Development and validation of prediction model using nursing notes on sentiment scores for prognosis of patients with severe acute kidney injury receiving continuous renal replacement therapy based on computational intelligence algorithms
Introduction
Acute kidney injury (AKI) is a serious complication in critically ill patients, with high rates of prevalence and mortality (1). Nearly 30–60% of critically ill patients in intensive care units (ICUs) are diagnosed with AKI (2). Renal replacement therapy (RRT) provides renal support for critically ill AKI patients, with approximately 2–7% of AKI patients requiring RRT (3). Continuous RRT (CRRT) is a preferred treatment option for haemodynamically unstable AKI patients in the ICU (3). Despite advances in clinical care and CRRT treatment in patients with AKI in the ICU, the prognoses of those patients were still unfavorable (4). As reported, the mortality of AKI patients with CRRT is about 40–70% (5). Thus, identifying severe AKI patients receiving CRRT with a high risk of mortality is required.
A study has reported that the mortality of patients with severe AKI receiving CRRT is influenced by various factors. A recent meta-analysis showed that older age and sepsis were risk factors for mortality in AKI patients undergoing CRRT (6). Some other studies have indicated that cumulative fluid balance, mechanical ventilation duration, fluid overload, and disease severity are risk factors for mortality in AKI patients with CRRT (4,7,8). In addition, several scoring systems, including the sequential organ failure assessment (SOFA) and acute physiology and chronic health evaluation II (APACHE-II), have been proposed for predicting the mortality in AKI patients receiving CRRT (9-11). The AUC was 0.68 (95% CI: 0.64–0.71) for the APACHE II, and 0.69 (95% CI: 0.66–0.73) for the SOFA in predicting the mortality in AKI patients receiving CRRT based on the data from Demirjian et al. (9). The C-index was 0.82 (95% CI: 0.76–0.88) in the prediction constructed by da Hora Passos et al. based on norepinephrine utilization, liver failure, medical condition, lactate and pre-dialysis creatinine (10). However, these prediction models have shown ordinary predictive power for the mortality of AKI patients requiring CRRT. Thus, establishing a better prediction model for these patients is necessary.
Nursing notes were previously reported to be an important predictor of in-hospital mortality in ICU patients (12). The nursing notes written by clinicians include important information on the health status of patients. Sentiment analysis, which is a technique that processes natural language, helps to identify the attitudes or impressions of clinicians to patients using computational algorithms for extracting subjective information in the written text and classifying subjective properties (13). In previous studies, nursing notes were applied in prediction model to improve the clinical outcome prediction of patients with various diseases (12,14,15). Whether nursing notes could improve the predictive accuracy of model for the mortality of AKI patients requiring CRRT remains unclear.
The present study aimed to identify the predictors, especially sentiment scores, from nursing notes of AKI patients receiving CRRT and establish different prediction models according to these predictors based on the data collected from the Medical Information Mart for Intensive Care III (MIMIC III) database. We compared the predictive value of different models (with or without the sentiment scores) and identified the optimum model for predicting the mortality in AKI patients receiving CRRT. We present the following article in accordance with the TRIPOD reporting checklist (available at https://atm.amegroups.com/article/view/10.21037/atm-22-4403/rc).
Methods
Study design and population
This cohort study collected the data of 682 AKI patients undergoing CRRT from MIMIC III version 1.4 (MIMIC III v1.4). MIMIC-III is a free single-center critical care database established by the Institutional Review Boards of Beth Israel Deaconess Medical Center (BIDMC, Boston, USA) and the Massachusetts Institute of Technology (MIT, Cambridge, USA), and contains the data of 46,520 patients admitted to the ICUs of BIDMC between 2001 and 2012 (16). The information recorded in the database includes the patients’ demographics, vital signs, laboratory tests, fluid balance, and vital status; documents such as the International Classification of Diseases and Ninth Revision (ICD-9) codes; the recorded hourly physiologic data from bedside monitors verified by ICU nurses; and the stored written evaluations of radiologic films by specialists from the corresponding period.
Also, the narrative notes included in the MIMIC III dataset under the label NOTEEVENTS were extracted and the notes from 12 h before the patient’s death were excluded. Among 682 AKI patients undergoing CRRT, 412 patients lacked nursing notes data and were excluded. AKI was diagnosed according to Kidney Disease: Improving Global Outcomes (KDIGO) criteria (17). Finally, a total of 270 patients were included and randomly divided into a training set (n=189) and a testing set (n=81) at a ratio of 7:3 (Figure 1). The study was conducted in accordance with the Declaration of Helsinki (as revised in 2013).
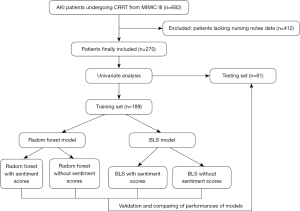
Text mining process
Sentiment scores, including the polarity and subjectivity scores, were defined according to the nursing notes in ICU by the Python programming language (Version 3.7; Python Software Foundation, Delaware, USA) and TextBlob natural language processing library (Version 0.15.3; Python Software Foundation). The polarity score ranged from −1 to 1, and higher scores were indicative of more positive emotions (and vice versa). The subjectivity score ranged from 0 to 1, and higher scores denoted more subjective emotions. Two techniques were applied to combine the nurses’ and chiefs’ notes into sentiment scores: (I) directly extracting sentiment based on the meaning of the text achieved by a classifier trained on a set of labeled and annotated text (chief polarity, chief subjectivity, nurse polarity, nurse subjectivity, polarity, and subjectivity); or (II) using the minimum polarity score value and the maximum subjectivity score value to prevent the influence of irrelevant notes on the average sentiment scores (nurse polarity*, nurse subjectivity*, chief polarity*, chief subjectivity*, polarity*, and subjectivity*). Finally, 12 sentiment scores were obtained.
