Identification of ATP1B1, a key copy number driver gene in diffuse large B-cell lymphoma and potential target for drugs
Introduction
Lymphoma is a malignant tumor that originates from the lymphoid hematopoietic system. Hodgkin’s and non-Hodgkin’s lymphomas are two common types. Existing data surveys have estimated that in 2020, there were 509,590 new cases as well as more than 248,724 lymphoma deaths worldwide (1). Diffuse large B-cell lymphoma (DLBCL) is the most common non-Hodgkin’s lymphoma, accounting for approximately 30% of all cases, which also includes specific subtypes or disease entities (2). Among the current clinical diagnostic and therapeutic strategies, R-CHOP (retuximab, cyclophosphamide, doxorubicin, vincristine, and prednisone) is the preferred treatment choice and has an excellent ability to inhibit disease development (3). There are many patients who either relapse or experience primary refractory disease and eventually succumb to the illness (4,5). To provide new diagnostic and therapeutic ideas for the detection and treatments of DLBCL, DLBCL biomarker-related research and further exploration of the related molecular mechanisms are in demand. Thus, our research provides a new diagnostic and treatment strategy for the clinical treatment of DLBCL.
Genetic elements are key to DLBCL’s etiology. Meanwhile, the development and progression of DLBCL are derived from a multistep pathway that involves genetic alteration accumulations at proto-oncogenes and carcinogenesis of tumor suppressive genes. The mechanisms of the above-mentioned process include chromosomal alterations, single nucleotide variants, copy number variations (CNVs), and epigenetic alterations (6,7). DLBCL carcinogenetic abnormality detection may act as a key indicator of prognosis, and could also act as a key contributing factor to the customized treatment of special markers.
CNVs are a recognized cancer hallmark that may result in the activation of oncogenes and the inactivation of genes that express tumor-suppressing attributes in several malignancies. Meanwhile, they also play an important role in understanding disease progression as well as the clinical identification of biomarkers. These CNV genes can be used to identify the risk of relapse and detect treatment responses (8-10). A previous review focusing on the type and frequency of regional DNA CNVs in DLBCL (not described separately) observed the transformation of indolent lymphoma and DLBCL in immunodeficiency patients in two specific cases (11). A previous study performed a comprehensive genetic analysis of DLBCLs that identifies low-frequency alterations, captured recurrent mutations, somatic copy number alterations and structural variants (12). These studies support the assumption that genetic changes could potentially be treated as diagnostic and prognostic elements as well as treatment targets.
Our study integrated and analyzed CNVs and related differentially expressed genes (DEGs) in The Cancer Genome Atlas (TCGA) DLBCL data to demonstrate the genetic alteration profile of DLBCL. We also analyzed large amounts of patient data acquired from TCGA and combined this data with in vitro experiments conducted in our study to identify the key copy number driver gene, ATP1B1, which is associated with DLBCL, and assess its role in diagnosing and predicting the prognosis of DLBCL. Furthermore, we also aimed to explore its molecular mechanisms in the progression of DLBCL and its potential as a promising target for the treatment of DLBCL. We present the following article in accordance with the MDAR reporting checklist (available at https://atm.amegroups.com/article/view/10.21037/atm-22-4709/rc).
Methods
Data collection and identification of DEGs
The gene expression profile datasets (GSE25638, GSE56315, and GSE10846) were acquired from Gene Expression Omnibus (GEO) (https://www.ncbi.nlm.nih.gov/geo/). The GSE56315 dataset included 55 DLBCL tissues and 33 normal control tissues. As for the GSE25638 dataset, 84 DLBCL tissues and 13 normal control tissues were profiled. The GSE10846 dataset included 420 DLBCL tissues with clinical information, including survival and status. Forty-eight DLBCL tissue copy numbers (CNs) and data related to messenger RNA (mRNA) expression were acquired from the TCGA database (web link: https://portal.gdc.cancer.gov/). The R language package DESeq was used to determine DEGs between the DLBCL samples and non-cancerous tissues (adjusted P<10−5 and |log foldchange| >1 as the cut-off criterion), respectively. The study was conducted in accordance with the Declaration of Helsinki (as revised in 2013).
Construction of the WGCNA co-expression network and identification of the key modules
In this study, we construct a scale-free network. We used a topological adjacency matrix with a weighted coefficient for the estimation of the network connectivity into the overlapping matrix (TOM). We then utilized the clustering method, which is hierarchical, to form the TOM clustering tree structure to determine the different branches representing the various gene modules. Moreover, the different modules were marked with different colors. Furthermore, according to the gene weighted correlation coefficients, we conducted classification based on different genes’ expressive patterns to acquire and analyze the different modules.
Identification of DLBCL key genes after data integration
Linear regression analyses were conducted using the rsm package in R software. The gene expression data used were obtained from TCGA database. In the linear regression analysis, element CN was treated as the dependent variable to evaluate the independent variable, namely, gene expression. We utilized the following equation: Y = b1 * X1, where b1 denoted CN and X1 represented gene expression. The cut-off criteria were R>0.6 and P<0.05.
