Bioinformatic analysis of potential biomarkers and mechanisms of immune infiltration in mitral regurgitation complicated by atrial fibrillation
Introduction
Mitral regurgitation (MR) is one of the most prevalent valvular heart diseases worldwide, leading to frequent hospitalization, impaired heart function, and inevitable clinical intervention (1,2). The incidence of MR increases with age (3) and the management of such patients can be complicated by concomitant cardiovascular diseases, such as atrial fibrillation (AF) and heart failure. The mitral valve is an intricate apparatus allowing blood inflow from the left atrium to the left ventricle during diastole. It is composed of leaflets, the annulus, and an even more complex subvalvular structure, the deterioration of which causes abnormal systolic coaptation, namely MR. The major cause of surgical degenerative MR in Western countries is mitral valve prolapse (MVP) (3-5). Functional MR is secondary to an imbalanced tethering force and a change in volume, which are often the result of AF or ventricular dysfunction (6,7). The natural history of MR has been poorly defined, largely because of limitations in severity assessment and dynamic inherence. A 10-year observation cohort study highlighted the necessity of prompt surgery in patients with MR, indicating that the incidence of cardiac events and mortality may rise if left untreated (8). Another observational study showed the incidence of ischemic neurological events in the course of natural development (9). This latter study showed that the aged population with MR had a lifetime stroke risk in excess of that in a community group, and an independent predictor of this risk was the occurrence of AF.
A series of reports have shown that despite a reduced mortality of less than 1% associated with mitral valve repair surgery in patients with MR, AF is a robust predictor of a reduced survival, even after adjusting for other known risk factors (10,11). Indeed, the durability of the MV repair has been shown to be compromised in patients with AF (11). Notably, the proportion of patients with AF who receive transcatheter mitral valve repair using the MitraClip system (Abbott Vascular, Santa Clara, CA, USA) ranges from 38.5% to 67.3% (12), and such patients have a similar risk of procedural failure and in-hospital mortality compared with patients without AF (13,14). However, special considerations regarding safety and efficacy should be evaluated, as a pooled analysis illustrated the higher risk of all-cause mortality, bleeding, and heart failure hospitalization in patients with AF undergoing MV repair (15). MVP, which is the most common reason for MR, is associated with a similar degree of proinflammation and immune infiltration as AF. Myxomatous degeneration in MVP is associated with the extracellular matrix (ECM) status because proteolytic enzymes, which induce interleukins, are overexpressed in valvular interstitial cells (16,17). Nevertheless, to date, only a few studies have assessed the mechanisms of comorbidity in MVP and AF. In particular, there is a paucity of bioinformatics studies examining the mechanisms involving immune cells and hub genes, which may reveal potential therapeutic targets. Understanding the molecular and cellular basis of MVP is crucial in the development of novel pharmacological therapies or targets, and management strategies for patients with MVP and AF.
This current investigation identified the co-expressed differentially expressed genes (co-DEGs) in patients with persistent AF and MVP. The molecular mechanisms and the pathology of AF-related differentially expressed genes (DEGs) and MVP-related DEGs were determined through enrichment analyses. Additionally, a bioinformatics analysis of the DEGs and the predicted microRNAs (miRNAs) was conducted for patients with MVP who are prone to AF. Finally, immune infiltration analysis on 22 subtypes of immune cells was performed via CIBERSORTx, and this may be helpful for examining the imbalance in immune system homeostasis and the mechanisms of how MVP is related to AF. We present the following article in accordance with the STREGA reporting checklist (available at https://atm.amegroups.com/article/view/10.21037/atm-22-4595/rc).
Methods
Data filtering and processing
Datasets used for analysis in this study were obtained from the Gene Expression Omnibus (GEO) database (https://www.ncbi.nlm.nih.gov/geo/). The following inclusion criteria were applied: (I) samples in the dataset were collected from myocardial tissue; (II) samples were obtained from patients with AF or MR, and the corresponding control group; and (III) datasets were based on human gene expression profiles by array. The exclusion criteria were unavailability of raw data of microarray data or gene counts of the high-throughput sequencing data. After thorough screening, two datasets, GSE79768 and GSE109744, for AF and MR, respectively, that satisfied the inclusion criteria were included in this study. Using the platform GPL570 ([HG-U133_Plus_2] Affymetrix Human Genome U133 Plus 2.0 Array), GSE79768 was obtained via mRNA and miRNA microarrays. The gene expression profiling data of GSE109744 were obtained via the platform of GPL19298 (Phalanx Human One Array (version 4.3), with intrinsic probes designed for human genome contents.