Outcome variable
The mortality of patients within 5 days was the outcome of our study. The follow-up was ended when the patients died, and all follow-ups were ceased on the fifth day.
Potential predictors
The potential predictors for mortality in AKI patients undergoing CRRT included the following: gender, length of stay (LOS, day), congestive heart failure (yes or no), cardiac arrhythmias (yes or no), valvular disease (yes or no), pulmonary circulation disease (yes or no), peripheral vascular diseases (yes or no), hypertension (yes or no), paralysis (yes or no), other neurological disease (yes or no), chronic pulmonary diseases (yes or no), diabetes (yes or no), hypothyroidism (yes or no), renal failure (yes or no), liver disease (yes or no), peptic ulcer (yes or no), lymphoma (yes or no), metastatic cancer (yes or no), solid tumor (yes or no), rheumatoid arthritis (yes or no), coagulopathy (yes or no), obesity (yes or no), weight loss (yes or no), fluid electrolyte (yes or no), blood loss anemia (yes or no), deficiency anemias (yes or no), alcohol abuse (yes or no), drug abuse (yes or no), mean arterial pressure (MAP, mmHg), respiration rate (time), model for end stage liver disease (MELD), Glasgow coma scale (GCS), SOFA, simplified acute physiology score II (SAPS-II), age (years), chief polarity, chief subjectivity, nurse polarity, nurse subjectivity, polarity, subjectivity, nurse polarity*, nurse subjectivity*, chief polarity*, chief subjectivity*, polarity*, subjectivity*.
Construction of random forest model or BLS model
In total, the data of 270 patients were analyzed there was 131 patients survived and 139 patients died at the end of the follow-up. Then the potential predictors were screened using the data of these patients through comparing the characteristics of patients in the survival group or death group. Characteristics with statistical difference were included as potential predictors. Furthermore, all patients were divided into the training set and the testing set randomly. Among them, 70% of the samples were included in the training set (n=189) to ensure a sufficient number of samples for the construction of more reliable models; 30% of the samples were used as the testing set (n=81) to test the diagnostic efficiency of the models (18,19). The equilibrium test was conducted between the training and testing sets, and the prediction models were established in the training set and validated in the testing set. Variables with statistical differences in the univariate analysis were included as independent variables, and random forest models and broad learning system (BLS) models (with and without sentiment scores) were respectively established.
Statistical analysis
Normality analysis was conducted; normally distributed measurement data were described as the mean ± SD, and the independent t-test was employed for the comparison between groups. Non-normal data were displayed as the median (interquartile range) [M (Q1, Q3)], and comparisons between groups were performed using the Mann-Whitney U rank-sum test. Enumeration data were displayed as n (%), and comparisons between groups were analyzed by the chi-square test (χ2) or Fisher’s exact probability method. Univariate analysis was conducted to screen out the possible predictors of mortality in AKI patients receiving CRRT.
The random forest model was parameterized using the GridSearchCV grid method (Python Software Foundation), and the optimal model parameters were identified by six-fold cross-validation. The goodness of fit was evaluated in the training and testing sets and the combined prediction probability was calculated. The cutoff value was determined by the Youden index and the performances of the models were assessed by the sensitivity, specificity, positive predictive value (PPV), negative prediction value (NPV), area under the curve (AUC), and accuracy. The receiver operator characteristic (ROC) and Kolmogorov-Smirnov (KS) curves were applied to evaluate the predictive value of the models. The threshold of AUC >0.8 was regarded as good predictive ability. The Delong test was applied to compare the AUCs of the four models. P<0.05 was two-sided and considered statistically significant.
Results
The baseline characteristics of participants
In total, 270 AKI patients receiving CRRT were included. As shown in Figure 2, the median survival time of all patients was 102 hours (about 5 days), and thus, 5 days was selected as the in-hospital mortality cutoff time. Among all subjects, 131 patients were in the survival group and 139 were in the death group. Also, 162 (60.00%) patients were males and 108 (40.00%) subjects were females, and the median age of participants was 63.40 years old. The median LOS of all patients was 8.79 days. The median SOFA and SAPS-II scores of all patients were 12 and 50, respectively. The median chief polarity of all people was 0.04 and the chief subjectivity was 0.36. The median nurse polarity was −0.01 and the nurse subjectivity was 0.43. The total polarity was 0.01 and the total subjectivity was 0.41 in all patients. Furthermore, the minimum chief polarity was −0.65 and the maximum chief subjectivity was 0.99. The minimum nurse polarity was 0.65 and the maximum nurse subjectivity was 0.98. The minimum total polarity was 0.72 and the maximum total subjectivity was 1.00 (Table 1).
