Biomarkers of coordinate metabolic reprogramming and the construction of a co-expression network in colorectal cancer
Introduction
Colorectal cancer (CRC) is one of the most common digestive tract malignancies worldwide, with the third highest incidence and the second highest mortality (1,2). In China, CRC ranks second in morbidity and mortality among various cancers. Despite the continuous improvement of screening strategies and treatment methods, distant metastases are present in approximately 25% of patients at initial diagnosis, and half of CRC patients will develop metastatic disease (3). The 5-year survival rate of advanced CRC patients is low, which seriously threatens human health. The pathogenesis of CRC is complex and is affected by multiple factors, including lifestyle, genetic factors, inflammation, intestinal microbiota, and so on (4,5).
Currently, there are several ways to detect CRC, such as fecal occult blood test (FOBT), proctoscopy, colonoscopy, computed tomography (CT) scan, and multi-target stool DNA testing (6,7). These techniques have limitations: potentially invasive; can be uncomfortable for the patient or have risks of bleeding, bowel tears, or infection in the cases of colonoscopy or proctoscopy; or exhibit low sensitivity or false-positive results in the case of the FOBT, multi-target stool DNA testing, or CT scan. Therefore, research aimed at developing new screening strategies and biomarkers for the early detection of CRC is urgently needed. Compared with the current methods, new non-invasive molecular tests based on the detection of CRC alterations seem to be more sensitive and specific (8).
Altered metabolism is one of the defining features of cancer (9). Cancer cells remodel their metabolic network to support biogenesis, caused by or resulting in the dysregulation of various metabolites. Metabolomics refers to a comprehensive analysis and integration of different biological metabolites conducted using either nuclear magnetic resonance (NMR) spectroscopy or a form of coupled chromatography-mass spectrometry (10,11). Determining the final products of metabolism of the host or microbial community could reveal the direct characteristics of the subject’s current biochemical state (12,13). Metabolite signalling is involved in the regulation of malignant transformation, cell proliferation, epithelial-to-mesenchymal transition, cancer stemness, drug resistance and so on (14,15). Additionally, intercellular metabolite signalling modulates inflammatory response and immunosurveillance in the tumor microenvironment. An in depth understanding of metabolite signaling will provide new opportunities for the development of therapeutic interventions that target cancer. At present, it is one of the most effective research methods and has been widely used to study the metabolite changes in the process of various diseases and identify the biomarkers of a multitude of cancers, including CRC (16-18). Liu et al. found that the risk of CRC was positively correlated with the production of damaged intestinal epithelium biomarkers, including diamine oxide, D-lactic acid, and lipopolysaccharide (LPS) (19). Shu et al. reported that lipid metabolism, especially glycerol phospholipid metabolism, is directly related to the risk of CRC (20). Previous studies have shown the important predictive role of metabolic disorders in CRC patients by comparing differential metabolites between CRC patients and healthy controls (21-23). It is also worth to mention that the metabolic reprogramming takes place at the paracancerous tissues and at the early stages of CRC. Thus, in our current study, we included the different stages of CRC patients and demonstrated the differential metabolic characteristics between the cancer, paracancerous, and normal tissues.
In this study, liquid chromatography-mass spectrometry (LC-MS)-based tissue metabolomics was performed to analyze the metabolites of CRC patients in different tissues and stages. Our findings may be of great importance in identifying potential non-invasive, early biomarkers for CRC progression. We present the following article in accordance with the STREGA reporting checklist (available at https://atm.amegroups.com/article/view/10.21037/atm-22-4767/rc).
Methods
Study design, setting, and participants
Nine rectal cancer patients with a scheduled total mesorectal excision (TME) in the Department of Anorectal Surgery, Hwa Mei Hospital, were recruited for this study. These nine patients had similar clinical characteristics (male; age 62±6 years; no metastasis; no use of antibiotics within 2 months), and were all local people with similar dietary habits and living environments. According to the TNM Classification of Malignant Tumors (TNM), the patients were classified in three different stages of cancer (stages IIA, IIB, and IIIC), and the three patients in the same stage had a smaller age gap. With the patients’ informed consent, their cancer, paracancerous, and normal tissues were collected during cancer resection (Table S1). The study was conducted in accordance with the Declaration of Helsinki (as revised in 2013). The study was approved by the ethics committee of Hwa Mei Hospital, the University of Chinese Academy of Sciences (No. SL-NBYJK-KY-2022-120-01). Informed consent was obtained from all of the individual participants.