We then utilized the above-mentioned equation to screen the DEGs, WGCNA module genes, and CN expression-related genes, which were displayed as an overlapping area on a Venn diagram. For TCGA data, we treated homozygous deletion and high-level amplification as an amplified copy number or a deleted gene. During the above-mentioned process, the copy number values were denoted as follows: “hemizygous deletion” was equal to negative 1; “neutral/no change” was equal to 0; “gain” was equal to 1; and “high-level amplification” was equal to 2. We then counted the percentage of the above four copy number cases of the screened genes, and the gene with a high copy number percentage was selected for subsequent study.
Model of logistic regression as well as five-fold cross-validation
During our research, we adopted the logistic regression model demonstrated in TCGA database to perform five-fold cross-validation. Next, we evaluated the diagnostic performance of this gene in DLBCL. A new dataset was formed by randomly selecting 70% of the tumors and controls to avoid imbalanced sizing between groups. We utilized Python version 3.6 (https://www.python.org) to build a logistic regression model. We then evaluated the classification model performance by analyzing the recall, precision, accuracy, and F1 score. Subsequently, we divided the number of true positives by the predicted number of true positives to evaluate the precision of the classification model. Highly accurate DLBCL prediction through the key gene was represented by high precision. We defined “recall” as true positive numbers divided by the real positive numbers. We defined “accuracy” as the proportion of correct predictions found in all samples. To avoid the mutual influence of precision and recall rate on the prediction results, we calculated the comprehensive evaluation index, namely, the F1-score, which is the average of accuracy and recall combined.
Identification of the key gene associated with DLBCL prognosis
After identifying the key gene, we performed a univariate Cox regression analysis to verify whether the gene prognostic predicting power was separated from other clinical characteristics of DLBCL patients, including age, gender, stage, and ECOG status. We set the prognostic and clinical characteristics as independent variables, and overall survival (OS) was set as the dependent variable. We treated a bilateral P value of less than 0.05 (P<0.05) as statistically significant. To further explore the correlation between DLBCL key gene expression and clinical characteristics, the R package was utilized to determine the potential correlation between clinical characteristics (age, gender, stage, ECOG performance status) and the key gene expression levels. Furthermore, we also calculated the hazard ratio and 95% confidence interval (CI) for each clinical variable separately. At the same time, we used the time-dependent receiver operating characteristic (ROC) curve provided by the R survival package to assess the key gene expression accuracy, to allow for prognostic prediction in DLBCL as well as analysis of the 1-, 3-, and 5-year survival of patients, respectively. The results were considered statistically significant when P<0.05.
Functional enrichment analysis and Cmap analysis
To further examine the underlying mechanism between the different ATP1B1 expression groups in the TCGA DLBCL samples, we carried out gene set enrichment analysis (GSEA) using a gene set from the Kyoto Encyclopedia of Genes and Genomes (KEGG) as a reference. We also used a connectivity map (Cmap) to screen out the candidate key gene drugs in DLBCL (13). The average connective tissue scores of small molecule drugs <−0.2 and P<0.05 were considered potential therapeutic drugs for ATP1B1 in DLBCL.
Cell culture and transfection
Cells from the normal control [Cluster of Differentiation 19+B cell (CD19+B)] were isolated from peripheral blood mononuclear cells using CD19 microbeads (Miltenyi Biotec, Auburn, CA, USA). The human DLBCL cell lines, SUDHL5 (DHL5), OCILy3 (Ly3), WSUDLCL2 (LCL2), and NUDUL1 (DUL1), were purchased from Deutsche Sammlung von Microorganismenund Zellkulturen Gmbh (DSMZ: https://www.dsmz.de/). All cell lines were developed in Roswell Park Memorial Institute (RPMI) 1640 medium (Invitrogen, Carlsbad, USA), supplemented with 10% or 20% fetal bovine serum (FBS) (Gibco Invitrogen, Paisley, UK). In this experiment, we used a small interfering RNA (siRNA) targeting ATP1B1 (NM_001677.4) that was manufactured by Guangzhou Ruibo Biological Co., Ltd. (Guangzhou, China).
The siRNA sequence obtained in the experiments is detailed in Table S1. Non-targeting siRNA (si-NC) was used as a negative control. Based on the practical guidelines, the Lipofectamine® 2000 transfection reagent (Thermo Fisher, USA Cat. #11668019) was used for siRNA transfection. After 24 hours, we performed in vitro experiments. The previously constructed plasmid pcDNA3.1-ATP1B1 was used to overexpress ATP1B1. All cells used in the above-mentioned experiments underwent treatment for 4 weeks by G418 with a concentration of 500 µg/mL (Santa Cruz Biotechnology, USA Cat.#sc-29,065) after transfection to acquire a stable and consistent cell line. We utilized the empty vector as a negative control data source.
RNA extraction and quantitative real-time-polymerase chain reaction (qRT-PCR)
The total RNA extracted from cells was treated with the Trizol Reagent (Invitrogen, USA). The RNA sample was later reverse transcribed using a genomic DNA (gDNA) Eraser RT kit (TaKaRa, Japan). We then utilized SYBR-Green (TaKaRa, Japan) and the qRT-PCR method to detect the expression level of complementary DNA (cDNA). Meanwhile, we adopted β-ACTIN as an internal reference. The primers used in our experiment are listed as follows: ATP1B1, Forward, represented by "F":5'-CATCAGAGAGCAACTGATCC-3', Reverse, represented by "R": 5'-ATGCCATTCCGAATCTCAGC-3'; and hβ-ACTIN, Forward, represented by "F": 5'-AGTTGCGTTACACCCTTTCTTG-3', Reverse, represented by "R": 5'-CACCTTCACCGTTCCAGTTTT-3'.
