Polymorphisms in drug metabolism genes predict the risk of refractory myasthenia gravis
Introduction
Myasthenia gravis (MG) is a rare autoantibody-mediated disease characterized by muscle weakness and fatiguability (1). The majority of MG patients showed marked improvement or remission via the use of pyridostigmine (Pyri), glucocorticoids (GCs), immunosuppressants, and/or thymectomy (2). However, about 10% to 20% of MG patients are classified as refractory due to a suboptimal response or intolerance to conventional treatment (3,4).
It is well recognized that single nucleotide polymorphisms (SNPs) in genes coding for drug-metabolizing enzymes and transporters influence the response to medicines (5). GCs are used as first-line immunosuppressants for MG patients (2). Glucocorticoid receptor (GR; NR3C1, nuclear receptor subfamily 3 group C member 1) polymorphisms contribute to the efficacy of GCs in MG patients (6). FK506 binding protein 5 (FKBP5) represents a target for drugs such as rapamycin and tacrolimus and its genetic variation exerts a role in chemoresistance (7). Allelic variants of heat shock protein 90 Alpha family class A member 1 (HSP90AA1) (8,9) and multidrug resistance protein 1 (MDR1) are reasons for the refractory to pharmaceutical treatment (10,11). In addition, genetic polymorphisms of cytochrome P450 (CYP) 3A4/3A5 influence the blood trough concentration and efficacy of tacrolimus for MG patients (12,13).
Genetic risk factors that determine the patients at high risk for refractory MG remain unknown (14). In this study, we analyzed 13 SNPs in six drug metabolism genes (NR3C1, FKBP5, HSP90AA1, MDR1, CYP3A4, and CYP3A5) to explore their roles in developing refractory MG. We present the following article in accordance with the TRIPOD reporting checklist (available at https://atm.amegroups.com/article/view/10.21037/atm-22-2543/rc).
Methods
Study design and participants
The sample size was calculated to be at least 26 for the refractory group [α =0.05; power =0.80; minor allele frequency (MAF) =0.204 (minimum MAF for all candidate SNPs from databases)] using Quanto 1.2.4 (http://biostats.usc.edu/Quanto.html). One hundred and thirty-one MG patients who were admitted to the Department of Neurology, Tongji Hospital, Tongji Medical College, Huazhong University of Science and Technology from October 2013 to July 2020 were eventually included in this retrospective study. The study was conducted in accordance with the Declaration of Helsinki (as revised in 2013). All participants or their legal guardians gave written informed consents. Ethical approval for this study was obtained from the Ethics Committee of Tongji Hospital (protocol ID: TJ-IRB20191208). Clinical data as well as blood samples were collected at the time of enrollment.
Inclusion criteria: (I) patients of all ages with a diagnosis of MG; (II) followed up for at least 1 year. MG diagnosis was based on typical fluctuating muscle weakness with one or more of the following criteria (15): (I) presence of AChR or MuSK antibodies; (II) a decrement over 10% on repetitive nerve stimulation; (III) Pyri treatment was effective. Patients complicated with malignancies, infections, neurodegenerative diseases, or other autoimmune diseases were excluded.
Refractory MG was defined as at least one of the following during the follow-up (16): (I) patients respond insufficiently to maximal safe doses of GCs and at least one immunosuppressive drug at an adequate dose and duration; (II) inability to reduce immunosuppressive therapy without clinical relapse or require repeated rescue therapy such as intravenous immunoglobulin (IVIG) or plasma exchange (PLEX); (III) intolerable adverse effects from immunosuppressive therapy; (IV) comorbid conditions that restrict the use of conventional therapies; (V) frequent myasthenic crises even while on therapy.
The severity of the disease was assessed by Myasthenia Gravis Foundation of America (MGFA) clinical classification (17) and quantified MG (QMG) scores (18). Clinical status of the patients was determined by the MGFA post-intervention status (PIS) (17).
SNP selection and genotyping
Gene information was available from the National Center for Biotechnology Information (NCBI) dbSNP database (https://www.ncbi.nlm.nih.gov/projects/SNP). We investigated the impact of 13 polymorphisms in six genes potentially associated with pharmacogenetics of immunologic agents, including NR3C1 rs17209237, rs9324921; FKBP5 rs1360780, rs4713904, rs9296158; HSP90AA1 rs10873531, rs2298877, rs7160651; MDR1 rs1045642, rs1128503, rs2032582; CYP3A4 rs2242480; and CYP3A5 rs776746. DNA from blood was extracted from peripheral blood using Gentra Puregene Blood Kit (Qiagen). SNP genotyping was performed by the improved multiplex ligation detection reaction (iMLDR) technique (Genesky Biotechnologies Inc., Shanghai, China) as previously described (19). Primer sequences for multiplex polymerase chain reaction (PCR). are provided in Table S1. Negative controls and duplicate samples were used to monitor genotyping quality. Genotypes were conducted using GeneMapper 4.0 (Applied Biosystems, USA).