Metabolome testing
In this study, ultra-high-performance liquid chromatography-tandem mass spectrometry (Thermo Scientific UltiMate 3000 HPLC; Thermo Scientific, (Hangzhou, Zhejiang)) was used for metabolome profiling. A high-resolution mass spectrometer (Q-Exactive; Thermo Scientific) was used to detect the samples. XCMS software (Gary Siuzdak; America) was used to perform peak alignment processing on the mass spectrometry data to more accurately quantify the metabolites. We used Compound Discoverer 3.1.0 software (Thermo Fisher Scientific, USA) to perform metabolite identification on the mass spectrometry raw data, including peak extraction, baseline filtering, sample retention time alignment, adduction pooling, missing value filling, and finally, information obtaining. Metabolite identification combines an in-house metabolite secondary mass spectrometry library, mzCloud, mzVault, Mass list, ChemSpider (Human Metabolome Database (HMDB), Kyoto Encyclopedia of Genes and Genomes (KEGG), LipidMaps) and multiple databases.
Multivariate statistical analysis
We used the vegan (version 2.6-2) and stats (version 3.6.0) R packages to perform principal component analysis (PCA). Specifically, we first calculated the z-score on the profile and then used the prcomp function to perform dimensionality reduction analysis. Orthogonal partial least-squares discriminant analysis (OPLS-DA) also represents an alternative method for selecting the most significant metabolites as biomarkers. The fitting parameter (R2) and predictive ability parameter (Q2) were utilized to evaluate the quality of the models. A two-tailed Student’s t-test was used to verify the significance of the differences between the groups. Metabolites with VIP (Variable Importance for the Projection) >1.0 and P<0.05 were selected as differentially abundant. Fold change was defined as the average mass response (area) ratio between two groups. Metabolic data were all log-transformed and normalized.
Trend analysis of the deterioration degree of CRC
Short Time-series Expression Mine software (STEM) (performed on OmicShare tools platform; www.omicshare.com/tools) simulates the possible change trends and then calculates the correlation coefficient between each factor and the preset trend, and divides the factors into the most similar trends. We preprocessed the data by log2 and set 1.2 as the minimum fold change of the trend. Finally, a Venn diagram was analyzed.
Weighted Gene Correlation Network Analysis (WGCNA)
WGCNA, an analytical method based on correlation pattern recognition and phenotype correlation, has been widely applied in clinical disease research. WGCNA can cluster genes with similar expression patterns and further analyze the relationship between modules and specific traits or phenotypes. Therefore, this method was applied in our current study. We screened the top 50% of metabolites using analysis of variance (ANOVA) and formed 14 effective modules by hierarchical clustering of the topological overlap matrix. The relevant metabolites were selected from the significant modules associated with different tissues and ages by correlation analysis and KEGG pathway enrichment analysis.
Statistical analysis
To calculate the mean and standard deviation, the numerical data were analyzed by one-way ANOVA. A two-tailed Student’s t-test was used to verify the significance of the differences between the groups. P value <0.05 was considered as statistical significance.
Results
Multivariate statistical analysis of samples
By using the untargeted LC/MS metabolomics method described, we extracted a total of 5,834 metabolites from the included samples. Among them, there were 960 metabolites annotated on the HMDB database and the specific classification results are shown in the HMDB Super class classification map (Figure S1). Surprisingly, based on the 27 samples, the PCA results revealed that the three groups overlapped significantly, which can be attributed to the similarity between the adjacent and cancer tissues (Figure S2A) and the insignificant differences between different cancer stages (Figure S2B).