Table 1
Variable | Total (n=270) | Survival (n=131) | Death (n=139) | Statistical significance | P |
---|---|---|---|---|---|
Gender, n (%) | χ2=1.307 | 0.253 | |||
Male | 162 (60.00) | 74 (56.49) | 88 (63.31) | ||
Female | 108 (40.00) | 57 (43.51) | 51 (36.69) | ||
LOS, M (Q1, Q3) | 8.79 (3.95, 17.12) | 7.47 (3.59, 16.93) | 9.79 (4.50, 18.08) | Z=−1.358 | 0.174 |
Congestive heart failure, n (%) | χ2=2.268 | 0.132 | |||
No | 146 (54.07) | 77 (58.78) | 69 (49.64) | ||
Yes | 124 (45.93) | 54 (41.22) | 70 (50.36) | ||
Cardiac arrhythmias, n (%) | χ2=7.385 | 0.007 | |||
No | 144 (53.33) | 81 (61.83) | 63 (45.32) | ||
Yes | 126 (46.67) | 50 (38.17) | 76 (54.68) | ||
Valvular disease, n (%) | χ2=1.569 | 0.210 | |||
No | 225 (83.33) | 113 (86.26) | 112 (80.58) | ||
Yes | 45 (16.67) | 18 (13.74) | 27 (19.42) | ||
Pulmonary circulation, n (%) | χ2=1.781 | 0.182 | |||
No | 240 (88.89) | 113 (86.26) | 127 (91.37) | ||
Yes | 30 (11.11) | 18 (13.74) | 12 (8.63) | ||
Peripheral vascular, n (%) | χ2=5.869 | 0.015 | |||
No | 226 (83.70) | 117 (89.31) | 109 (78.42) | ||
Yes | 44 (16.30) | 14 (10.69) | 30 (21.58) | ||
Hypertension, n (%) | χ2=1.253 | 0.263 | |||
No | 90 (33.33) | 48 (36.64) | 42 (30.22) | ||
Yes | 180 (66.67) | 83 (63.36) | 97 (69.78) | ||
Paralysis, n (%) | Fisher | 1.000 | |||
No | 264 (97.78) | 128 (97.71) | 136 (97.84) | ||
Yes | 6 (2.22) | 3 (2.29) | 3 (2.16) | ||
Other neurological, n (%) | χ2=0.949 | 0.330 | |||
No | 216 (80.00) | 108 (82.44) | 108 (77.70) | ||
Yes | 54 (20.00) | 23 (17.56) | 31 (22.30) | ||
Chronic pulmonary, n (%) | χ2=0.083 | 0.773 | |||
No | 200 (74.07) | 96 (73.28) | 104 (74.82) | ||
Yes | 70 (25.93) | 35 (26.72) | 35 (25.18) | ||
Diabetes uncomplicated, n (%) | χ2=6.718 | 0.010 | |||
No | 206 (76.30) | 109 (83.21) | 97 (69.78) | ||
Yes | 64 (23.70) | 22 (16.79) | 42 (30.22) | ||
Diabetes complicated, n (%) | χ2=0.068 | 0.795 | |||
No | 210 (77.78) | 101 (77.10) | 109 (78.42) | ||
Yes | 60 (22.22) | 30 (22.90) | 30 (21.58) | ||
Hypothyroidism, n (%) | χ2=0.963 | 0.326 | |||
No | 229 (84.81) | 114 (87.02) | 115 (82.73) | ||
Yes | 41 (15.19) | 17 (12.98) | 24 (17.27) | ||
Renal failure, n (%) | χ2=0.731 | 0.392 | |||
No | 106 (39.26) | 48 (36.64) | 58 (41.73) | ||
Yes | 164 (60.74) | 83 (63.36) | 81 (58.27) | ||
Liver disease, n (%) | χ2=6.031 | 0.014 | |||
No | 176 (65.19) | 95 (72.52) | 81 (58.27) | ||
Yes | 94 (34.81) | 36 (27.48) | 58 (41.73) | ||
Peptic ulcer, n (%) | Fisher | 0.676 | |||
No | 265 (98.15) | 128 (97.71) | 137 (98.56) | ||
Yes | 5 (1.85) | 3 (2.29) | 2 (1.44) | ||
Lymphoma, n (%) | Fisher | 1.000 | |||
No | 264 (97.78) | 128 (97.71) | 136 (97.84) | ||
Yes | 6 (2.22) | 3 (2.29) | 3 (2.16) | ||
Metastatic cancer, n (%) | Fisher | 0.623 | |||
No | 266 (98.52) | 130 (99.24) | 136 (97.84) | ||
Yes | 4 (1.48) | 1 (0.76) | 3 (2.16) | ||
Solid tumor, n (%) | Fisher | 0.448 | |||
No | 263 (97.41) | 129 (98.47) | 134 (96.40) | ||
Yes | 7 (2.59) | 2 (1.53) | 5 (3.60) | ||
Rheumatoid arthritis, n (%) | χ2=1.049 | 0.306 | |||
No | 259 (95.93) | 124 (94.66) | 135 (97.12) | ||
Yes | 11 (4.07) | 7 (5.34) | 4 (2.88) | ||
Coagulopathy, n (%) | χ2=7.384 | 0.007 | |||
No | 163 (60.37) | 90 (68.70) | 73 (52.52) | ||
Yes | 107 (39.63) | 41 (31.30) | 66 (47.48) | ||
Obesity, n (%) | χ2=0.800 | 0.371 | |||
No | 245 (90.74) | 121 (92.37) | 124 (89.21) | ||
Yes | 25 (9.26) | 10 (7.63) | 15 (10.79) | ||
Weight loss, n (%) | χ2=1.899 | 0.168 | |||
No | 247 (91.48) | 123 (93.89) | 124 (89.21) | ||
Yes | 23 (8.52) | 8 (6.11) | 15 (10.79) | ||
Fluid electrolyte, n (%) | χ2=0.127 | 0.722 | |||
No | 94 (34.81) | 47 (35.88) | 47 (33.81) | ||
Yes | 176 (65.19) | 84 (64.12) | 92 (66.19) | ||
Blood loss anemia, n (%) | Fisher | 0.174 | |||
No | 261 (96.67) | 129 (98.47) | 132 (94.96) | ||
Yes | 9 (3.33) | 2 (1.53) | 7 (5.04) | ||
Deficiency anemias, n (%) | Fisher | 1.000 | |||
No | 261 (96.67) | 127 (96.95) | 134 (96.40) | ||
Yes | 9 (3.33) | 4 (3.05) | 5 (3.60) | ||
Alcohol abuse, n (%) | χ2=0.134 | 0.714 | |||
No | 239 (88.52) | 115 (87.79) | 124 (89.21) | ||
Yes | 31 (11.48) | 16 (12.21) | 15 (10.79) | ||
Drug abuse, n (%) | Fisher | 0.531 | |||
No | 260 (96.30) | 125 (95.42) | 135 (97.12) | ||
Yes | 10 (3.70) | 6 (4.58) | 4 (2.88) | ||
MAP, mmHg, mean ± SD | 75.68±21.63 | 79.78±26.01 | 71.81±15.61 | t=3.03 | 0.003 |
Respiratory, times, mean ± SD | 19.79±5.74 | 18.81±5.02 | 20.72±6.23 | t=−2.78 | 0.006 |
MELD, mean ± SD | 27.80±7.89 | 26.45±6.70 | 29.08±8.70 | t=−2.79 | 0.006 |
GCS, M (Q1, Q3) | 10.00 (5.00, 14.00) | 14.00 (5.00, 15.00) | 10.00 (5.00, 14.00) | Z=1.598 | 0.110 |
SOFA, M (Q1, Q3) | 12.00 (10.00, 15.00) | 12.00 (9.00, 14.00) | 13.00 (10.00, 16.00) | Z=−2.364 | 0.018 |
