Construction of a multiple-class classifier based on mRNAs and lncRNA FAM66A and PSORS1C3 for predicting distant metastasis in lung adenocarcinoma
Introduction
Lung adenocarcinoma (LUAD) accounts for about 40% of all lung cancer types, and is one of the most aggressive and fatal tumor types (1). Progress has been made in developing gene expression patterns that share some molecular characteristics (2,3). Some gene alterations in LUAD could be associated with resistance to drug therapy, and could be used as therapeutic target to LUAD with characterized gene alteration (4,5). For example, the Kirsten rat sarcoma viral oncogene homolog gene (KRAS) mutant, the anaplastic lymphoma kinase gene (ALK) positive, and the epidermal growth factor receptor (EGFR) gene have been found to be related to distinctive clinicopathological features and specific immunohistochemical loss (6,7). There has been a lot of research in identifying subgroups of LUAD and the discovery the distinct biology and therapeutic vulnerabilities of LUAD (8).
Some studies have found that the expression profiles of non-coding genes are associated with frequent lymph nodal metastasis and highly invasive outcomes in LUAD (9,10). Long non-coding RNA (lncRNA) gene expression plays an important role in the early stages of pre-invasive LUAD (11). Some studies screening and evaluation of hub genes for predicting distant metastasis in lung adenocarcinoma, others suggested that some lncRNAs involved in specific signaling pathways could regulate the development of LUAD (12,13). In addition, lncRNAs have been found to reinforce migration and the invasion of LUAD cells by directly binding to the related factors or increasing oxidative stress (14,15). It has also been reported that lncRNAs are expressed differently in LUAD and could be potentially therapeutic and prognostic targets (16,17). Therefore, further research needs to be aimed at the selection of lncRNAs with high useable targets. Future studies in cancer research also needs to include the classification of subtypes with different clinical outcomes (18), particularly studies on distant metastasis in LUAD.
Using the bioinformatics method, genome analysis for distinguishing pathological states in LUAD is a current developing trend (19-21). Biomedical research has led to demands for tools and strategies so that information can be obtained from genome-wide expression data. Several studies have used statistical methods to analyze clinical data to find differences and correlations between LUAD and characteristic genes (22-24). Furthermore, classification systems are built through multiple machine learning and computational procedures (25). Classification systems allow us to identify the query samples’ type/category and search for the separation between various disease subtypes, as well as distinguish pathological states in disease. In machine learning, multiclass classification, often known as multinomial classification, refers to the challenge of classifying events into one of three or more classes (classifying instances into one of two classes is called binary classification) (26). Many studies have focused on specifically alterative genes and their gene spaces in each disease subtype (27,28). Gene space is divided into regions that includes genes associated with specific pathological states because one or several biological processes could be affected and altered by a disease (26). In this theoretical scenario, genes that are affected by a given disease can overlap with the ones affected by a similar pathological state. Therefore, we can differentiate genes that can be altered in multiple pathologies with genes that are only affected by a specific malignancy when compared with other diseases. Cutting-edge bioinformatic approach for the classification of distant metastasis in LUAD is needed; therefore, the purpose of the present study was to construct a multiple-class classifier for predicting distant metastasis in LUAD.
We implemented a method to identify RNAs in LUAD. Samples in the LUAD dataset were collected from The Cancer Genome Atlas (TCGA). We then ranked gene sets (or gene signatures) by their posterior probability in each sample with distant metastasis. Feature ranking was based on the parametric empirical Bayes method (29). We then integrated several existing machine learning and statistical methods to build the multiclass classification model. The machine learning method was implemented iteratively by adding genes in order of discriminant powers. Double-nested internal cross-validation was used for the feature selection process and to estimate the generalization error of the classifier. Gene networks associated to each subtype were based on ranked gene sets and co-expression (30). Finally, the hub genes in the network were identified as key genes. We present the following article in accordance with the STREGA reporting checklist (available at https://atm.amegroups.com/article/view/10.21037/atm-22-4651/rc).
Methods
RNA sequencing (RNA-Seq) dataset
The RNA-Seq dataset was downloaded from TCGA (http://tcga-data.nci.nih.gov). Of the available samples in TCGA, we divided 576 samples into subtypes as follows: (I) normal tissue; (II) LUAD patients with distant metastasis; and (III) LUAD patients without metastasis (non-metastasis). We collected the preprocessed RNA-Seq expression data matrices, including the RNA-Seq by expectation-maximization, from TCGA and used log2 for further analysis. The study was conducted in accordance with the Declaration of Helsinki (as revised in 2013).
