Persistent dysregulation of genes in the development of endometriosis
Introduction
Endometriosis is a chronic and often undiagnosed disease affecting 5–10% of women of child-bearing age. It is characterized by the growth of extrauterine endometrial tissue, most commonly in the abdominal cavity (1), and can manifest as pelvic pain, heterotopia, dysuria, dysmenorrhea, and infertility (2). Unfortunately, for endometriosis and other diseases with similar symptoms, the overall sensitivity and specificity of transvaginal ultrasonography (TVUS) ranges from 53% to 93% (3). At present, there is no reliable marker to treat this disease (4).
The pathophysiology of endometriosis is complex and not fully understood (5). Study showed that endometriosis results from a combination of anatomical, hormonal, immune, response, oestrogen, genetic, epigenetic and environmental factors in affected women (6). There is increasing evidence that hormones and immune factors may create a microenvironment conducive to inflammation, thereby promoting the persistence of endometriosis (7). The molecular studies have been supported by clinical observations of disease resolution, symptom relief, and so-called “treatment” of endometriosis response to anti-inflammatory drugs (8). However, due to the lack of reliable diagnostic tools and nonspecific symptoms, diagnosis of endometriosis can be delayed by up to 8–10 years. This results in a heavy economic burden as the chronic and debilitating pain caused by endometriosis may hinder work efficiency, while infertility can lead to serious psychological and economic pressures on the affected women and their partners (9).
The American Society of Reproductive Medicine (ASRM) guidelines classify endometriosis into 4 stages using a scoring system, namely stage I, II, III, and IV, which correspond to minimal, mild, moderate, and severe endometriosis, respectively (10). While the mechanisms of malignant transformation of endometriosis have not been fully elucidated, oxidative stress, inflammation, and changes in the hormone environment are all plausible pathogenic factors (11). Hormone levels, especially estrogen, plays an important regulatory role in the deterioration of endometriosis (12). In addition, some high throughput studies have found a role for certain candidate genes in the pathogenesis of endometriosis (13-15). Currently, there is no cure for endometriosis and existing drugs can only help to reduce the symptoms of the disease, with a recurrence rate of 35–50%. Therefore, it is necessary to develop diagnostic indicators and potential treatments for the management of patients with endometriosis (16).
An increasingly accepted view is that diseases can be considered a disturbance to a genetic network (17). The protein-protein interaction (PPI) with known disease genes have been used to identify new disease genes (18). This current study screened and identified potential biomarkers for endometriosis with different severity and further examined the molecular mechanisms of endometriosis. The following article is presented in accordance with the STREGA reporting checklist (available at https://atm.amegroups.com/article/view/10.21037/atm-22-4806/rc).
Methods
Data source and difference analysis
The GSE51981 dataset for endometriosis was obtained from the Gene Expression Omnibus (GEO) database and analyzed. The dataset included 34 control samples, 28 samples from patients with mid endometriosis, and 49 samples from patients with severe disease. The study was conducted in accordance with the Declaration of Helsinki (as revised in 2013). The original data from GSE51981 was standardized with the RMA function of the Affy R package to construct the gene expression profile. The differential expression between the mild and control cases, as well as the severe and mild cases, were constructed using the limma R package. The filter threshold was set to a P value <0.05. The Short Time Series Expression Miner (STEM) (v1.3.11) software was used to cluster the differentially expressed genes (DEGs) to screen out the genes with persistent expression disorder (19). Then, DEGs between early-secretory and proliferation, or between mid-secretory and early-secretory were identified using limma R package.
PPI network and decision curve analysis (DCA)
The PPI network was constructed by mapping the genes with continuous expression disorder into the String database (20). The network was visualized using the Cytoscape (3.6.1) software. The important modules in the PPI network were determined using the Molecular Complex Detection (MCODE) plug-in (21), where K-score =5 was used as the standard of MCODE analysis. The R-package circlize was used to show the differences in the genes from each module. Finally, the top 5 genes were selected as the core genes using the Betweenness algorithm of the CytoHubba plug-in.