SAPS-II, M (Q1, Q3) | 50.00 (40.00, 62.00) | 46.00 (36.00, 53.00) | 56.00 (45.00, 66.00) | Z=−5.535 | <0.001 |
Age, M (Q1, Q3) | 63.40 (53.98, 74.31) | 60.12 (50.48, 68.68) | 67.12 (57.34, 77.77) | Z=−4.014 | <0.001 |
Chief polarity, M (Q1, Q3) | 0.04 (0.02, 0.05) | 0.04 (0.02, 0.06) | 0.03 (0.01, 0.05) | Z=0.218 | 0.827 |
Chief subjectivity, mean ± SD | 0.36±0.03 | 0.36±0.04 | 0.36±0.03 | t=0.87 | 0.386 |
Nurse polarity, M (Q1, Q3) | −0.01 (−0.03, 0.01) | −0.01 (−0.03, 0.01) | −0.01 (−0.03, 0.01) | Z=0.317 | 0.752 |
Nurse subjectivity, mean ± SD | 0.43±0.04 | 0.43±0.04 | 0.44±0.04 | t=−0.70 | 0.485 |
Polarity, M (Q1, Q3) | 0.01 (−0.01, 0.02) | 0.01 (−0.01, 0.02) | 0.01 (−0.01, 0.02) | Z=−0.173 | 0.863 |
Subjectivity, mean ± SD | 0.41±0.03 | 0.41±0.03 | 0.41±0.03 | t=−0.06 | 0.956 |
Nurse polarity*, mean ± SD | −0.65±0.19 | −0.62±0.19 | −0.68±0.18 | t=2.42 | 0.016 |
Nurse subjectivity*, mean ± SD | 0.98±0.07 | 0.98±0.06 | 0.98±0.07 | t=−0.05 | 0.958 |
Chief polarity*, mean ± SD | −0.65±0.17 | −0.63±0.17 | −0.68±0.16 | t=2.39 | 0.017 |
Chief subjectivity*, mean ± SD | 0.99±0.06 | 0.98±0.09 | 1.00±0.02 | t=−2.34 | 0.021 |
Polarity*, mean ± SD | −0.72±0.15 | −0.70±0.16 | −0.75±0.14 | t=2.62 | 0.009 |
Subjectivity*, mean ± SD | 1.00±0.02 | 1.00±0.03 | 1.00±0.00 | t=−1.72 | 0.088 |
*, represents the minimum value for polarity score and the maximum value for subjectivity score to prevent the influence of irrelevant notes on the average sentiment scores. LOS, length of stay; M (Q1, Q3), median (interquartile range); MAP, mean arterial pressure; MELD, model for end stage liver disease; GCS, Glasgow coma scale; SOFA, sequential organ failure assessment; SAPS-II, simplified acute physiology score II.
Evaluating the potential predictors for mortality in AKI patients receiving CRRT
As shown in Table 1, the proportions of patients with cardiac arrhythmias (54.68% vs. 38.17%, P=0.007), peripheral vascular diseases (21.58% vs. 10.69%, P=0.015), diabetes (30.22% vs. 16.79%, P=0.010), liver disease (41.73% vs. 27.48%, P=0.014), and coagulopathy (47.48% vs. 31.30%, P=0.007) in death group were statistically higher than those in the survival group. The average MAP in the death group was lower than that in the survival group (71.81 vs. 79.78 mmHg, P=0.003). The respiration rate (20.72 vs. 18.81 times, P=0.006) and MELD (29.08 vs. 26.45, P=0.006) in the death group were higher than those in the survival group.
Moreover, the median SOFA score (13.00 vs. 12.00, P=0.018), SAPS-II score (56.00 vs. 46.00, P<0.001), age (67.12 vs. 60.12 years, P<0.001), and chief subjectivity* (1.00 vs. 0.98, P=0.021) were high in the death group compared with the survival group. Also, the nurse polarity* (−0.68 vs. −0.62, P=0.016), chief polarity* (−0.68 vs. −0.63, P=0.017), and polarity* (−0.75 vs. −0.70, P=0.009) were lower in the death group than those in the survival group.
Equilibrium test of the characteristics between the training and testing sets
All of the patients were divided into training and testing sets at a ratio of 7:3. As shown in Table 1, the equilibrium analysis results depicted that there were no statistically significant differences in variables such as demographic variables, clinical variables, accompanying diseases, laboratory examination indexes, and sentiment scores between the training and testing sets.
Construction of the random forest and BLS models without the sentiment scores
Variables with statistically significant differences between the survival and death groups were included in the random forest and BLS models. Regarding the random forest model, the optimal model was as follows: number of decision trees, 100; and maximum depth, 2. The importance of variables is shown in Figure 3. As displayed in Table 2, the SAPS-II score, age, and SOFA score were the most important variables related to the mortality of AKI patients receiving CRRT. In the training set, the sensitivity was 0.91 (95% CI: 0.86–0.97), the specificity was 0.63 (95% CI: 0.53–0.73), the PPV was 0.74 (95% CI: 0.67–0.82), the NPV was 0.86 (95% CI: 0.77–0.94), the AUC was 0.85 (95% CI: 0.80–0.90), and the accuracy was 0.78 (95% CI: 0.72–0.84). In the testing set, the sensitivity was 0.81 (95% CI: 0.68–0.94), the specificity was 0.45 (95% CI: 0.31–0.60), the PPV was 0.56 (95% CI: 0.42–0.69), the NPV was 0.74 (95% CI: 0.58–0.91), the AUC was 0.72 (95% CI: 0.60–0.83), and the accuracy was 0.62 (95% CI: 0.51–0.72). The ROC curves of the training and testing sets are shown in Figure 4A,4B, respectively. According to the ROC curve of the training set, the cutoff point of the combined prediction probability was 0.463. The Hosmer-Lemeshow test in the training (χ2=9.12, P=0.43) and testing (χ2=8.5, P=0.13) sets indicated that the model fit well.