Statistical methods and algorithm procedures
Gene ranking
An expectation-maximization algorithm was used to compare differential expression patterns across multiple conditions and to provide posterior probability. This algorithm was used for the gene expression mixture models. The posterior probability was calculated for each gene-class pair with the One-versus-Rest (OvR) method to compare samples of 1 class versus all the other samples. How much each gene differentiates a class from the other classes (1 being the best value, and 0 the worst) is represented by the posterior probability in this method. Genes were arranged in order of decreased posterior probability for each class to build the gene ranking. The value of the difference between the signal expression mean for each gene in the given class and the mean in the closest class was used to resolve ties in the algorithm. Then the ranking procedure assigned each gene to the class in which it has the best ranking Due to this process, even if a gene is discovered to associate with several classes during the expression analysis, it will only be on the ranking of its best class. Before building the ranking, this process filters out genes that do not show any significant difference between classes. The default threshold of the posterior probability was set at >0.95.
Classifier
Support vector machine (SVM) is a multiclass classifier available in R package, included in the algorithm. Using the OvR approach, SVM enables linear kernel implementation that allows the classification of multiple classes, in which all the binary classifications are fitted and the correct class is built on a voting system. The classifier was implemented using geNetClassifier package in R 4.0.2 on the Genelibs platform (www.genelibs.com).
Gene selection
Gene selection was completed through a wrapper forward selection scheme based on 8-fold cross-validation. Each cross-validation iteration started with genes in each class that ranked first, which trains a temporary internal classifier with these genes and evaluates its performance. At each step, an additional gene is added to those classes for which a “perfect prediction” is achieved; that is, the genes are arranged in order from the gene ranking of each class until no errors are reached, or the maximum number of genes allowed is reached. It selects the minimum number of genes per class, which produced the classifier with minimum error when finishing the cross-validation loop. Cross-validation was repeatedly run with new samples many times to ensure the best stability in the number of selected genes. In each of these iterations, the minor number of genes with the smallest error is selected. The genes selected in each of the iterations are the basis for the final selection. The highest number of genes selected in the cross-validation iterations were used to select the top ranked genes for each class, and possible outlier numbers were excluded.
Discriminant power
Discriminant power is a parameter calculated based on the Lagrange coefficients (alpha) of the support vectors for all the genes selected for the classification. The multiclass SVM algorithm produces a set of support vectors for each binary comparison between classes because it is an OvR implementation. Adding up the Lagrange coefficients of all the support vectors for each class gives a value per class for each gene. The difference between the largest value and the closest one is regarded as the discriminant power.
Gene network
Cellular machinery is based on functional interactions between genes in cells and their corresponding protein. Therefore, the proteins’ connectivity network needs to be considered to fully understand the clinical characteristics of LUAD. The gene network was built by accessing the gene co-expression network and gene-gene interaction database, STRING (https://string-db.org/). Gene co-expression was identified by Pearson correlation, with a threshold of 0.8. The STRING database (version 11) includes experimental datasets that are used to find protein-protein association networks and support functional discovery in whole genomes. The network was visualized using Cytoscape 3.7.2 (https://cytoscape.org/).
Results
Dataset
This study was based on the RNA-seq from TCGA and included 525 LUAD cases, with the aim of classifying the 3 subtypes of LUAD according to the metastasis of the patients. We first ranked genes by identification of differentially expressed genes for each subtype (distant metastasis, non-metastasis, and normal tissue; information for criteria used to determine subtypes are described in the Methods). We carried out a differential expression analysis using an empirical Bayes approach with an OvR strategy (31). The analysis enabled us to quantify the size of the gene signature assigned to each subtype (compared with the other subtypes in this study) and to compare the biological or pathological conditions represented in the samples specifically. The set of genes considered significant for each of the classes was determined by a common threshold for the posterior probability (threshold =0.95, P=0.05).
Compared with the adjacent normal tissue, LUAD tissue showed a large amount of differentially expressed genes. Further analysis of the 3 subtypes found that each subtype had a corresponding characteristic gene and ranked gene lists. The subtype of normal tissue was assigned 8,083 genes, distant metastasis was assigned 329 genes, while non-metastasis was assigned 397 genes (Figure 1).
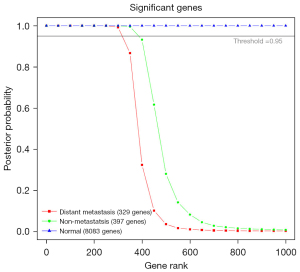
Multiclass classification model
For performing feature selection of our classification model, we further selected subset of genes, which is good enough to do the classification of the subtypes (15). Several internal SVM classifiers were trained iteratively. The iteration started with 1 gene for each subtype with the highest posterior probability. Then, genes with top-ranked posterior probability lists were gradually added to the set of genes (model was trained with increasing number of genes).
