A transcriptome-wide association study provides new insights into the etiology of osteoarthritis
Introduction
Osteoarthritis (OA), a common bone disease in clinical practice, is characterized by inflammation and articular cartilage damage and manifested as severe pain synovial edema, osteophytes, and subchondral sclerosis (1). During aging, the articular cartilage is destroyed, which leads to joint stiffness and deformity, ultimately leading to an inability to move (2). According to a survey in the United States, the prevalence of OA in community-dwelling adults can reach 10.4%, and the high cost of treatment for OA results in heavy economic burden (3). With the development of bioinformatics, more research is focusing on the genetic factors of OA. For example, genetic variants in the OPRM1 and OPRD1 genes have been shown to be associated with the pain phenotype of OA (4). This study suggests that genetic variants in certain genes may affect the sensitization of the nociceptive system, leading to increased sensitivity to noxious stimuli in humans, with OPRM1 thought to be associated with altered thresholds for pressure pain (from muscle) and OPRD1 thought to be associated with altered pain thresholds for contact heat stimulations (4). Genome-wide association studies (GWAS) have been widely used to identify variants associated with disease. A previous GWAS identified an OA-related locus on chromosome 12, located near the matrix Gla protein and CCDC91 gene (5). Although thousands of single-nucleotide polymorphisms (SNPs) related to human diseases have been identified by GWAS, certain limitations remain. For example, first, the disease-related loci identified by GWAS are often located in noncoding regions of the genome and usually require subsequent colocalization analysis to elucidate relevant functions and in vitro experiments for validation (6). Second, related studies have reported that insignificant regulatory variants in GWAS account for a large proportion of the genetic power of traits (7,8). Third, the locus identified by GWAS are often difficult to characterize biologically, and these studies associate locus with recent genes, which inevitably leads to a preference for long genes and does not necessarily accurately characterize the true role of the locus.
In contrast, because transcriptome-wide association studies (TWAS) use disease-associated cell types and tissues, and the availability of databases detailing tissue-specific expression, more interpretable biologically relevant results are available (9). The approach derives relationships between genotype and gene expression to create reference panels consisting of predictive models applicable to larger independent data sets (10). Ultimately, TWAS offers the opportunity to increase the ability to detect putative genes associated with the disease. Dall’Aglio et al. performed a TWAS analysis for major depression and identified 94 transcriptome-wide genes with significant difference, about half of which were not identified by the original GWAS analysis (11). This result suggested that TWAS was more valid than GWAS. In addition, some scholars have recently applied Mendelian randomization (MR) to the study of genetic variation as well. MR is a common method that allows the inference of causal relationships between exposures and disease outcomes (12). It treats genetic variants that are significantly associated with exposure (e.g., gene expression) as genetic tools that are then used to detect a causal effect of the outcome. If a causal relationship exists, the variation in exposure affects the outcome in proportion (12). Compared to observational epidemiology, MR has some advantages in its ability to control for environmental confounders (13). Further, Zhu et al. proposed a method named summary-data-based Mendelian randomization (SMR), by which Zhu et al. successfully identified 33 novel relevant genes for complex traits (14,15).
A previous TWAS for OA identified several variant genes by a loose significance threshold; however, an extensive description on the association was not provided and the sample size in the study was relatively small (6,858 OA patients and 27,478 controls) (16). Therefore, we performed TWAS and SMR analysis using more GWAS summary-data obtained from the UK Biobank (30,727 OA patients and 297,191 controls) to identify genetic loci and risk genes. The associations were also systematically characterized using confocal analysis, conditional analysis, fine-mapping analysis, and HaploReg (http://pubs.broadinstitute.org/mammals/haploreg/haploreg.php) (Figure 1). As a result, some of the shortcomings in previous studies were rectified and new insights were provided for subsequent functional studies on OA. We present the following article in accordance with the STREGA reporting checklist (available at https://atm.amegroups.com/article/view/10.21037/atm-22-4471/rc).
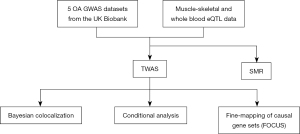
Methods
The study was conducted in accordance with the Declaration of Helsinki (as revised in 2013).
Study cohort
First, 5 OA GWAS datasets from the UK Biobank were used, including self-reported (SR), hospital diagnosis (HD), HD of knee OA (HD-knee), HD of hip OA (HD-hip), and HD of hip or knee OA (HD-hip/knee) (17). A total of 30,727 OA patients of European genetic ancestry and 297,191 controls were included. Second, SNP weights from different samples (European genetic ancestry) and tissues (muscle-skeletal tissue and whole blood) were used. The SNP weight indicated the correlation between the SNP and its annotated gene expression (9). SNP weights for muscle-skeletal tissue and whole blood were downloaded from the TWAS FUSION website (http://gusevlab.org/projects/fusion/#reference-functional-data), after selection based on previous literature (16). Finally, linkage disequilibrium (LD) from the 1000 Genomes Project Phase 3 European subset (n=489) was downloaded from the FUSION website (http://gusevlab.org/projects/fusion/) (18).