The R packages “GEOquery”, “limma”, and “ggplot2” (http://www.bioconduct or.org/packages/release/bioc/html/affy.html), provided by the bioconductor project, were used to analyze the GSE79768 and GSE109744 raw data. After background correction, quantile normalization, probe summarization, and log2 transformation, two robust expression arrays with perfectly matched probes from homologous microarray platforms were established. The Benjamini-Hochberg method was used to adjust the original p values. The false discovery rate was used to calculate fold changes (FCs), which served as a critical criterion for identifying the DEGs. Adjusted P values <0.05 and |log FC| >1 were used as the cut-off criteria for AF-DEGs. Gene counts that originated from right atrial tissue were excluded because our study was designed to investigate the DEGs associated with AF-related MR. Most situations were considered to be related to left atrial distention and hemodynamic disturbance underlying differentiated gene expression. The criterion for filtering MVP DEGs was relatively rigorous owing to the Phalanx series arrays containing a large number of features and mismatched probes. MVP DEGs were determined in line with the criterion of an adjusted P value <0.05 and |log FC| >2.3. Additionally, DEGs in the two datasets were calculated, and Venn diagrams for co-DEGs for AF DEGs and MVP DEGs were created. The study was conducted in accordance with the Declaration of Helsinki (as revised in 2013).
Gene Ontology (GO) and Kyoto Encyclopedia of Genes and Genomes (KEGG) pathway enrichment analysis of the DEGs
The annotation, visualization, and integrated discovery of DEGs were performed using an online database (DAVID database, v6.8; https://david.ncifcrf.gov/). The GO and KEGG pathway enrichment analyses of AF DEGs and MVP DEGs were conducted and visualized using bubble charts and histograms. Particularly, the GO and KEGG pathway functional enrichment analyses of co-DEGs were performed. GO terms and KEGG pathways of biological functions were considered to be significantly enriched when P values were <0.05.
Prediction of potential miRNA targets
Potential miRNA targets were predicted by applying the online databases miRWalk (http://mirwalk.umm.uni-heidelberg.de/) (18), microRNA Data Integration Portal (miDIP) (http://ophid.utoronto.ca/mirDIP/) (19,20), miRDB (http://mirdb.org/) (21), ENCORI (Encyclopedia of RNA Interactomes, https://starbase.sysu.edu.cn/) (22), and the new automated web server supporting pipelines for integrating high-throughput data into advanced miRNA analyses, DIANA-microT-CDS (http://diana.imis.athena-innovation.gr/DianaTools/index.php) (23,24). The top five miRNA targets, which were present in more than two databases, were determined for each co-DEG. A relatively high integration score was used based on previous prediction results. To predict the miRNA pathways, DIANA-miRPath v3.0 (http://diana.imis.athena-innovation.gr/DianaTools/index.php) (25) was used to decipher the biofunctions of the miRNA targets accordingly. Enrichment analyses were then performed based on the predicted miRNAs.
Construction of the protein-protein interaction (PPI) networks, miRNA-target gene regulatory networks, and module analysis
The PPI networks of the AF DEGs and MVP DEGs were analyzed using the STRING database (V11.5; https://cn.string-db.org/) for retrieving interacting genes that predicted protein functional associations and PPIs. Subsequently, PPI networks were visualized using Cytoscape software (26) (V3.9.0; http://cytoscape.org/). These networks included biological networks and node degrees to determine the relationship and contribution of each pitch point. Analytical results with a medium confidence score >0.4 were obtained from the STRING database. In further analysis, hub genes were identified using the CytoHubba (27) plugin, which is a tool for examining important nodes and subnetworks by maximal clique centrality and degree algorithms. Densely connected regions were authenticated as functional clusters developed by MCODE (28) plugin in the networks. The top 10 nodes ranked by different algorithms were listed with their first stage nodes. Additionally, highly interconnected regions were graphed after the advanced options were set as a degree cut-off =2, K-core =2, and node score cut-off =0.2.