For each iteration, we built the classifier using C-classification type and a linear SVM-kernel, and evaluated the model using double-nested cross-validation. In the new classification model, classifiers with the optimum number of genes were evaluated using 8-fold cross-validation. This iteration procedure was used until any minimal errors were found. The minimal number of genes that provides the best performance was selected as feature set, and used to train and build a final classifier.
For the first classifier, which aimed to classify normal and cancer tissues in LUAD (normal/cancer classification), we trained the model with all 576 samples and selected 97 genes (Figure 2A). In the second classifier, which aimed to classify normal, distant metastasis, and non-metastasis tissues (metastasis/non-metastasis/normal classification), we trained the model with 256 samples and selected 282 genes (distant metastasis, non-metastasis, normal tissues all have similar gene number of 94, as shown in Figure 2B,2C).
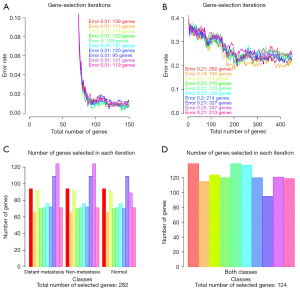
To achieve the best stability in the gene selection procedure, gene selections and cross-validations were carried out 10 times with different samples. In each of these repeats, the minor number of genes that provided the smallest error was selected. Figure 2C,2D shows the total number of genes explored in each iteration. The total number of selected genes in each iteration ranged from around 150 to 70.
Evaluation of genes in each subtype
To search the enriched pathways in those genes that provided the smallest error, we analyzed the gene list and carried out enrichment analysis by using an over-representation approach for each subtype. As shown in Figure 3, the PPAR signaling pathway was significantly enriched in the distant metastasis subtype, and the neuroactive ligand-receptor interaction pathway was top enriched in the non-metastasis pathway, while nitrogen metabolism was enriched in normal tissue. Those pathways indicated the specific pathway features for each subtype.
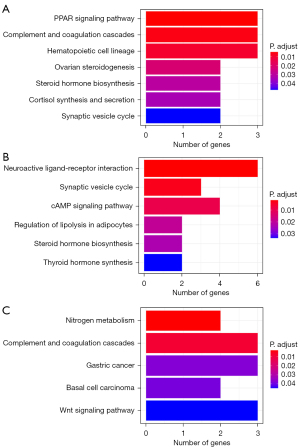
We also introduced a discriminant power that was calculated based on the Lagrange coefficients of all the support vectors in the final classification model so that we could calculate the contribution of each gene to determine the subtypes in our model. The top discriminant power in distant metastasis was cytochrome P450 family 4 subfamily F member 12 (CYP4F12), the top discriminant power in non-metastasis was down syndrome cell adhesion molecule (DSCAM), while the top ranked gene in normal tissue was solute carrier family 6 member 4 (SLC6A4) (Figure 4).
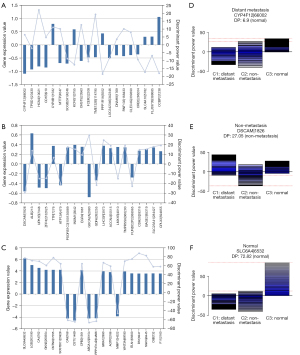
Among those genes, lncRNAs were found. In distant metastasis class, we found lncRNA family with sequence similarity 66 member A (FAM66A). In non-metastasis class, we found lncRNA PSORS1C3 (Figure 5).
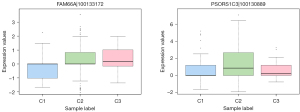
Gene co-expression networks associated with each subtype
We also identified some key genes by accessing the co-expression networks in each subtype. Co-expression networks were built for searching the mechanism of our classification model. To search the connections in those genes, we analyzed the gene network of each subtype gene, and the results are shown in Figure 6. The connection was calculated based on the backbone STRING database and co-expression correlation. The network shows a small world network to analyze the relationship among proteins. The 2 subnetworks with 3 vertices in distant metastasis were related to GPLD1 and CXCL6, respectively. No featured network cluster was found in the non-metastasis class, while genes in normal tissue class clustered into SLC6A4- and LRRC18-related subnetworks.