Since PCA did not distinguish the differences between the samples well, we verified the permutation analysis using the Orthogonal Partial Least Squares Discriminant Analysis (OPLS-DA) model. As shown in Figure 1, permutation analysis showed a clear separation between the cancer, paracancerous, and normal tissues (Q2=0.412, R2X=0.617, R2Y=0.844, Figure 1A), and a clear separation between the different rectal cancer stages (stage IIA, stage IIB, stage IIIC, Q2=0.452, R2X=0.64, R2Y=0.986, Figure 1B).
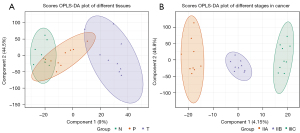
Identification of the differential metabolites in different cancerous tissues
Based on the above results, we further screened the differential metabolites in rectal cancers of different stages and tissues. First, we compared the differential metabolites between tumor (T), normal (N), and paracancerous (P) tissues of the same stage by OPLS_DA, and also included the overall tissue differences that did not differentiate between the various stages. Next, we classified the metabolites that were up-regulated or down-regulated in the same group by Venn diagram classification and obtained the key metabolites.
As shown in Figure 2, we found that, except for stage IIB, the samples had fewer differential metabolites between the paracancerous and normal tissues, indicating that the two tissues have marked similarities. Meanwhile, there were more differential metabolites, especially up-regulated metabolites, between the tumor tissues and other tissues, indicating that the metabolites were altered and some key metabolites may play a role during tumor development.
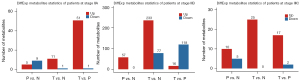
Notably, as shown in Figure S3, compared with the normal tissues, tumor tissues in stage IIB had more differential metabolites (233 up-regulated and 77 down-regulated). In addition, the Venn diagram classification demonstrated that the differential metabolites in stage IIB were very similar to those that were not divided by cancer stage (170 common up-regulated metabolites and 38 down-regulated metabolites). These 170 metabolites included hydroxyquinolines, phosphate phosphates, fatty acyls, as well as steroids and steroid derivatives.
Trend analysis of tumor progression in the different stages
To further explore the changing trend of important metabolites in CRC cancer staging, we used STEM software to analyze the trend of samples. As shown in Figure 3, we clustered seven regulatory trends in the three tissues.
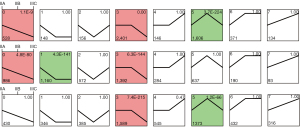
Profile 0 exhibited a significant decrease in metabolites as the degree of deterioration deepened (IIA to IIB to IIIC stage). The metabolites of this trend in tumor tissues were mainly enriched in fatty acid biosynthesis and galactose metabolism. Among them, 33 metabolites were dysregulated in these three tissues including N-Acetyl-leucyl-leucine, 1-methylpyrrolinium, alprenolol, epristeride, and glycocholic-acid (Figure 4A).
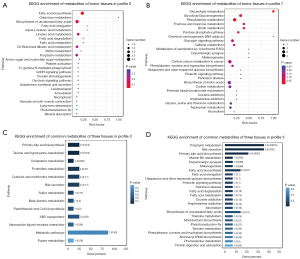
Profile 7 showed a persistent increase in metabolites with cancer advanced, which may contain key metabolites for tumor progression. A total of 316 metabolites in tumor cells were mainly concentrated in glycerolipid metabolism, phenylalanine metabolism, glycolysis/gluconeogenesis, as well as fructose and mannose metabolism. However, in this trend, there were fewer metabolites in all three tissues, the enrichment was not significant, and there were no common metabolites (Figure 4B).
In addition, profile 3 was also a notable mode, in which the metabolites were significantly enriched. Their common metabolites were enriched in primary bile acid biosynthesis, taurine and hypotaurine metabolism, and cholesterol metabolism (Figure 4C). Profile 5 was also markedly enriched in normal and tumor tissues and the common metabolites in the three tissues were mainly enriched in porphyrin metabolism (P<0.001), bile secretion, primary bile acid biosynthesis, and vitamin B6 metabolism (Figure 4D).