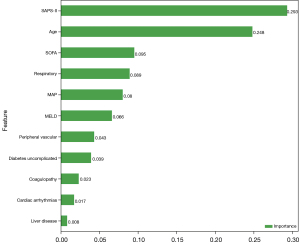
Table 2
Data set | Sensitivity (95% CI) | Specificity (95% CI) | PPV (95% CI) | NPV (95% CI) | AUC (95% CI) | Accuracy (95% CI) |
---|---|---|---|---|---|---|
Random forest without sentiment scores | ||||||
Training set | 0.91 (0.86–0.97) | 0.63 (0.53–0.73) | 0.74 (0.67–0.82) | 0.86 (0.77–0.94) | 0.85 (0.80–0.90) | 0.78 (0.72–0.84) |
Testing set | 0.81 (0.68–0.94) | 0.45 (0.31–0.60) | 0.56 (0.42–0.69) | 0.74 (0.58–0.91) | 0.72 (0.60–0.83) | 0.62 (0.51–0.72) |
BLS without sentiment scores | ||||||
Training set | 0.84 (0.77–0.91) | 0.71 (0.62–0.81) | 0.77 (0.70–0.85) | 0.79 (0.71–0.88) | 0.87 (0.82–0.92) | 0.78 (0.72–0.84) |
Testing set | 0.59 (0.44–0.75) | 0.73 (0.60–0.86) | 0.65 (0.49–0.81) | 0.68 (0.55–0.81) | 0.75 (0.64–0.85) | 0.67 (0.56–0.77) |
Random forest without sentiment scores | ||||||
Training set | 0.72 (0.63–0.80) | 0.87 (0.80–0.94) | 0.87 (0.80–0.94) | 0.72 (0.64–0.81) | 0.86 (0.81–0.91) | 0.79 (0.73–0.85) |
Testing set | 0.65 (0.49–0.80) | 0.75 (0.62–0.88) | 0.69 (0.53–0.84) | 0.72 (0.59–0.85) | 0.78 (0.68–0.88) | 0.70 (0.60–0.80) |
BLS with sentiment scores | ||||||
Training set | 0.95 (0.91–0.99) | 0.48 (0.38–0.59) | 0.68 (0.61–0.76) | 0.89 (0.81–0.98) | 0.87 (0.82–0.92) | 0.74 (0.67–0.80) |
Testing set | 0.41 (0.25–0.56) | 0.98 (0.93–1.00) | 0.94 (0.82–1.00) | 0.66 (0.55–0.78) | 0.82 (0.73–0.91) | 0.72 (0.62–0.81) |
PPV, positive predictive value; NPV, negative prediction value; AUC, area under the curve; BLS, broad learning system.
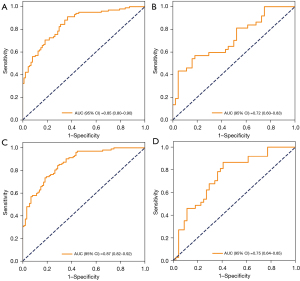
As for the BLS model, the optimal parameters were as follows: nodes belonging to each window =10, feature mapping layer windows =1, shrink coefficient =0.8, enhancement nodes =70, incremental steps =4, and adding enhance nodes =120. In the training set, the sensitivity was 0.84 (95% CI: 0.77–0.91), the specificity was 0.71 (95% CI: 0.62–0.81), the PPV was 0.77 (95% CI: 0.70–0.85), the NPV was 0.79 (95% CI: 0.71–0.88), the AUC was 0.87 (95% CI: 0.82–0.92), and the accuracy was 0.78 (95% CI: 0.72–0.84). In the testing set, the sensitivity was 0.59 (95% CI: 0.44–0.75), the specificity was 0.73 (95% CI: 0.60–0.86), the PPV was 0.65 (95% CI: 0.49–0.81), the NPV was 0.68 (95% CI: 0.55–0.81), the AUC was 0.75 (95% CI: 0.64–0.85), and the accuracy was 0.67 (95% CI: 0.56–0.77) (Table 2). The ROC curves of the training and testing sets are displayed in Figure 4C,4D, respectively. The cut-off value of the model in the training set was 0.50. The Hosmer-Lemeshow test in the training (χ2=4.56, P=0.87) and testing (χ2=7.5, P=0.48) sets suggested that the model fit well.
Construction of the random forest model and BLS model including the sentiment scores
Variables with statistically significant differences between the survival and death groups, as well as chief polarity, chief subjectivity, nurse polarity, nurse subjectivity, polarity, and subjectivity were included in the random forest and BLS models. In the random forest model, the optimal model was as follows: number of decision trees, 18; and maximum depth, 2. Age, SAPS-II score, and SOFA score were the most important variables related to the mortality of AKI patients receiving CRRT (Figure 5). In the training set, the sensitivity was 0.72 (95% CI: 0.63–0.80), the specificity was 0.87 (95% CI: 0.80–0.94), the PPV was 0.87 (95% CI: 0.80–0.94), the NPV was 0.72 (95% CI: 0.64–0.81), the AUC was 0.86 (95% CI: 0.81–0.91), and the accuracy was 0.79 (95% CI: 0.73–0.85). In the testing set, the sensitivity was 0.65 (95% CI: 0.49–0.80), the specificity was 0.75 (95% CI: 0.62–0.88), the PPV was 0.69 (95% CI: 0.53–0.84), the NPV was 0.72 (95% CI: 0.59–0.85), the AUC was 0.78 (95% CI: 0.68–0.88), and the accuracy was 0.70 (95% CI: 0.60–0.80) (Table 2). The ROC and KS curves of the training and testing sets are displayed in Figure 6A,6B, respectively. The cutoff value in the training set was 0.56. The Hosmer-Lemeshow test in the training (χ2=12.74, P=0.17) and testing (χ2=8.18, P=0.15) sets indicated that the model fit well.