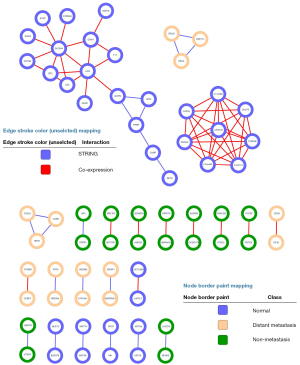
Discussion
Cancer deaths around the world are mainly from non-small cell lung cancer (NSCLC), and histopathological assessment is involved in its diagnosis (32,33). Experienced pathologists mainly evaluate the stage, type, and subtype of lung cancer by visual inspection of histopathological slides (34,35). Pathology and molecular biomarkers for distant metastasis or other clinical cancer subtypes have recently become a focus of research. Coudray et al. have constructed deep-learning models to classify and predict mutation from NSCLC by using a deep convolutional neural network (CNN) (36). Wei et al. also used a CNN to classify NSCLC histopathology types and transcriptomic subtypes (37), and Le Page et al. used CNN to classify NSCLC based on diagnostic histopathology HES images (38). Smedley et al. used deep neural networks and interpretability methods to identify gene expression patterns that predict radiomic features and histology in NSCLC (39).
Several genes have been linked to the development of cancer. In this study, for example, CYP4F12 was discovered to be the top gene in the ranking of distant metastasis cases. Similarly, a recent study found that increased CYP4F12 expression in hepatocellular carcinoma is inversely connected with decreased expression of cell cycle-associated genes (40). Based on data from DMET Console software®, CYP4F12 is found to associate with colorectal cancer (41).
It is reported that DSCAM plays key roles in regulating estrogen receptor, mediating tumor progression and affecting tamoxifen resistance. Meantime, breast cancer patients with high expression of DSCAM can obtain better outcome of treatment, suggesting that DSCAM may be a prognosis biomarker of breast cancer (42,43). It has reported that netrin-1 in primary cortical neurons stimulates the interaction between DSCAM and uncoordinated-5C (44). According to a study, DSCAM regulates the miR-577/HMGB1 axis in the pathogenesis of NSCLC, providing a promising therapeutic target for NSCLC (45). A recent publication reported that the expression of DSCAM is related to neuronal self-avoidance, which promotes oligodendrocyte differentiation via the neuroactive ligand-receptor interaction signaling pathway (46).
Our findings indicated that SLC6A4 is associated with normal tissues. SLC6A4 as a protein coding gene participates in the action of serotonin and recycles via the encoded protein. The 5-hydroxytryptamine transporter (5-HTT) protein encoded by SLC6A4 has also been implicated in inflammation, and SLC6A4 variations could be associated with poor survival in colorectal cancer patients (47). Pathways associated with SLC6A4 include monoamine transport, the selective serotonin reuptake inhibitor pathway, and pharmacodynamics. Warchal et al. have demonstrated the selective activity of serotonin receptor modulators upon growth and survival of breast cancer cells, which suggested SLC6A4 for the diagnosis and treatment in breast cancer patients (48). Several studies have demonstrated that gene polymorphisms could play vital roles in the occurrence of lung cancer. SLC6A4 gene polymorphisms and 5-HTT are possibly associated with the occurrence of pain triggered by lung cancer and could influence the chemotherapeutic sensitivity of lung cancer cells, which suggests a new potential therapeutic target for lung cancer cells (49-51). SLC6A4 directly regulated the following 8 genes in our study: SYN2, ST8SIA6, SGCG, CNTN6, RS1, CA4, LGI3, and GRIA1. LGI3 functioned as a multifunctional cytokine and could increase the expression of inflammatory proteins, including tumor necrosis factor-α in macrophages (52). SLC6A4 has been shown to mediate the roles of the competitive endogenous RNA network. This RNA network has demonstrated that SLC6A4 is associated with tumor growth by activating the downstream complement and coagulation cascades pathway (53,54).
In addition, lncRNAs play a key role in cellular processes, and new evidence presents an opportunity for large-scale identification of lncRNA genes critical to lung cancer progression (10,55). LncRNAs have multiple biological roles, such as regulating gene expression and interacting with epigenetic factors. Recent studies have demonstrated the essential role of some lncRNAs in different pathologies, including cancer, and indicated that lncRNAs are valuable clinical biomarkers (56-58). Therefore, we selected the lncRNA genes for our candidates (59).
Some consistencies were observed when we used the algorithm of multiclass classifier (26) to train on a dataset of LUAD 576 samples. We found that lncRNA FAM66A and lncRNA PSORS1C3 were associated with tumor metastasis in LUAD. There is increasing evidence supporting that FAM66A can predict early wake-up-related gene expression in cancer cells (60,61). Some studies have demonstrated that the vitamin D receptor signaling pathway participates in various cancers and is regulated by the overexpression of FAM66A (62). LncRNA PSORS1C3 is located upstream of the Oct4 gene, and Oct4 expression can be regulated by PSORS1C3, which can lead to cell differentiation (63). Compared with the normal samples, lncRNA PSORS1C3 in LUAD had high sensitivity and specificity, indicating its value as a diagnostic biomarker (64).