Construction of a weighted gene co-expression network
It is well known that different components of a given metabolic pathway synergistically regulate the occurrence and development of CRC in different tissues, and age and staging are also important influencing factors affecting metabolic regulation. Thus, WGCNA was used to identify the metabolic modules of CRC. As shown in Figure 5A,5B, this analysis clustered the 5,834 metabolites into 15 different modules, of which four modules (MEbrown, MEblue; marked by a red five-pointed star) were significantly correlated with tissue specificity. The MEbrown (r=0.49, P=0.01) was positively correlated with tumor tissues that were mainly involved in sulfur metabolism, choline metabolism, primary bile acid biosynthesis, taurine and hypotaurine metabolism, glycerophospholipid metabolism, fatty acid metabolism, and other pathways, and was especially enriched in glycerophospholipids, fatty acyl groups, including metabolites such as 1-oleoylglycerophosphocholine (Figure 6). Similar to the MEbrown, MEblue was negatively correlated to the tumor tissues and was mainly involved in pyrimidine metabolism and diabetic cardiomyopathy.
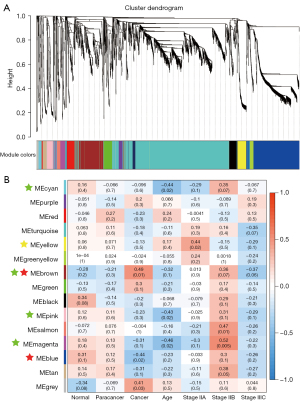
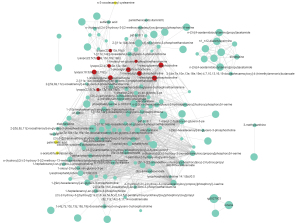
Notably, metabolites in the MEyellow module (marked with a yellow five-pointed star) were enriched in the early cancer stage and decreased with disease progression. The metabolites of this module were mainly enriched in insulin resistance and fatty acid biosynthetic pathways, including organoheterocyclic compounds and lipid-like molecules, especially fatty acyl groups in abundance.
It is noteworthy that the age indicator had a strong negative correlation with stage IIB, which was especially evident in four modules including MEcyan, MEbrown, MEpink, and MEmagenta (marked by a green five-pointed star). Therefore, we speculated that the OPLS_DA results (shown in Figure 2A) of the specificity of stage IIB might be related to age. These metabolites were significantly enriched in sulfur metabolism, diabetic cardiomyopathy, and adrenergic signaling in cardiomyocytes, and also contained large amounts of lipids and lipid-like molecules (fatty acyl and glycerophospholipids).
Collectively, the analysis of CRC metabolites from different tissues or stages involved a large number of glycerophospholipids and fatty acyl-related metabolites, which might be key metabolites for the occurrence and development of CRC. In addition, the high correlation between stage IIB and age implies that age is an important factor affecting cancer progression.
Discussion
Numerous reports have demonstrated that there are many metabolic material changes during the development of CRC, including potential markers, etc., which are not only related to tissue location but also significantly related to tumor progression (23-25). As shown in Figure 1, cluster analysis of the metabolites showed notable differences in the different cancer stages, which implied that there are significant metabolite changes in the cancer process. Further analysis of these differential metabolites showed that several metabolites were dysregulated in cancer stage IIB, including hydroxyquinoline, phosphate, fatty acyl, as well as steroids and steroid derivatives (Figure 2 and Figure S3), which is consistent with previous studies (26,27). Cholesterol, as an important metabolite of tumor development, has been widely recognized (28,29). Garcia-Etxebarria et al. also highlighted the importance of cholesterol in adenoma and CRC risk (30) and showed that cholesterol products were markedly correlated to tumor development. It was also found that the gene expression related to the cholesterol metabolism pathway was dysregulated in CRC, which further illustrated the important cholesterol metabolite landmarks in CRC (31-33).