Statistical analysis
Continuous variables were expressed as median (interquartile range) and categorical variables were described as frequency (percentages). Comparisons between groups were analyzed using Mann-Whitney U-test or Chi-squared test as appropriate. All genotypes were examined for Hardy-Weinberg equilibrium (HWE). Multivariable logistic regression was performed to determine the genetic risk factors associated with the incidence of refractory MG after adjusting for age, sex, MGFA classification, QMG scores, medicine treatment, and history of thymectomy. We used receiver operating characteristics curves with area under the curve (AUC) values to evaluate the logistic regression model.
No correction for multiple testing was applied due to the exploratory character of this study. Two-sided statistical significance was set at P value <0.05. Data were analyzed by GraphPad Prism 8.01 (GraphPad Prism, San Diego, CA, USA) and SPSS 24 (IBM, Armonk, NY, USA). Linkage disequilibrium (LD) blocks were constructed with Haploview software (http://www.broad.mit.edu/mpg/haploview) and haplotype-based association analyses were performed by SHEsis software (http://analysis.bio-x.cn/myAnalysis.php). LD analysis was assessed by D’ and r2 values as described elsewhere (20). Gene-gene interactions were analyzed using generalized multifactor dimensionality reduction (GMDR) software (21).
Results
SNPs information
All the polymorphisms were in HWE (P>0.05). The 13 SNPs included one in the 3’ flanking sequence, four in exons, and eight in introns. The genotype information for all candidate SNPs is summarized in Table 1.
Table 1
SNPs | Gene | Chr | Chr position | HGVS names | SNP property | Functional change |
P value for HWE |
MAF | |
---|---|---|---|---|---|---|---|---|---|
Database# | Detected in this study | ||||||||
rs17209237 | NR3C1 | 5 | 142657212 | NM_000176.2:c.*4242T>C | 3’-Flanking | – | 0.347 | 0.204 | 0.176 |
rs9324921 | NR3C1 | 5 | 142767740 | NM_000176.2:c.1184+11481G>T | intron2 | – | 0.899 | 0.266 | 0.267 |
rs1360780 | FKBP5 | 6 | 35607571 | NM_004117.3:c.106-2636A>G | intron2 | – | 0.805 | 0.286 | 0.263 |
rs4713904 | FKBP5 | 6 | 35625147 | NM_004117.3:c.-19-14527G>A | intron1 | – | 1.000 | 0.324 | 0.271 |
rs9296158 | FKBP5 | 6 | 35567082 | NM_004117.3:c.509-1901T>C | intron5 | – | 0.249 | 0.344 | 0.321 |
rs10873531 | HSP90AA1 | 14 | 102568296 | NM_001017963.2:c.282C>T | synon_exon2 | p.=(Thr94Thr) | 0.378 | 0.258 | 0.271 |
rs2298877 | HSP90AA1 | 14 | 102548224 | NM_001017963.2:c.2456-66A>G | intron11 | – | 0.331 | 0.235 | 0.240 |
rs7160651 | HSP90AA1 | 14 | 102564159 | NM_001017963.2:c.366+4053C>T | intron2 | – | 0.222 | 0.226 | 0.218 |
rs1045642 | MDR1 | 7 | 87138645 | NM_000927.4:c.3435T>C | synon_exon27 | p.=(Ile1145Ile) | 0.902 | 0.383 | 0.370 |
rs1128503 | MDR1 | 7 | 87179601 | NM_000927.4:c.1236T>C | synon_exon13 | p.=(Gly412Gly) | 0.471 | 0.374 | 0.389 |
rs2032582 | MDR1 | 7 | 87160618 | NM_000927.4:c.2677T>G | nonsynon_exon22 | p.Ser893Ala | 0.233 | 0.434 | 0.355 |
NM_000927.4:c.2677T>A | p.Ser893Thr | ||||||||
rs2242480 | CYP3A4 | 7 | 99361466 | NM_017460.5:c.1026+12G>A | intron10 | – | 0.280 | 0.321 | 0.290 |
rs776746 | CYP3A5 | 7 | 99270539 | NM_000777.5:c.219-237A>G | intron3 | – | 0.191 | 0.287 | 0.244 |
#, MAF in the Asian population from dbSNP databases. SNPs, single nucleotide polymorphisms; Chr, chromosome; HGVS, Human Genome Variation Society; HWE, Hardy-Weinberg equilibrium; MAF, minor allele frequency.
Demographic and disease characteristics
Clinical characteristics are shown in Table 2. The median age of the patients in the study (86 females and 45 males) was 24.50 (11.76 to 47.14) years and the median follow-up duration was 2.53 (1.47–7.20) years. Patients were divided into refractory group (n=33, 25.2%) and non-refractory group (n=98, 74.8%) based on their treatment responses throughout the follow-up period. Refractory patients were more severely ill at enrollment, with higher MGFA classification (P=0.012), QMG scores (P<0.001). More patients in the refractory group received aggressive medicine treatment (P=0.025) and underwent thymectomy (P=0.037) compared with patients in the non-refractory group.