Western blot
The concentration of total proteins extracted with lysis buffer, including protease inhibitors, was measured using a BCA protein assay kit (Thermo Scientific Pierce, USA). Polyvinylidene fluoride (PVDF) membranes (Millipore, Bedford, MA, USA) with proteins isolated on Sodium Dodecyl Sulfate PolyAcrylamide Gel Electrophoresis (SDS-PAGE) gels were blocked using 5% non-fat dry milk and incubated with primary antibodies at 4 ℃ overnight. Western blot was performed with an electrochemiluminescent (ECL) assay reagent (Millipore, USA) according to the manufacturer’s protocol. The following antibodies were used: ATP1B1 (Abcam, Cambridge, UK, ab193669) and glyceraldehyde-3-phosphate dehydrogenase (GAPDH) (Santa Cruz Biotechnology, sc-47724).
Cell proliferation, Transwell migration, and invasion assays
All cells mentioned above were acquired 24 hours after transfection and seeded in 96-well plates, giving us an allocation of 5×103 cells per well. We incubated all of the cells overnight and used the Cell Counting Kit-8 (Roche Applied Science) to determine the cell proliferation results at 24, 48, 72, and 96 hours after transfection. A Transwell plate with 24-wells (8-µm pore size) manufactured by Corning (Cat. #3422) was adopted to determine the Transwell. We harvested 1×105 cells (treated with 100 µL serum-free medium) during the migration assay experiment and added these cells to the above compartment with no Matrigel.
As for the invasion test, we pre-coated Matrigel (dilution ration of 1:8) (BD, USA, catalog number #356234) with a Transwell filter before adding the cell suspension. Next, we placed 600 µL of 30% FBS medium into the bottom of the compartment as the origin of chemical attractants in the compartment. After allowing the cells to culture for 24 hours, we fixed those cells that crossed the inserts and stained them with crystal violet. We then identified and photographed migrated cells, and also counted these cells using an inverted microscope at a magnification of 100 times.
Cell adhesion assay
According to the related operation guidelines, we used the Vybrant cell adhesion detection kit (Molecular probe, USA) for cell adhesion detection in a 96-well culture plate coated with fibronectin (7 µg/cm2). The cells used in all experiments were in similar growth conditions (semi-fusion). After harvesting, the cells were washed with phosphate buffered saline (PBS) and then resuspended in a medium containing 5-µM dye with Calcein Acetoxymethyl Ester (calcein AM). Subsequently, we washed these cells and resuspended them in recombinant stromal cell-derived factor-1 (SDF-1) or conditioned medium with or without Plerixafor (AMD3100). Later, we seeded the cells in a 96-well culture plate coated with fibronectin, in which the cell density reached 5×104 cells per well in triplicate. We incubated the cells for 15 minutes, facilitating the most optimal conditions for cell adhesion to occur. Non-adherent cells were appropriately screened and washed out using a PBS agent. We then identified the adherent cells using an Enzyme-Linked Immunosorbent Assay (ELISA) reagent kit in a chemiluminescent immunoassay analyzer (Abbott, USA) with a wavelength of 494±20 nm. We performed all of the above-mentioned experiments independently and separately three times, and the results obtained from these experiments reflected the relative cell adhesion rate.
Statistical analysis
The data were compiled and analyzed using SPSS 16.0 software (IBM, New York, USA). A two-tailed Student’s t-test and one-way analysis of variance (ANOVA) were used for comparisons between two groups and multi-group (more than two) comparisons, respectively. We considered P<0.05 as statistically significant. Data were presented as the mean ± standard error of mean (SEM) from one representative experiment of three independent experiments, and every representative experiment was repeated three times. Based on the different ATP1B1 expression levels, we aimed to explore the correlation between DLBCL patients’ clinicopathological characteristics by utilizing Chi-squared analysis.
Results
Identification of DEGs in DLBCL
Compared to the normal controls, the volcano plots and profiles of gene expression acquired from the GSE25638 dataset showed 2,227 DEGs in the DLBCL samples, including upregulated 1,317 genes and 910 genes downregulated genes (Figure 1A). Also, a total of 7,791 DEGs were identified from the GSE56315 dataset, of which 3,437 genes were upregulated and 4,354 genes were downregulated in DLBCL samples (Figure 1B). According to the standard cut-off threshold, we selected DEGs as the data source for integrated analysis. As shown in the Venn diagram, we obtained 1,594 common DEGs from the above two datasets (Figure 1C). As shown in see Figure 1D, the heat map displays the common DEGs in the GSE25638 dataset in the tumor and normal expression profiles.