To further clarify the landmark metabolites in CRC development, we carried out a trend analysis of different cancer stages and predicted seven different significant modules. We found that fatty acid biosynthesis and galactose metabolism decreased substantially in cancer tissues (Figures 3,4A), and 33 metabolites were found in all three tissues, including n-acetylleucine, 1-methylpyrroline, aprenol, eplate, glycocholic acid, indicating that these metabolites may participate in basic cell growth, while the former two may be closely related to cancer development. Studies have reported that the accumulation of free fatty acids is a typical metabolite accumulation of cancer proliferation (34,35). Due to the increased proliferation and oxidative stress of cancer cells, numerous ROS (reactive oxygen species) will be produced in cancer, which may lead to cell death. Therefore, cancer cells will enhance their antioxidant and osmoregulation capabilities by synthesizing organic osmoregulants and enriching galactose metabolites (36).
Similarly, in Profile 3, the metabolites (including primary bile acid biosynthesis, taurine and hypotaurine metabolism, and cholesterol metabolism), which have been reported to have a significant correlation with the development of cancer, were significantly enriched (Figure 4C). For example, bile acids can change the intestinal microenvironment and promote the new biotransformation of bile acids by intestinal bacteria, resulting in complex interactions between a variety of bile acid compounds and CRC development. It has also been found that bilirubin may be negatively correlated with the risk of CRC (37-39). On the other hand, it could lead to the destruction of the stability of intestinal microorganisms, which has been shown to have a direct effect on the development of CRC (40).
Network analysis of all differential metabolites was carried out to explore the differences between different metabolites in cancer tissues and stages. The results showed that glycosphingolipids and fatty acyl groups, such as 1-oleoylglycerophosphate choline, exhibited a significant tissue difference (Figure 6). Through blood metabolite analysis in 25 CRC patients, Shu et al. observed that lipid metabolites were significantly related to the cancer risk, and glycerol phospholipids were significantly representative, suggesting that the imbalance of glycerol phospholipids may be a direct cause of CRC risk (20). Moreover, these differential metabolites could be the candidate targets for the treatment of CRC.
Moreover, the metabolites also exhibit marked differences in the various cancer stages, in which fatty acids, organic heterocyclic compounds, and lipid molecules are significantly related, indicating that these molecules might be biomarkers of cancer development (41,42) and prognostic markers for CRC. While the exact role of these related molecules in the tumor progression and tumor prognosis needs further research. For example, Quan et al. reported that members in long-chain acyl-coenzyme A synthases (ACSLs) family which are responsible for activating long-chain fatty acid can act as tumor suppressors or oncogenes, indicating the potential biomarkers and therapeutic targets for cancer treatment (43). Previous studies have reported that fatty acid changes could occur in CRC tumor tissues (44,45). Mika et al. showed that CRC tissues express more polyunsaturated fatty acids than normal colorectal mucosa; however, the levels are lower in the serum of CRC patients than in healthy controls. Furthermore, cell culture experiments have shown that cancer cells can preferentially ingest these polyunsaturated fatty acids, and thus play an important role in the formation of cell membrane phospholipids during the rapid proliferation of cancer cells (42). Similarly, in our study, significant changes in fatty acids and glycerophospholipids were also found in stage IIB of CRC, illustrating the representativeness of the molecules during CRC occurrence and implying that these metabolites play important roles in the development of CRC. Moreover, abnormal fatty acid metabolic pathways were reported to drive tumor development and progression, correlating with a poor prognosis in CRC patients.
In summary, our results suggested that fatty acids and glycerophospholipids were significantly related to the development of CRC. Thus, future studies focused on the role of fatty acids and glycerophospholipids in CRC could be deeply investigated. Gut metabolome, such as lipid metabolism, is influenced by intestinal microbiota (46,47). Furthermore, the abnormal metabolic pathways, the tumor microenvironment and the intestinal microbiota always interact with each other, contributing to the CRC progression and tightly related to the therapy effectiveness. Based on this, probiotics and bacteriophages may be developed to regulate intestinal microorganisms to prevent or treat CRC.