TWAS for OA
To identify the genes associated with the risk of OA, TWAS analyses were conducted using FUSION with default settings. FUSION leveraged a set of reference individuals to measure both gene expression and SNPs. The cis components of genetic expression were then utilized for imputation among a much larger set of individuals using the SNP genotype data (9). The precomputed expression reference weights of different tissues were downloaded from the FUSION websites. For TWAS of OA, 2 expression reference panels were applied, including muscle-skeletal tissue and whole blood (19). TWAS P value was obtained for each gene. In addition, the Bonferroni correction was applied to correct for significance threshold: P=0.05/number of genes (18). Further, we tested the association between the best SNPs in OA GWAS and the corresponding best expression quantitative trait loci (eQTL) SNPs in the Genotype-Tissue Expression (GTEx) project database (v7) using HaploReg (v4.1). Whether LD was met was determined based on r2.
Bayesian colocalization
To explore whether SNPs of GWAS could exert synergistic effects with eQTLs, we used the COLOC R package (https://cran.r-project.org/web/packages/coloc/,version5.1.0). All genes with transcriptome-wide significance (PTWAS <0.05) and within a 1.0-megabase (Mb) window were used in Bayesian colocalization analysis. Bayesian colocalization is driven by a shared causal variable or variant in strong LD and estimates the association between 2 outcomes (GWAS and eQTL signals) within a locus, i.e., the posterior probability (PP). PP4 is the set of PPs that we focused on. When PP4 >75% (20), GWAS and eQTL signals were consistent, and genes in the credible group that met this PP (PP4 >75%) were preferentially identified as putative causal genes (20).
Joint/conditional analysis
To determine whether multiple association features were independent signals, we performed conditional analysis on the genome-wide Bonferroni-corrected TWAS signals using FUSION software. The association gene model was used for each OA GWAS association with an SNP, 1 SNP at a time (21). In addition, conditional analysis demonstrated the extent to which the GWAS association signal within each locus could be explained by the functional associations detected by TWAS (22). Each significant locus was aligned using FUSION. The loci tested by alignment showed heterogeneity in expression capture, which ensured that the association was independent. In this study, 1,000 permutation tests were performed for each TWAS gene, with P<0.05 indicating the significance of the permutation test (22).
TWAS fine mapping
To address the issue of coregulation and LD, we used fine-mapping of causal gene sets (FOCUS) to prioritize genes with strong causal evidence in TWAS analysis (23). FOCUS integrates GWAS summary-data and eQTL weights (consistent with the eQTL reference panel used by TWAS) and includes LD for all SNPs in the risk region. TWAS hits were prioritized such that they were included in the default 90% confidence set, and a posteriori inclusion probability (PIP) was estimated. PIP value >0.5 for each gene in the region of interest indicated that the gene was more likely to be causally related than any other gene in the region of interest (11).
SMR analysis
To assess the relationship between genetic variation, gene expression, and OA, SMR (https://cnsgenomics.com/software/smr/) analysis was conducted (14). In SMR analysis, we integrated 2 eQTL datasets [muscle-skeletal tissue and whole blood from GTEx (v7)] (19) and 5 GWAS datasets (17) to examine pleiotropic associations between gene expression and OA. This method uses pooled data to perform transcriptome-wide association analysis and improves statistical power by using large sample sizes of GWAS and eQTL data.
In our SMR analysis, cis-eQTL was used as an instrumental variable, gene expression was the exposure, and OA was the outcome. To control the rate of genome-wide type I error, Bonferroni correction was used to account for multiple testing so that the significance level for each trait or tissue was P=0.05/number of genes. Since 2 eQTL datasets and 5 GWAS datasets were used, 10 significance thresholds were set (details are presented in Table S1 in the supplementary material). In addition, to assess whether the observed associations were caused by a single causal variable, heterogeneity in dependent instruments (HEIDI) was used to test the heterogeneity of the statistics on resulting associations, and the probes with low heterogeneity were retained (13). Only genes detected by SMR with PHEIDI >0.05 were considered significantly different. Finally, the results were visualized by R code provided by Zhu et al. (14).
Statistical analysis
Statistical analyses were conducted using the statistical computing programming language R (version 4.0.3). The results were visualized with R package qqman (https://cran.r-project.org/web/packages/qqman/index.html).
Results
TWAS analysis performed by FUSION
To reveal novel genes significantly associated with OA susceptibility, 5 publicly available GWAS datasets (SR, HD, HD-hip, HD-knee, and HD-hip/knee) were obtained from a European case-control cohort. In 2 SNP weight sets (muscle-skeletal tissue and whole blood), we identified 6 significant transcriptome-wide features (6 unique genes) (Table 1, Figure 2, Figure S1). Four significant transcriptome-wide loci were observed for the 6 unique genes (Figure 3). Since only significant features from 3 GWAS datasets (SR, HD-hip, and HD-hip/knee) were detected in this study, and no genes below the significance threshold were available in the other 2 GWAS datasets (HD and HD-knee), we only described and presented the relevant genes closest to the significance threshold in these 2 GWAS datasets (HD and HD-knee) (details are elucidated in Table S2 and Figure S2 in the supplementary material).
