue module for function enrichment analysis. We extracted all genes from the MEBlue module and determined the common genes. Then, enrichment analysis was used to investigate the GO keywords and pathways associated with the common genes. The enrichment of GO terms was determined using the clusterProfiler and DOSE packages in R (Figure 2A). As shown by the data, the shared genes associated with NR and CTR are mostly involved in the control of neuron projection development, including neuron-to-neuron synapse formation, RNA splicing, axonogenesis, and small GTPase-mediated signal transduction.
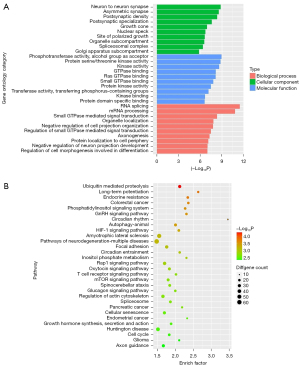
In order to determine the pathways of common genes, KEGG pathway analysis was conducted (Figure 2B). The shared genes are mostly involved in pathways including ubiquitin-mediated proteolysis, circadian rhythm, long-term potentiation, endocrine resistance autophagy, the HIF1 signaling pathway, and amyotrophic lateral sclerosis. While some of these molecular mechanisms have been documented in several publications, others, such as circadian rhythm, long-term potentiation, and autophagy, are novel to us.
Detection of hub genes
We carried out the establishment and analysis of PPI networks. PPI networks were created with the help of the STRING database 11.5 (https://www.string-db.org/). The hub genes were found using Cytoscape3.9.0 based on their nodal degree (number of genes associated with the target gene) (Figure 3). The top 10 genes involved in PPIs were designated as hub genes. Finally, as indicated in Table 1, we discovered ten hub genes (Ctnnb1, Rps24, Mdm2, Crebbp, Rps13, Itgb1, Gnb2l1, Pten, Rptor, and Map1lc3b).
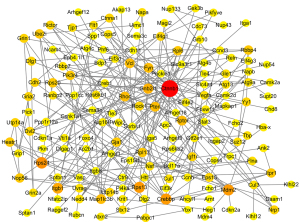
Table 1
Gene | GeneCards ID | Gene name | Diseases involved |
---|---|---|---|
Ctnnb1 | GC03P041236 | Catenin beta 1 | Pilomatrixoma and neurodevelopmental disorder with spastic diplegia and visual defects |
Rps24 | GC10P078033 | Ribosomal protein S24 | Diamond-Blackfan anemia 3 and Diamond-Blackfan anemia |
Mdm2 | GC12P068808 | MDM2 proto-oncogene | Lessel-Kubisch syndrome and accelerated tumor formation |
Crebbp | GC16M005093 | CREB binding protein | Rubinstein-Taybi syndrome 1 and Menke-Hennekam syndrome 1 |
Rps13 | GC11M017384 | Ribosomal protein S13 | Hypertensive encephalopathy and neuroblastoma |
Itgb1 | GC10M032909 | Integrin subunit beta 1 | Gallbladder cancer and breast cancer |
Gnb2l1 | GC05M181511 | Receptor for activated C kinase 1 | Wells’ syndrome and lung cancer |
Pten | GC10P087863 | Phosphatase and tensin homolog | Cowden syndrome 1 and macrocephaly/autism syndrome |
Rptor | GC17P080544 | Regulatory associated protein of MTOR complex 1 | Tuberous sclerosis 1 and tuberous sclerosis |
Map1lc3b | GC03P041236 | Catenin beta 1 | Pilomatrixoma and neurodevelopmental disorder with spastic diplegia and visual defects |
GeneCards identifiers and gene-related diseases are from the GeneCards database (https://www.genecards.org/).
Niacin-supplemented foods (NiSF) improved the cognitive ability of AD mice in a Morris water maze
To investigate the effect of NiSF on the behavior of the APP/PS1 mice, their performance in the Morris water maze was measured. In the Morris water maze training phase, the APP/PS1 mice spent more time finding the hidden platform, while the NiSF-Group APP/PS1 mice required less time to find the platform, showing that NiSF improves learning in APP/PS1 mice (Figure 4A,4B). Data: mean ± SEM. Statistical significance was performed with a one-way ANOVA followed by Bonferroni’s multiple comparison test.