Furthermore, DCA curve (22) was used to evaluate the diagnostic roles for core genes in GSE51981.
Enrichment analysis
The Gene Ontology (GO) and Kyoto Encyclopedia of Gene and Genome (KEGG) pathways were identified using the clusterProfiler R package (23). The significance level of enrichment of GO and KEGG pathways was set at P<0.05. In addition, the Gene Set Variation Analysis (GSVA) R software package was used to determine the most relevant pathway of persistent maladjustment genes (24).
Regulator prediction
The data of transcriptional target regulation relationship was obtained from the RAID v2.0 database (25). Under the background of transcription factor pairing data, the P value was set to <0.01. The regulatory gene network was then displayed using the Cytoscape software.
The DGIdb (https://www.dgidb.org/) database was used to predict the relationship between core genes and drugs.
Immune levels in endometriosis
Single-sample Gene Set Enrichment Analysis (ssGSEA) was used to calculate the levels of 24 immune cell types between the mild and control cases, or between the severe and mild in GSE51981. Correlation analysis to further show the core genes correlated with immune cells.
Statistical analysis
Using bioinforcloud platform (http://www.bioinforcloud.org.cn) to analyze the all methods in this study. The P value <0.05 was considered statistical significance.
Results
Persistent dysregulated genes involved in endometriosis
The normal, mild, and severe endometriosis samples in the GSE51981 dataset were examined using principal component analysis (PCA). The outlier level between the disease and the control samples was higher, and that of the mild and severe cases were close (Figure 1A). A total of 16,453 DEGs were identified between mild endometriosis and normal samples (table available at https://cdn.amegroups.cn/static/public/10.21037atm-22-4806-1.xlsx, Figure 1B), while 7,793 DEGs were found between severe and mild endometriosis (table available at https://cdn.amegroups.cn/static/public/10.21037atm-22-4806-2.xlsx). Among them, 6,768 genes were common in the 2 groups of DEGs (Figure 1C). To identify the expression trend of these common genes, 3,472 genes were obtained through STEM clustering and shown to be continuously upregulated or downregulated in the progression from normal to mild and then from mild to severe endometriosis, suggesting that these DEGs are persistent dysfunctional genes in the progression of endometriosis. Four significant clustering modules were further identified (Figure 1D,1E), and these may be associated with the continuous deterioration of endometriosis (tables available at https://cdn.amegroups.cn/static/public/10.21037atm-22-4806-1.xlsx and https://cdn.amegroups.cn/static/public/10.21037atm-22-4806-2.xlsx, Figure 1).
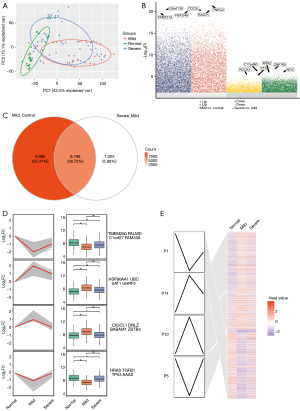
Identification of key disordered genes
To identify the key factors associated with the continued deterioration of endometriosis, 3,472 genes were mapped into the PPI network (Figure 2A). A total of 466 genes and 11,211 interacting pairs were obtained, and 12 modules were identified (Figure 2B,2C). Correlation analysis showed that modules 6, 7, and 9 were significantly correlated with normal and disease state, and module genes may be related to the occurrence and development of disease (Figure 2D).
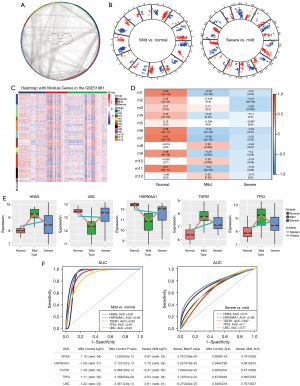
The top 5 genes were considered core genes, including HRAS, HSP90AA1, TGFB1, TP53, and UBC. Among them, HRAS, TGFB1, and TP53 were upregulated in the process of endometriosis deterioration, while HSP90AA1 and UBC were downregulated (Figure 2E). Their expression trend was consistent with the continuous up-down relationship between the STEM box chart and the heat chart. In addition, using receiver operating characteristic (ROC) curve analyses, the area under the curve (AUC) values for mild and severe endometriosis cases revealed that these genes had good clinical diagnostic ability (Figure 2F). Using the DCA analysis to assess the diagnostic performance, we found the higher net benefit in the mild endometriosis (Figure 3A) and severe endometriosis (Figure 3B), thus with better diagnostic role for endometriosis.
