Artificial intelligence scale-invariant feature transform algorithm-based system to improve the calculation accuracy of Ki-67 index in invasive breast cancer: a multicenter retrospective study
Introduction
Ki-67 is a nuclear marker of cell proliferation which labels cells in all phases of the cell cycle except for G0 phase. As a key indicator of the proliferation activity of tumors, its expression positively correlates with the proliferation rate of tumor cells (1,2). In breast cancer, Ki-67 is not only a critical index to guide treatment, but is also a significant prognostic indicator. The Ki-67 index refers to the percentage of nuclear-positive tumor cells among all the tumor cells in a microscope view. In practice, Ki-67-positive cells are detected by an immunohistochemistry (IHC) staining method. Currently, there are multiple ways to count Ki-67-positive cells, including manual counting, visual estimation, and computer-aided automatic counting. There is a low Ki-67 rate in normal breast tissue (usually <3%) and Ki-67 expression correlates with the density of breast tissue (3,4). The Ki-67 index increases in about 40% of cases of ductal carcinoma in situ (DCIS). There is an even higher Ki-67 expression level in invasive breast cancer, in which the Ki-67 index is correlated with pathological grade, invasion degree, tumor size, and lymph node metastasis (5). In the 2020 National Comprehensive Cancer Network (NCCN) guidelines, a Ki-67-positive rate of 20–30% is recommended as the cut-off value for high-risk tumors. However, this 20–30% cut-off is still an imprecise value.
Ki-67 is one of the main parameters to distinguish the molecular subtypes of breast cancer. In 2013, the St. Gallen consensus categorized breast cancers into the following subtypes: luminal A subtype [estrogen receptor (ER) positive and progesterone receptor (PR) positive, human epidermal growth factor receptor 2 (HER2) negative and Ki-67 <14%], luminal B/HER2-negative subtype (ER positive, HER2 negative, with one of the following: Ki-67 ≥14%, PR negative, or low PR), luminal B/HER2-positive subtype (ER positive, HER2 positive), HER2 overexpression subtype (ER negative, PR negative, HER2 positive), and triple negative subtype (ER negative, PR negative, and HER2 negative). In addition, the Ki-67 index is also used to predict prognosis and the sensitivity of adjuvant chemotherapy and endocrine therapy, as well as to assess the risk of tumor residual disease and evaluate the dynamic response to neoadjuvant therapy (6-10). However, the reported thresholds of the Ki-67 index for these implications are varied. For example, a Korean study suggests that 10% is the threshold for breast cancer overall survival (OS), metastasis, and recurrence (11). Another study recommends 15% to be the optimal cut-off point for OS and 30% as the threshold for partial remission (12). In addition, the Ki-67 index is a predictor of pathological complete response (pCR) for neoadjuvant chemotherapy (NAC) (13,14). The Ki-67 index also has predictive value in postoperative adjuvant therapy (15-18), targeted therapy, and postoperative radiotherapy (19,20).
The IHC marker Ki-67 coupled with genetic aberrations has shown clinical significance. For example, Ki-67 coupled with the mitotic index (MI) or p53 could predict treatment response and prognosis (21,22). The combination of Ki-67 with other biomarkers might provide more accurate information for the prognosis and individualized treatment of breast cancer. Taken together, the Ki-67 index is an effective prognostic and predictive factor. However, there is no available standardized criterion for sample preparation and Ki-67 evaluation. The harms caused by different cut-off value of Ki-67 included low credibility, inconsistent diagnostic and therapeutic criterion, and poor repeatability. Thus, the standardized assessment of the Ki-67 index is urgently needed in clinical application.
Ki-67 is a pivotal index for oncologists to evaluate tumor cell proliferation, predict prognosis, guide adjuvant treatment choice, and predict response to neoadjuvant treatment. Currently, the manual counting method is time consuming and has poor repeatability. In order to improve efficiency, we developed a computer-aided system for the assessment of the Ki-67 index. The AI-based algorithm can save time, the output is stable and not disturbed by external human factors.
A computer-aided system makes the standardized assessment of the Ki-67 index feasible (23). In this study, we developed a computer-aided diagnosis system based on an automatic pathological diagnosis model of breast cancer. We established a precise Ki-67 calculation system using a pyramid model and the scale-invariant feature transform (SIFT) feature matching method. Combined with the clinical data, this system can help guide clinical treatment. We present the following article in accordance with the TRIPOD reporting checklist (available at https://atm.amegroups.com/article/view/10.21037/atm-22-4254/rc).