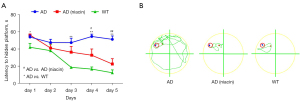
Discussion
We investigated the impact of niacin on AD mice using a systems biology approach combining WGCNA with behavioral tests. Our findings show that, in addition to behavioral and physiological profiling, WGCNA may be a helpful method for assessing nutritional effectiveness in human neurodegenerative and neuropsychiatric disorders. Traditional biological research focuses on understanding disease etiology at the molecular level and is incapable of completely and efficiently exploring the biological meaning contained in vast amounts of data. As WGCNA is a complete network-based systems biology technique, it offers a number of benefits over standard transcriptional studies, including the ability to provide more trustworthy findings. WGCNA also identifies the most highly linked or central genes within each module, referred to as “hubs”, inside groupings of highly coexpressed genes (“modules”). Modules are the fundamental functional components of the transcriptional network. Ten hub genes were found (Ctnnb1, Mdm2, Crebbp, Rps13, Itgb1, Gnb2l1/RACK1, Pten, Rptor, and Map1lc3b).
CTNNB1 is a critical downstream component of the canonical Wnt signaling pathway, which is associated with the insulin internalization pathway CDK2-PTPN6-CTNNB1-CEACAM1 (49). Wnt-signaling can inhibit GSK3β, permitting β-catenin to accumulate in the cytoplasm and translocate into the nucleus to mediate the transcription of genes involved in the pathogenesis of AD (50). CTNNB1 is negatively regulated by APC, transducing wingless signals and interacting with components of the LEF1 and TCF4 transcription complexes, urokinase, and PSEN1. Both the Wnt signaling system and PSEN1 have been linked to degenerative disorders in research. Numerous degenerative genetic illnesses are caused by mutations in Wnt signaling components, either somatic or hereditary (51). PSEN1 and PSEN2 mutations, as well as APP (amyloid precursor protein) mutations, all play significant roles in AD. Disease-associated mutations result in an increase in the synthesis of A in its longer form. PSEN1, which catalyzes the formation of CTNNB1 and CTNND1, is extensively expressed at low levels throughout the CNS, primarily in the neocortex, dentate gyrus, hippocampus, cerebellar cortex, and deep nuclei (52). PSEN1 may catalyze the intramembrane cleavage of integral membrane proteins such as Notch receptors and APP, although this activity is dependent on the presence of other members of the gamma-secretase complex. PSEN1 contributes to Notch and Wnt signaling cascades and downstream processes by processing critical regulatory proteins and by modulating cytosolic CTNNB1 levels.
Both MDM2 and CREBBP are involved in post-translational modifications.
Premature aging is associated with MDM2/p53 axis malfunction (53), which encodes a nuclear-localized E3 ubiquitin ligase that catalyzes p53/TP53 ubiquitination and destruction by the proteasome. By binding to its transcriptional activation domain, it inhibits p53/TP53- and p73/TP73-mediated cell cycle arrest and apoptosis (54).
CREBBP acetylates histones, hence providing a unique tag for transcriptional activation (55). Additionally, this enzyme acetylates non-histone proteins such as DDX21, IRF2, and FOXO1 (56). FOXO1 transcription factor serves as the primary target of insulin signaling and is involved in the regulation of metabolic balance in response to oxidative stress (57,58). The Cdk1-FOXO1 signaling pathway may have a number of roles in maintaining cellular homeostasis, including regulating neuronal death and degeneration throughout brain development and illness. Additionally, CREBBP functions as a circadian transcriptional coactivator, amplifying the activity of circadian transcriptional activators: Heterodimers of NPAS2-ARNTL/BMAL1 and CLOCK-ARNTL/BMAL1 (59).
Gnb2l1/RACK1 induces apoptosis by enhancing BAX oligomerization and disrupting BAX-BCL2L interactions. Gnb2l1/RACK1 is associated with microbial infection because it binds to Y.pseudotuberculosis yopK, inhibiting phagocytosis and bacterial survival after host cell infection. This suggests that Gnb2111/RACK1 may be associated with the inflammatory theory of AD.
PTEN acts as a critical regulator of the AKT-mTOR signaling pathway, regulating the rate of integration of newborn neurons during adult neurogenesis, including proper neuron placement, dendritic growth, and synaptic formation. Through crosstalk, shortcuts, and feedback loops with AMPK and Ulk1/2, mTOR controls autophagy (60). Autophagy is implicated in the aging process and the development of neurodegenerative disorders. PTEN promotes COX activity and ATP synthesis in the mitochondrial energy metabolism by collaborating with isoform 1 to increase PINK1 protein levels. PINK1 is hypothesized to protect cells against mitochondrial malfunction caused by stress. Mutations in this gene induce an autosomal recessive variant of Parkinson’s disease with early onset. Numerous studies have shown a link between mitochondrial energy metabolism and aging and neurodegeneration. The PTEN-AKT-mTOR-AMPK axis is involved in autophagy, and enhanced autophagy may contribute to cognitive improvement.