Table 2
Variables | Non-refractory (n=98) | Refractory (n=33) | P value |
---|---|---|---|
Current age, years, median (25% to 75%) | 23.55 (10.82–47.03) | 29.84 (13.80–52.77) | 0.395 |
Sex, females, n (%) | 67 (68.4) | 19 (57.6) | 0.259 |
Age at onset, years, median (25% to 75%) | 14.00 (3.42–40.75) | 24.00 (4.50–47.75) | 0.395 |
MGFA classification, n (%) | |||
I–II | 77 (78.6) | 19 (57.6) | 0.012* |
III–IV | 19 (19.4) | 9 (27.3) | |
V | 2 (2.0) | 5 (15.2) | |
Antibodies, n (%) | |||
AChR-Ab (+) | 87 (88.8) | 31 (93.9) | 0.602 |
Seronegative | 11 (11.2) | 2 (6.1) | |
QMG scores, years, median (25% to 75%) | 3.00 (2.00–8.00) | 5.50 (4.00–20.00) | <0.001* |
Medicine treatment, n (%) | |||
Pyri | 25 (25.5) | 4 (12.1) | 0.025* |
Pyri + Pred | 48 (49.0) | 12 (36.4) | |
Pyri + Pred + TAC | 11 (11.2) | 9 (27.3) | |
Pyri + Pred + MMF | 2 (2.0) | 1 (3.0) | |
Pyri + Pred + AZA | 1 (1.0) | 0 (0.0) | |
IVIG/PLEX | 2 (2.0) | 5 (15.2) | |
History of thymectomy, n (%) | 16 (16.3) | 11 (33.3) | 0.037* |
Thymus histology, n (%) | |||
Hyperplasia | 4 (4.1) | 1 (3.0) | 0.080 |
Thymoma | 8 (8.2) | 10 (30.3) | |
Thymolipoma | 3 (3.1) | 0 (0.0) | |
Normal | 1 (1.0) | 0 (0.0) | |
Follow-up, years, median (25% to 75%) | 3.32 (1.38–7.40) | 1.98 (1.56–6.13) | 0.280 |
MGFA-PIS at last visit, n (%) | |||
CSR + PR | 11 (11.2) | 0 (0.0) | <0.001* |
MM | 51 (52.0) | 1 (3.0) | |
Improvement | 27 (27.6) | 10 (30.3) | |
Unchanged | 2 (2.0) | 18 (54.5) | |
Worse | 3 (3.1) | 2 (6.1) | |
Exacerbation | 1 (1.0) | 0 (0.0) | |
Death | 3 (3.1) | 2 (6.1) |
*, P value <0.05. MGFA, Myasthenia Gravis Foundation of America; AChR-Ab, acetylcholine receptor antibody; QMG, quantified myasthenia gravis; Pyri, pyridostigmine; Pred, prednisone; TAC, tacrolimus; MMF, mycophenolate mofetil; AZA, azathioprine; IVIG, intravenous immunoglobulin; PLEX, plasma exchange; PIS, post-intervention status; CSR, complete stable remission; PR, pharmacologic remission; MM, minimal manifestations.
The distribution of SNPs in the MG patients
Results of multivariable logistic regression are presented in Table 3. CC genotype of the HSP90AA1 rs7160651 was associated with the increased risk of refractory MG than CT genotype [odds ratio (OR) =0.26; 95% confidence interval (CI): 0.07–0.94; P=0.041] and CT + TT genotype (dominant model, OR =0.24; 95% CI: 0.07–0.82; P=0.022). T-allele of HSP90AA1 rs7160651 frequency was less susceptible to refractory MG compared with C-allele (OR =0.31; 95% CI: 0.11–0.84; P=0.022). For CYP3A5 rs776746, AA genotype was associated with refractory MG compared with AG genotype (OR =0.11; 95% CI: 0.02–0.68; P=0.017), GG genotype (OR =0.18; 95% CI: 0.04–0.87; P=0.033), and AG + GG genotype (dominant model, OR =0.16; 95% CI: 0.03–0.75; P=0.020). There were no statistical differences in the other SNPs (Table S2).