Methods
Study design and data collection
This study aimed to incorporate the identification of invasive breast cancer and the registration and counting of Ki-67-positive breast cancer cells using an integrative algorithm by an artificial intelligence (AI)-coupled automatic microscope system (Lensee Intelligent Tech). The study design and dataflow were shown in Figure S1. Pathologically confirmed breast invasive lobular and ductal carcinoma from TCGA and three independent centers (Hunan Cancer Hospital; the First Hospital of Changsha City; the Second Xiangya Hospital of Central South University) were assessed for eligibility. In this study, 1,872 HE-staining WSIs from TCGA database and 771 HE-staining whole slide images (WSIs) from three independent centers were divided into training set and test set for AI-based invasive cancerous region identification. Matched Ki-67-staining WSIs from three-independent centers were registered with HE-staining WSIs and then the proportion of Ki-67 positive cells in cancerous region was calculated.
The study was conducted in accordance with the Declaration of Helsinki (as revised in 2013). The study was approved by the Ethics Committee of Hunan Cancer Hospital (No. KYJJ-2018-047). The other 2 centers (the First Hospital of Changsha City and the Second Xiangya Hospital of Central South University) also were informed and agreed on the study. Informed consent was taken from all the patients.
To construct the algorithm for automatically counting Ki-67-positive cells in the cancerous region, this study also included consecutive matched slides of HE staining and Ki-67 staining of 240 IDC cases and 60 lobular carcinoma cases. Eligibility criteria included the following: (I) histologically diagnosed primary breast IDC or breast lobular carcinomas with WHO ICD-O-3 code of 8500/3 and 8520/3; (II) confirmed medical records of the Ki-67index in the cancerous region determined by at least 3 experienced pathologists; (III) confirmed hormone receptor (ER, PR) and HER2 pathological report; and (IV) available consecutively matched pathological ER/PR/HER2-stained slides. The exclusion criteria included: (I) IDC or invasive lobular carcinoma (ILC) mixed with other pathological types of breast cancer; (II) cancerous tissues from metastatic lesions; (III) slides without qualified WSIs for AI recognition. After quality control, all TCGA HE-stained slides were eligible for study, and 43 of 900 slides from 3 hospitals were removed due to the poor quality of tissue staining or slide scanning. All eligible slides were scanned by a digital slide scanner (Lensee Intelligent Tech) and annotated by experienced pathologists (Figure S2).
HE-staining and Ki-67 IHC
Remove the dried slices form the oven and immediately put the paraffin in dimethylbenzene for 5–10 min. Then, transfer to anhydrous alcohol (100%), 90% alcohol, 80% alcohol, and 70% alcohol for about 2 min each. Wash the alcohol in water for 2–3 min and move into distilled water for 2 min. Skim surface of hematoxylin with wipe to remove oxidized particles. Blot excess water from slide holder. Hematoxylin staining for 1 min, rinsed in the deionized water. Tap water to allow stain to develop for 5 min. Eosine staining for 30 sec, then tap for each alcohol container for 15 times. Leave slides in xylem overnight to get good clearing of alcohol. Covership slides using Permout (xylene based). Dry overnight.
Ki-67 IHC was performed on additional sections of formalin-fixed paraffin embedded (FFPE) resections or biopsy tissue using recombinant rabbit Ki-67 monoclonal antibody (ab16667, Abcam, Cambridge, Massachusetts) following a previously described IHC protocol (24). Briefly, antigen retrieval was performed at 85 ℃ for 30 minutes and at 75 ℃ for 10 minutes in sodium citrate buffer (pH 6.0). The tissue sections were washed, then peroxidase blocking was performed for 30 minutes. Sections were then incubated with anti-Ki-67 antibody (1:200 dilution) for 2 hours at room temperature. The secondary mouse antibody was incubated for 15 minutes at room temperature. Then, the tissues were washed with universal horseradish peroxidase (HRP) reagent and incubated with diaminobenzidine tetrahydrochloride (DAB) for 5 minutes. The automatic IHC staining system (BENCHMARK, Roche, USA) was used in this study.