Table 1
Trait | Gene | Chr | Best GWAS IDa | eQTL IDb | TWAS Zc | TWAS P | Tissue |
---|---|---|---|---|---|---|---|
HD-hip | TCEA3 | 1 | rs1555024 | rs1555024 | −5.1 | 4.24E−07 | Whole blood |
ASAP3 | 1 | rs1555024 | rs1555024 | −5.0 | 6.63E−07 | Muscle-skeletal | |
ABCA9 | 17 | rs2521348 | rs2251855 | 5.2 | 1.78E−07 | Whole blood | |
SR | UQCC1 | 20 | rs224331 | rs1540927 | 5.2 | 2.00E−07 | Muscle-skeletal |
MYH7B | 20 | rs224331 | rs17092378 | −4.9 | 1.03E−06 | Whole blood | |
HD-hip/knee | RWDD2B | 21 | rs2832155 | rs2150403 | −5.4 | 5.93E−08 | Whole blood |
a, the SNP showed the most significant association with OA in this locus; b, the SNP showed the most significant association with gene expression in this locus; c, the Z statistic reflects the association strength between this gene and OA. Z<0 suggests that this gene was predicted to be downregulated in OA compared with controls and vice versa. TWAS, transcriptome-wide association studies; OA, osteoarthritis; Chr, chromosome; GWAS, genome-wide association studies; eQTL, expression quantitative trait loci; HD-hip, hospital diagnosis of hip OA; SR, self-reported; HD-hip/knee, hospital diagnosis of hip or knee OA; SNP, single-nucleotide polymorphism.
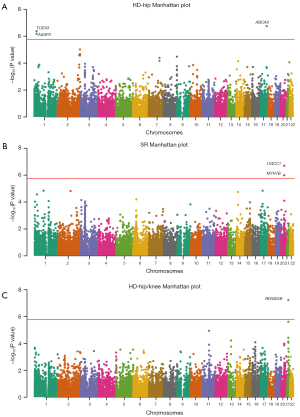
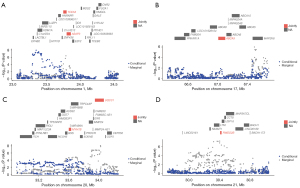
Chromosome 1q36.12
ASAP3 (PTWAS =6.63E−07) and TCEA3 (PTWAS =4.24E−07) are located within the chromosome 1q36.12 locus (Figure 3A). In this locus, the top GWAS SNP [rs1555024, odds ratio (OR) =0.84, PGWAS =4.24E−07] was the same as the best eQTL SNP in the GTEx database (v7) associated with the expression of ASAP3 (muscle-skeletal tissue) (PeQTL =1.11E−47) and TCEA3 (whole blood) (PeQTL =8.88E−5). Bayesian colocalization showed that PP4 for ASAP3 and TCEA3 was 99.9% and 83.7%, respectively, suggesting that GWAS and eQTL (muscle-skeletal tissue and whole blood) shared the same variant at this locus. To further determine whether multiple association features within the locus were independent signals, a conditional analysis was performed. The results showed that ASAP3 and TCEA3 genes were related to all signals at their loci (best SNP: rs1555024, PGWAS =4.24E−07; conditional on ASAP3 and TCEA3, PGWAS =1.000) (Table S3). In addition, to prioritize putative causal genes, FOCUS was used to assign a PIP for genes in each transcriptome-wide risk region and associated tissue type. However, no gene in the chromosome 1q36.12 locus was included in the default 90% confidence set, which was probably due to few genes meeting the default significance threshold of FUSION in the GWAS dataset used in our study (17).
Chromosome 17q24.2
Within the chromosome 17q24.2 locus, ABCA9 (PTWAS =1.78E−07) corresponded to a significant association feature with transcriptome-wide significance (Figure 3B). In this locus, rs2521348 was the most significant SNP associated with OA (OR =1.18, PGWAS =1.01E−06) Furthermore, rs2251855 was the best eQTL SNP associated with the expression of ABCA9 (PeQTL =4.98E−4) in whole blood from the GTEx database (v7), which showed a strong LD with rs2521348 (r2=0.8). Bayesian colocalization analysis demonstrated a PP4 of 70.6% for ABCA9, suggesting that GWAS and eQTL (whole blood) might be driven by the same causal variant on the ABCA9 locus. In addition, conditional analysis found that ABCA9 was related to 98.8% of the signal at this locus (best SNP: rs2521348, PGWAS =1.01E−06; conditional on ABCA9, PGWAS =0.588) (Table S3).
Chromosome 20q11.22
Both UQCC1 (PTWAS =2.00E−07) and MYH7B (PTWAS =1.03E−06) were located within the chromosome 20q11.22 locus (Figure 3C). In this locus, rs224331 was the most significant SNP associated with OA (OR =0.91, PGWAS =8.17E−09). Furthermore, rs1540927 was the best eQTL SNP associated with the expression level of UQCC1 (PeQTL =2.05E−25) in muscle-skeletal tissue from the GTEx database (v7), which showed a strong LD with rs224331 (r2=0.81). Rs17092378 was the best eQTL SNP associated with the expression level of MYH7B (PeQTL =6.75E−5) in whole blood from the GTEx database (v7), which showed a weak LD with rs224331. In addition, Bayesian colocalization analysis presented a PP4 of 95.9% for UQCC1, suggesting that the significant GWAS signal and the expression level of the UQCC1 gene were driven by the same causal variant. However, PP4 was 8.6% for MYH7B gene in the same genomic region. Thus, we could not conclude that the significant GWAS signal and whole-blood eQTL signaling shared the same variants at their loci.