Table 3
SNPs | Genotype (total =131) |
Non-refractory (n=98), n (%) | Refractory (n=33), n (%) |
χ2 | P valuea | Logistic regression | |
---|---|---|---|---|---|---|---|
OR (95% CIs) | P valueb | ||||||
HSP90AA1 (rs7160651) | CC | 56 (57.1%) | 27 (81.8%) | 7.016 | 0.030* | Reference | |
CT | 34 (34.7%) | 5 (15.2%) | 0.26 (0.07–0.94) | 0.041* | |||
TT | 8 (8.2%) | 1 (3.0%) | 0.19 (0.02–2.28) | 0.188 | |||
CT + TT | 42 (42.9%) | 6 (18.2%) | 6.475 | 0.011* | 0.24 (0.07–0.82) | 0.022* | |
C-allele | 146 (74.5%) | 59 (89.4%) | 6.443 | 0.011* | Reference | ||
T-allele | 50 (25.5%) | 7 (10.6) | 0.31 (0.11–0.84) | 0.022* | |||
CYP3A5 (rs776746) | AA | 5 (5.1%) | 6 (18.2%) | 5.211 | 0.074 | Reference | |
AG | 34 (34.7%) | 8 (24.2%) | 0.11 (0.02–0.68) | 0.017* | |||
GG | 59 (60.2%) | 19 (57.6%) | 0.18 (0.04–0.87) | 0.033* | |||
AG + GG | 93 (94.9%) | 27 (81.8%) | 3.922 | 0.048* | 0.16 (0.03–0.75) | 0.020* | |
A-allele | 44 (22.4%) | 20 (30.3%) | 1.650 | 0.199 | Reference | ||
G-allele | 152 (77.6%) | 46 (69.7%) | 0.58 (0.27–1.24) | 0.157 |
The allele frequency was calculated by dividing the number of alleles by twice the number of cases. a, P value for genotype frequencies using χ2 test; b, P value from multivariable logistic regression analysis after adjusting for age, sex, MGFA classification, QMG scores, medicine treatment, and history of thymectomy; *, P value <0.05. SNPs, single nucleotide polymorphisms; OR, odds ratio; CIs, confidence intervals; MGFA, Myasthenia Gravis Foundation of America; QMG, quantified myasthenia gravis.
Analysis of LD haplotypes
Two haplotype blocks were identified: Block 1 contained FKBP5 rs1360780 and rs4713904 (17 kb) and Block 2 contained HSP90AA1 rs7160651 and rs10873531 (4 kb). The results of LD analysis revealed strong linkages between FKBP5 rs1360780 and rs4713904 (D’ =0.96, r2=0.88) as well as HSP90AA1 rs10873531 and rs2298877 (D’ =1.00, r2=0.85). Figure 1 illustrates LD among SNPs.
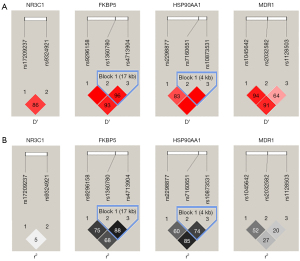
Considering LD of genes, haplotype analyses were further performed (Table 4). Haplotype analysis at rs10873531, rs2298877, rs7160651 of HSP90AA1 gene manifested that the refractory group had a lower CAT haplotype frequency (OR =0.33; 95% CI: 0.11–0.97; P=0.044).
Table 4
Haplotype | Frequencies n (%) | χ2 | P valuea | Logistic regression | ||
---|---|---|---|---|---|---|
Non-refractory (n=196) | Refractory (n=66) | OR (95% CIs) | P valueb | |||
NR3C1 (rs17209237, rs9324921) | ||||||
TT | 54 (27.6%) | 14 (21.2%) | 1.032 | 0.310 | 0.61 (0.27–1.34) | 0.217 |
TG | 108 (55.1%) | 40 (60.6%) | 0.609 | 0.435 | 1.77 (0.87–3.59) | 0.115 |
CT | 2 (1.0%) | 0 (0.0%) | 0.000 | 1.000 | – | – |
CG | 32 (16.3%) | 12 (18.2%) | 0.122 | 0.727 | 0.84 (0.32–2.23) | 0.726 |
KBP5 (rs1360780, rs4713904, rs9296158) | ||||||
GGC | 2 (1.0%) | 1 (1.5%) | 0.000 | 0.987 | 0.19 (0.01–2.87) | 0.232 |
GAT | 11 (5.6%) | 3 (4.5%) | 0.000 | 0.987 | 0.64 (0.14–2.83) | 0.554 |
GAC | 129 (65.8%) | 46 (69.7%) | 0.335 | 0.563 | 1.35 (0.64–2.83) | 0.428 |
AGT | 53 (27.0%) | 14 (21.2%) | 0.881 | 0.348 | 0.93 (0.40–2.14) | 0.861 |
AAT | 1 (0.5%) | 1 (1.5%) | 0.000 | 1.000 | 0.16 (0.01–20.76) | 0.464 |
GGT | 0 (0.0%) | 1 (1.5%) | 0.328 | 0.567 | – | – |
HSP90AA1 (rs10873531, rs2298877, rs7160651) | ||||||
TGC | 138 (70.4%) | 53 (80.3%) | 2.447 | 0.118 | 1.65 (0.73–3.74) | 0.226 |
CGT | 7 (3.6%) | 1 (1.5%) | 0.182 | 0.670 | 0.37 (0.04–3.43) | 0.379 |
CAT | 43 (21.9%) | 6 (9.1%) | 5.360 | 0.021 | 0.33 (0.11–0.97) | 0.044* |
CAC | 8 (4.1%) | 6 (9.1%) | 1.559 | 0.118 | 3.60 (0.98–13.26) | 0.054 |
MDR1 (rs1045642, rs1128503, rs2032582) | ||||||
TTT | 69 (35.2%) | 25 (37.9%) | 0.154 | 0.695 | 1.31 (0.65–2.61) | 0.449 |
TCG | 1 (0.5%) | 0 (0.0%) | 0.000 | 1.000 | – | – |
TCA | 2 (1.0%) | 0 (0.0%) | 0.000 | 0.995 | – | – |
CTT | 6 (3.1%) | 2 (3.0%) | 0.000 | 1.000 | 2.54 (0.31–2.23) | 0.382 |
CTG | 44 (22.4%) | 19 (28.8%) | 1.086 | 0.297 | 1.27 (0.59–2.74) | 0.549 |
CTA | 3 (1.5%) | 1 (1.5%) | 0.000 | 1.000 | – | – |
CCG | 44 (22.4%) | 10 (15.2%) | 1.067 | 0.205 | 0.42 (0.16–1.08) | 0.072 |
CCA | 27 (13.8%) | 9 (13.6%) | 0.001 | 0.977 | 1.28 (0.50–3.27) | 0.600 |
a, P value for genotype frequencies using χ2 test; b, P value from multivariable logistic regression analysis after adjusting for age, sex, MGFA classification, QMG scores, medicine treatment, and history of thymectomy; *, P value <0.05. OR, odds ratio; CIs, confidence intervals; MGFA, Myasthenia Gravis Foundation of America; QMG, quantified myasthenia gravis.