Cancerous region identification
Datasets
The first step for AI identification was to generate the datasets. All included WSIs were randomly divided into the training set, verification set, and test set according to a fixed ratio of 7:2:1. Subsequently, the training dataset was produced. As shown in Figure S3, each WSI was stored in the format of a pyramid file. When the sample set was specified, the image with lower resolution at the top level was taken for image foreground segmentation. In order to facilitate computer processing, the deep learning network framework adopted in this paper was the ResNet neural network framework, and the input image size was 244×244. Therefore, the resolution difference between the top image with low resolution and the bottom image with high resolution was 256 times. In this way, each pixel of the segmented foreground image directly represented a tile. Then, according to the foreground pixel index, the tissue region was segmented, and according to this, the tiles of the bottom image were obtained by the index, and the tiles were cut into the training dataset. In the same way, the corresponding verification and test training sets could be generated.
Training and testing processes
The training and testing process of the network are shown in Figure S4. Firstly, the training set and verification set were used for network training. The network architecture adopted the ResNet network. The verification process of network performance was accompanied by the training process of the network. Finally, the training accuracy rate obtained in the network training process was 99.5%, and the verification accuracy was 97.5%. After the optimized training network was obtained, network testing was carried out. The testing process of a single WSI is shown in Figure S5. The WSI was inferred to obtain the thermos dynamic diagram, which showed the probability of whether each tile was a tumor. The receiver operating characteristic (ROC) curve was obtained, and the accuracy of the test was 97.7%.
Rapid registration procedure based on the pyramid model
The registration process between HE-stained WSIs and Ki-67-stained WSIs were completed by using the SIFT-based feature registration method (25,26). The registration accuracy of SIFT was high. However, the dis-advantage was that such a process was slow, especially when reference HE-stained WSIs or the registered Ki-67-stained WSIs were large.
In this study, we applied the pyramid model and SIFT fast feature matching method to register the digital microscopic pathology HE WSIs and Ki-67 WSIs. By using the pyramid model, the registration parameters, such as rotation angle, scaling ratio, and displacement, could be extracted quickly. Usually, the registration speed of the SIFT method was very slow, especially for WSIs with high resolution. To speed up the registration process, the pyramid model was adopted and the top images with low resolution were used for registration. Then, the parameters such as rotation angle, scaling ratio, and displacement extracted from the registration were directly used for the large-scale images with high resolution. After the one-to-one pairing of HE-stained WSI blocks and Ki-67-stained WSI blocks, we could map the HE blocks that were identified and classified as cancer tissue regions to the corresponding Ki-67 block, and then quickly found the cancer tissue region in Ki-67 WSIs.
Since both the HE-stained and Ki-67-stained images were stored in pyramid file format, it was unnecessary to use the entire WSIs for analysis. SIFT registration could be performed by using the smaller layers in the middle and high layers of the pyramid to obtain the corresponding registration parameters. Because the size and shape of the consecutively cut tissues were very similar between HE-stained slides and Ki-67-stained slides, we were able to use the featured parameters of rotation angle, scaling ratio, and displacement to realize the registration process between HE-stained images and Ki-67-stained images.
After obtaining the registration parameters, we extracted the featured parameters, including the corresponding rotation angle, scale ratio, and the shifting amount. These featured parameters were then applied to match the images at the bottom of the pyramid. Each tile of the HE-stained image and Ki-67-stained image was matched one by one (Figure 1).
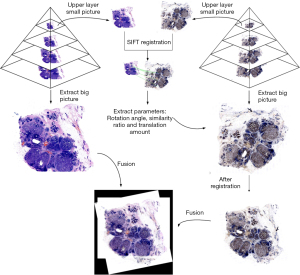
Calculation of the Ki-67 index
The Ki-67 counting method used in this experiment involved the complete counting of the total number of invasive tumor cells, although the hot spot counting method is mostly used in practical application. There are also reports on the count of the Ki-67 index at the tumor invasive fronts. These counting methods require manually counting 500–1,000 tumor cells. To meet this criterion and ensure the accuracy of our algorithm, we firstly counted the invasive tumor cells in WSIs to make sure there were more than 500 invasive cancer cells in our cases.