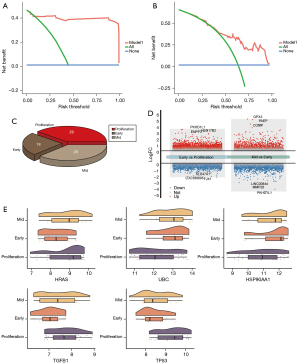
In addition, we found there were 28, 18, and 29 samples of endometriosis in proliferation, early-secretory, and mid-secretory, respectively (Figure 3C). The difference analysis results showed that there were10,499 DEGs between early-secretory and proliferation, and 7,086 DEGs between mid-secretory and early-secretory (Figure 3D). Among these, we found core genes were also differed significantly (Figure 3E).
Molecular mechanism of endometriosis
To identify the molecular mechanisms of these DEGs, the 466 genes were analyzed using GO and KEGG enrichment. A total of 3,197 biological processes (BP), 401 cell components (CC), 508 molecular functions (MF), and 126 KEGG signaling pathways were screened. Among them, module 6 genes were significantly related to oxygen levels, module 7 genes were significantly related to metallic process, and module 9 genes were significantly related to hormone levels (Figure 4A,4B).
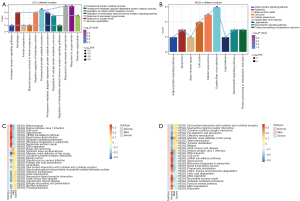
Through GSVA, we calculated the top changes of KEGG pathways in normal, mild, and severe endometriosis (Figure 4C,4D). In the upregulated pathway, the changes of olfactory transduction, taste transduction, autoimmune thyroid disease, and nicotine addiction were more obvious. Furthermore, the changes in Fanconi anemia pathway, and ribosome and DNA replication were more obvious (Figure 4).
Transcriptional regulators associated with the core disordered genes
To investigate the pathogenesis of endometriosis, the transcription factors that regulate the core genes were predicted. A total of 9 transcription factors were found to regulate the core genes. Among them, JUND and TGFβ1, STAT1 and HSP90AA1, and TP53 and HRAS were highly correlated, and thus, JUND, STAT1, and TP53 were considered key regulators (Figure 5A). TP53 was also one of the core genes. These transcription factors that regulate the core genes were found to be involved in many signaling pathways, including oxidative stress, hormone regulation, and inflammation related signaling pathways (Figure 5B), as well as playing a key role in the disease progression of endometriosis. In addition, drug prediction analyses using the DGIdb database identified 792 drugs that target the core genes (table available at https://cdn.amegroups.cn/static/public/10.21037atm-22-4806-3.xlsx, Figure 5).
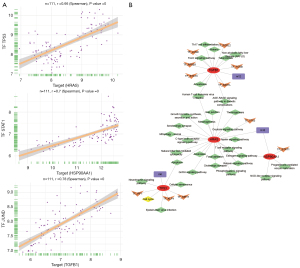
Immune analysis
Through ssGSEA, we found that Th2, T helper cells, and central memory T cells (Tcm) were significantly decreased in mild compared with normal (Figure 6A), while significantly elevated in severe compared with mild (Figure 6B). The follicular helper T cells (Tfh) and immature dendritic cell (iDC) were significantly elevated in mild compared with normal, and significantly decreased in severe compared with mild. Correlation results showed that T helper cells and natural killer (NK) cells had the biggest correlation with core genes in both mild (Figure 6C) and severe (Figure 6D) endometriosis. Interestingly, there was a completely opposite correlation between NK and core genes, and between T helper cells and core genes.