Similarly, Cytoscape was used to visualize the complex network between the predicted miRNA and co-DEGs, and we integrated the interaction between predicted miRNA targets and co-DEGs to construct a ceRNA regulatory network. By connecting source nodes and target nodes, the ceRNA network was constructed, where we found that certain miRNAs may regulate multiple genes simultaneously.
Analysis of immune cell infiltration
The development of diseases and common causative gene expression are associated with imbalanced immune homeostasis. An immune infiltration analysis of the dataset was performed using CIBERSORTx (https://cibersortx.stanford.edu/).The expression matrices of the GSE79768 and GSE109744 datasets were uploaded to CIBERSORTx to analyze the immune cell infiltration, which uses a deconvolution method to extract features from single-cell RNA sequencing data, and then reverse deduces the proportion of bulk sequencing components. This process provides a P value and confidence for each sample, with P<0.1 considered robust in our study. The proportion of immune cell types in each sample is shown by histograms produced using the R package “ggpubr” (https://CRAN.R-project.org/package=ggpubr). The expression of certain types of immune cells between the disease group and the control group was compared using bar plots created using the R package “ggplot2” and the Wilcoxon test.
Verification of the correlations between hub genes and disease phenotype
The Comparative Toxicogenomics Database (http://ctdbase.org/) provides manually curated information regarding chemical-gene interactions/PPIs and chemical-disease and gene-disease relationships (29). This database was used to determine the underlying relationships between corresponding miRNAs, cardiovascular disease phenotypes, and immune infiltration mechanisms. Moreover, ontology terms were analyzed to verify the accuracy and to annotate the biofunctions of the identified hub genes through the AmiGO 2 database (http://amigo.geneontology.org/amigo/landing) (30).
Results
Data mining and identification of the DEGs
The GSE79768 dataset included paired left atrial and right atrial specimens, which were obtained from 7 patients with persistent AF and 6 patients with sinus rhythm who received valvular surgery. The GSE109744 dataset contained microarray analysis results of mitral valve interstitial tissue that was harvested from 12 patients with MVP and from 12 healthy control patients from a heart transplant project. There were no missing values in the two datasets. However, as mentioned previously, right atrial specimens were excluded. Prior to analysis, all data were subjected to normalization and background correction, and the results are shown in Figure S1. 180 DEGs were screened from the GSE79768 dataset, including 85 upregulated genes and 95 downregulated genes. Additionally, 491 DEGs, including 143 upregulated genes and 348 downregulated genes, were identified in the GSE109744 dataset. The DEGs of the two datasets were visualized using a volcano map (Figure 1A,1B). Details of the DEGs are listed available online (https://cdn.amegroups.cn/static/public/atm-22-4595-1.xlsx and https://cdn.amegroups.cn/static/public/atm-22-4595-2.xlsx) Cluster heatmaps comprising the top 30 DEGs are shown in Figure 1C,1D.
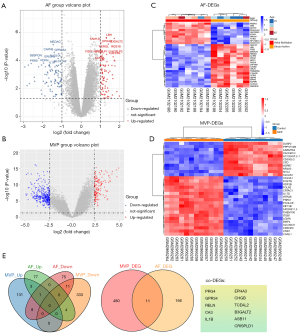
The Venn diagram in Figure 1E shows the expressed AF DEGs and MVP DEGs, as well as the co-expressed genes. Notably, 11 co-expressed genes, namely proteoglycan 4 (PRG4), probable G-protein coupled receptor 34 (GPR34), reelin (RELN), carbonic anhydrase 3 (CA3), interleukin-1 beta (IL1B), ephrin type-A receptor 3 (EPHA3), secretogranin-1 (CHGB), transcription elongation factor A protein-like 2 (TCEAL2), beta-1,3-galactosyltransferase 2 (B3GALT2), ankyrin repeat and SOCS box protein 11 (ASB11), and cysteine-rich secretory protein LCCL domain containing 1 (CRISPLD1) were identified. The AmiGO database was used to confirm GO enrichment terms related to biological processes, molecular functions, and cellular components, and the co-DEGs were found to be associated with various processes, including immune response, response to oxidative stress and integral component of plasma membrane etc. (Table 1). These findings may provide new directions for determining the pathogenic gene-regulated mechanisms of MVP and AF.