Then, after the completion of the feature registration process between HE-stained images and Ki-67-stained images, the system automatically lineated the cancerous region for the corresponding region of Ki-67 staining area by the AI algorithm. Then, the statistics of positive cancer cells with Ki-67 nuclear-staining within the cancerous region were required. In the cancerous region, the corresponding nuclear region statistics were determined for each tile recognized using the deep residual learning method (27,28). As shown in Figures S1,S6, the process of standing separation and color normalization of IDC tissue images was completed using the color deconvolution method (29). The nucleus was segmented by the watershed method, and the nucleus was separated by image processing, so as to obtain the statistics of cell number.
In this study, the pathological expert’s manual count (hot spot counting) was used as the gold standard. Two experienced pathologists were involved in the evaluation process. There was a third to arbitrate when the 2 pathologists’ assessments were inconsistent. To evaluate the overall concordance rate of the AI method and manual counting, the AI-based Ki-67 calculation was compared with the pathologists’ reports. Generally, a score difference of ±10% is acceptable for clinical significance, but for a lower Ki-67 value within 20%, the difference should be within 5% when it is closely related to clinical significance (such as grading and medication).
Inflammatory cells in the invasive tumor area would not affect the AI-based Ki-67 counting since cancer cells could be distinguished from inflammatory cells based on the architecture and cell morphology.
Statistical analysis
To ensure the diagnostic accuracy, four pathologists browsed the images in isolated places, labeled cancer cells on the images, calculated the positive rate of Ki-67. Researchers collected the Ki-67 positive rate values of all sections, compared the positive rates of the diagnoses of pathologist 1, pathologist 2 and pathologist 3 on the same section, and obtained the final manual diagnosis results of the pathologist as follows: (I) the difference of the three positive rate values is not more than 10%, and the average of the three positive rate values is taken as the final manual diagnosis result of the pathologist; (II) if the difference of the three positive rate values is greater than 10%, the section shall be submitted to the pathologist for further diagnosis, and then the positive rate value diagnosed by the expert shall be compared with the original three positive rate values again. At this time, if the difference of the three positive rate values is not greater than 10%, the average of the three positive rate values shall be taken as the final pathologist’s manual diagnosis result, otherwise the section shall be removed. The researchers compared the manual diagnosis results of pathologists and the AI-assisted diagnosis results of each slice, and analyzed and counted the research results.
All statistical analyses were conducted by using SAS 9.4 software (SAS Institute Inc., NC, USA) and R 4.1.1 (https://www.r-project.org/). All hypothesis tests were two sided and conducted at a significance level of 0.05.
Patient and public involvement statement
The data set included 1,872 HE-stained tissue slides of 509 invasive ductal carcinoma (IDC, WHO ICD-O-3: 8500/3) cases and 115 lobular carcinoma (WHO ICD-O-3: 8520/3) cases from TCGA-BRCA (The Cancer Genome Atlas-breast cancer) project, along with 900 HE-stained tissue slides (including both the slides of the needle biopsy specimen and the slides of the surgically removed tumor tissues) of 240 IDC cases and 60 lobular carcinoma cases from Hunan Cancer Hospital, the First Hospital of Changsha City and the Second Xiangya Hospital of Central South University.
Results
The accuracy of the AI-based algorithm in the identification of invasive breast cancer tissues in HE-stained slides
In this study, 1,872 HE-stained WSIs from TCGA database and 771 HE-stained WSIs from 3 independent hospitals were collected. Invasive carcinoma regions were annotated on each WSI by experienced pathologists (Figure S2). A ResNet network was used for data training and verification. In order to adjust the training network, the training process was always accompanied by verification. The training accuracy rate was 99.5% and the verification accuracy was 97.5%. Eventually, we obtained an optimized model and carried out network testing. As shown in Figure 2, the test area under the curve (AUC) was 97.7%. The sensitivity and specificity were 0.9168 and 0.9352, respectively.