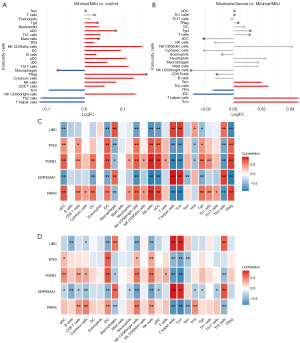
Discussion
The implantation and growth of endometrial cells is the main cause of endometriosis. Endometriosis exerts a significant social and psychological burden on women in many respects, including quality of life, intimacy, childbearing, education and work, and emotional health (26). Furthermore, endometriosis is often related to cardiovascular and autoimmune diseases (27). Recent advances in the field of endometriosis have identified novel biomarkers that may be developed as targets for the treatment of endometriosis, including immunomodulators and inhibitors, which have been tested on animal models (28). This current study identified and examined the molecular targets and mechanisms involved in the progression of endometriosis. This data will contribute to the diagnosis, treatment, and management of patients with endometriosis.
The continuous disordered expression of certain genes is likely to be involved in the development of endometriosis and may be potential therapeutic targets. This study screened and identified a series of persistent maladjustment genes. Among them, HRAS, HSP90AA1, TGFB1, TP53, and UBC were identified as core factors. Previous studies have shown that HRAS is significantly related to the occurrence and development of endometriosis (29). The HRAS proto-oncogene and GTPase (HRAS) can regulate the level of ferritin, thus increasing the pool of unstable iron and the proliferation index in cells (30). In addition, HRAS is related to the chemical family of polychlorinated biphenyls (PCBs), and PCB exposure is closely related to the development of endometriosis (31). Interestingly, the expression of the HSP90AA1 gene differs significantly between severe and mild endometriosis (32). TGF-β plays an important role in the pathogenesis of peritoneal endometriosis (32). Furthermore, it is differentially expressed in patients with endometriosis, and may promote invasion, differentiation, angiogenesis, and immune escape (33). The expression of TGF-β1 and its signal targets in the eutopic endometrium of patients with endometriosis is different to that in healthy women (34). Compared with normal endometrium, tumor protein p53 (TP53) is more frequently detected in endometriosis tissues (35). TP53 is believed to be a transcriptional regulator of HRAS and may play a more important role in the development of endometriosis. Therefore, these core genes that are dysregulated in endometriosis are more likely to be potential therapeutic targets. This may pave the way for improved early diagnosis and more effective treatment of endometriosis by using the unique and persistent biomarkers identified herein.
There is evidence that the destruction of female hormones, local inflammation, and the immune process are involved in the occurrence and development of endometriosis (36). In this study, we identified the effects of oxidative stress, hormones, and immune inflammation on endometriosis. Hypoxia plays an important role in the development of endometriosis (37). The invasive mechanism of endometriosis may be related to oxidative stress-related proliferative activity (4). In addition, the tissue niche provides a chronic inflammatory environment for the development of endometriosis, especially in the abdominal cavity, ovary, and uterus (38). Inflammation may lead to increased expression of mitogen activated protein kinase (MAPK) and other signal pathway components in endometriosis (39). Many changes occur in the immune system during endometriosis, including inhibition of T cell cytotoxicity to endometrial cells, reduction of NK cell activity, and increase of activated macrophages and pro-inflammatory cytokines (40). The occurrence and maintenance of endometriosis can be divided into two stages: the initial stage of interleukin 6 (IL-6)-mediated early immunity, and the hormone dominated stage of estrogen signal (41). Indeed, these factors may have a comprehensive impact on the occurrence and development of endometriosis.
The markers of endometriosis have a strong interaction with inflammatory components and the hormone environment, which has a negative impact on its expression and function, so as to promote the sustainable development of endometriosis. We then found high levels of immune cells in the endometriosis. NK cells and dendritic cells, may play specific roles in angiogenesis, growth and invasion of endometriosis cells (42). Podgaec et al., showed that the inflammatory symptoms of endometriosis may involve a shift towards Th2 immune response components (43). The dysregulation of immune cells showed no progressive trend in the course of endometriosis. Although the reason for this phenomenon is unknown, it is suggested that core genes improve the immune response of endometriosis through multiple pathways. This may provide a new ideas and directions for the diagnosis and treatment of endometriosis.