The lncRNA FAM66A was discovered to be linked to ovarian carcinosarcoma. However, Feng et al. stated in their study that FAM66A is a gene signature that can be used to predict prognosis in small cell lung cancer (SCLC). Moreover, several investigations have discovered that PSORS1C3 can have a role in the advancement of benign lung disease. In our investigation, we discovered for the first time that the lncRNAs FAM66A and PSORS1C3 have a role in distant metastasis in LUAD (65,66).
There are clear limitations in this study. First, the sample size from the TCGA database was relatively small. Given the sample size and the exploratory nature of the study, as well as the available resources, it is impossible to effectively validate the results. Further clinical studies are needed, and an LUAD cell model should be validated in the observation experiment. Second, cytometric bead-array assay and additional histological descriptors were not available (67). Future research should include additional experiments to validate the conclusions. The findings of the present provide a unique insight into the LUAD transcriptional changes that occur after distant metastasis. Moreover, our results described transcription differences of lncRNA FAM66A and PSORS1C3 between normal distant metastasis and non-metastasis patients, which need to be validated in cell models. However, there was considerable variation in that sampling time that warrants further investigation.
Acknowledgments
Funding: None.
Footnote
Reporting Checklist: The authors have completed the STREGA reporting checklist. Available at https://atm.amegroups.com/article/view/10.21037/atm-22-4651/rc
Conflicts of Interest: All authors have completed the ICMJE uniform disclosure form (available at https://atm.amegroups.com/article/view/10.21037/atm-22-4651/coif). The authors have no conflicts of interest to declare.
Ethical Statement: The authors are accountable for all aspects of the work in ensuring that questions related to the accuracy or integrity of any part of the work are appropriately investigated and resolved. The study was conducted in accordance with the Declaration of Helsinki (as revised in 2013).
Open Access Statement: This is an Open Access article distributed in accordance with the Creative Commons Attribution-NonCommercial-NoDerivs 4.0 International License (CC BY-NC-ND 4.0), which permits the non-commercial replication and distribution of the article with the strict proviso that no changes or edits are made and the original work is properly cited (including links to both the formal publication through the relevant DOI and the license). See: https://creativecommons.org/licenses/by-nc-nd/4.0/.
References
- Inamura K. Clinicopathological Characteristics and Mutations Driving Development of Early Lung Adenocarcinoma: Tumor Initiation and Progression. Int J Mol Sci 2018;19:1259. [Crossref] [PubMed]
- Wu Y, Wu P, Xu S, et al. Genome-Wide Identification, Expression Patterns and Sugar Transport of the Physic Nut SWEET Gene Family and a Functional Analysis of JcSWEET16 in Arabidopsis. Int J Mol Sci 2022;23:5391. [Crossref] [PubMed]
- Ma X, Yan X, Ke R, et al. Comparative Transcriptome Sequencing Analysis of Hirudo nipponia in Different Growth Periods. Front Physiol 2022;13:873831. [Crossref] [PubMed]
- Skoulidis F, Goldberg ME, Greenawalt DM, et al. STK11/LKB1 Mutations and PD-1 Inhibitor Resistance in KRAS-Mutant Lung Adenocarcinoma. Cancer Discov 2018;8:822-35. [Crossref] [PubMed]
- Ren Z, Hu M, Wang Z, et al. Ferroptosis-Related Genes in Lung Adenocarcinoma: Prognostic Signature and Immune, Drug Resistance, Mutation Analysis. Front Genet 2021;12:672904. [Crossref] [PubMed]
- Kim H, Yang JM, Jin Y, et al. MicroRNA expression profiles and clinicopathological implications in lung adenocarcinoma according to EGFR, KRAS, and ALK status. Oncotarget 2017;8:8484-98. [Crossref] [PubMed]
- Saifullah Tsukahara T. Integrated analysis of ALK higher expression in human cancer and downregulation in LUAD using RNA molecular scissors. Clin Transl Oncol 2022;24:1785-99. [Crossref] [PubMed]
- Wang H, Zhang W, Wang K, et al. Correlation between EML4-ALK, EGFR and clinicopathological features based on IASLC/ATS/ERS classification of lung adenocarcinoma. Medicine (Baltimore) 2018;97:e11116. [Crossref] [PubMed]
- Li L, Peng M, Xue W, et al. Integrated analysis of dysregulated long non-coding RNAs/microRNAs/mRNAs in metastasis of lung adenocarcinoma. J Transl Med 2018;16:372. [Crossref] [PubMed]