Acknowledgments
Funding: This work was supported by the National Natural Science Foundation of China (No. 42006093), the Medical Health Science and Technology project of Ningbo (No. 2021Y31), the Natural Science Foundation of Ningbo (No. 2021J323), the Research Initiation Project of Ningbo Institute of Life and Health Industry, Chinese Academy of Sciences (Nos. 2021YJY1010 and 2022YJY0204), the Ningbo Medical Key Discipline (No. 2022-B11), the Public Welfare Foundation of Ningbo (No. 2021S10), and the Medical Science and Technology Project of Zhejiang Province (No. 2021KY338).
Footnote
Reporting Checklist: The authors have completed the STREGA reporting checklist. Available at https://atm.amegroups.com/article/view/10.21037/atm-22-4767/rc
Data Sharing Statement: Available at https://atm.amegroups.com/article/view/10.21037/atm-22-4767/dss
Conflicts of Interest: All authors have completed the ICMJE uniform disclosure form (available at https://atm.amegroups.com/article/view/10.21037/atm-22-4767/coif). The authors have no conflicts of interest to declare.
Ethical Statement: The authors are accountable for all aspects of the work in ensuring that questions related to the accuracy or integrity of any part of the work are appropriately investigated and resolved. The study was conducted in accordance with the Declaration of Helsinki (as revised in 2013). The study was approved by the ethics committee of Hwa Mei Hospital, the University of Chinese Academy of Sciences (No. SL-NBYJK-KY-2022-120-01), and informed consent was obtained from all of the individual participants.
Open Access Statement: This is an Open Access article distributed in accordance with the Creative Commons Attribution-NonCommercial-NoDerivs 4.0 International License (CC BY-NC-ND 4.0), which permits the non-commercial replication and distribution of the article with the strict proviso that no changes or edits are made and the original work is properly cited (including links to both the formal publication through the relevant DOI and the license). See: https://creativecommons.org/licenses/by-nc-nd/4.0/.
References
- Siegel RL, Miller KD, Fuchs HE, et al. Cancer Statistics, 2021. CA Cancer J Clin 2021;71:7-33. [Crossref] [PubMed]
- Sung H, Ferlay J, Siegel RL, et al. Global Cancer Statistics 2020: GLOBOCAN Estimates of Incidence and Mortality Worldwide for 36 Cancers in 185 Countries. CA Cancer J Clin 2021;71:209-49. [Crossref] [PubMed]
- Argilés G, Tabernero J, Labianca R, et al. Localised colon cancer: ESMO Clinical Practice Guidelines for diagnosis, treatment and follow-up. Ann Oncol 2020;31:1291-305. [Crossref] [PubMed]
- Kuzma J, Chmelař D, Hájek M, et al. The role of intestinal microbiota in the pathogenesis of colorectal carcinoma. Folia Microbiol (Praha) 2020;65:17-24. [Crossref] [PubMed]
- Ghafouri-Fard S, Hussen BM, Badrlou E, et al. MicroRNAs as important contributors in the pathogenesis of colorectal cancer. Biomed Pharmacother 2021;140:111759. [Crossref] [PubMed]
- Wu Z, Li Y, Zhang Y, et al. Colorectal Cancer Screening Methods and Molecular Markers for Early Detection. Technol Cancer Res Treat 2020;19:1533033820980426. [Crossref] [PubMed]
- Simon K. Colorectal cancer development and advances in screening. Clin Interv Aging 2016;11:967-76. [Crossref] [PubMed]
- Zygulska AL, Pierzchalski P. Novel Diagnostic Biomarkers in Colorectal Cancer. Int J Mol Sci 2022;23:852. [Crossref] [PubMed]