The results of the conditional analysis showed that UQCC1 and MYH7B were related to all signals at its loci (best SNP: rs224331, PGWAS =8.17E−09; conditioned on UQCC1 and MYH7B, PGWAS =1.000) (Table S3). In addition, FOCUS results showed that both UQCC1 (muscle-skeletal tissue, PIP =0.295) and MYH7B (whole blood, PIP =0.00326) were also included in the 90% confidence set within the genomic locus 20:32813689-20:34960446.
Chromosome 21q21.3
In the chromosome 21q21.3 locus, only RWDD2B (PTWAS =5.93E−08) corresponded to a significant association feature with a transcriptome-wide significance (Figure 3D). Within this locus, rs2832155 was the most significant SNP associated with OA (OR =1.13, PGWAS =5.39E−08). In addition, rs2150403 was the best eQTL SNP associated with the expression of RWDD2B (PeQTL =1.55E−12) in whole blood from the GTEx database (v7), which showed an extremely strong LD with rs2832155 (r2=0.99). Bayesian colocalization analysis showed that PP4 was 99.6% for RWDD2B, indicating that GWAS had the same variation as eQTL (whole blood) at the RWDD2B locus. Conditional analysis showed that RWDD2B was related to 99.6% of the signals at this locus (best SNP: rs2832155, PGWAS =5.39E−08; conditional on RWDD2B, PGWAS =0.148) (Table S3). Within the genomic locus 20:32813689-20:34960446, RWDD2B (whole blood, PIP =0.0238) was included in the 90% confidence set of genes.
SMR analysis results
Next, we performed SMR using GWAS and eQTL data. Two risk genes related to OA were identified by SMR integrative analysis: UQCC1 (PSMR =2.05E−6, beta =0.311674) and ASAP3 (PSMR =1.49E−6, beta =−0.374363) (corrected by Bonferroni multiple comparison testing) (Figure 4, Table 2). In addition, the HEIDI test results found that UQCC1 and ASAP3 could pass the heterogeneity test (PHEIDI >0.05) (13). Overall, this MR study showed a causal relationship between high UQCC1 expression and low ASAP3 expression and OA risk (Figure S3).
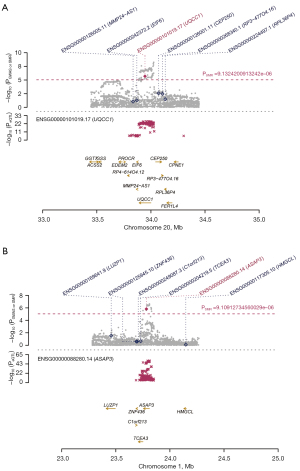
Table 2
Gene | Chr | Top SNP | Top SNP_Chr | A1 | A2 | HEIDI Pa | SMR P | Tissue | Trait |
---|---|---|---|---|---|---|---|---|---|
UQCC1 | 20 | rs6579234 | 20 | G | A | 2.14E−1 | 2.05E−6 | Muscle-skeletal | SR |
ASAP3 | 1 | rs6686497 | 1 | C | T | 5.07E−1 | 1.49E−6 | Muscle-skeletal | HD-hip |
a, HEIDI test was used to distinguish pleiotropy from the linkage. If a gene passes the HEIDI test (P>0.05), it suggests that there is a single causal variant influencing both disease risk and gene expression. Thus, expression change of this gene may have a role in disease susceptibility. SMR, summary-data-based Mendelian randomization; OA, osteoarthritis; Chr, chromosome; SNP, single-nucleotide polymorphism; HEIDI, heterogeneity in dependent instruments; HD-hip, hospital diagnosis of hip OA; SR, self-reported; OA, osteoarthritis.
Comparison with previous literature
The significance threshold in previous TWAS for OA was set loosely (P<0.05) (16), and thus some spurious OA risk genes might have been included in the results. Our study used Bonferroni correction to correct for significance thresholds to obtain more reasonable results. A previous TWAS for OA (16) identified a total of 572 risk genes using TWAS analysis, among which ASAP3, TCEA3, UQCC1, and RWDD2B risk genes overlapped with our TWAS results. The 5 GWAS datasets used in our study were derived from the study by Zengini et al. (17). By constructing Manhattan plots (Figure S4) and quantile-quantile plots (Figure S5) for these 5 datasets, we found that the observed P value distribution was well matched with the expected one, indicating less overall inflation of genome-wide statistics. In addition, by comparing our results with the GWAS for OA by Zengini et al. (17), we found that 2 important TWAS genes (UQCC1 and RWDD2B) overlapped with the GWAS results by Zengini et al. (17). Overall, our study identified 2 new OA susceptibility genes (ABCA9 and MYH7B) compared with the previous TWAS and GWAS (16,17).