Table 1
Gene/product | GO class (direct) | Evidence | Evidence with | Reference |
---|---|---|---|---|
PRG4 | Scavenger receptor activity | IEA | InterPro:IPR020436 | GO_REF: 0000002 |
Polysaccharide binding | IEA | InterPro:IPR020436 | GO_REF: 0000002 | |
Endocytosis | IEA | GO:0005044 | GO_REF: 0000108 | |
Immune response | IEA | InterPro:IPR020436 | GO_REF: 0000002 | |
Extracellular region | IEA | UniProtKB-SubCell:SL-0243 | GO_REF: 0000044 | |
GPR34 | G protein-coupled purinergic nucleotide receptor signaling pathway | IEA | GO:0045028 | GO_REF: 0000108 |
G protein-coupled receptor signaling pathway | IBA | UniProtKB:Q9H244 | PMID: 21873635 | |
G protein-coupled purinergic nucleotide receptor activity | IBA | UniProtKB:Q9H244 | PMID: 21873635 | |
RELN | Serine-type peptidase activity | IEA | UniProtKB-KW:KW-0720 | GO_REF: 0000043 |
Metal ion binding | IEA | UniProtKB-KW:KW-0479 | GO_REF: 0000043 | |
Lipoprotein particle receptor binding | ISS | UniProtKB:Q60841 | PMID: 10571240 | |
Very-low-density lipoprotein particle receptor binding | ISS | UniProtKB:Q60841 | PMID: 10571240 | |
Cell morphogenesis involved in differentiation | ISS | UniProtKB:Q60841 | GO_REF: 0000024 | |
CA3 | Nickel cation binding | IEA | UniProtKB:P16015 | GO_REF: 0000107 |
Phosphatase activity | IEA | UniProtKB:P14141 | GO_REF: 0000107 | |
Response to oxidative stress | IEA | UniProtKB:P14141 | GO_REF: 0000107 | |
IL1B | Inflammatory response | IDA | PMID: 21147091 | |
Cytokine activity | IDA | PMID: 1919436 | ||
Cytosol | TAS | Reactome: R-HSA-448703 | ||
Extracellular space | IBA | UniProtKB:P01584 | PMID: 21873635 | |
EPHA3 | Plasma membrane | IDA | GO_REF: 0000052 | |
Protein binding | IPI | UniProtKB:P20827 | PMID: 11519828 | |
Integral component of plasma membrane | IDA | UniProtKB:P06213 | PMID: 21873635 | |
ATP binding | IEA | UniProtKB-KW:KW-0067 | GO_REF: 0000043 | |
CHGB | Secretory granule | IBA | UniProtKB:P05059 | PMID: 21873635 |
Extracellular space | IBA | UniProtKB:P05059 | PMID: 21873635 | |
TCEAL2 | WW domain binding | IBA | PANTHER:PTN001028010 | PMID: 21873635 |
Nucleus | IBA | UniProtKB:Q9BRU2 | PMID: 21873635 | |
B3GALT2 | Glycosyltransferase activity | IBA | UniProtKB:Q7Z7M8 | PMID: 21873635 |
Transferase activity | IEA | UniProtKB-KW:KW-0808 | ZFIN: ZDB-PUB-020723-1 | |
Hexosyltransferase activity | IEA | ZFIN: ZDB-PUB-031118-3 | ||
Protein glycosylation | IEA | InterPro:IPR002659 | ZFIN: ZDB-PUB-020724-1 | |
Integral component of membrane | IEA | UniProtKB-KW:KW-0812 | ZFIN: ZDB-PUB-020723-1 | |
ASB11 | Protein ubiquitination | IEA | UniPathway:UPA00143 | GO_REF: 0000041 |
Intracellular signal transduction | IEA | InterPro:IPR036036 | GO_REF: 0000002 | |
Protein ubiquitination | IBA | UniProtKB:Q96DX5 | PMID: 21873635 | |
Positive regulation of protein catabolic process | IBA | PANTHER:PTN000651312 | PMID: 21873635 | |
UniProtKB:Q96DX5 | ||||
CRISPLD1 | Extracellular exosome | HDA | PMID: 19199708 | |
Extracellular space | IBA | UniProtKB:A5D8T8 | PMID: 21873635 |
GO, Gene Ontology; IEA, inferred from electronic annotation; IBA, inferred from a biological aspect of an ancestor; ISS, inferred from a sequence or structural similarity; IDA, inferred from a direct assay; TAS, traceable author statement; IPI, inferred from a physical interaction; HDA, inferred from a high-throughput direct assay.