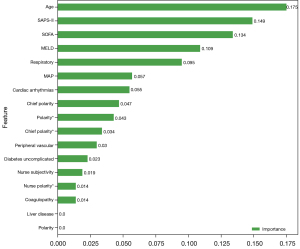
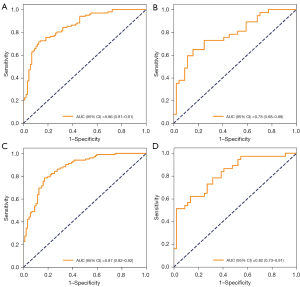
As for the BLS model, the optimal parameters were as follows: nodes belong to each window =10, feature mapping layer windows =1, shrink coefficient =0.8, enhancement nodes =76, incremental steps =4, and adding enhance nodes =120. In the training and testing sets, the sensitivity was 0.95 (95% CI: 0.91–0.99) and 0.41 (95% CI: 0.25–0.56), the specificity was 0.48 (95% CI: 0.38–0.59) and 0.98 (95% CI: 0.93–1.00), the PPV was 0.68 (95% CI: 0.61–0.76) and 0.94 (95% CI: 0.82–1.00), the NPV was 0.89 (95% CI: 0.81–0.98) and 0.66 (95% CI: 0.55–0.78), the AUC was 0.87 (95% CI: 0.82–0.92) and 0.82 (95% CI: 0.73–0.91) (Figure 6C,6D), and the accuracy was 0.74 (95% CI: 0.67–0.80) and 0.72 (95% CI: 0.62–0.81) (Table 2), respectively. The cut-off value of the model in the training set was 0.40. The Hosmer-Lemeshow test in the training (χ2=13.75, P=0.13) and testing (χ2=3.13, P=0.21) sets suggested that the model fit well.
Comparison of the predictive values between the random forest and BLS models
As depicted in Figure 7, the AUC of the random forest model including the sentiment scores was higher than that of the random forest model without the sentiment scores (training set: Z=9.24, P<0.001; testing set: Z=24.94, P<0.001). The AUC of the BLS model with the sentiment scores was higher than that of the BLS model without the sentiment scores (training set: Z=5.54, P<0.001; testing set: Z=31.89, P<0.001). The AUC of the BLS model with the sentiment scores was higher than that of the random forest model with the sentiment scores (training set Z=11.09, P<0.001; testing set: Z=17.86, P<0.001). The AUC of the BLS model without the sentiment scores was higher than that of the random forest model without the sentiment scores (training set Z=20.33, P<0.001; testing set: Z=44.64, P<0.001). The BLS model showed a better predictive value than the random forest model, and the model with the sentiment scores was better than the model without the sentiment scores (P<0.05) (Table 3).
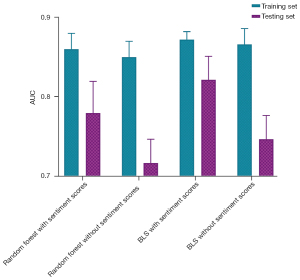
Table 3
Data set | a | b | c |
---|---|---|---|
Training set | Z=5.54, P<0.001 | Z=11.09, P<0.001 | Z=20.33, P<0.001 |
Testing set | Z=31.89, P<0.001 | Z=17.86, P<0.001 | Z=44.64, P<0.001 |
P represents the P value of the Delong tests between the different models. a represents the BLS model without sentiment scores vs. the BLS model with sentiment scores; b represents the Random forest model with sentiment scores vs. the BLS model with sentiment scores; c represents Random forest model without sentiment scores vs. the BLS model with sentiment scores. BLS, broad learning system.
Discussion
The present study evaluated the predictors for mortality in AKI patients receiving CRRT and established four models for predicting mortality based on the data of 270 AKI patients receiving CRRT. The results revealed that age, SOFA score, and SAPS-II score were important factors associated with the mortality of these patients. Additionally, the predictive values of the random forest and BLS models (with or without the sentiment scores) were compared in our study. The data showed that the BLS model with the sentiment scores had the best predictive value for the mortality of AKI patients receiving CRRT.
The sentiments of clinicians or nurses on patients are shown as the relative polarity or positivity of the nursing text, which ranges from −1 (very negative) to 1 (very positive) (20). The clinicians’ and nurses’ sentiments reflect their emotions and attitudes towards patients as well as their intuitions and experiences, for predicting patient outcomes (21). In the clinic, the sentiments of clinicians or nurses have been shown to be associated with the rates of readmission and mortality among patients (22,23). In our study, the sentiments of clinicians or nurses on patients were converted into subjectivity and polarity scores, and higher subjectivity scores and lower polarity scores were observed in the death group compared to the survival people. This was supported by a previous study, which noted that higher subjectivity scores were related to an elevated risk of mortality in ICU patients and higher polarity scores were related to a reduced risk of mortality in ICU patients (24). This reminded us that clinical notes were essential predictors for the mortality of AKI patients undergoing CRRT. In the future, clinical notes should be recorded and studied in greater detail to identify patients with a high risk of mortality and provide timely treatment for these patients. SAPS-II is a composite score that combines the patient’s age, 12 physiological variables, type of admission, and three co-morbidity variables, and is a useful tool for predicting the mortality of ICU patients (25).