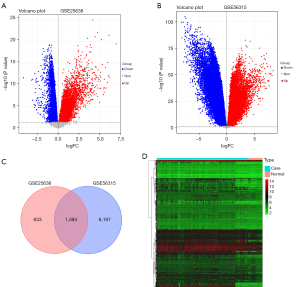
Co-expression network construction and module identifications
A total of 420 DLBCL patients and related survival data acquired from the GSE10846 dataset were included in the WGCNA (Figure 2A), based on which we identified the key model with related genes. To construct a scale-free network, we decided to select (scale-free R2=0.900) β2=2 as the soft threshold (Figure 2B). We later adopted the merged dynamic tree for cutting and excluding as well as for screening gray modules. As shown in Figure 2C, we identified a total of six gene co-expression modules. In our study, TOM between 1,000 selected genes was depicted using a heat map, where it was found that each module served as an independent validation to another one (Figure 2D). Subsequently, we identified the turquoise module in our test as the important module, accounting for the highest correlation with factor os_time (R2=0.25, P=3e-07) and status (R2=−0.29, P=9e-10) in DLBCL. In the turquoise gene module, 160 genes met the conditions of module membership (MM) <−0.5 and gene significance (GS) >0.20 (Figure 2E).
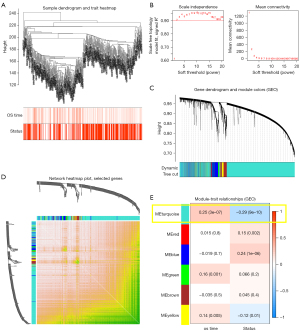
Identification of DLBCL key genes after data integration
It is known that CNV is related to alterations in gene expression. A total of 2,192 expression-copy number variations-related (Exp-CNV-related) genes were identified by linear regression analysis of CNV and differential gene expression in TCGA, with a cutoff R>0.4 and P<0.05 (Figure 3A). Next, we obtained two overlapping genes, namely ATP1B1 and LIMA1, by overlapping DEGs with module genes and Exp-CNV-related genes (Figure 3B). Thereafter, we found that both ATP1B1 and LIMA1 were copy number driven genes, where the copy number amplification of ATP1B1 was 33.33% (48 total patients, 16 patients with increased CNV, Figure 3C), while the copy number amplification of LIMA1 was 20.83% (48 total patients, 10 patients with increased CNV, Figure 3D). In short, we observed a significant abnormality in the ATP1B1 copy number in TCGA, indicating that it was the key gene suitable for further DLBCL study.
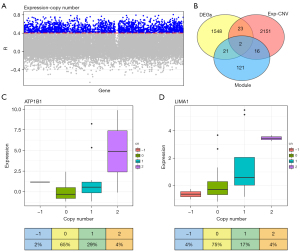
ATP1B1 is overexpressed and can be a biomarker for DLBCL diagnosis
To explore the undetermined physiological role of ATP1B1 in DLBCL in depth, we examined the ATP1B1 message RNA (mRNA) expression degree in four DLBCL cell lines, namely DHL5, Ly3, LCL2, and DUL1. We also examined the ATP1B1 mRNA expression level in the normal control, namely the CD19+B cell line. The results revealed that ATP1B1 was upregulated in the DLBCL cell lines compared to the CD19+B cell line, as shown in Figure 4A. The Ly3 cell line, which showed the highest ATP1B1 expression level, and the DUL1 cell line, which exhibited the lowest ATP1B1 expression level, were selected as the DLBCL cell lines for subsequent experiments.

It is well known that early screening and prognosis are vital for cancer patients. We screened the copy number driver gene, ATP1B1, from TCGA DLBCL data, which is a potential biomarker in DLBCL; as shown in Figure 4B, an AUC statistical mean (0.940±0.050) was obtained from the ROC curve of ATP1B1. As shown in Figure 4C, the accuracy, recall, precision, F1 score, and AUC were measured as 0.94, 0.76, 0.85, 0.79, and 0.94, respectively. These results demonstrated that ATP1B1 could potentially be utilized to distinguish DLBCL samples from the normal controls, thus making it a potential biomarker.
ATP1B1 is an independent biomarker for predicting DLBCL prognosis
Prognostic prediction is very helpful in guiding the clinical prognosis and treatment strategies of cancer patients. Thus, to further analyze the prognostic impact of ATP1B1 on DLBCL patients, a univariate Cox analysis was performed using the GSE10846 dataset. The analytical results indicated that only ATP1B1 was related to the OS shown in the study, and the difference was statistically significant (P<0.001, Figure 5A). This suggested that the ATP1B1 gene expression levels had a greater impact on the prognosis of patients with DLBCL compared to other clinical characteristics.
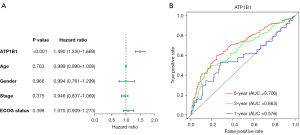
The time-dependent ROC curve showed that the AUC values for ATP1B1 expression to predict the survival of DLBCL patients at 1, 3, and 5 years were 0.576, 0.663, and 0.706 respectively (Figure 5B). These results indicated that the ATP1B1 gene expression level has potential and promising value for the long-term prognostic survivability of patients diagnosed with DLBCL. The Chi-square test results showed that high ATP1B1 expression was only associated with ECOG performance status (P=0.036) but not with age, gender, or stage (P>0.05), as shown in Table 1.