- Park JH, Pyun WY, Park HW. Cancer Metabolism: Phenotype, Signaling and Therapeutic Targets. Cells 2020;9:2308. [Crossref] [PubMed]
- Clish CB. Metabolomics: an emerging but powerful tool for precision medicine. Cold Spring Harb Mol Case Stud 2015;1:a000588. [Crossref] [PubMed]
- Klassen A, Faccio AT, Canuto GA, et al. Metabolomics: Definitions and Significance in Systems Biology. Adv Exp Med Biol 2017;965:3-17. [Crossref] [PubMed]
- Couvillion SP, Agrawal N, Colby SM, et al. Who Is Metabolizing What? Discovering Novel Biomolecules in the Microbiome and the Organisms Who Make Them. Front Cell Infect Microbiol 2020;10:388. [Crossref] [PubMed]
- Berg G, Rybakova D, Fischer D, et al. Microbiome definition re-visited: old concepts and new challenges. Microbiome 2020;8:103. [Crossref] [PubMed]
- Stine ZE, Schug ZT, Salvino JM, et al. Targeting cancer metabolism in the era of precision oncology. Nat Rev Drug Discov 2022;21:141-62. [Crossref] [PubMed]
- Farhadi P, Yarani R, Dokaneheifard S, et al. The emerging role of targeting cancer metabolism for cancer therapy. Tumour Biol 2020;42:1010428320965284. [Crossref] [PubMed]
- Tounta V, Liu Y, Cheyne A, et al. Metabolomics in infectious diseases and drug discovery. Mol Omics 2021;17:376-93. [Crossref] [PubMed]
- Kumar A, Misra BB. Challenges and Opportunities in Cancer Metabolomics. Proteomics 2019;19:e1900042. [Crossref] [PubMed]
- Zhang F, Zhang Y, Zhao W, et al. Metabolomics for biomarker discovery in the diagnosis, prognosis, survival and recurrence of colorectal cancer: a systematic review. Oncotarget 2017;8:35460-72. [Crossref] [PubMed]
- Liu X, Cheng Y, Shao L, et al. Alterations of the Predominant Fecal Microbiota and Disruption of the Gut Mucosal Barrier in Patients with Early-Stage Colorectal Cancer. Biomed Res Int 2020;2020:2948282. [Crossref] [PubMed]
- Shu X, Xiang YB, Rothman N, et al. Prospective study of blood metabolites associated with colorectal cancer risk. Int J Cancer 2018;143:527-34. [Crossref] [PubMed]
- Zhang C, Zhou S, Chang H, et al. Metabolomic Profiling Identified Serum Metabolite Biomarkers and Related Metabolic Pathways of Colorectal Cancer. Dis Markers 2021;2021:6858809. [Crossref] [PubMed]
- Fleming CA, Mohan HM, O'Leary DP, et al. Metabolomic Pathway Activity with Genomic Single-Nucleotide Polymorphisms Associated with Colorectal Cancer Recurrence and 5-Year Overall Survival. J Gastrointest Cancer 2022; Epub ahead of print. [Crossref] [PubMed]
- Guo J, Pan Y, Chen J, et al. Serum metabolite signatures in normal individuals and patients with colorectal adenoma or colorectal cancer using UPLC-MS/MS method. J Proteomics 2022;270:104741. [Crossref] [PubMed]
- Chattopadhyay I, Dhar R, Pethusamy K, et al. Exploring the Role of Gut Microbiome in Colon Cancer. Appl Biochem Biotechnol 2021;193:1780-99. [Crossref] [PubMed]
- La Vecchia S, Sebastián C. Metabolic pathways regulating colorectal cancer initiation and progression. Semin Cell Dev Biol 2020;98:63-70. [Crossref] [PubMed]
- Cornish AJ, Law PJ, Timofeeva M, et al. Modifiable pathways for colorectal cancer: a mendelian randomisation analysis. Lancet Gastroenterol Hepatol 2020;5:55-62. [Crossref] [PubMed]
- Passarelli MN, Newcomb PA, Makar KW, et al. Blood lipids and colorectal polyps: testing an etiologic hypothesis using phenotypic measurements and Mendelian randomization. Cancer Causes Control 2015;26:467-73. [Crossref] [PubMed]
- Dashti SG, Li WY, Buchanan DD, et al. Type 2 diabetes mellitus, blood cholesterol, triglyceride and colorectal cancer risk in Lynch syndrome. Br J Cancer 2019;121:869-76. [Crossref] [PubMed]