Gong et al. demonstrated that SAPS-II was a reliable in-hospital mortality predictor for critically ill patients with AKI (26). In the present study, the SAPS-II score was also identified as a vital predictor of the mortality of AKI patients receiving CRRT. The SOFA score is a scoring system that evaluates organ dysfunction based on six routinely measured variables (respiratory, cardiovascular, hepatic, coagulation, renal, and neurological systems) (27). A previous study has indicated that the SOFA score is a simple and useful tool for predicting the prognosis of critically ill patients (28). Additionally, the SOFA score combined with serum neutrophil gelatinase-associated lipocalin and urinary neutrophil gelatinase-associated lipocalin was reported to help predict in-hospital mortality in septic AKI patients (29). These studies reinforce the findings of our study. Herein, the SOFA score was revealed as an important factor associated with the mortality of AKI patients receiving CRRT.
Furthermore, factors associated with the mortality of AKI patients receiving CRRT were also identified in this study, and four models (including random forest models and BLS models with or without the sentiment scores) were constructed. Validation of the predictive values of the models was performed in the testing set and ROC curves were plotted to determine the performance of each model. The predictive values of the BLS models were superior to those of the random forest models, and the predictive values in models including sentiment scores were better than those without sentiment scores in both the training and testing sets. A presently available prediction model for the 28-day mortality of AKI patients with CRRT treatment has an AUC of 0.77, which was lower than the AUC value of our BLS model (30). Another model for predicting the 7-day mortality of AKI patients receiving CRRT treatment was constructed based on the HEpatic failure, LactatE, NorepInephrine, medical Condition, and Creatinine (HELENICC) score exhibited an AUC value of 0.82 (10), which was also poorer than our model.
In our cohort, we planned to study early mortality (5-day mortality) from the beginning of CRRT, because our patients were very ill, had high SOFA and SAPS-II scores, and the median survival time of all patients was only 102 h (about 5 days). CRRT is a definite method for the treatment and rescue of AKI patients associated with a high risk of mortality, and a precise mortality prediction model is essential for the monitoring and management of patients undergoing CRRT. In this respect, the prediction model in our study might help the clinicians to identify patients with a high risk of death, manage each patient’s hospital course, and provide patients timely interventions to improve their prognosis.
There were several limitations in our study that should be noted. Firstly, the sample size was small, which might decrease the statistical power of our results. Secondly, external validation of the results in our study was not performed. In the future, studies with larger sample sizes from multiple centers and external validations are required to validate the results of this study.
Conclusions
In this study, predictors for the mortality of AKI patients receiving CRRT were identified and four models (including random forest models and BLS models with or without the sentiment scores) were constructed. The BLS models including the sentiment scores showed the best predictive value for the mortality of AKI patients receiving CRRT, which might help to quickly identify patients with a high risk of mortality and offer timely treatments to improve the prognosis of these patients.
Acknowledgments
Funding: This study was supported by Nursing Plateau Discipline Construction Talents Program - Backbone of Scientific Research (hlgy16024kygg).
Footnote
Reporting Checklist: The authors have completed the TRIPOD reporting checklist. Available at https://atm.amegroups.com/article/view/10.21037/atm-22-4403/rc
Conflicts of Interest: All authors have completed the ICMJE uniform disclosure form (available at https://atm.amegroups.com/article/view/10.21037/atm-22-4403/coif). The authors have no conflicts of interest to declare.
Ethical Statement: The authors are accountable for all aspects of the work in ensuring that questions related to the accuracy or integrity of any part of the work are appropriately investigated and resolved. The study was conducted in accordance with the Declaration of Helsinki (as revised in 2013).
Open Access Statement: This is an Open Access article distributed in accordance with the Creative Commons Attribution-NonCommercial-NoDerivs 4.0 International License (CC BY-NC-ND 4.0), which permits the non-commercial replication and distribution of the article with the strict proviso that no changes or edits are made and the original work is properly cited (including links to both the formal publication through the relevant DOI and the license). See: https://creativecommons.org/licenses/by-nc-nd/4.0/.
References
- Pickkers P, Darmon M, Hoste E, et al. Acute kidney injury in the critically ill: an updated review on pathophysiology and management. Intensive Care Med 2021;47:835-50. [Crossref] [PubMed]
- Gameiro J, Carreiro C, Fonseca JA, et al. Acute kidney disease and long-term outcomes in critically ill acute kidney injury patients with sepsis: a cohort analysis. Clin Kidney J 2021;14:1379-87. [Crossref] [PubMed]
- Paškevičius Ž, Skarupskienė I, Balčiuvienė V, et al. Mortality Prediction in Patients with Severe Acute Kidney Injury Requiring Renal Replacement Therapy. Medicina (Kaunas) 2021;57:1076. [Crossref] [PubMed]
- Medina-Liabres KRP, Jeong JC, Oh HJ, et al. Mortality predictors in critically ill patients with acute kidney injury requiring continuous renal replacement therapy. Kidney Res Clin Pract 2021;40:401-10. [Crossref] [PubMed]