Table 1
Variables | High | Low | P value |
---|---|---|---|
Age (years) | |||
>50 | 134 | 146 | 0.1789 |
≤50 | 50 | 38 | |
Gender | |||
Male | 111 | 98 | 0.2067 |
Female | 73 | 86 | |
Stage | 0.1439 | ||
I–II | 95 | 80 | |
III–IV | 89 | 104 | |
ECOG performance status | 0.03622 | ||
0 | 37 | 43 | |
1–2 | 133 | 123 | |
3–4 | 14 | 18 |
DLBCL, diffuse large B-cell lymphoma; ECOG, Eastern Cooperative Oncology Group.
ATP1B1 promoted cell proliferation and induced cell migration, invasion, and adhesion in DLBCL cell lines
To explore the underlying biological function of ATP1B1 in DLBCL, we performed a KEGG pathway analysis of the ATP1B1 co-expression genes. We first divided the DLBCL tumor patients into high- and low-expression groups based on the median expression of ATP1B1 in TCGA database. Genome-wide t-values were obtained by Bayesian analysis in R software for KEGG pathway enrichment analysis. The results showed that ATP1B1 was associated with ECM receptor interactions (hsa04512), olfactory transduction (hsa04740), focal adhesion (hsa04510), cytokine-cytokine receptor interactions (CCRIs) (hsa04060), and hematopoietic cell lineages (hsa04640), as shown in Figure 6A,6B. Therefore, we suggested that this gene was associated with tumor metastasis.
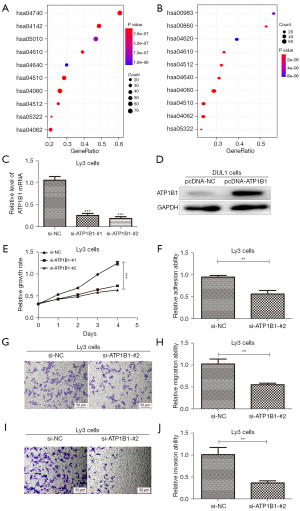
For in-depth verification of the above bioinformatics analysis results, we used siRNAs to knock down the expression of endogenous ATP1B1 in Ly3 cells, as shown in Figure 6C. The DNA plasmid vectors pcDNA3.1-ATP1B1 was adopted to transfect DUL1 cells for ATP1B1 overexpression (Figure 6D). According to the above results, siATP1B1-#2 was used in the research. The effect of ATP1B1 knockdown on the proliferation of cells was analyzed. The CCK-8 assay within Ly3 cells demonstrated that si-ATP1B1-#2 significantly inhibited cell proliferation (Figure 6E).
Cell adhesion is key in cancer invasion and metastasis (14), and therefore, we aimed to identify whether ATP1B1 could be used as a regulator to adjust the adhesion ability of cells. During our experiment, ATP1B1 knockdown in Ly3 cells restricted the cell adhesion ability (Figure 6F). The Transwell assay demonstrated that the expression level of ATP1B1 was positively correlated to the migration and invasion ability in Ly3 cells (Figure 6G-6J). These results provide evidence that ATP1B1 acts as a promoter of cell proliferation, cell adhesion, and tumor invasiveness in DLBCL.
Furthermore, we also aimed to explore new DLBCL therapeutic regimens through the Cmap database. We obtained and listed the nine most related molecule drugs that are small in size, which are displayed in Table 2). As shown in Figure 7A-7C, among these small molecule drugs, the chemical structures of the three most significant small molecule drugs were subsequently obtained from the DRUG BANK (https://go.drugbank.com/). Subsequently, roxithromycin (mean connective score =−0.613; P=0.00233; Table 2) was identified as a potential targeted therapeutic drug for ATP1B1 in DLBCL. Roxithromycin partially rescued the overexpression of ATP1B1 to induce cell proliferation in DUL1 cells (Figure 7D). Our experimental results showed that ATP1B1 could manage to increase the DLBCL cell lines’ migration, invasion, and adhesion abilities.
Table 2
Cmap name | Mean connective Score | Number | Enrichment | P | Specificity | Percent non-null |
---|---|---|---|---|---|---|
Roxithromycin | −0.613 | 4 | −0.812 | 0.00233 | 0.0278 | 100 |
Daunorubicin | −0.601 | 4 | −0.894 | 0.00024 | 0.0088 | 100 |
Fipexide | −0.569 | 3 | −0.804 | 0.01528 | 0.0221 | 100 |
Idazoxan | −0.546 | 4 | −0.79 | 0.00396 | 0.0109 | 100 |
hydrochloride | −0.54 | 2 | −0.85 | 0.04481 | 0.1572 | 100 |
Betamethasone | −0.534 | 3 | −0.825 | 0.01076 | 0.021 | 100 |
Cobalt chloride | −0.487 | 3 | −0.755 | 0.02985 | 0.0315 | 100 |
Bupivacaine | −0.486 | 4 | −0.765 | 0.00619 | 0 | 100 |
Caffeic acid | −0.474 | 3 | −0.756 | 0.02981 | 0.0185 | 100 |
DLBCL, diffuse large B-cell lymphoma; Cmap, Connectivity Map.