Discrimination of the predictive model
AUC values were calculated from the logistic regression model including clinical covariates together with genetic variants. As shown in Figure 2, CYP3A5 rs776746 and HSP90AA1 rs7160651 had good predictive accuracy (AUC >0.800). Among these models, the allele comparison model of HSP90AA1 rs7160651 achieves the best prediction performance with the highest AUC value of 0.852 (Figure 2E).
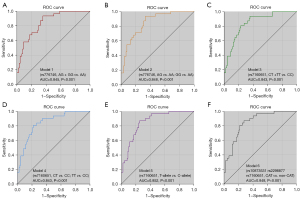
Gene-gene interactions
GMDR analysis showed that the single-locus model (rs7160651) was regarded as the optimal model based on the testing balanced accuracy, cross-validation consistency, and significant P values. Therefore, our results yielded no gene-related interactions for all possible one- to thirteen-locus models (Table 5).
Table 5
Interacting SNPs | Testing balanced accuracy | CVC | P value |
---|---|---|---|
rs7160651 | 0.626 | 10/10 | 0.011* |
rs2032582*rs7160651 | 0.502 | 5/10 | 0.828 |
rs2032582*rs9296158*rs7160651 | 0.590 | 5/10 | 0.055 |
rs2032582*rs17209237*rs9296158*rs7160651 | 0.466 | 4/10 | 0.623 |
rs2032582*rs2242480*rs17209237*rs9296158*rs10873531 | – | 5/10 | 0.377 |
rs1128503*rs2032582*rs2242480*rs17209237*rs9296158*rs7160651 | – | 5/10 | 0.172 |
rs1128503*rs2032582* rs776746*rs2242480* rs9324921* rs4713904*rs10873531 | – | 5/10 | 0.828 |
rs1045642*rs1128503*rs2032582*rs776746*rs2242480*rs17209237*rs9324921* rs9296158 | – | 2/10 | 0.999 |
rs1045642*rs1128503*rs2032582*rs776746*rs2242480*rs17209237*rs9324921*rs9296158*rs10873531 | – | 4/10 | 0.989 |
rs1045642*rs1128503*rs2032582*rs776746*rs2242480*rs17209237*rs9324921*rs1360780*rs9296158*rs10873531 | – | 10/10 | 0.945 |
rs1045642*rs1128503*rs2032582*rs776746*rs2242480*rs17209237*rs9324921*rs1360780*rs4713904*rs9296158*rs10873531 | – | 9/10 | 0.945 |
rs1045642*rs1128503*rs2032582*rs776746*rs2242480*rs17209237*rs9324921*rs1360780*rs4713904*rs9296158*rs10873531*rs2298877 | – | 10/10 | 0.945 |
rs1045642*rs1128503*rs2032582*rs776746*rs2242480*rs17209237*rs9324921*rs1360780*rs4713904*rs9296158*rs10873531*rs2298877*rs7160651 | – | 10/10 | 0.945 |
P value was calculated with covariate adjustment for age, sex, MGFA classification, QMG scores, medicine treatment, and history of thymectomy. *, P value <0.05. GMDR, generalized multifactor dimensionality reduction; SNPs, single nucleotide polymorphisms; CVC, cross validation consistency; MGFA, Myasthenia Gravis Foundation of America; QMG, quantified myasthenia gravis.
Discussion
In the current study, we have evaluated 13 SNPs for the treatment response in 131 patients with MG. After adjusting for possible confounding factors by logistic regression, genetic polymorphisms of CYP3A5 rs776746 and HSP90AA1 rs7160651 showed statistically significant associations with the risk of refractory MG. No significant SNP pairs were found in the gene-gene interaction analyses.