Discussion
OA is one of the more common musculoskeletal disorders at present (24), and it is also the leading cause of disability worldwide. OA affects 40% of people over 70 years of age and is associated with a higher risk of comorbidity and death (25). Although GWAS has recently been successfully applied in identifying risk loci associated with OA, the functional significance of these associations remains elusive because tissue-specific and tissue-associated genes cannot be finely mapped. Our study aimed to detect candidate genes closely associated with OA and explain the relationship between these genes and the disease. First, we identified UQCC1, MYH7B, ABCA9, TCEA3, ASAP3, and RWDD2B as risk genes for OA by integrating the larger GWAS dataset from UK Biobank and eQTL data for TWAS analysis. MYH7B and ABCA9, which were not identified in previous TWAS and GWAS (16,17,26-28), were localized in 4 different regions: chromosome 1q36.12, chromosome 17q24.2, chromosome 20q11.22, and chromosome 21q21.3.
We further investigated the significant associations through a conditional analysis to determine whether gene associations within the same genomic region were independent or whether the associations resulted from correlated predicted expression. The results showed that the 6 risk genes adequately explained most of the genetic variation signals in the region where the 4 loci were located. We compared GWAS (17,28) summary statistics before and after conditioning on significant TWAS associations. It was revealed that GWAS (17,28) associations could be explained to a major extent by TWAS associations, further suggesting the possibility of transcriptomic mediation in genetic risk for OA.
To further determine whether GWAS and eQTL signals were driven by the same variants, we performed a Bayesian colocalization analysis to calculate PP4 of each gene. We found that 4 of them were greater than 75% (20), indicating that these 4 significant features could be considered as colocalized states and the same genetic variants could drive the association with OA through these 4 features. Although the importance of several candidate genes was demonstrated, none of the above results could identify whether their association with OA was causal or not. Therefore, SMR analysis was conducted and corrected by Bonferroni's multiple comparison test. Two genes, UQCC1 and ASAP3, were identified, and both passed the test of heterogeneity (PHEIDI >0.05) (13), and thus their association with OA was considered a causal effect.
Our study again demonstrated the importance of the ASAP3, TCEA3, UQCC1, and RWDD2B genes in OA genetic variation. Related studies have shown that UQCC1 is expressed during chondrocyte differentiation (29). In humans, polymorphisms in this gene are mostly associated with bone mass (30), hip dysplasia (31), hip shaft length (32), and height (33). Additionally, 2 genome-wide and candidate gene association studies showed that common variants between growth/differentiation factor 5 (GDF5) and UQCC1 resulted in a 2-fold increased risk of OA in the hip and knee joints (33,34). ASAP3, a member of the ASAP subfamily, is mainly involved in cellular endocytosis and actin cytoskeleton remodeling (35). According to an earlier study, the content of cytoskeletal actin and wave proteins in knee chondrocytes varied greatly in OA patients but remained relatively constant in healthy people (36). TCEA3, an isoform of the transcriptional elongation factor TFIIS, has been shown to promote cell differentiation and myotubular fusion (37). TCEA3 can regulate the proliferation of muscle precursor cells and myotube formation by interacting with myogenic regulatory factors, facilitating the recruitment of RNA polymerase II (RNAPII) to the promoter, and moving with RNAPII to the coding region of the gene (38,39). RWDD2B has been confirmed as a target for OA-related genetic and epigenetic effects by a previous study (40).
In addition, based on previous studies, we identified 2 susceptibility genes for OA, namely MYH7B and ABCA9. MYH7B is a myosin heavy chain gene, expressed in slow-twitch muscle fibers (type I fibers) (41,42). Altered types and reduced numbers of muscle fibers are thought to be associated with quadriceps weakness and pain in knee OA (43,44). A recent study showed a significant increase in type IIa/x mixed fibers and a significant decrease in type I fibers in the muscle of the OA group compared to the control group (45). ABCA9, a member of the adenosine triphosphate (ATP)-binding cassette (ABC) transporter superfamily (46), plays an important role in intracellular cholesterol storage (47). Cholesterol metabolic pathways have a regulatory role in cartilage differentiation, and dysfunction of the pathways may disrupt the physiological metabolism of chondrocytes, which in turn leads to the development of OA (48).
The discovery of novel genetic risk factors may hasten the development of predictive biomarkers and personalized OA therapies (49). The development of TWAS has, in some ways, restricted the screening scope of OA genetic risk genes. Firstly, we found two significant transcriptome-wide loci and six risk genes using TWAS analysis; Bayesian colocalization discovered UQCC1, ABCA9, TCEA3, ASAP3, and RWDD2B as potential causal genes; according to the joint/conditional analysis, all six risk genes were correlated with the majority of the signals on their loci. Through TWAS fine mapping, the UQCC1 and MYH7B risk genes were found more likely to be causative than other genes in the region of interest. Indeed, some OA medications in current clinical trials, such as intra-articular TGF-b and FGF18 growth factor treatments and Wnt inhibitors (50), target proteins from GWAS-identified genes (17,28). Is it possible to reposition therapeutic modalities for drugs that are clinically correlated with new genetic risk factors (for the treatment of other diseases)? This requires further exploration. Furthermore, our study failed to explain the correlation between joint sites and genetic risk variants, despite the use of GWAS datasets including knee, hip, hip/knee, and all OA (SR and HD), and the discovery of respective relevant genes and risk loci. In addition, a previous study showed that the association between genetically determined BMI and femoral neck bone mineral density varied with joint sites in humans (51). This indicates that exploring the association between genetic variation and phenotype is helpful for an indirect probe into the link between the joint site and genetic risk variation.