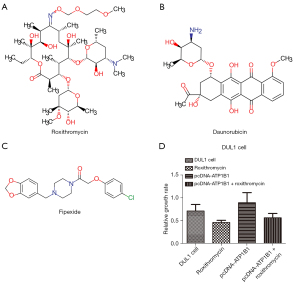
Discussion
Based on our CNV analysis, we found that the ATP1B1-adenosine triphosphatase Na+/K+ (ATPase Na+/K+) transporting subunit beta 1 is a promising biomarker in DLBCL. The complex membrane protein Na+/K+-ATPase uses ATP to transport Na+/K+ across the plasma membranes. This enzyme is comprised of three elements, namely, the α-subunit (112 kDa), β-subunit (55 kDa), and other elements of the FXYD family, that is phenylalanine (F), X, tyrosine (Y), and aspartate (D) (15,16). Oncogenic transformation of renal epithelial cells has been reported to lead to reduced ATP1B1 expression as well as the promotion of cell invasiveness and metastatic behaviors (17,18). Among the various cancer cells that experience epithelial-mesenchymal transition (EMT), the ATP1B1 level also demonstrates a corresponding decreasing trend. This process is related to cancer progression and metastasis-related disease (19). Since ATP1B1 suppresses these characteristics, we propose that ATP1B1 is a potential tumor suppressor.
Previous studies have pointed out that Na+/K+-ATPase is a kind of transduction that plays an essential role in cell adhesion (20), cell motility (21), cell proliferation, apoptosis (22), and signal transduction (23). Therefore, Na+/K+-ATPase is considered to be the primary target of tumor therapy. Emerging research has demonstrated that Na+/K+-ATPase is abnormally expressed among various types of cancers (24) and is related to cancer prognosis (25). The present research mainly focuses on exploring the function of the α-subunit; however, research on the function of the β-subunit remains limited. The Na+/K+-ATPase β1 subunit is encoded by ATP1B1 to establish and maintain the electrochemical gradients of Na+ and K+ across the plasma membrane. Moreover, recent studies have reported that ATP1B1 is downregulated in human epithelial cancer cells (26), and impaired ATP1B1 expression may lead to the development of pulmonary fibrosis (27). Moreover, ATP1B1 methylation restricts the β-subunit’s activity and simultaneously promotes the development of renal cell carcinoma (28). Cheon et al. proposed ATP1B1 as a new type of Neuronal Growth Regulator 1 (NEGR1) interacting protein, which may help decipher the molecular network of human diseases related to NEGR1 (29). However, our understanding of the clinical relevance of ATP1B1 in DLBCL is still limited, and more studies on the biological mechanism of ATP1B1 are needed.
In this study, multiple public databases were used for the first time to comprehensively analyze the expression of ATP1B1 in DLBCL, assess its correlation with DLBCL prognosis, and reveal its possible mechanism in DLBCL. We screened the copy number driver gene ATP1B1, which is associated with DLBCL, using the GEO and TCGA databases. Our findings suggested that ATP1B1 is significantly upregulated in DLBCL, with logFC >1 and P<0.01, using TCGA and GEO datasets. These results indicated that ATP1B1 could potentially play a role during DLBCL development. Subsequently, ATP1B1 was experimentally verified as a potential marker of DLBCL diagnosis, exhibiting high sensitivity and specificity via the ROC curve analytical method. Next, our study examined the clinicopathological relevance of ATP1B1 expression in DLBCL patients. From our experiments, we found that a high expression level of ATP1B1 is potentially corrlated with the ECOG performance status (P<0.05). The univariate Cox analysis and time-dependent ROC curve indicated that the ATP1B1 gene expression level has a high prognostic value in patients with DLBCL. These results illustrated that ATP1B1 is a useful biomarker for the clinical diagnosis and prognosis of DLBCL.
Recent studies have pointed out that mutations found within the sodium-potassium pump could potentially result in cancer cell proliferation. This process is caused by the joint action of multiple signaling pathways. The phosphatidylinositol 3-kinase/AKT/mammalian target of rapamycin, PI3K/Akt/mTOR, (PAM) signaling, EMT, the p38 mitogen-activated protein kinase (MAPK) cascade, p21 Cip, and the cholesterol steady state constitute evidence that supplements the above. Furthermore, these pathways have been shown to promote cancer development and are related to the α and β subunits of Na+/K+-ATPase (30). Among them, the β-subunit potentially plays an important role in the suppression of cancer tumorigenesis and metastasis (31). To study the potential mechanism of ATP1B1 in DLBCL, we analyzed the co-expressed genes of ATP1B1 and found that ATP1B1 is mainly involved in information adhesion, olfactory transduction, CCRI, and extracellular matrix-receptor (ECM receptor) interaction. Therefore, it can be assumed that ATP1B1 may potentially participate in the regulatory process of DLBCL focal adhesions.
In addition, compared with the normal CD19+B cell lines, ATP1B1 in multiple DLBCL cell lines showed a significant increasing trend. The biological characteristics of tumor cells can explain these findings. Compared with normal cells, the metabolism of tumor cells is more vigorous; therefore, as the level of Na+/K+-ATPase increases, the expression of ATP1B1 also exhibits an increasing trend. Moreover, we also confirmed that silenced ATP1B1 may potentially restrict DLBCL cell migration, proliferation, and invasion, thereby inhibiting cell adhesion in DLBCL cell lines. In summary, our experimental results showed that ATP1B1 could potentially act as an oncogene while promoting DLBCL tumorigenicity.