However, this study also has limitations. First, the data used in this study were obtained from public databases, and more clinical information on patients may be missing. Second, for the significant analytical results we obtained, further in vivo and in vitro experimental validation is warranted. In addition, we should expand the sample size in future studies to enhance the clinical applicability of the results. The method of screening modules and core genes through the PPI network was universal and may lack individualization. Further research using a large number of clinical samples are needed to verify our results.
Conclusions
Endometriosis is a debilitating disease that affects the quality of life of adults and adolescents. Delays in diagnosis are common and can lead to a decline in reproductive potential and fertility. Recognizing disease progression and deterioration may help to improve therapeutic treatments and outcomes. These potential biomarkers may reduce the cost of surgical intervention through early diagnosis of cases, so as to improve the clinical management of the disease.
Acknowledgments
Funding: This work was supported by the Liuzhou City Science and Technology Research Projects (Nos. 2021YB0104A003 and 2021YB0104B020) and the Scientific Research Fund of the Health and Family Planning Commission of Guangxi Zhuang Autonomous Region (Nos. Z2016795 and Z20211443).
Footnote
Reporting Checklist: The authors have completed the STREGA reporting checklist. Available at https://atm.amegroups.com/article/view/10.21037/atm-22-4806/rc
Conflicts of Interest: All authors have completed the ICMJE uniform disclosure form (available at https://atm.amegroups.com/article/view/10.21037/atm-22-4806/coif). The authors have no conflicts of interest to declare.
Ethical Statement: The authors are accountable for all aspects of the work, including ensuring that any questions related to the accuracy or integrity of any part of the work have been appropriately investigated and resolved. The study was conducted in accordance with the Declaration of Helsinki (as revised in 2013).
Open Access Statement: This is an Open Access article distributed in accordance with the Creative Commons Attribution-NonCommercial-NoDerivs 4.0 International License (CC BY-NC-ND 4.0), which permits the non-commercial replication and distribution of the article with the strict proviso that no changes or edits are made and the original work is properly cited (including links to both the formal publication through the relevant DOI and the license). See: https://creativecommons.org/licenses/by-nc-nd/4.0/.
References
- Bendifallah S, Puchar A, Suisse S, et al. Machine learning algorithms as new screening approach for patients with endometriosis. Sci Rep 2022;12:639. [Crossref] [PubMed]
- Missmer SA, Tu F, Soliman AM, et al. Impact of endometriosis on women's life decisions and goal attainment: a cross-sectional survey of members of an online patient community. BMJ Open 2022;12:e052765. [Crossref] [PubMed]
- Guerriero S, Ajossa S, Minguez JA, et al. Accuracy of transvaginal ultrasound for diagnosis of deep endometriosis in uterosacral ligaments, rectovaginal septum, vagina and bladder: systematic review and meta-analysis. Ultrasound Obstet Gynecol 2015;46:534-45. [Crossref] [PubMed]
- Rolla E. Endometriosis: advances and controversies in classification, pathogenesis, diagnosis, and treatment. F1000Res 2019;8:eF1000 Faculty Rev-529.