The related pathways were ubiquitin-mediated proteolysis, circadian rhythm, long-term potentiation, endocrine resistance autophagy, the HIF-1 signaling pathway, amyotrophic lateral sclerosis, and pathways of multiple neuro-degeneration diseases.
Together, our research suggests that niacin improves AD mice’s cognitive ability through multiple mechanisms: reducing Aβ formation, improving mitochondrial metabolism, regulating autophagy, and reducing inflammation, which has been reported. Our research also found that niacin can improve AD cognitive ability through circadian rhythm, ubiquitin-mediated proteolysis, and long-term potentiation.
Conclusions
Our study indicates that WGCNA may be a useful tool for evaluating nutrient supplements for interventions in human neurodegenerative and neuropsychiatric diseases. Niacin may improve AD cognitive ability through multiple mechanisms. This provides a new insight into the research of nutritional intervention in AD. In order to eliminate the potential limitations of the study, further studies and validation of the hub genes are required. Further study is needed to determine if NA supplements are suitable for healthy individuals, which calls for larger clinical controlled study.
Acknowledgments
Funding: This research was funded by the National Natural Science Foundation of China (No. 81760541); Foundation of Guangxi Key Laboratory of Brain and Cognitive Neuroscience in China (No. GKLBCN-20190105-05); Foundation of Guangxi Key Laboratory of Diabetic Systems Medicine in China (No. GKLCDSM-20220101-07).
Footnote
Reporting Checklist: The authors have completed the ARRIVE reporting checklist. Available at https://atm.amegroups.com/article/view/10.21037/atm-22-1148/rc
Conflicts of Interest: All authors have completed the ICMJE uniform disclosure form (available at https://atm.amegroups.com/article/view/10.21037/atm-22-1148/coif). The authors have no conflicts of interest to declare.
Ethical Statement: The authors are accountable for all aspects of the work in ensuring that questions related to the accuracy or integrity of any part of the work are appropriately investigated and resolved. Experiments were performed under a project license (No. 2020XY-GLMC030211) granted by the Institutional Review Board of the Animal Ethics Committee at Xiangya Hospital, Central South University, in compliance with the rules of the National Science and Technology Commission of the People’s Republic of China on the care and use of animals.
Open Access Statement: This is an Open Access article distributed in accordance with the Creative Commons Attribution-NonCommercial-NoDerivs 4.0 International License (CC BY-NC-ND 4.0), which permits the non-commercial replication and distribution of the article with the strict proviso that no changes or edits are made and the original work is properly cited (including links to both the formal publication through the relevant DOI and the license). See: https://creativecommons.org/licenses/by-nc-nd/4.0/.
References
- Jia J, Xu J, Liu J, et al. Comprehensive Management of Daily Living Activities, behavioral and Psychological Symptoms, and Cognitive Function in Patients with Alzheimer’s Disease: A Chinese Consensus on the Comprehensive Management of Alzheimer’s Disease. Neurosci Bull 2021;37:1025-38. [Crossref] [PubMed]
- Jia J, Wei C, Chen S, et al. The cost of Alzheimer’s disease in China and re-estimation of costs worldwide. Alzheimers Dement 2018;14:483-91. [Crossref] [PubMed]
- Corsi M, Di Raimo T, Di Lorenzo C, et al. Cognitive disability in alzheimer’s disease and its management. Clin Ter 2016;167:e123-6. [PubMed]
- Reitz C, Mayeux R. Alzheimer disease: epidemiology, diagnostic criteria, risk factors and biomarkers. Biochem Pharmacol 2014;88:640-51. [Crossref] [PubMed]
- Revett TJ, Baker GB, Jhamandas J, et al. Glutamate system, amyloid ß peptides and tau protein: functional interrelationships and relevance to Alzheimer disease pathology. J Psychiatry Neurosci 2013;38:6-23. [Crossref] [PubMed]
- Loera-Valencia R, Cedazo-Minguez A, Kenigsberg PA, et al. Current and emerging avenues for Alzheimer’s disease drug targets. J Intern Med 2019;286:398-437. [Crossref] [PubMed]
- Losada A, Márquez-González M, Romero-Moreno R, et al. Cognitive-behavioral therapy (CBT) versus acceptance and commitment therapy (ACT) for dementia family caregivers with significant depressive symptoms: Results of a randomized clinical trial. J Consult Clin Psychol 2015;83:760-72. [Crossref] [PubMed]
- Clarke K, Mayo-Wilson E, Kenny J, et al. Can non-pharmacological interventions prevent relapse in adults who have recovered from depression? A systematic review and meta-analysis of andomized controlled trials. Clin Psychol Rev 2015;39:58-70. [Crossref] [PubMed]
- Chen HM, Huang MF, Yeh YC, et al. Effectiveness of coping strategies intervention on caregiver burden among caregivers of elderly patients with dementia. Psychogeriatrics 2015;15:20-5. [Crossref] [PubMed]
- Ali S, Bokharey IZ. Efficacy of cognitive behavior therapy among caregivers of dementia: An outcome study. Pakistan Journal of Psychological Research 2015;30:249-69.