Chemotherapy is the preferred treatment strategy for DLBCL. To further explore new treatment options for DLBCL, we used the Cmap database to screen potential small-molecule targeted drugs (Roxithromycin, Daunorubicin, Haloperidol, and Imidazoxan) that can provide benefits for DLBCL patients exhibiting high ATP1B1 expression. However, research on the effects of these drugs on tumors is limited, and more experiments are needed to evaluate their clinical therapeutic effects. Among the above-mentioned drugs, roxithromycin (RXM) has a broad antibacterial spectrum and immunomodulatory effect on pathogens. Previous research had indicated that RXM could effectively restrict the growth and tumor-induced angiogenesis of tumor cells in mouse models, and may also affect the growth of human liver cancer cells (32,33). Additionally, we also observed that Roxithromycin effectively inhibited the overexpression of ATP1B1 by inducing cell proliferation in DUL1 cells.
In summary, our research shows that a high level of ATP1B1 expression in human DLBCL tissues and cell lines is closely correlated to the clinical characteristics of DLBCL patients. The ATP1B1 protein may affect DLBCL tumorigenesis and tumor progression by regulating cell adhesion pathways. Therefore, the expression of ATP1B1 may be a reliable diagnostic and prognostic biomarker for DLBCL. This study further demonstrated that CNV plays an important role in DLBCL, and high copy number of ATP1B1 led to increased expression of ATP1B1 gene, which resulted in increased proliferation and cell invasiveness of DLBCL cells. and direction. Meanwhile, this study found that Roxithromycin, a target drug of ATP1B1, can effectively inhibit the proliferation of DLBCL, which is a potential therapeutic target and direction. In a word, ATP1B1 may also act as an effective yet potential therapeutic target for DLBCL treatment.
Acknowledgments
Funding: None.
Footnote
Reporting Checklist: The authors have completed the MDAR reporting checklist. Available at https://atm.amegroups.com/article/view/10.21037/atm-22-4709/rc
Conflicts of Interest: All authors have completed the ICMJE uniform disclosure form (available at https://atm.amegroups.com/article/view/10.21037/atm-22-4709/coif). The authors have no conflicts of interest to declare.
Ethical Statement: The authors are accountable for all aspects of the work in ensuring that questions related to the accuracy or integrity of any part of the work are appropriately investigated and resolved. The study was conducted in accordance with the Declaration of Helsinki (as revised in 2013).
Open Access Statement: This is an Open Access article distributed in accordance with the Creative Commons Attribution-NonCommercial-NoDerivs 4.0 International License (CC BY-NC-ND 4.0), which permits the non-commercial replication and distribution of the article with the strict proviso that no changes or edits are made and the original work is properly cited (including links to both the formal publication through the relevant DOI and the license). See: https://creativecommons.org/licenses/by-nc-nd/4.0/.
References
- Sung H, Ferlay J, Siegel RL, et al. Global Cancer Statistics 2020: GLOBOCAN Estimates of Incidence and Mortality Worldwide for 36 Cancers in 185 Countries. CA Cancer J Clin 2021;71:209-49. [Crossref] [PubMed]
- Bakhshi TJ, Georgel PT. Genetic and epigenetic determinants of diffuse large B-cell lymphoma. Blood Cancer J 2020;10:123. [Crossref] [PubMed]
- Susanibar-Adaniya S, Barta SK. 2021 Update on Diffuse large B cell lymphoma: A review of current data and potential applications on risk stratification and management. Am J Hematol 2021;96:617-29. [Crossref] [PubMed]
- Morrison VA, Shou Y, Bell JA, et al. Evaluation of treatment patterns and survival among patients with diffuse large B-cell lymphoma in the USA. Future Oncol 2019;15:1021-34. [Crossref] [PubMed]
- Friedberg JW. Relapsed/refractory diffuse large B-cell lymphoma. Hematology Am Soc Hematol Educ Program 2011;2011:498-505. [Crossref] [PubMed]
- Bakhoum SF, Danilova OV, Kaur P, et al. Chromosomal instability substantiates poor prognosis in patients with diffuse large B-cell lymphoma. Clin Cancer Res 2011;17:7704-11. [Crossref] [PubMed]
- Li P, Chai J, Chen Z, et al. Genomic Mutation Profile of Primary Gastrointestinal Diffuse Large B-Cell Lymphoma. Front Oncol 2021;11:622648. [Crossref] [PubMed]