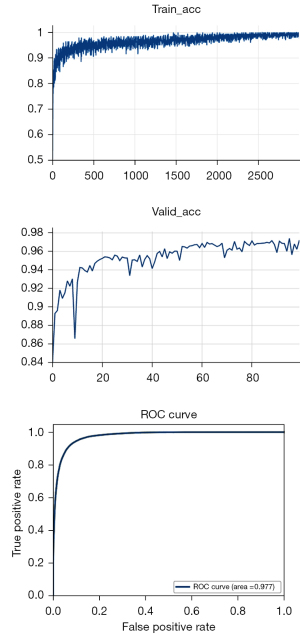
Registration results of Ki-67 staining and corresponding IDC region
A total of 771 pairs of HE and Ki-67 slides from 257 cases were qualified for HE and Ki-67 registration and AI-based identification. The registration process between images of HE-stained slides and Ki-67-stained slides was based on the feature matching method of SIFT. Since both HE images and Ki-67 images were stored in pyramid file format, we firstly used the smaller layers in the middle and high levels of the pyramid for SIFT registration to obtain the corresponding registration parameters. As the size and shape of contiguous HE and Ki-67 slides were very similar, the registration process could be realized by extracting parameters such as rotation angle, scaling ratio, and displacement. Then, the registration parameters were applied to the image at the bottom of the pyramid to complete the one-to-one matching process of each tile of HE-stained WSIs and Ki-67-stained WSIs. Figure 3A-3D shows the registration output of 4 pairs of HE and Ki-67 WSIs.
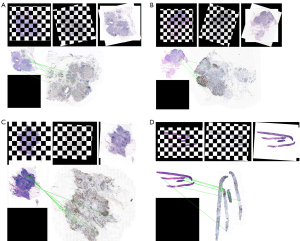
The agreement of the AI-based algorithm with the gold standard of Ki-67 index calculation
After matching the image features of HE- and Ki-67-stained WSIs, we obtained the image regions of Ki-67 corresponding to the HE-stained cancerous region identified by AI. In the cancerous region, we counted the Ki-67 index in the regions corresponding to each tile identified by AI. Compared to the gold standard percentage obtained from the pathological report, we found that AI-based calculation results highly agreed with the gold standard reports, with an average Ki-67-positive rate of 42.24%±0.14% vs. 44.50%±0.18%. The AI-based automatic labeling example is exhibited in Figure 4. The accuracy of AI-based calculation of the Ki-67 index was 91.5%.
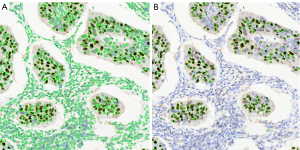
Time consumption of AI-based registration and calculation
In our AI-based system, the time consumption of the whole process of the identification of the cancerous region on HE-stained WSIs and the registration of HE-stained WSIs and Ki-67-stained WSIs was less than 1 second for a pair of HE-stained and Ki-67-stained slides. For all 771 pairs of slides, the total time was less than 1 hour. Figure S7 shows a WSI and 3 high-definition images of Ki-67 count results to demonstrate that positive nuclei were accurately counted. Compared to the gold standard, the accuracy rate of AI-based calculation for the Ki-67 index was 91.5%.
Discussion
The clinical examination of Ki-67 mainly depends on the results of IHC. However, at present, pathology departments generally rely on pathologists reading slides under a microscope to obtain the results. Therefore, the accuracy of the results depends on the pathologist’s experience and is limited by the accuracy limitations of human vision.
In addition, there are objective differences in Ki-67 values between different laboratories (30). Therefore, some clinical studies involving Ki-67 may draw different or even completely opposite conclusions. For example, the PACS01 and BCIRG001 studies suggest that Ki-67 has predictive value for chemotherapy efficacy (31,32), while the IBCSGVIII and IX studies do not (33). This requires us to fully consider the factors that affect the Ki-67 value when interpreting these research results or designing clinical studies, including positive judgment, statistical correction, calibration of test results, and the application of digital image analysis systems, among others.
The application of AI-based automatic calculation of the Ki-67 index is a practical method to solve the issue of inconsistency. Several studies have developed AI-based automated quantitative tools for Ki-67 calculation (34-36). Feng et al. developed a platform to obtain an automated Ki-67 score (34). However, their system is time consuming, with 10 slides processed in 23 minutes and 19 seconds. In this study, we first used the pyramid model for tumor region recognition and registration, which saves a lot of time on the premise of ensuring accuracy. Our platform http://newfar.lenseebio.cn is accessible with permission.
There is a gradual increase in the Ki-67 index between normal breast, DCIS, and invasive breast cancer tissue. In addition, the prognostic relevance of the Ki-67 index differs according to breast cancer subtypes. The STEPP analysis showed that low Ki-67 had a different predictive value between ILC and IDC (37). However, in this study, we focused on invasive breast cancer without differentiating between ILC and IDC. In fact, the AI-based accurate and consistent Ki-67 counting system could ensure a more reliable and repeatable conclusion without bias.