HSP90AA1 is efficiently expressed under pathological conditions, such as tumors, infection, and autoimmune disorders (9,22). A previous study has found that HSP90AA1 is closely associated with resistance to cancer therapy by inhibiting apoptosis and inducing autophagy (23). But the relationship between autophagy and therapy resistance in MG remains unclear and needs further investigation. In the present study, rs7160651 in HSP90AA1 was significantly associated with the response to immunosuppressive therapy under a dominant genetic model, which was consistent with the previous report (8). Furthermore, we found that HSP90AA1 CAT haplotype and T-allele were associated with a lower risk of refractory MG. The protein encoded by the HSP90AA1 gene is the cytoplasmic/nuclear form of heat shock protein 90 (HSP90) (24). Ouyang et al. demonstrated that the increased presence of HSP90 in the nucleus could hinder DNA-binding and transcriptional activity of GR, which might lead to steroid resistance in patients with idiopathic nephrotic syndrome (25). However, the functional significance of the polymorphisms in HSP90AA1 is not fully understood, thus how HSP90AA1 genetic polymorphisms influence the treatment response of MG remains to be determined.
CYP3A5 protein is polymorphically expressed in the liver and intestines and impacts the drug disposition (26,27). GCs (28) and tacrolimus (29) are the most frequently used pharmacological agents for our cohort, which are both metabolized by CYP3A5 enzyme. The A to G substitution at rs776746 polymorphism is responsible for aberrantly spliced transcript and low protein expression of CYP3A5 in many populations (30). Consistent with the previous study (29), our results demonstrated an association between the dominant model of CYP3A5 rs776746 polymorphism and poor response to therapy. Therefore, we speculate that rs776746 variation significantly affects drug disposal and may play a predictive role in the refractory status of MG.
Non-genetic factors related to refractory MG were used as covariates to correct the disease model. A recent study showed that patients with drug-refractory MG were more frequently younger at onset, females, and thymectomized (14). However, age at onset and sex were not significantly different between refractory and non-refractory MG in our current study. Childhood-onset MG mostly occurs in women with a high rate of spontaneous remission, which accounts for 10–20% of all MG patients in western countries, but more than 50% in Asians (31,32). Differences in the age distribution between Asian and Western populations could be one possible reason for the different clinical characteristics across studies. We demonstrated that the patients in the refractory group were more often treated with thymectomy but did not achieve symptom remission. The ineffectiveness of thymectomy can be attributed to circulating plasma cells that are often long-lived and can secrete antibodies in the absence of T cells (33).
MG is a multifactorial disease involving complex gene-gene and gene-environment interactions (34-36). No gene-gene interactions were identified among possible SNP combinations in GMDR analysis, but we cannot exclude the possibility of other multiple interactions that influence the therapeutic effects of MG. Several investigations suggested that MDR1, NR3C1, and FKBP5 polymorphisms may confer resistance to immunosuppressive therapy (37-39). The present study did not find any association between their genotypes and refractory MG. Further work targeting more diverse genotypes will be necessary.
Limitations exist for this study. Firstly, the present study is a single-center retrospective study with small-sample data. Secondly, the relationship between genetic polymorphisms and the effects of different drugs or different drug combinations used by the participants is unclear. Thirdly, the current study spreads over a large age range. Finally, we did not correct for multiple testing as the present study is exploratory.
Conclusions
This article is the first to explore the association between polymorphisms of drug-metabolizing genes and refractory MG. The results suggested that variants of CYP3A5 rs776746 and HSP90AA1 rs7160651 were clinical risk factors for the refractory status of MG. Drugs that are not metabolized by CYP3A5 and HSP90AA1 might be an option for refractory MG with rs776746 AA genotype and rs7160651 CC genotype, respectively. Further studies are required to confirm these findings and their clinical applications.
Acknowledgments
Funding: This study was supported by a grant from the National Natural Science Foundation of China (No. 81873758 to Bitao Bu).
Footnote
Reporting Checklist: The authors have completed the TRIPOD reporting checklist. Available at https://atm.amegroups.com/article/view/10.21037/atm-22-2543/rc
Data Sharing Statement: Available at https://atm.amegroups.com/article/view/10.21037/atm-22-2543/dss
Peer Review File: Available at https://atm.amegroups.com/article/view/10.21037/atm-22-2543/prf
Conflicts of Interest: All authors have completed the ICMJE uniform disclosure form (available at https://atm.amegroups.com/article/view/10.21037/atm-22-2543/coif). BB reports that study was supported by a grant from the National Natural Science Foundation of China (No. 81873758). The other authors have no conflicts of interest to declare.
Ethical Statement: The authors are accountable for all aspects of the work in ensuring that questions related to the accuracy or integrity of any part of the work are appropriately investigated and resolved. The study was conducted in accordance with the Declaration of Helsinki (as revised in 2013) and approved by the Ethical Committee of Tongji Hospital, Tongji Medical College, Huazhong University of Science and Technology (protocol ID: TJ-IRB20191208). Informed consents were obtained from all individual participants or their legal guardians.