- Yang D, Niu Y, Ni H, et al. Identification of metastasis-related long non-coding RNAs in lung cancer through a novel tumor mesenchymal score. Pathol Res Pract 2022;237:154018. [Crossref] [PubMed]
- Chen H, Carrot-Zhang J, Zhao Y, et al. Genomic and immune profiling of pre-invasive lung adenocarcinoma. Nat Commun 2019;10:5472. [Crossref] [PubMed]
- Chen C, Guo Q, Tang Y, et al. Screening and evaluation of the role of immune genes of brain metastasis in lung adenocarcinoma progression based on the TCGA and GEO databases. J Thorac Dis 2021;13:5016-34. [Crossref] [PubMed]
- Lai XR, Wang CL, Qin FZ. The mechanism of LncRNA01977 in lung adenocarcinoma through the SDF-1/CXCR4 pathway. Transl Cancer Res 2022;11:475-87. [Crossref] [PubMed]
- Moreno Leon L, Gautier M, Allan R, et al. The nuclear hypoxia-regulated NLUCAT1 long non-coding RNA contributes to an aggressive phenotype in lung adenocarcinoma through regulation of oxidative stress. Oncogene 2019;38:7146-65. [Crossref] [PubMed]
- Peng Z, Wang J, Shan B, et al. The long noncoding RNA LINC00312 induces lung adenocarcinoma migration and vasculogenic mimicry through directly binding YBX1. Mol Cancer 2018;17:167. [Crossref] [PubMed]
- Yang Z, Li H, Wang Z, et al. Microarray expression profile of long non-coding RNAs in human lung adenocarcinoma. Thorac Cancer 2018;9:1312-22. [Crossref] [PubMed]
- Wang C, Guo J, Jiang R, et al. Long Non-Coding RNA AP000695.2 Acts as a Novel Prognostic Biomarker and Regulates the Cell Growth and Migration of Lung Adenocarcinoma. Front Mol Biosci 2022;9:895927. [Crossref] [PubMed]
- Szarvas T, Oláh C, Riesz P, et al. Molecular subtype classification of urothelial bladder cancer and its clinical relevance. Orv Hetil 2019;160:1647-54. [Crossref] [PubMed]
- Bueno R, Stawiski EW, Goldstein LD, et al. Comprehensive genomic analysis of malignant pleural mesothelioma identifies recurrent mutations, gene fusions and splicing alterations. Nat Genet 2016;48:407-16. [Crossref] [PubMed]
- Wang X, Xu Z, Liu Z, et al. Characterization of the Immune Cell Infiltration Landscape Uncovers Prognostic and Immunogenic Characteristics in Lung Adenocarcinoma. Front Genet 2022;13:902577. [Crossref] [PubMed]
- Wang H, Wang X, Xu L, et al. Nonnegative matrix factorization-based bioinformatics analysis reveals that TPX2 and SELENBP1 are two predictors of the inner sub-consensuses of lung adenocarcinoma. Cancer Med 2021;10:9058-77. [Crossref] [PubMed]
- Zhang HM, Yang B, Chen HF, et al. Prognosis-related miRNA bioinformatics screening of lung adenocarcinoma and its clinical significance. Zhongguo Ying Yong Sheng Li Xue Za Zhi 2018;34:530-5. [PubMed]
- Zhao C, Xiong K, Adam A, et al. Necroptosis Identifies Novel Molecular Phenotypes and Influences Tumor Immune Microenvironment of Lung Adenocarcinoma. Front Immunol 2022;13:934494. [Crossref] [PubMed]
- Huang D, Tang E, Zhang T, et al. Characteristics of Fatty Acid Metabolism in Lung Adenocarcinoma to Guide Clinical Treatment. Front Immunol 2022;13:916284. [Crossref] [PubMed]
- Glaab E, Bacardit J, Garibaldi JM, et al. Using rule-based machine learning for candidate disease gene prioritization and sample classification of cancer gene expression data. PLoS One 2012;7:e39932. [Crossref] [PubMed]
- Aibar S, Fontanillo C, Droste C, et al. Analyse multiple disease subtypes and build associated gene networks using genome-wide expression profiles. BMC Genomics 2015;16:S3. [Crossref] [PubMed]
- Zhang K, Pirooznia M, Arabnia HR, et al. Genomic signatures and gene networking: challenges and promises. BMC Genomics 2011;12:I1. [Crossref] [PubMed]
- Ghaffari S, Hanson C, Schmidt RE, et al. An integrated multi-omics approach to identify regulatory mechanisms in cancer metastatic processes. Genome Biol 2021;22:19. [Crossref] [PubMed]
- Friston KJ, Litvak V, Oswal A, et al. Bayesian model reduction and empirical Bayes for group (DCM) studies. Neuroimage 2016;128:413-31. [Crossref] [PubMed]
- Szklarczyk D, Gable AL, Lyon D, et al. STRING v11: protein-protein association networks with increased coverage, supporting functional discovery in genome-wide experimental datasets. Nucleic Acids Res 2019;47:D607-13. [Crossref] [PubMed]
- Kendziorski CM, Newton MA, Lan H, et al. On parametric empirical Bayes methods for comparing multiple groups using replicated gene expression profiles. Stat Med 2003;22:3899-914. [Crossref] [PubMed]
- Andrini E, Mosca M, Galvani L, et al. Non-small-cell lung cancer: how to manage RET-positive disease. Drugs Context 2022;11:e2022-1-5.