Functional enrichment of the co-expressed DEGs
GO function terms consist of three parts: biological process, molecular function, and cell component (https://cdn.amegroups.cn/static/public/atm-22-4595-3.xlsx). According to the DAVID database, the top five GO terms related to biological processes associated with the DEGs in the AF dataset were immune response (q value: 2.34E-05), inflammatory response (q value: 1.45E-04), complement activation (q value: 0.001), positive regulation of the inflammatory response (q value: 0.004), and B cell receptor signaling pathway (q value: 0.012). The top cell component terms were blood microparticles (q value: 6.82E-06), extracellular space (q value: 1.12E-05), extracellular exosomes (q value: 1.68E-05), extracellular region (q value: 4.22E-05), and plasma membrane (q value: 4.83E-04). Immunoglobulin receptor binding was the top molecular function associated with the AF DEGs (q value: 0.019). The results of GO term analysis in AF DEGs are shown in shown in Figure 2A. A total of 125 GO terms were associated with MVP DEGs, and muscle filament sliding (q value: 8.00E-09), muscle contraction (q value: 8.31E-07), sarcomere organization (q value: 2.82E-06), cardiac muscle contraction (q value: 6.37E-06), and cell-cell signaling (q value: 1.90E-05) were the top biological process. Thirty-four cell component terms, such as the Z disc (q value: 6.37E-06), and 24 molecular function terms, such as a proteinaceous ECM (q value: 6.37E-06) and titin binding (q value: 2.09E-04), were enriched among the MVP DEGs (Figure 2B). Besides, extracellular region (q value: 1.90E-03), extracellular space (q value: 1.00E-02), etc. GO terms were significantly associated with co-DEGs.
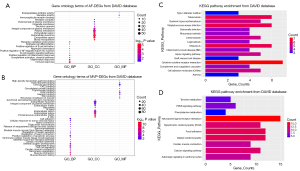
KEGG pathway analysis (Figure 2C,2D) suggested that the AF DEGs were mainly enriched in the pathways of Staphylococcus aureus infection (q value: 6.96E-05), cytokine-cytokine receptor interaction (q value: 0.013), and the Hippo signaling pathway (q value: 0.033). KEGG terms, such as dilated cardiomyopathy (q value: 1.26E-05), hypertrophic cardiomyopathy (q value: 3.81E-05), cardiac muscle contraction (q value: 8.21E-04), and calcium signaling pathways (q value: 0.021), were enriched in MVP DEGs. Other GO/KEGG terms from the REACTOME database added additional information regarding functional enrichment of both datasets, and the integration results are shown available online (https://cdn.amegroups.cn/static/public/atm-22-4595-4.xlsx).
Associations between co-expressed DEGs and disease phenotype
To investigate and further verify the associations between previously determined co‑DEGs and particular diseases, the Comparative Toxicogenomics Database was used to determine the co-DEGs that are associated with cardiovascular disease (https://cdn.amegroups.cn/static/public/atm-22-4595-5.xlsx). Interestingly, several co‑DEGs, such as PRG4 and IL1B, showed a distinct association with arrhythmias or heart diseases, and were highly related to cardiomegaly based on direct evidence from the marker/mechanism category. These findings suggested a predominant role of co‑DEGs in the pathogenesis of disease, which may be important for future research in this field.