Open Access Statement: This is an Open Access article distributed in accordance with the Creative Commons Attribution-NonCommercial-NoDerivs 4.0 International License (CC BY-NC-ND 4.0), which permits the non-commercial replication and distribution of the article with the strict proviso that no changes or edits are made and the original work is properly cited (including links to both the formal publication through the relevant DOI and the license). See: https://creativecommons.org/licenses/by-nc-nd/4.0/.
References
- Narayanaswami P, Sanders DB, Wolfe G, et al. International Consensus Guidance for Management of Myasthenia Gravis: 2020 Update. Neurology 2021;96:114-22. [Crossref] [PubMed]
- Lascano AM, Lalive PH. Update in immunosuppressive therapy of myasthenia gravis. Autoimmun Rev 2021;20:102712. [Crossref] [PubMed]
- Schneider-Gold C, Hagenacker T, Melzer N, et al. Understanding the burden of refractory myasthenia gravis. Ther Adv Neurol Disord 2019;12:1756286419832242. [Crossref] [PubMed]
- Tran C, Biswas A, Mendoza M, et al. Performance of different criteria for refractory myasthenia gravis. Eur J Neurol 2021;28:1375-84. [Crossref] [PubMed]
- Ahmed S, Zhou Z, Zhou J, et al. Pharmacogenomics of Drug Metabolizing Enzymes and Transporters: Relevance to Precision Medicine. Genomics Proteomics Bioinformatics 2016;14:298-313. [Crossref] [PubMed]
- Xie Y, Meng Y, Li HF, et al. GR gene polymorphism is associated with inter-subject variability in response to glucocorticoids in patients with myasthenia gravis. Eur J Neurol 2016;23:1372-9. [Crossref] [PubMed]
- Li L, Lou Z, Wang L. The role of FKBP5 in cancer aetiology and chemoresistance. Br J Cancer 2011;104:19-23. [Crossref] [PubMed]
- Zou YF, Xu JH, Gu YY, et al. Single nucleotide polymorphisms of HSP90AA1 gene influence response of SLE patients to glucocorticoids treatment. Springerplus 2016;5:222. [Crossref] [PubMed]
- Zuehlke AD, Beebe K, Neckers L, et al. Regulation and function of the human HSP90AA1 gene. Gene 2015;570:8-16. [Crossref] [PubMed]
- Zhou Z, Xiong L, Wu Z, et al. Nkx2.8 promotes chemosensitivity in bladder urothelial carcinoma via transcriptional repression of MDR1. Cell Death Dis 2022;13:492. [Crossref] [PubMed]
- Parvin MN, Aziz MA, Rabbi SNI, et al. Assessment of the Link of ABCB1 and NR3C1 gene polymorphisms with the prednisolone resistance in pediatric nephrotic syndrome patients of Bangladesh: A genotype and haplotype approach. J Adv Res 2021;33:141-51. [Crossref] [PubMed]
- Chen D, Hou S, Zhao M, et al. Dose optimization of tacrolimus with therapeutic drug monitoring and CYP3A5 polymorphism in patients with myasthenia gravis. Eur J Neurol 2018;25:1049-e80. [Crossref] [PubMed]
- Peng Y, Jiang F, Zhou R, et al. Clinical Evaluation of the Efficacy and Safety of Co-Administration of Wuzhi Capsule and Tacrolimus in Adult Chinese Patients with Myasthenia Gravis. Neuropsychiatr Dis Treat 2021;17:2281-9. [Crossref] [PubMed]
- Cortés-Vicente E, Álvarez-Velasco R, Pla-Junca F, et al. Drug-refractory myasthenia gravis: Clinical characteristics, treatments, and outcome. Ann Clin Transl Neurol 2022;9:122-31. [Crossref] [PubMed]
- Fan Z, Li Z, Shen F, et al. Favorable Effects of Tacrolimus Monotherapy on Myasthenia Gravis Patients. Front Neurol 2020;11:594152. [Crossref] [PubMed]
- Mantegazza R, Antozzi C. When myasthenia gravis is deemed refractory: clinical signposts and treatment strategies. Ther Adv Neurol Disord 2018;11:1756285617749134. [Crossref] [PubMed]
- Barohn RJ. Standards of measurements in myasthenia gravis. Ann N Y Acad Sci 2003;998:432-9. [Crossref] [PubMed]
- de Meel RHP, Verschuuren JJGM, Tannemaat MR. Distinct representation of muscle weakness in QMG and MG-ADL. Lancet Neurol 2018;17:204-5. [Crossref] [PubMed]
- Fan HH, Li BQ, Wu KY, et al. Polymorphisms of Cytochromes P450 and Glutathione S-Transferases Synergistically Modulate Risk for Parkinson’s Disease. Front Aging Neurosci 2022;14:888942. [Crossref] [PubMed]