A prior epidemiological study also found that cholesterol metabolism had a strong association with hand, knee, hip, and systemic OA (52). Based on our analysis results and other investigators’ reports (47,48), genetic variants in the ABCA9 risk gene are considered to impact the occurrence and progression of OA via regulating cholesterol metabolism. As a result, it becomes a critical follow-up issue to investigate the relationship between genetic variants of ABCA9 gene and cholesterol metabolism in OA patients, which may also drive the development of personalized management models for patients with OA. Similarly, this holds true for subsequent studies on the association between genetic variants in the remaining risk genes (UQCC1, MYH7B, TCEA3, ASAP3, and RWDD2B) and OA.
In conclusion, we performed TWAS and SMR analysis by integrating larger GWAS pooled data on OA and expression weight sets. New OA-associated susceptibility genes and risk loci were found, and the identified associations were extensively characterized using confocal analysis, conditional analysis, fine-mapping analysis, and HaploReg. We provided new insights for subsequent studies on OA and also highlighted the potential and importance of resource integration in the era of big data for biomedical research. However, our study also had some limitations. First, only individuals of European ancestry were included in the analysis, and therefore the results might not be applicable to other ethnic groups. Second, although the sample size included in the current study was larger than the previous study, it was still somewhat restrictive, leading to possible bias in the results. Third, the results were not validated by experiments in this study, and the reliability of the results still need to be examined.
Acknowledgments
The authors thank all researchers and participants of the UK Biobank for sharing these data.
Funding: This study was funded by the Guangxi Natural Science Foundation Project (grant No. 2021GXNSFAA196033) and the Guangxi Appropriate Technology Development and Promotion of Chinese Medicine Project (grant No. GZSY21-78).
Footnote
Reporting Checklist: The authors have completed the STREGA reporting checklist. Available at https://atm.amegroups.com/article/view/10.21037/atm-22-4471/rc
Conflicts of Interest: All authors have completed the ICMJE uniform disclosure form (available at https://atm.amegroups.com/article/view/10.21037/atm-22-4471/coif). All authors report that this study was funded by the Guangxi Natural Science Foundation Project (grant No. 2021GXNSFAA196033) and the Guangxi Appropriate Technology Development and Promotion of Chinese Medicine Project (grant No. GZSY21-78). The authors have no other conflicts of interest to declare.
Ethical Statement: The authors are accountable for all aspects of the work in ensuring that questions related to the accuracy or integrity of any part of the work are appropriately investigated and resolved. The study was conducted in accordance with the Declaration of Helsinki (as revised in 2013).
Open Access Statement: This is an Open Access article distributed in accordance with the Creative Commons Attribution-NonCommercial-NoDerivs 4.0 International License (CC BY-NC-ND 4.0), which permits the non-commercial replication and distribution of the article with the strict proviso that no changes or edits are made and the original work is properly cited (including links to both the formal publication through the relevant DOI and the license). See: https://creativecommons.org/licenses/by-nc-nd/4.0/.
References
- Zeng WN, Zhang Y, Wang D, et al. Intra-articular Injection of Kartogenin-Enhanced Bone Marrow-Derived Mesenchymal Stem Cells in the Treatment of Knee Osteoarthritis in a Rat Model. Am J Sports Med 2021;49:2795-809. [Crossref] [PubMed]
- Abramoff B, Caldera FE. Osteoarthritis: Pathology, Diagnosis, and Treatment Options. Med Clin North Am 2020;104:293-311. [Crossref] [PubMed]
- Lo J, Chan L, Flynn S. A Systematic Review of the Incidence, Prevalence, Costs, and Activity and Work Limitations of Amputation, Osteoarthritis, Rheumatoid Arthritis, Back Pain, Multiple Sclerosis, Spinal Cord Injury, Stroke, and Traumatic Brain Injury in the United States: A 2019 Update. Arch Phys Med Rehabil 2021;102:115-31. [Crossref] [PubMed]
- Olesen AE, Nielsen LM, Feddersen S, et al. Association Between Genetic Polymorphisms and Pain Sensitivity in Patients with Hip Osteoarthritis. Pain Pract 2018;18:587-96. [Crossref] [PubMed]