- Gemmell LC, Webster KE, Kirtley S, et al. The management of menopause in women with a history of endometriosis: a systematic review. Hum Reprod Update 2017;23:481-500. [Crossref] [PubMed]
- Laganà AS, Garzon S, Götte M, et al. The Pathogenesis of Endometriosis: Molecular and Cell Biology Insights. Int J Mol Sci 2019;20:5615. [Crossref] [PubMed]
- Smolarz B, Szyłło K, Romanowicz H. Endometriosis: Epidemiology, Classification, Pathogenesis, Treatment and Genetics (Review of Literature). Int J Mol Sci 2021;22:10554. [Crossref] [PubMed]
- Inceboz U. Endometriosis after menopause. Womens Health (Lond) 2015;11:711-5. [Crossref] [PubMed]
- Simoens S, Dunselman G, Dirksen C, et al. The burden of endometriosis: costs and quality of life of women with endometriosis and treated in referral centres. Hum Reprod 2012;27:1292-9. [Crossref] [PubMed]
- Revised American Society for Reproductive Medicine classification of endometriosis: 1996. Fertil Steril 1997;67:817-21. [Crossref] [PubMed]
- Brichant G, Laraki I, Henry L, et al. New Therapeutics in Endometriosis: A Review of Hormonal, Non-Hormonal, and Non-Coding RNA Treatments. Int J Mol Sci 2021;22:10498. [Crossref] [PubMed]
- Wang CT, Wang DB, Liu KR, et al. Inducing malignant transformation of endometriosis in rats by long-term sustaining hyperestrogenemia and type II diabetes. Cancer Sci 2015;106:43-50. [Crossref] [PubMed]
- Sapkota Y, Low SK, Attia J, et al. Association between endometriosis and the interleukin 1A (IL1A) locus. Hum Reprod 2015;30:239-48. [Crossref] [PubMed]
- Babayev SN, Park CW, Keller PW, et al. Androgens Upregulate Endometrial Epithelial Progesterone Receptor Expression: Potential Implications for Endometriosis. Reprod Sci 2017;24:1454-61. [Crossref] [PubMed]
- Chatterjee K, Jana S, DasMahapatra P, et al. EGFR-mediated matrix metalloproteinase-7 up-regulation promotes epithelial-mesenchymal transition via ERK1-AP1 axis during ovarian endometriosis progression. FASEB J 2018;32:4560-72. [Crossref] [PubMed]
- Eisenberg VH, Weil C, Chodick G, et al. Epidemiology of endometriosis: a large population-based database study from a healthcare provider with 2 million members. BJOG 2018;125:55-62. [Crossref] [PubMed]
- Wang X, Gulbahce N, Yu H. Network-based methods for human disease gene prediction. Brief Funct Genomics 2011;10:280-93. [Crossref] [PubMed]
- Ratnakumar A, Weinhold N, Mar JC, et al. Protein-Protein interactions uncover candidate 'core genes' within omnigenic disease networks. PLoS Genet 2020;16:e1008903. [Crossref] [PubMed]
- Ernst J, Bar-Joseph Z. STEM: a tool for the analysis of short time series gene expression data. BMC Bioinformatics 2006;7:191. [Crossref] [PubMed]
- Szklarczyk D, Morris JH, Cook H, et al. The STRING database in 2017: quality-controlled protein-protein association networks, made broadly accessible. Nucleic Acids Res 2017;45:D362-8. [Crossref] [PubMed]
- Bader GD, Hogue CW. An automated method for finding molecular complexes in large protein interaction networks. BMC Bioinformatics 2003;4:2. [Crossref] [PubMed]
- Vickers AJ, Elkin EB. Decision curve analysis: a novel method for evaluating prediction models. Med Decis Making 2006;26:565-74. [Crossref] [PubMed]
- Yu G, Wang LG, Han Y, et al. clusterProfiler: an R package for comparing biological themes among gene clusters. OMICS 2012;16:284-7. [Crossref] [PubMed]
- Hänzelmann S, Castelo R, Guinney J. GSVA: gene set variation analysis for microarray and RNA-seq data. BMC Bioinformatics 2013;14:7. [Crossref] [PubMed]
- Yi Y, Zhao Y, Li C, et al. RAID v2.0: an updated resource of RNA-associated interactions across organisms. Nucleic Acids Res 2017;45:D115-8. [Crossref] [PubMed]