In our study, although the overall concordance rate of the AI method and manual counting was more than 90%, there were minimal differences between the AI-based calculation and counting by pathologists. The main reason for the inconsistency was the accuracy of registration. Because a small portion of HE-stained sections and Ki-67 IHC sections were not completely consistent in all aspects of deformation, some of them could not be accurately registered. In the future updated algorithm, we would calculate the registration accuracy and screen out inconsistent registrations for pathologist evaluation. In addition, pathologists count Ki-67-positive cells in hot spots in clinical practice. However, the AI-based Ki-67 counting method is not only in hot spots, but in the whole cancerous area. This distinction might lead to variation in clinical practice. Our team has begun to generate an AI-based hot spot counting method which will continue to be developed in the near future.
Here, accuracy and time efficiency are the two main advantages of our AI SIFT algorithm-based Ki-67 calculation system. The ultimate goal of our AI-based algorithm is to construct an AI-based Ki-67 calculation system for breast cancer diagnosis and treatment. Thus, in future research, we will analyze its correlation with tumor-related factors such as tumor stage and prognosis [disease-free survival (DFS), OS]. Finally, we hope that AI can perform more accurate evaluations than humans.
Acknowledgments
We acknowledge Yunzhijian Tech. for contributing to the computational analyses.
Funding: This study was supported by Changsha Science and Technology Project (No. kq2004137); Hunan Nature and Science Foundation (Nos. 2020JJ8064, 2019JJ80111, 2019JJ50360); Hunan Health Commission Program (Nos. B2019089, C2019070); National Science Foundation of China (No. 61972147); and All-In-One Smart Pathology Solution, Thinking Group Business Plan (No. 2021ZHCXYD060004).
Footnote
Reporting Checklist: The authors have completed the TRIPOD reporting checklist. Available at https://atm.amegroups.com/article/view/10.21037/atm-22-4254/rc
Data Sharing Statement: Available at https://atm.amegroups.com/article/view/10.21037/atm-22-4254/dss
Conflicts of Interest: All authors have completed the ICMJE uniform disclosure form (available at https://atm.amegroups.com/article/view/10.21037/atm-22-4254/coif). Li Yu and Shaobing Huang are from Ningbo Lensee Intelligent Technology Co. Ltd. All authors acknowledge Yunzhijian Tech. for contributing to the computational analyses. The authors have no other conflicts of interest to declare.
Ethical Statement: The authors are accountable for all aspects of the work in ensuring that questions related to the accuracy or integrity of any part of the work are appropriately investigated and resolved. The study was conducted in accordance with the Declaration of Helsinki (as revised in 2013). The study was approved by the Ethics Committee of Hunan Cancer Hospital (No. KYJJ-2018-047). The other 2 centers (the First Hospital of Changsha City and the Second Xiangya Hospital of Central South University) also were informed and agreed on the study. Informed consent was taken from all the patients.
Open Access Statement: This is an Open Access article distributed in accordance with the Creative Commons Attribution-NonCommercial-NoDerivs 4.0 International License (CC BY-NC-ND 4.0), which permits the non-commercial replication and distribution of the article with the strict proviso that no changes or edits are made and the original work is properly cited (including links to both the formal publication through the relevant DOI and the license). See: https://creativecommons.org/licenses/by-nc-nd/4.0/.