- den Hollander W, Boer CG, Hart DJ, et al. Genome-wide association and functional studies identify a role for matrix Gla protein in osteoarthritis of the hand. Ann Rheum Dis 2017;76:2046-53. [Crossref] [PubMed]
- Hindorff LA, Sethupathy P, Junkins HA, et al. Potential etiologic and functional implications of genome-wide association loci for human diseases and traits. Proc Natl Acad Sci U S A 2009;106:9362-7. [Crossref] [PubMed]
- Lee D, Gorkin DU, Baker M, et al. A method to predict the impact of regulatory variants from DNA sequence. Nat Genet 2015;47:955-61. [Crossref] [PubMed]
- Boyle EA, Li YI, Pritchard JK. An Expanded View of Complex Traits: From Polygenic to Omnigenic. Cell 2017;169:1177-86. [Crossref] [PubMed]
- Gusev A, Ko A, Shi H, et al. Integrative approaches for large-scale transcriptome-wide association studies. Nat Genet 2016;48:245-52. [Crossref] [PubMed]
- Wainberg M, Sinnott-Armstrong N, Mancuso N, et al. Opportunities and challenges for transcriptome-wide association studies. Nat Genet 2019;51:592-9. [Crossref] [PubMed]
- Dall'Aglio L, Lewis CM, Pain O. Delineating the Genetic Component of Gene Expression in Major Depression. Biol Psychiatry 2021;89:627-36. [Crossref] [PubMed]
- Davey Smith G, Hemani G. Mendelian randomization: genetic anchors for causal inference in epidemiological studies. Hum Mol Genet 2014;23:R89-98. [Crossref] [PubMed]
- Mo X, Guo Y, Qian Q, et al. Mendelian randomization analysis revealed potential causal factors for systemic lupus erythematosus. Immunology 2020;159:279-88. [Crossref] [PubMed]
- Zhu Z, Zhang F, Hu H, et al. Integration of summary data from GWAS and eQTL studies predicts complex trait gene targets. Nat Genet 2016;48:481-7. [Crossref] [PubMed]
- Pavlides JM, Zhu Z, Gratten J, et al. Predicting gene targets from integrative analyses of summary data from GWAS and eQTL studies for 28 human complex traits. Genome Med 2016;8:84. [Crossref] [PubMed]
- Qi X, Yu F, Wen Y, et al. Integration of transcriptome-wide association study and messenger RNA expression profile to identify genes associated with osteoarthritis. Bone Joint Res 2020;9:130-8. [Crossref] [PubMed]
- Zengini E, Hatzikotoulas K, Tachmazidou I, et al. Genome-wide analyses using UK Biobank data provide insights into the genetic architecture of osteoarthritis. Nat Genet 2018;50:549-58. [Crossref] [PubMed]
- Pain O, Pocklington AJ, Holmans PA, et al. Novel Insight Into the Etiology of Autism Spectrum Disorder Gained by Integrating Expression Data With Genome-wide Association Statistics. Biol Psychiatry 2019;86:265-73. [Crossref] [PubMed]
- Carithers LJ, Moore HM. The Genotype-Tissue Expression (GTEx) Project. Biopreserv Biobank 2015;13:307-8. [Crossref] [PubMed]
- Thériault S, Gaudreault N, Lamontagne M, et al. A transcriptome-wide association study identifies PALMD as a susceptibility gene for calcific aortic valve stenosis. Nat Commun 2018;9:988. [Crossref] [PubMed]
- Park S, Kim D, Song J, et al. An Integrative Transcriptome-Wide Analysis of Amyotrophic Lateral Sclerosis for the Identification of Potential Genetic Markers and Drug Candidates. Int J Mol Sci 2021;22:3216. [Crossref] [PubMed]
- Liao C, Laporte AD, Spiegelman D, et al. Transcriptome-wide association study of attention deficit hyperactivity disorder identifies associated genes and phenotypes. Nat Commun 2019;10:4450. [Crossref] [PubMed]
- Mancuso N, Freund MK, Johnson R, et al. Probabilistic fine-mapping of transcriptome-wide association studies. Nat Genet 2019;51:675-82. [Crossref] [PubMed]
- Loeser RF, Goldring SR, Scanzello CR, et al. Osteoarthritis: a disease of the joint as an organ. Arthritis Rheum 2012;64:1697-707. [Crossref] [PubMed]
- Cibrián Uhalte E, Wilkinson JM, Southam L, et al. Pathways to understanding the genomic aetiology of osteoarthritis. Hum Mol Genet 2017;26:R193-201. [Crossref] [PubMed]
- Li P, Ning Y, Guo X, et al. Integrating transcriptome-wide study and mRNA expression profiles yields novel insights into the biological mechanism of chondropathies. Arthritis Res Ther 2019;21:194. [Crossref] [PubMed]
- Xu J, Zeng Y, Si H, et al. Integrating transcriptome-wide association study and mRNA expression profile identified candidate genes related to hand osteoarthritis. Arthritis Res Ther 2021;23:81. [Crossref] [PubMed]
- Tachmazidou I, Hatzikotoulas K, Southam L, et al. Identification of new therapeutic targets for osteoarthritis through genome-wide analyses of UK Biobank data. Nat Genet 2019;51:230-6. [Crossref] [PubMed]