- El Hou A, Rocha D, Venot E, et al. Long-range linkage disequilibrium in French beef cattle breeds. Genet Sel Evol 2021;53:63. [Crossref] [PubMed]
- Wei BL, Yin RX, Liu CX, et al. CYP17A1-ATP2B1 SNPs and Gene-Gene and Gene-Environment Interactions on Essential Hypertension. Front Cardiovasc Med 2021;8:720884. [Crossref] [PubMed]
- Liu A, Shi FD, Cohen IR, et al. DNA vaccine encoding heat shock protein 90 protects from murine lupus. Arthritis Res Ther 2020;22:152. [Crossref] [PubMed]
- Xiao X, Wang W, Li Y, et al. HSP90AA1-mediated autophagy promotes drug resistance in osteosarcoma. J Exp Clin Cancer Res 2018;37:201. [Crossref] [PubMed]
- Morishima Y, Mehta RK, Yoshimura M, et al. Chaperone Activity and Dimerization Properties of Hsp90α and Hsp90β in Glucocorticoid Receptor Activation by the Multiprotein Hsp90/Hsp70-Dependent Chaperone Machinery. Mol Pharmacol 2018;94:984-91. [Crossref] [PubMed]
- Ouyang J, Chen P, Jiang T, et al. Nuclear HSP90 regulates the glucocorticoid responsiveness of PBMCs in patients with idiopathic nephrotic syndrome. Int Immunopharmacol 2012;14:334-40. [Crossref] [PubMed]
- Williams ML, Kannankeril PJ, Breeyear JH, et al. Effect of CYP3A5 and CYP3A4 Genetic Variants on Fentanyl Pharmacokinetics in a Pediatric Population. Clin Pharmacol Ther 2022;111:896-908. [Crossref] [PubMed]
- Dagli-Hernandez C, de Freitas RCC, Marçal EDSR, et al. Late response to rosuvastatin and statin-related myalgia due to SLCO1B1, SLCO1B3, ABCB11, and CYP3A5 variants in a patient with Familial Hypercholesterolemia: a case report. Ann Transl Med 2021;9:76. [Crossref] [PubMed]
- Gabryel M, Skrzypczak-Zielinska M, Kucharski MA, et al. The impact of genetic factors on response to glucocorticoids therapy in IBD. Scand J Gastroenterol 2016;51:654-65. [Crossref] [PubMed]
- Cargnin S, Ravegnini G, Soverini S, et al. Impact of SLC22A1 and CYP3A5 genotypes on imatinib response in chronic myeloid leukemia: A systematic review and meta-analysis. Pharmacol Res 2018;131:244-54. [Crossref] [PubMed]
- Rodriguez-Antona C, Savieo JL, Lauschke VM, et al. PharmVar GeneFocus: CYP3A5. Clin Pharmacol Ther 2022; Epub ahead of print. [Crossref] [PubMed]
- Gui M, Luo X, Lin J, et al. Long-term outcome of 424 childhood-onset myasthenia gravis patients. J Neurol 2015;262:823-30. [Crossref] [PubMed]
- VanderPluym J, Vajsar J, Jacob FD, et al. Clinical characteristics of pediatric myasthenia: a surveillance study. Pediatrics 2013;132:e939-44. [Crossref] [PubMed]
- Lisak RP, Richman DP. Thymectomy and myasthenia gravis. Proc Natl Acad Sci U S A 2020;117:32195-6. [Crossref] [PubMed]
- Bo C, Zhang H, Cao Y, et al. Construction of a TF-miRNA-gene feed-forward loop network predicts biomarkers and potential drugs for myasthenia gravis. Sci Rep 2021;11:2416. [Crossref] [PubMed]
- Alboini PE, Spagni G, Evoli A. In sickness and in health: when myasthenia gravis is a conjugal matter. Neurol Sci 2021;42:2099-101. [Crossref] [PubMed]
- Zhou Y, Kaminski HJ, Gong B, et al. RNA expression analysis of passive transfer myasthenia supports extraocular muscle as a unique immunological environment. Invest Ophthalmol Vis Sci 2014;55:4348-59. [Crossref] [PubMed]
- Maltese P, Palma L, Sfara C, et al. Glucocorticoid resistance in Crohn’s disease and ulcerative colitis: an association study investigating GR and FKBP5 gene polymorphisms. Pharmacogenomics J 2012;12:432-8. [Crossref] [PubMed]
- Aziz MA, Islam MS. The role of ABCB1 gene polymorphisms in steroid-resistant nephrotic syndrome: Evidence from a meta-analysis of steroid-receiving patients. J Gene Med 2022;24:e3436. [Crossref] [PubMed]
- Hamadeh IS, Zhang Q, Steuerwald N, et al. Effect of CYP3A4, CYP3A5, and ABCB1 Polymorphisms on Intravenous Tacrolimus Exposure and Adverse Events in Adult Allogeneic Stem Cell Transplant Patients. Biol Blood Marrow Transplant 2019;25:656-63. [Crossref] [PubMed]