- Yu JT, Xu W, Tan CC, et al. Evidence-based prevention of Alzheimer’s disease: systematic review and meta-analysis of 243 observational prospective studies and 153 randomised controlled trials. J Neurol Neurosurg Psychiatry 2020;91:1201-9. [Crossref] [PubMed]
- Xu Q, Zhang Y, Zhang X, et al. Medium-chain triglycerides improved cognition and lipid metabolomics in mild to moderate Alzheimer’s disease patients with APOE4-/-: A double-blind, randomized, placebo-controlled crossover trial. Clin Nutr 2020;39:2092-105. [Crossref] [PubMed]
- Imaoka M, Nakao H, Nakamura M, et al. Effect of Multicomponent Exercise and Nutrition Support on the Cognitive Function of Older Adults: A Randomized Controlled Trial. Clin Interv Aging 2019;14:2145-53. [Crossref] [PubMed]
- Tian DY, Wang J, Sun BL, et al. Spicy food consumption is associated with cognition and cerebrospinal fluid biomarkers of Alzheimer disease. Chin Med J (Engl) 2020;134:173-7. [Crossref] [PubMed]
- Wang J, Sun BL, Xiang Y, et al. Capsaicin consumption reduces brain amyloid-beta generation and attenuates Alzheimer’s disease-type pathology and cognitive deficits in APP/PS1 mice. Transl Psychiatry 2020;10:230. [Crossref] [PubMed]
- Hill E, Goodwill AM, Gorelik A, et al. Diet and biomarkers of Alzheimer’s disease: a systematic review and meta-analysis. Neurobiol Aging 2019;76:45-52. [Crossref] [PubMed]
- Gauthier S, Feldman HH, Schneider LS, et al. Efficacy and safety of tau-aggregation inhibitor therapy in patients with mild or moderate Alzheimer’s disease: a andomized, controlled, double-blind, parallel-arm, phase 3 trial. Lancet 2016;388:2873-84. [Crossref] [PubMed]
- Ngandu T, Lehtisalo J, Solomon A, et al. A 2 year multidomain intervention of diet, exercise, cognitive training, and vascular risk monitoring versus control to prevent cognitive decline in at-risk elderly people (FINGER): a andomized controlled trial. Lancet 2015;385:2255-63. [Crossref] [PubMed]
- Phillips MCL, Deprez LM, Mortimer GMN, et al. Randomized crossover trial of a modified ketogenic diet in Alzheimer’s disease. Alzheimers Res Ther 2021;13:51. [Crossref] [PubMed]
- Liu X, Morris MC, Dhana K, et al. Mediterranean-DASH Intervention for Neurodegenerative Delay (MIND) study: Rationale, design and baseline characteristics of a randomized control trial of the MIND diet on cognitive decline. Contemp Clin Trials 2021;102:106270. [Crossref] [PubMed]
- Fortier M, Castellano CA, St-Pierre V, et al. A ketogenic drink improves cognition in mild cognitive impairment: Results of a 6-month RCT. Alzheimers Dement 2021;17:543-52. [Crossref] [PubMed]
- Coelho-Júnior HJ, Trichopoulou A, Panza F. Cross-sectional and longitudinal associations between adherence to Mediterranean diet with physical performance and cognitive function in older adults: A systematic review and meta-analysis. Ageing Res Rev 2021;70:101395. [Crossref] [PubMed]
- Neth BJ, Mintz A, Whitlow C, et al. Modified ketogenic diet is associated with improved cerebrospinal fluid biomarker profile, cerebral perfusion, and cerebral ketone body uptake in older adults at risk for Alzheimer’s disease: a pilot study. Neurobiol Aging 2020;86:54-63. [Crossref] [PubMed]
- Norwitz NG, Saif N, Ariza IE, et al. Precision Nutrition for Alzheimer’s Prevention in ApoE4 Carriers. Nutrients 2021;13:1362. [Crossref] [PubMed]