- Varma S, Pommier Y, Sunshine M, et al. High resolution copy number variation data in the NCI-60 cancer cell lines from whole genome microarrays accessible through CellMiner. PLoS One 2014;9:e92047. [Crossref] [PubMed]
- Hanahan D, Weinberg RA. Hallmarks of cancer: the next generation. Cell 2011;144:646-74. [Crossref] [PubMed]
- Wang Y, Wenzl K, Manske MK, et al. Amplification of 9p24.1 in diffuse large B-cell lymphoma identifies a unique subset of cases that resemble primary mediastinal large B-cell lymphoma. Blood Cancer J 2019;9:73. [Crossref] [PubMed]
- Cascione L, Aresu L, Baudis M, et al. DNA Copy Number Changes in Diffuse Large B Cell Lymphomas. Front Oncol 2020;10:584095. [Crossref] [PubMed]
- Chapuy B, Stewart C, Dunford AJ, et al. Molecular subtypes of diffuse large B cell lymphoma are associated with distinct pathogenic mechanisms and outcomes. Nat Med 2018;24:679-90. [Crossref] [PubMed]
- Lamb J. The Connectivity Map: a new tool for biomedical research. Nat Rev Cancer 2007;7:54-60. [Crossref] [PubMed]
- Gkretsi V, Stylianopoulos T. Cell Adhesion and Matrix Stiffness: Coordinating Cancer Cell Invasion and Metastasis. Front Oncol 2018;8:145. [Crossref] [PubMed]
- Rajasekaran SA, Rajasekaran AK. Na,K-ATPase and epithelial tight junctions. Front Biosci (Landmark Ed) 2009;14:2130-48. [Crossref] [PubMed]
- Vagin O, Dada LA, Tokhtaeva E, et al. The Na-K-ATPase α1β1 heterodimer as a cell adhesion molecule in epithelia. Am J Physiol Cell Physiol 2012;302:C1271-81. [Crossref] [PubMed]
- Rajasekaran SA, Palmer LG, Quan K, et al. Na,K-ATPase beta-subunit is required for epithelial polarization, suppression of invasion, and cell motility. Mol Biol Cell 2001;12:279-95. [Crossref] [PubMed]
- Inge LJ, Rajasekaran SA, Yoshimoto K, et al. Evidence for a potential tumor suppressor role for the Na,K-ATPase beta1-subunit. Histol Histopathol 2008;23:459-67. [PubMed]
- Espineda CE, Chang JH, Twiss J, et al. Repression of Na,K-ATPase beta1-subunit by the transcription factor snail in carcinoma. Mol Biol Cell 2004;15:1364-73. [Crossref] [PubMed]
- Magpusao AN, Omolloh G, Johnson J, et al. Cardiac glycoside activities link Na(+)/K(+) ATPase ion-transport to breast cancer cell migration via correlative SAR. ACS Chem Biol 2015;10:561-9. [Crossref] [PubMed]
- Fujii T, Shimizu T, Yamamoto S, et al. Crosstalk between Na+,K+-ATPase and a volume-regulated anion channel in membrane microdomains of human cancer cells. Biochim Biophys Acta Mol Basis Dis 2018;1864:3792-804. [Crossref] [PubMed]
- Felippe Gonçalves-de-Albuquerque C, Ribeiro Silva A, Ignácio da Silva C, et al. Na/K Pump and Beyond: Na/K-ATPase as a Modulator of Apoptosis and Autophagy. Molecules 2017;22:578. [Crossref] [PubMed]
- Wang HY, O'Doherty GA. Modulators of Na/K-ATPase: a patent review. Expert Opin Ther Pat 2012;22:587-605. [Crossref] [PubMed]
- Mijatovic T, Dufrasne F, Kiss R. Na+/K+-ATPase and cancer. Pharm Pat Anal 2012;1:91-106. [Crossref] [PubMed]
- Alevizopoulos K, Calogeropoulou T, Lang F, et al. Na+/K+ ATPase inhibitors in cancer. Curr Drug Targets 2014;15:988-1000. [Crossref] [PubMed]
- Rajasekaran SA, Barwe SP, Rajasekaran AK. Multiple functions of Na,K-ATPase in epithelial cells. Semin Nephrol 2005;25:328-34. [Crossref] [PubMed]
- Li B, Huang X, Xu X, et al. The profibrotic effect of downregulated Na,K ATPase β1 subunit in alveolar epithelial cells during lung fibrosis. Int J Mol Med 2019;44:273-80. [Crossref] [PubMed]
- Selvakumar P, Owens TA, David JM, et al. Epigenetic silencing of Na,K-ATPase β 1 subunit gene ATP1B1 by methylation in clear cell renal cell carcinoma. Epigenetics 2014;9:579-86. [Crossref] [PubMed]
- Cheon Y, Yoo A, Seo H, et al. Na/K-ATPase beta1-subunit associates with neuronal growth regulator 1 (NEGR1) to participate in intercellular interactions. BMB Rep 2021;54:164-9. [Crossref] [PubMed]
- Durlacher CT, Chow K, Chen XW, et al. Targeting Na+/K+ -translocating adenosine triphosphatase in cancer treatment. Clin Exp Pharmacol Physiol 2015;42:427-43. [Crossref] [PubMed]
- Silva CID, Gonçalves-de-Albuquerque CF, Moraes BPT, et al. Na/K-ATPase: Their role in cell adhesion and migration in cancer. Biochimie 2021;185:1-8. [Crossref] [PubMed]
- Yatsunami J, Tsuruta N, Fukuno Y, et al. Inhibitory effects of roxithromycin on tumor angiogenesis, growth and metastasis of mouse B16 melanoma cells. Clin Exp Metastasis 1999;17:119-24. [Crossref] [PubMed]
- Aoki D, Ueno S, Kubo F, et al. Roxithromycin inhibits angiogenesis of human hepatoma cells in vivo by suppressing VEGF production. Anticancer Res 2005;25:133-8. [PubMed]
(English Language Editor: A. Kassem)