References
- Gimotty PA, Van Belle P, Elder DE, et al. Biologic and prognostic significance of dermal Ki67 expression, mitoses, and tumorigenicity in thin invasive cutaneous melanoma. J Clin Oncol 2005;23:8048-56. [Crossref] [PubMed]
- Mate JL, Ariza A, Aracil C, et al. Cyclin D1 overexpression in non-small cell lung carcinoma: correlation with Ki67 labelling index and poor cytoplasmic differentiation. J Pathol 1996;180:395-9. [Crossref] [PubMed]
- Pang JM, Byrne DJ, Takano EA, et al. Breast Tissue Composition and Immunophenotype and Its Relationship with Mammographic Density in Women at High Risk of Breast Cancer. PLoS One 2015;10:e0128861. [Crossref] [PubMed]
- Clarke RB. Steroid receptors and proliferation in the human breast. Steroids 2003;68:789-94. [Crossref] [PubMed]
- Molino A, Micciolo R, Turazza M, et al. Ki-67 immunostaining in 322 primary breast cancers: associations with clinical and pathological variables and prognosis. Int J Cancer 1997;74:433-7. [Crossref] [PubMed]
- van Leeuwen FW, Buckle T, Kersbergen A, et al. Noninvasive functional imaging of P-glycoprotein-mediated doxorubicin resistance in a mouse model of hereditary breast cancer to predict response, and assign P-gp inhibitor sensitivity. Eur J Nucl Med Mol Imaging 2009;36:406-12. [Crossref] [PubMed]
- Xu J, Guo X, Jing M, et al. Prediction of tumor mutation burden in breast cancer based on the expression of ER, PR, HER-2, and Ki-67. Onco Targets Ther 2018;11:2269-75. [Crossref] [PubMed]
- Pan Y, Yuan Y, Liu G, et al. P53 and Ki-67 as prognostic markers in triple-negative breast cancer patients. PLoS One 2017;12:e0172324. [Crossref] [PubMed]
- Hao J, Zhang W, Lyu Y, et al. Combined Use of cyclinD1 and Ki67 for Prognosis of Luminal-Like Breast Cancer Patients. Front Oncol 2021;11:737794. [Crossref] [PubMed]
- Nielsen TO, Leung SCY, Rimm DL, et al. Assessment of Ki67 in Breast Cancer: Updated Recommendations From the International Ki67 in Breast Cancer Working Group. J Natl Cancer Inst 2021;113:808-19. [Crossref] [PubMed]
- Kim J, Han W, Jung SY, et al. The Value of Ki67 in Very Young Women with Hormone Receptor-Positive Breast Cancer: Retrospective Analysis of 9,321 Korean Women. Ann Surg Oncol 2015;22:3481-8. [Crossref] [PubMed]
- Ács B, Zámbó V, Vízkeleti L, et al. Ki-67 as a controversial predictive and prognostic marker in breast cancer patients treated with neoadjuvant chemotherapy. Diagn Pathol 2017;12:20. [Crossref] [PubMed]
- Flores-Díaz D, Arce C, Flores-Luna L, et al. Impact of invasive lobular carcinoma on long-term outcomes in Mexican breast cancer patients. Breast Cancer Res Treat 2019;176:243-9. [Crossref] [PubMed]
- Cabrera-Galeana P, Muñoz-Montaño W, Lara-Medina F, et al. Ki67 Changes Identify Worse Outcomes in Residual Breast Cancer Tumors After Neoadjuvant Chemotherapy. Oncologist 2018;23:670-8. [Crossref] [PubMed]
- Viale G, Giobbie-Hurder A, Regan MM, et al. Prognostic and predictive value of centrally reviewed Ki-67 labeling index in postmenopausal women with endocrine-responsive breast cancer: results from Breast International Group Trial 1-98 comparing adjuvant tamoxifen with letrozole. J Clin Oncol 2008;26:5569-75. [Crossref] [PubMed]
- Criscitiello C, Disalvatore D, De Laurentiis M, et al. High Ki-67 score is indicative of a greater benefit from adjuvant chemotherapy when added to endocrine therapy in luminal B HER2 negative and node-positive breast cancer. Breast 2014;23:69-75. [Crossref] [PubMed]
- Jacquemier J, Boher JM, Roche H, et al. Protein expression, survival and docetaxel benefit in node-positive breast cancer treated with adjuvant chemotherapy in the FNCLCC-PACS 01 randomized trial. Breast Cancer Res 2011;13:R109. [Crossref] [PubMed]
- Joosten SEP, Wellenstein M, Koornstra R, et al. IHC-based Ki67 as response biomarker to tamoxifen in breast cancer window trials enrolling premenopausal women. NPJ Breast Cancer 2021;7:138. [Crossref] [PubMed]