- Chen X, Zhou J, Fang M, et al. Incidence- and In-hospital Mortality-Related Risk Factors of Acute Kidney Injury Requiring Continuous Renal Replacement Therapy in Patients Undergoing Surgery for Acute Type a Aortic Dissection. Front Cardiovasc Med 2021;8:749592. [Crossref] [PubMed]
- Hansrivijit P, Yarlagadda K, Puthenpura MM, et al. A meta-analysis of clinical predictors for renal recovery and overall mortality in acute kidney injury requiring continuous renal replacement therapy. J Crit Care 2020;60:13-22. [Crossref] [PubMed]
- Lin J, Zhuang HZ, Zhi Y, et al. Impact of Cumulative Fluid Balance During Continuous Renal Replacement Therapy on Mortality in Patients With Septic Acute Kidney Injury: A Retrospective Cohort Study. Front Med (Lausanne) 2021;8:762112. [Crossref] [PubMed]
- Liu C, Zhang HT, Yue LJ, et al. Risk factors for mortality in patients undergoing continuous renal replacement therapy after cardiac surgery. BMC Cardiovasc Disord 2021;21:509. [Crossref] [PubMed]
- Demirjian S, Chertow GM, Zhang JH, et al. Model to predict mortality in critically ill adults with acute kidney injury. Clin J Am Soc Nephrol 2011;6:2114-20. [Crossref] [PubMed]
- da Hora Passos R, Ramos JG, Mendonça EJ, et al. A clinical score to predict mortality in septic acute kidney injury patients requiring continuous renal replacement therapy: the HELENICC score. BMC Anesthesiol 2017;17:21. [Crossref] [PubMed]
- Wang H, Kang X, Shi Y, et al. SOFA score is superior to APACHE-II score in predicting the prognosis of critically ill patients with acute kidney injury undergoing continuous renal replacement therapy. Ren Fail 2020;42:638-45. [Crossref] [PubMed]
- Gao Q, Wang D, Sun P, et al. Sentiment Analysis Based on the Nursing Notes on In-Hospital 28-Day Mortality of Sepsis Patients Utilizing the MIMIC-III Database. Comput Math Methods Med 2021;2021:3440778. [Crossref] [PubMed]
- Tang JE, Arvind V, White CA, et al. Using Sentiment Analysis to Understand What Patients Are Saying About Hand Surgeons Online. Hand (N Y) 2021; Epub ahead of print. [Crossref] [PubMed]
- Huang K, Gray TF, Romero-Brufau S, et al. Using nursing notes to improve clinical outcome prediction in intensive care patients: A retrospective cohort study. J Am Med Inform Assoc 2021;28:1660-6. [Crossref] [PubMed]
- Kang Y, Topaz M, Dunbar SB, et al. The Utility of Nursing Notes Among Medicare Patients With Heart Failure to Predict 30-Day Rehospitalization: A Pilot Study. J Cardiovasc Nurs 2022;37:E181-6. [Crossref] [PubMed]
- Johnson AE, Pollard TJ, Shen L, et al. MIMIC-III, a freely accessible critical care database. Sci Data 2016;3:160035. [Crossref] [PubMed]
- Kellum JA, Lameire NKDIGO AKI Guideline Work Group. Diagnosis, evaluation, and management of acute kidney injury: a KDIGO summary (Part 1). Crit Care 2013;17:204. [Crossref] [PubMed]
- Chen M, Yang Q, Xu Z, et al. Survival Analysis and Prediction Model for Pulmonary Sarcomatoid Carcinoma Based on SEER Database. Front Oncol 2021;11:630885. [Crossref] [PubMed]
- Tang L, Li W, Zeng X, et al. Value of artificial intelligence model based on unenhanced computed tomography of urinary tract for preoperative prediction of calcium oxalate monohydrate stones in vivo. Ann Transl Med 2021;9:1129. [Crossref] [PubMed]
- de Las Heras-Pedrosa C, Sánchez-Núñez P, Peláez JI. Sentiment Analysis and Emotion Understanding during the COVID-19 Pandemic in Spain and Its Impact on Digital Ecosystems. Int J Environ Res Public Health 2020;17:5542. [Crossref] [PubMed]
- Collins SA, Vawdrey DK. "Reading between the lines" of flow sheet data: nurses' optional documentation associated with cardiac arrest outcomes. Appl Nurs Res 2012;25:251-7. [Crossref] [PubMed]
- Ghassemi MM, Mark RG, Nemati S. A Visualization of Evolving Clinical Sentiment Using Vector Representations of Clinical Notes. Comput Cardiol (2010) 2015;2015:629-32.
- McCoy TH, Castro VM, Cagan A, et al. Sentiment Measured in Hospital Discharge Notes Is Associated with Readmission and Mortality Risk: An Electronic Health Record Study. PLoS One 2015;10:e0136341. [Crossref] [PubMed]
- Waudby-Smith IER, Tran N, Dubin JA, et al. Sentiment in nursing notes as an indicator of out-of-hospital mortality in intensive care patients. PLoS One 2018;13:e0198687. [Crossref] [PubMed]
- Le Gall JR, Lemeshow S, Saulnier F. A new Simplified Acute Physiology Score (SAPS II) based on a European/North American multicenter study. JAMA 1993;270:2957-63. [Crossref] [PubMed]
- Gong Y, Ding F, Zhang F, et al. Investigate predictive capacity of in-hospital mortality of four severity score systems on critically ill patients with acute kidney injury. J Investig Med 2019;67:1103-9. [Crossref] [PubMed]
- Vincent JL, Moreno R, Takala J, et al. The SOFA (Sepsis-related Organ Failure Assessment) score to describe organ dysfunction/failure. On behalf of the Working Group on Sepsis-Related Problems of the European Society of Intensive Care Medicine. Intensive Care Med 1996;22:707-10. [Crossref] [PubMed]
- Fernando SM, Rochwerg B, Seely AJE. Clinical implications of the Third International Consensus Definitions for Sepsis and Septic Shock (Sepsis-3). CMAJ 2018;190:E1058-9. [Crossref] [PubMed]
- Lee CW, Kou HW, Chou HS, et al. A combination of SOFA score and biomarkers gives a better prediction of septic AKI and in-hospital mortality in critically ill surgical patients: a pilot study. World J Emerg Surg 2018;13:41. [Crossref] [PubMed]
- Bai ZH, Guo XQ, Dong R, et al. A Nomogram to Predict the 28-day Mortality of Critically Ill Patients With Acute Kidney Injury and Treated With Continuous Renal Replacement Therapy. Am J Med Sci 2021;361:607-15. [Crossref] [PubMed]
(English Language Editor: A. Kassem)