- Bertolaccini L, Mohamed S, Bardoni C, et al. The Interdisciplinary Management of Lung Cancer in the European Community. J Clin Med 2022;11:4326. [Crossref] [PubMed]
- Inamura K, Yokouchi Y, Kobayashi M, et al. Tumor B7-H3 (CD276) expression and smoking history in relation to lung adenocarcinoma prognosis. Lung Cancer 2017;103:44-51. [Crossref] [PubMed]
- Nooreldeen R, Bach H. Current and Future Development in Lung Cancer Diagnosis. Int J Mol Sci 2021;22:8661. [Crossref] [PubMed]
- Coudray N, Ocampo PS, Sakellaropoulos T, et al. Classification and mutation prediction from non-small cell lung cancer histopathology images using deep learning. Nat Med 2018;24:1559-67. [Crossref] [PubMed]
- Wei JW, Tafe LJ, Linnik YA, et al. Pathologist-level classification of histologic patterns on resected lung adenocarcinoma slides with deep neural networks. Sci Rep 2019;9:3358. [Crossref] [PubMed]
- Le Page AL, Ballot E, Truntzer C, et al. Using a convolutional neural network for classification of squamous and non-squamous non-small cell lung cancer based on diagnostic histopathology HES images. Sci Rep 2021;11:23912. [Crossref] [PubMed]
- Smedley NF, Aberle DR, Hsu W. Using deep neural networks and interpretability methods to identify gene expression patterns that predict radiomic features and histology in non-small cell lung cancer. J Med Imaging (Bellingham) 2021;8:031906. [Crossref] [PubMed]
- Eun HS, Cho SY, Lee BS, et al. Profiling cytochrome P450 family 4 gene expression in human hepatocellular carcinoma. Mol Med Rep 2018;18:4865-76. [Crossref] [PubMed]
- Di Martino MT, Arbitrio M, Leone E, et al. Single nucleotide polymorphisms of ABCC5 and ABCG1 transporter genes correlate to irinotecan-associated gastrointestinal toxicity in colorectal cancer patients: a DMET microarray profiling study. Cancer Biol Ther 2011;12:780-7. [Crossref] [PubMed]
- Niknafs YS, Han S, Ma T, et al. The lncRNA landscape of breast cancer reveals a role for DSCAM-AS1 in breast cancer progression. Nat Commun 2016;7:12791. [Crossref] [PubMed]
- Khorshidi H, Azari I, Oskooei VK, et al. DSCAM-AS1 up-regulation in invasive ductal carcinoma of breast and assessment of its potential as a diagnostic biomarker. Breast Dis 2019;38:25-30. [Crossref] [PubMed]
- Purohit AA, Li W, Qu C, et al. Down syndrome cell adhesion molecule (DSCAM) associates with uncoordinated-5C (UNC5C) in netrin-1-mediated growth cone collapse. J Biol Chem 2012;287:27126-38. [Crossref] [PubMed]
- Qiu Z, Pan XX, You DY. LncRNA DSCAM-AS1 promotes non-small cell lung cancer progression via regulating miR-577/HMGB1 axis. Neoplasma 2020;67:871-9. [Crossref] [PubMed]
- Bernardo A, Giammarco ML, De Nuccio C, et al. Docosahexaenoic acid promotes oligodendrocyte differentiation via PPAR-γ signalling and prevents tumor necrosis factor-α-dependent maturational arrest. Biochim Biophys Acta Mol Cell Biol Lipids 2017;1862:1013-23. [Crossref] [PubMed]
- Savas S, Hyde A, Stuckless SN, et al. Serotonin transporter gene (SLC6A4) variations are associated with poor survival in colorectal cancer patients. PLoS One 2012;7:e38953. [Crossref] [PubMed]
- Warchal SJ, Dawson JC, Shepherd E, et al. High content phenotypic screening identifies serotonin receptor modulators with selective activity upon breast cancer cell cycle and cytokine signaling pathways. Bioorg Med Chem 2020;28:115209. [Crossref] [PubMed]
- Piva F, Giulietti M, Nardi B, et al. An improved in silico selection of phenotype affecting polymorphisms in SLC6A4, HTR1A and HTR2A genes. Hum Psychopharmacol 2010;25:153-61. [Crossref] [PubMed]