- Becker K, Heinemann K, Imthurn B, et al. Real world data on symptomology and diagnostic approaches of 27,840 women living with endometriosis. Sci Rep 2021;11:20404. [Crossref] [PubMed]
- Frincu F, Carp-Veliscu A, Petca A, et al. Maternal-Fetal Outcomes in Women with Endometriosis and Shared Pathogenic Mechanisms. Medicina (Kaunas) 2021;57:1258. [Crossref] [PubMed]
- D'Hooghe TM, Nugent NP, Cuneo S, et al. Recombinant human TNFRSF1A (r-hTBP1) inhibits the development of endometriosis in baboons: a prospective, randomized, placebo- and drug-controlled study. Biol Reprod 2006;74:131-6. [Crossref] [PubMed]
- Rockfield S, Flores I, Nanjundan M. Expression and function of nuclear receptor coactivator 4 isoforms in transformed endometriotic and malignant ovarian cells. Oncotarget 2018;9:5344-67. [Crossref] [PubMed]
- Kakhlon O, Gruenbaum Y, Cabantchik ZI. Repression of ferritin expression modulates cell responsiveness to H-ras-induced growth. Biochem Soc Trans 2002;30:777-80. [Crossref] [PubMed]
- Roy D, Morgan M, Yoo C, et al. Integrated Bioinformatics, Environmental Epidemiologic and Genomic Approaches to Identify Environmental and Molecular Links between Endometriosis and Breast Cancer. Int J Mol Sci 2015;16:25285-322. [Crossref] [PubMed]
- Young VJ, Brown JK, Saunders PT, et al. The peritoneum is both a source and target of TGF-β in women with endometriosis. PLoS One 2014;9:e106773. [Crossref] [PubMed]
- Ramírez-Pavez TN, Martínez-Esparza M, Ruiz-Alcaraz AJ, et al. The Role of Peritoneal Macrophages in Endometriosis. Int J Mol Sci 2021;22:10792. [Crossref] [PubMed]
- Johnson MC, Torres M, Alves A, et al. Augmented cell survival in eutopic endometrium from women with endometriosis: expression of c-myc, TGF-beta1 and bax genes. Reprod Biol Endocrinol 2005;3:45. [Crossref] [PubMed]
- Han SJ, O'Malley BW. The dynamics of nuclear receptors and nuclear receptor coregulators in the pathogenesis of endometriosis. Hum Reprod Update 2014;20:467-84. [Crossref] [PubMed]
- Le N, Cregger M, Brown V, et al. Association of microbial dynamics with urinary estrogens and estrogen metabolites in patients with endometriosis. PLoS One 2021;16:e0261362. [Crossref] [PubMed]
- Agrawal S, Tapmeier T, Rahmioglu N, et al. The miRNA Mirage: How Close Are We to Finding a Non-Invasive Diagnostic Biomarker in Endometriosis? A Systematic Review. Int J Mol Sci 2018;19:599. [Crossref] [PubMed]
- Lin YH, Chen YH, Chang HY, et al. Chronic Niche Inflammation in Endometriosis-Associated Infertility: Current Understanding and Future Therapeutic Strategies. Int J Mol Sci 2018;19:2385. [Crossref] [PubMed]
- McKinnon BD, Kocbek V, Nirgianakis K, et al. Kinase signalling pathways in endometriosis: potential targets for non-hormonal therapeutics. Hum Reprod Update 2016;22:382-403. [Crossref] [PubMed]
- Jeung I, Cheon K, Kim MR. Decreased Cytotoxicity of Peripheral and Peritoneal Natural Killer Cell in Endometriosis. Biomed Res Int 2016;2016:2916070. [Crossref] [PubMed]
- Burns KA, Thomas SY, Hamilton KJ, et al. Early Endometriosis in Females Is Directed by Immune-Mediated Estrogen Receptor α and IL-6 Cross-Talk. Endocrinology 2018;159:103-18. [Crossref] [PubMed]
- Abramiuk M, Grywalska E, Małkowska P, et al. The Role of the Immune System in the Development of Endometriosis. Cells 2022;11:2028. [Crossref] [PubMed]
- Podgaec S, Abrao MS, Dias JA Jr, et al. Endometriosis: an inflammatory disease with a Th2 immune response component. Hum Reprod 2007;22:1373-9. [Crossref] [PubMed]
(English Language Editor: J. Teoh)