- Zhang KL, Zhu WW, Wang SH, et al. Organ-specific cholesterol metabolic aberration fuels liver metastasis of colorectal cancer. Theranostics 2021;11:6560-72. [Crossref] [PubMed]
- Garcia-Etxebarria K, Clos-Garcia M, Telleria O, et al. Interplay between Genome, Metabolome and Microbiome in Colorectal Cancer. Cancers (Basel) 2021;13:6216. [Crossref] [PubMed]
- Han KT, Kim S. Do cholesterol levels and continuity of statin use affect colorectal cancer incidence in older adults under 75 years of age? PLoS One 2021;16:e0250716. [Crossref] [PubMed]
- Bull CJ, Bell JA, Murphy N, et al. Adiposity, metabolites, and colorectal cancer risk: Mendelian randomization study. BMC Med 2020;18:396. [Crossref] [PubMed]
- Jun SY, Brown AJ, Chua NK, et al. Reduction of Squalene Epoxidase by Cholesterol Accumulation Accelerates Colorectal Cancer Progression and Metastasis. Gastroenterology 2021;160:1194-1207.e28. [Crossref] [PubMed]
- Nomura DK, Long JZ, Niessen S, et al. Monoacylglycerol lipase regulates a fatty acid network that promotes cancer pathogenesis. Cell 2010;140:49-61. [Crossref] [PubMed]
- Lien EC, Westermark AM, Zhang Y, et al. Low glycaemic diets alter lipid metabolism to influence tumour growth. Nature 2021;599:302-7. [Crossref] [PubMed]
- Tsun ZY, Possemato R. Amino acid management in cancer. Semin Cell Dev Biol 2015;43:22-32. [Crossref] [PubMed]
- Kuhls S, Osswald A, Ocvirk S. Bile acids, bile pigments and colorectal cancer risk. Curr Opin Gastroenterol 2022;38:173-8. [Crossref] [PubMed]
- Ocvirk S, O'Keefe SJD. Dietary fat, bile acid metabolism and colorectal cancer. Semin Cancer Biol 2021;73:347-55. [Crossref] [PubMed]
- Roberts DC, Chidambaram S, Kinross JM. The role of the colonic microbiota and bile acids in colorectal cancer. Curr Opin Gastroenterol 2022;38:179-88. [Crossref] [PubMed]
- Zeng H, Umar S, Rust B, et al. Secondary Bile Acids and Short Chain Fatty Acids in the Colon: A Focus on Colonic Microbiome, Cell Proliferation, Inflammation, and Cancer. Int J Mol Sci 2019;20:1214. [Crossref] [PubMed]
- Chen L, Chen H, Li Y, et al. Endocannabinoid and ceramide levels are altered in patients with colorectal cancer. Oncol Rep 2015;34:447-54. [Crossref] [PubMed]
- Mika A, Kobiela J, Pakiet A, et al. Preferential uptake of polyunsaturated fatty acids by colorectal cancer cells. Sci Rep 2020;10:1954. [Crossref] [PubMed]
- Quan J, Bode AM, Luo X. ACSL family: The regulatory mechanisms and therapeutic implications in cancer. Eur J Pharmacol 2021;909:174397. [Crossref] [PubMed]
- Del Cornò M, Baldassarre A, Calura E, et al. Transcriptome Profiles of Human Visceral Adipocytes in Obesity and Colorectal Cancer Unravel the Effects of Body Mass Index and Polyunsaturated Fatty Acids on Genes and Biological Processes Related to Tumorigenesis. Front Immunol 2019;10:265. [Crossref] [PubMed]
- Tutino V, De Nunzio V, Caruso MG, et al. Elevated AA/EPA Ratio Represents an Inflammatory Biomarker in Tumor Tissue of Metastatic Colorectal Cancer Patients. Int J Mol Sci 2019;20:2050. [Crossref] [PubMed]
- Liebisch G, Plagge J, Horing M, et al. The effect of gut microbiota on the intestinal lipidome of mice. Int J Med Microbiol 2021;311:151488. [Crossref] [PubMed]
- Cai J, Sun L, Gonzalez FJ. Gut microbiota-derived bile acids in intestinal immunity, inflammation, and tumorigenesis. Cell Host Microbe 2022;30:289-300. [Crossref] [PubMed]
(English Language Editor: A. Kassem)