- Goldring MB, Tsuchimochi K, Ijiri K. The control of chondrogenesis. J Cell Biochem 2006;97:33-44. [Crossref] [PubMed]
- Deng FY, Dong SS, Xu XH, et al. Genome-wide association study identified UQCC locus for spine bone size in humans. Bone 2013;53:129-33. [Crossref] [PubMed]
- Sun Y, Wang C, Hao Z, et al. A common variant of ubiquinol-cytochrome c reductase complex is associated with DDH. PLoS One 2015;10:e0120212. [Crossref] [PubMed]
- Soranzo N, Rivadeneira F, Chinappen-Horsley U, et al. Meta-analysis of genome-wide scans for human adult stature identifies novel Loci and associations with measures of skeletal frame size. PLoS Genet 2009;5:e1000445. [Crossref] [PubMed]
- Sanna S, Jackson AU, Nagaraja R, et al. Common variants in the GDF5-UQCC region are associated with variation in human height. Nat Genet 2008;40:198-203. [Crossref] [PubMed]
- Miyamoto Y, Mabuchi A, Shi D, et al. A functional polymorphism in the 5' UTR of GDF5 is associated with susceptibility to osteoarthritis. Nat Genet 2007;39:529-33. [Crossref] [PubMed]
- Ha VL, Bharti S, Inoue H, et al. ASAP3 is a focal adhesion-associated Arf GAP that functions in cell migration and invasion. J Biol Chem 2008;283:14915-26. [Crossref] [PubMed]
- Kouri JB, Argüello C, Luna J, et al. Use of microscopical techniques in the study of human chondrocytes from osteoarthritic cartilage: an overview. Microsc Res Tech 1998;40:22-36. [Crossref] [PubMed]
- Zhu Y, Tong HL, Li SF, et al. Effect of TCEA3 on the differentiation of bovine skeletal muscle satellite cells. Biochem Biophys Res Commun 2017;484:827-32. [Crossref] [PubMed]
- Kazim N, Adhikari A, Davie J. The transcription elongation factor TCEA3 promotes the activity of the myogenic regulatory factors. PLoS One 2019;14:e0217680. [Crossref] [PubMed]
- Bentzinger CF, Wang YX, Rudnicki MA. Building muscle: molecular regulation of myogenesis. Cold Spring Harb Perspect Biol 2012;4:a008342. [Crossref] [PubMed]
- Parker E, Hofer IMJ, Rice SJ, et al. Multi-Tissue Epigenetic and Gene Expression Analysis Combined With Epigenome Modulation Identifies RWDD2B as a Target of Osteoarthritis Susceptibility. Arthritis Rheumatol 2021;73:100-9. [Crossref] [PubMed]
- Aksel T, Choe Yu E, Sutton S, et al. Ensemble force changes that result from human cardiac myosin mutations and a small-molecule effector. Cell Rep 2015;11:910-20. [Crossref] [PubMed]
- Walklate J, Geeves MA. Temperature manifold for a stopped-flow machine to allow measurements from -10 to +40°C. Anal Biochem 2015;476:11-6. [Crossref] [PubMed]
- Fink B, Egl M, Singer J, et al. Morphologic changes in the vastus medialis muscle in patients with osteoarthritis of the knee. Arthritis Rheum 2007;56:3626-33. [Crossref] [PubMed]
- Serrão PR, Vasilceac FA, Gramani-Say K, et al. Men with early degrees of knee osteoarthritis present functional and morphological impairments of the quadriceps femoris muscle. Am J Phys Med Rehabil 2015;94:70-81. [Crossref] [PubMed]
- Noehren B, Kosmac K, Walton RG, et al. Alterations in quadriceps muscle cellular and molecular properties in adults with moderate knee osteoarthritis. Osteoarthritis Cartilage 2018;26:1359-68. [Crossref] [PubMed]
- Locher KP. Mechanistic diversity in ATP-binding cassette (ABC) transporters. Nat Struct Mol Biol 2016;23:487-93. [Crossref] [PubMed]
- Karasinska JM, Rinninger F, Lütjohann D, et al. Specific loss of brain ABCA1 increases brain cholesterol uptake and influences neuronal structure and function. J Neurosci 2009;29:3579-89. [Crossref] [PubMed]
- Gentili C, Tutolo G, Pianezzi A, et al. Cholesterol secretion and homeostasis in chondrocytes: a liver X receptor and retinoid X receptor heterodimer mediates apolipoprotein A1 expression. Matrix Biol 2005;24:35-44. [Crossref] [PubMed]
- Grandi FC, Bhutani N. Epigenetic Therapies for Osteoarthritis. Trends Pharmacol Sci 2020;41:557-69. [Crossref] [PubMed]
- Oo WM, Hunter DJ. Disease modification in osteoarthritis: are we there yet? Clin Exp Rheumatol 2019;37:135-40. [PubMed]
- Funck-Brentano T, Nethander M, Movérare-Skrtic S, et al. Causal Factors for Knee, Hip, and Hand Osteoarthritis: A Mendelian Randomization Study in the UK Biobank. Arthritis Rheumatol 2019;71:1634-41. [Crossref] [PubMed]
- Gkretsi V, Simopoulou T, Tsezou A. Lipid metabolism and osteoarthritis: lessons from atherosclerosis. Prog Lipid Res 2011;50:133-40. [Crossref] [PubMed]
(English Language Editor: A. Muylwyk)