- Li D, Ren J, Wang T, et al. Anti-hypoxia effects of walnut oligopeptides (Juglans regia L.) in mice. Am J Transl Res 2021;13:4581-90. [PubMed]
- Liu L, Guo Q, Cui M, et al. Impact of maternal nutrition during early pregnancy and diet during lactation on lactoferrin in mature breast milk. Nutrition 2022;93:111500. [Crossref] [PubMed]
- Wang B, Cheng ZJ, Xu Q, et al. Dietary Structure and Nutritional Status of Chinese Beekeepers: Demographic Health Survey. JMIR Public Health Surveill 2021;7:e28726. [Crossref] [PubMed]
- Feng L, Romero-Garcia R, Suckling J, et al. Effects of choral singing versus health education on cognitive decline and aging: a randomized controlled trial. Aging (Albany NY) 2020;12:24798-816. [Crossref] [PubMed]
- Ma YH, Wu JH, Xu W, et al. Associations of Green Tea Consumption and Cerebrospinal Fluid Biomarkers of Alzheimer’s Disease Pathology in Cognitively Intact Older Adults: The CABLE Study. J Alzheimers Dis 2020;77:411-21. [Crossref] [PubMed]
- Mo M, Wang S, Chen Z, et al. A systematic review and meta-analysis of the response of serum 25-hydroxyvitamin D concentration to vitamin D supplementation from RCTs from around the globe. Eur J Clin Nutr 2019;73:816-34. [Crossref] [PubMed]
- Wan Z, Guo J, Pan A, et al. Association of Serum 25-Hydroxyvitamin D Concentrations With All-Cause and Cause-Specific Mortality Among Individuals With Diabetes. Diabetes Care 2021;44:350-7. [Crossref] [PubMed]
- Katsyuba E, Mottis A, Zietak M, et al. De novo NAD+ synthesis enhances mitochondrial function and improves health. Nature 2018;563:354-9. [Crossref] [PubMed]
- Marletta AS, Massarotti A, Orsomando G, et al. Crystal structure of human nicotinic acid phosphoribosyltransferase. FEBS Open Bio 2015;5:419-28. [Crossref] [PubMed]
- Brazill JM, Li C, Zhu Y, et al. NMNAT: It’s an NAD+ synthase… It’s a chaperone… It’s a neuroprotector. Curr Opin Genet Dev 2017;44:156-62. [Crossref] [PubMed]
- Rizzi M, Bolognesi M, Coda A. A novel deamido-NAD+-binding site revealed by the trapped NAD-adenylate intermediate in the NAD+ synthetase structure. Structure 1998;6:1129-40. [Crossref] [PubMed]
- Braidy N, Berg J, Clement J, et al. Role of Nicotinamide Adenine Dinucleotide and Related Precursors as Therapeutic Targets for Age-Related Degenerative Diseases: Rationale, Biochemistry, Pharmacokinetics, and Outcomes. Antioxid Redox Signal 2019;30:251-94. [Crossref] [PubMed]
- Rajman L, Chwalek K, Sinclair DA. Therapeutic Potential of NAD-Boosting Molecules: The In Vivo Evidence. Cell Metab 2018;27:529-47. [Crossref] [PubMed]
- Hou Y, Wei Y, Lautrup S, et al. NAD+ supplementation reduces neuroinflammation and cell senescence in a transgenic mouse model of Alzheimer’s disease via cGAS-STING. Proc Natl Acad Sci U S A 2021;118:e2011226118. [Crossref] [PubMed]
- Li G, Xu A, Sim S, et al. Transcriptomic Profiling Maps Anatomically Patterned Subpopulations among Single Embryonic Cardiac Cells. Dev Cell 2016;39:491-507. [Crossref] [PubMed]
- Zhu Y, Ding X, She Z, et al. Exploring Shared Pathogenesis of Alzheimer’s Disease and Type 2 Diabetes Mellitus via Co-expression Networks Analysis. Curr Alzheimer Res 2020;17:566-75. [Crossref] [PubMed]
- Yip AM, Horvath S. Gene network interconnectedness and the generalized topological overlap measure. BMC Bioinformatics 2007;8:22. [Crossref] [PubMed]
- Thalamuthu A, Mukhopadhyay I, Zheng X, et al. Evaluation and comparison of gene clustering methods in microarray analysis. Bioinformatics 2006;22:2405-12. [Crossref] [PubMed]