- Zhu XL, Han X, Xin XF, et al. Correlations of analgesic dosage of morphine with SLC6A4 gene polymorphisms in patients with lung cancer. Eur Rev Med Pharmacol Sci 2020;24:5046-52. [PubMed]
- Tu Y, Yao S, Chen Q, et al. 5-Hydroxytryptamine activates a 5-HT/c-Myc/SLC6A4 signaling loop in non-small cell lung cancer. Biochim Biophys Acta Gen Subj 2022;1866:130093. [Crossref] [PubMed]
- Kwon NS, Baek KJ, Kim DS, et al. Leucine-rich glioma inactivated 3: Integrative analyses reveal its potential prognostic role in cancer. Mol Med Rep 2018;17:3993-4002. [PubMed]
- Lin ZF, Shen XY, Lu FZ, et al. Reveals new lung adenocarcinoma cancer genes based on gene expression. Eur Rev Med Pharmacol Sci 2012;16:1249-56. [PubMed]
- Zhang Y, Wang H, Wang J, et al. Global analysis of chromosome 1 genes among patients with lung adenocarcinoma, squamous carcinoma, large-cell carcinoma, small-cell carcinoma, or non-cancer. Cancer Metastasis Rev 2015;34:249-64. [Crossref] [PubMed]
- Zhang H, Liu M, Yang Z, et al. Evaluating the utility of an immune checkpoint-related lncRNA signature for identifying the prognosis and immunotherapy response of lung adenocarcinoma. Sci Rep 2022;12:12785. [Crossref] [PubMed]
- Matouk IJ, Halle D, Raveh E, et al. The role of the oncofetal H19 lncRNA in tumor metastasis: orchestrating the EMT-MET decision. Oncotarget 2016;7:3748-65. [Crossref] [PubMed]
- Arenas AM, Cuadros M, Andrades A, et al. LncRNA DLG2-AS1 as a Novel Biomarker in Lung Adenocarcinoma. Cancers (Basel) 2020;12:2080. [Crossref] [PubMed]
- Huang J, Yu Q, Zhang J, et al. LncRNA NBR2 regulates cancer cell stemness and predicts survival in non-small cell cancer patients by downregulating TGF-β1. Curr Pharm Biotechnol 2022; Epub ahead of print. [Crossref] [PubMed]
- Otani T, Noma H, Nishino J, et al. Re-assessment of multiple testing strategies for more efficient genome-wide association studies. Eur J Hum Genet 2018;26:1038-48. [Crossref] [PubMed]
- Li J, Huang T. Predicting and analyzing early wake-up associated gene expressions by integrating GWAS and eQTL studies. Biochim Biophys Acta Mol Basis Dis 2018;1864:2241-6. [Crossref] [PubMed]
- Wu J, Huang H, Huang W, et al. Analysis of exosomal lncRNA, miRNA and mRNA expression profiles and ceRNA network construction in endometriosis. Epigenomics 2020;12:1193-213. [Crossref] [PubMed]
- Kholghi Oskooei V, Ghafouri-Fard S, Omrani Mir D. A Combined Bioinformatics and Literature Based Approach for Identification of Long Non-coding RNAs That Modulate Vitamin D Receptor Signaling in Breast Cancer. Klin Onkol Summer;31:264-9.
- Malakootian M, Mirzadeh Azad F, Naeli P, et al. Novel spliced variants of OCT4, OCT4C and OCT4C1, with distinct expression patterns and functions in pluripotent and tumor cell lines. Eur J Cell Biol 2017;96:347-55. [Crossref] [PubMed]
- Shete S, Liu H, Wang J, et al. A Genome-Wide Association Study Identifies Two Novel Susceptible Regions for Squamous Cell Carcinoma of the Head and Neck. Cancer Res 2020;80:2451-60. [Crossref] [PubMed]
- Feng S, Zhang X, Gu X, et al. Identification of six novel prognostic gene signatures as potential biomarkers in Small Cell Lung Cancer. Comb Chem High Throughput Screen 2022; Epub ahead of print. [Crossref] [PubMed]
- Jiang N, Meng X, Mi H, et al. Circulating lncRNA XLOC_009167 serves as a diagnostic biomarker to predict lung cancer. Clin Chim Acta 2018;486:26-33. [Crossref] [PubMed]
- Al Matari N, Deeb G, Mshiek H, et al. Anti-Tumor Effects of Biomimetic Sulfated Glycosaminoglycans on Lung Adenocarcinoma Cells in 2D and 3D In Vitro Models. Molecules 2020;25:2595. [Crossref] [PubMed]
(English Language Editor: R. Scott)