- Zurrida S, Bagnardi V, Curigliano G, et al. High Ki67 predicts unfavourable outcomes in early breast cancer patients with a clinically clear axilla who do not receive axillary dissection or axillary radiotherapy. Eur J Cancer 2013;49:3083-92. [Crossref] [PubMed]
- Selz J, Stevens D, Jouanneau L, et al. Prognostic value of molecular subtypes, ki67 expression and impact of postmastectomy radiation therapy in breast cancer patients with negative lymph nodes after mastectomy. Int J Radiat Oncol Biol Phys 2012;84:1123-32. [Crossref] [PubMed]
- Rossi L, Laas E, Mallon P, et al. Prognostic impact of discrepant Ki67 and mitotic index on hormone receptor-positive, HER2-negative breast carcinoma. Br J Cancer 2015;113:996-1002. [Crossref] [PubMed]
- Jing N, Fang C, Williams DS. Validity and reliability of Ki-67 assessment in oestrogen receptor positive breast cancer. Pathology 2017;49:371-8. [Crossref] [PubMed]
- Xu M, Tang Q, Li M, et al. An analysis of Ki-67 expression in stage 1 invasive ductal breast carcinoma using apparent diffusion coefficient histograms. Quant Imaging Med Surg 2021;11:1518-31. [Crossref] [PubMed]
- Ando K, Tohme YH, Srinivasiah A, et al. Developing a Phosphospecific IHC Assay as a Predictive Biomarker for Topoisomerase I Inhibitors. J Histochem Cytochem 2018;66:549-61. [Crossref] [PubMed]
- Al-Khafaji SL, Zhou Jun, Zia A, et al. Spectral-Spatial Scale Invariant Feature Transform for Hyperspectral Images. IEEE Trans Image Process 2018;27:837-50. [Crossref] [PubMed]
- Chen J, Tian J. Rapid multi-modality preregistration based on SIFT descriptor. Conf Proc IEEE Eng Med Biol Soc 2006;2006:1437-40. [Crossref] [PubMed]
- Gholizadeh-Ansari M, Alirezaie J, Babyn P. Low-dose CT Denoising with Dilated Residual Network. Annu Int Conf IEEE Eng Med Biol Soc 2018;2018:5117-20. [Crossref] [PubMed]
- Yu L, Chen H, Dou Q, et al. Automated Melanoma Recognition in Dermoscopy Images via Very Deep Residual Networks. IEEE Trans Med Imaging 2017;36:994-1004. [Crossref] [PubMed]
- Khan AM, Rajpoot N, Treanor D, et al. A nonlinear mapping approach to stain normalization in digital histopathology images using image-specific color deconvolution. IEEE Trans Biomed Eng 2014;61:1729-38. [Crossref] [PubMed]
- Wang M, McLaren S, Jeyathevan R, et al. Laboratory validation studies in Ki-67 digital image analysis of breast carcinoma: a pathway to routine quality assurance. Pathology 2019;51:246-52. [Crossref] [PubMed]
- Penault-Llorca F, André F, Sagan C, et al. Ki67 expression and docetaxel efficacy in patients with estrogen receptor-positive breast cancer. J Clin Oncol 2009;27:2809-15. [Crossref] [PubMed]
- Andre F, Broglio K, Roche H, et al. Estrogen receptor expression and efficacy of docetaxel-containing adjuvant chemotherapy in patients with node-positive breast cancer: results from a pooled analysis. J Clin Oncol 2008;26:2636-43. [Crossref] [PubMed]
- Liu Y, Yin W, Yan T, et al. The clinical significance of Ki-67 as a marker of prognostic value and chemosensitivity prediction in hormone-receptor-positive breast cancer: a meta-analysis of the published literature. Curr Med Res Opin 2013;29:1453-61. [Crossref] [PubMed]
- Feng M, Deng Y, Yang L, et al. Automated quantitative analysis of Ki-67 staining and HE images recognition and registration based on whole tissue sections in breast carcinoma. Diagn Pathol 2020;15:65. [Crossref] [PubMed]
- Wentzensen N, Lahrmann B, Clarke MA, et al. Accuracy and Efficiency of Deep-Learning-Based Automation of Dual Stain Cytology in Cervical Cancer Screening. J Natl Cancer Inst 2021;113:72-9. [Crossref] [PubMed]
- Finkelman BS, Meindl A, LaBoy C, et al. Correlation of manual semi-quantitative and automated quantitative Ki-67 proliferative index with OncotypeDXTM recurrence score in invasive breast carcinoma. Breast Dis 2022;41:55-65. [Crossref] [PubMed]
- Carbognin L, Sperduti I, Fabi A, et al. Prognostic impact of proliferation for resected early stage 'pure' invasive lobular breast cancer: Cut-off analysis of Ki67 according to histology and clinical validation. Breast 2017;35:21-6. [Crossref] [PubMed]
(English Language Editor: C. Betlazar-Maseh)