- Carlson MR, Zhang B, Fang Z, et al. Gene connectivity, function, and sequence conservation: predictions from modular yeast co-expression networks. BMC Genomics 2006;7:40. [Crossref] [PubMed]
- van der Laan MJ, Pollard KS. A new algorithm for hybrid hierarchical clustering with visualization and the bootstrap. Journal of Statistical Planning and Inference 2003;117:275-303. [Crossref]
- Dembélé D, Kastner P. Fuzzy C-means method for clustering microarray data. Bioinformatics 2003;19:973-80. [Crossref] [PubMed]
- Liang JW, Fang ZY, Huang Y, et al. Application of Weighted Gene Co-Expression Network Analysis to Explore the Key Genes in Alzheimer’s Disease. J Alzheimers Dis 2018;65:1353-64. [Crossref] [PubMed]
- Dinel AL, Lucas C, Guillemet D, et al. Chronic Supplementation with a Mix of Salvia officinalis and Salvia lavandulaefolia Improves Morris Water Maze Learning in Normal Adult C57Bl/6J Mice. Nutrients 2020;12:1777. [Crossref] [PubMed]
- Lescroart F, Chabab S, Lin X, et al. Early lineage restriction in temporally distinct populations of Mesp1 progenitors during mammalian heart development. Nat Cell Biol 2014;16:829-40. [Crossref] [PubMed]
- Fiset A, Xu E, Bergeron S, et al. Compartmentalized CDK2 is connected with SHP-1 and β-catenin and regulates insulin internalization. Cell Signal 2011;23:911-9. [Crossref] [PubMed]
- Norwitz NG, Mota AS, Norwitz SG, et al. Multi-Loop Model of Alzheimer Disease: An Integrated Perspective on the Wnt/GSK3β, α-Synuclein, and Type 3 Diabetes Hypotheses. Front Aging Neurosci 2019;11:184. [Crossref] [PubMed]
- Nusse R, Clevers H. Wnt/β-Catenin Signaling, Disease, and Emerging Therapeutic Modalities. Cell 2017;169:985-99. [Crossref] [PubMed]
- Chen KL, Li PX, Sun YM, et al. Very Early-Onset Alzheimer’s Disease in the Third Decade of Life with de novo PSEN1 Mutations. J Alzheimers Dis 2022;85:65-71. [Crossref] [PubMed]
- Lessel D, Wu D, Trujillo C, et al. Dysfunction of the MDM2/p53 axis is linked to premature aging. J Clin Invest 2017;127:3598-608. [Crossref] [PubMed]
- Elkholi R, Abraham-Enachescu I, Trotta AP, et al. MDM2 Integrates Cellular Respiration and Apoptotic Signaling through NDUFS1 and the Mitochondrial Network. Mol Cell 2019;74:452-65.e7. [Crossref] [PubMed]
- Das C, Roy S, Namjoshi S, et al. Binding of the histone chaperone ASF1 to the CBP bromodomain promotes histone acetylation. Proc Natl Acad Sci U S A 2014;111:E1072-81. [Crossref] [PubMed]
- Iyer-Bierhoff A, Krogh N, Tessarz P, et al. SIRT7-Dependent Deacetylation of Fibrillarin Controls Histone H2A Methylation and rRNA Synthesis during the Cell Cycle. Cell Rep 2018;25:2946-54.e5. [Crossref] [PubMed]
- Valis K, Prochazka L, Boura E, et al. Hippo/Mst1 stimulates transcription of the proapoptotic mediator NOXA in a FoxO1-dependent manner. Cancer Res 2011;71:946-54. [Crossref] [PubMed]
- Zhao Y, Yang J, Liao W, et al. Cytosolic FoxO1 is essential for the induction of autophagy and tumour suppressor activity. Nat Cell Biol 2010;12:665-75. [Crossref] [PubMed]
- Curtis AM, Seo SB, Westgate EJ, et al. Histone acetyltransferase-dependent chromatin remodeling and the vascular clock. J Biol Chem 2004;279:7091-7. [Crossref] [PubMed]
- Alers S, Löffler AS, Wesselborg S, et al. Role of AMPK-mTOR-Ulk1/2 in the regulation of autophagy: cross talk, shortcuts, and feedbacks. Mol Cell Biol 2012;32:2-11. [Crossref] [PubMed]