Construction of the PPI network and the miRNA-target gene regulatory network
Using the STRING database with the setting parameter of the minimum required interaction score, a total of 165 and 449 nodes from the PPI network of AF DEGs and MVP DEGs were identified, respectively (Figure S2). Hub genes were then identified using the CytoHubba plugin with the maximal clique centrality and degree algorithms (Figure 3A,3B). We found that IL1B, C-X-C chemokine receptor type 2, low affinity immunoglobulin gamma Fc region receptor III-A, growth-regulated alpha protein (CXCL1), and myeloid cell nuclear differentiation antigen were hub genes related to persistent maintenance of AF in both algorithms. However, titin (TTN), telethonin, myosin-binding protein C, troponin I, tropomyosin alpha-1 chain, alpha-actinin-2, troponin T, desmin, myosin regulatory light chain 2, and myosin regulatory light chain 4 (MYL4) were hub genes in MR. The top three clusters with a relatively high score for each dataset were identified using the Cytoscape plugin MCODE (Figure 3C,3D). Notably, the hub gene MYL4 served as the seed gene in module 1 of the MVP dataset, which indicated that MYL4 may be important in hemodynamic and dynamic changes in MVP. Other modules showed functional clusters with seed genes, despite the fact that these seed genes were not identified as hub genes and did not contribute significantly in the PPI network.
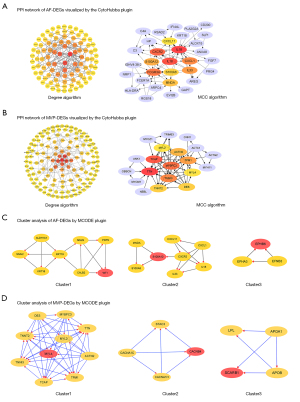
The miRNAs are a class of endogenous small RNAs, which are approximately 20–24 nucleotides in length and play a variety of important regulatory roles in cells. This intricate regulatory network can regulate the expression of multiple genes through a single miRNA or finely regulate the expression of a gene through the combination of several miRNAs (Figure 4). The miRNA-mRNA network consisted of 11 hub genes, 397 miRNAs, and 474 edges. The miRNA miR-3121-3p regulated B3GALT2, EPHA3, IL1B, and RELN expression Furthermore, miR-4428, miR-380-3p, miR-889-3p appeared to simultaneously regulate ASB11, CRISPLD1, and EPHA3 expression, while miR-1205 and miR-96-5p regulated B3GALT2, CRISPLD1 and EPHA3 expression. Additionally, miR-582-5p was predicted to regulate CRISPLD1, GPR34, and RELN expression (Figure 4). These findings of predicted miRNAs suggested that immune-inflammatory responses are involved in the miRNA-target gene regulatory network through leukocyte migration, the immune system process, innate immune responses, negative regulation of intrinsic apoptotic signaling pathways, positive regulation of type I interferon production, and the interaction of cytokines and chemokines (Table 2).
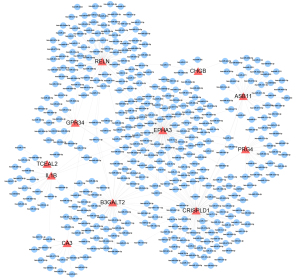
Table 2
Genes | Predicted miRNAs | Category | Evidence with | P value |
---|---|---|---|---|
PRG4 | hsa-miR-4728-5p hsa-miR-508-3p hsa-miR-6733-3p hsa-miR-30a-5p hsa-miR-664b-3p |
GO terms | Synaptic transmission | 0.047239406 |
Leukocyte migration | 0.042180294 | |||
Nucleic acid binding transcription factor activity | 0.003957964 | |||
Catabolic process | 8.99E-11 | |||
KEGG pathway | Cellular nitrogen compound metabolic process | 7.40E-76 | ||
Ion binding | 1.09E-70 | |||
Ubiquitin mediated proteolysis | 5.51E-06 | |||
Protein processing in endoplasmic reticulum | 0.00063029 | |||
Hippo signaling pathway | 0.003033683 | |||
TCEAL2 | hsa-miR-7150 hsa-miR-494-3p |
GO terms | NA | NA |
KEGG pathway | NA | NA | ||
GPR34 | hsa-miR-299-5p hsa-miR-582-5p hsa-miR-452-3p hsa-miR-183-5p hsa-miR-485-5p |
GO terms | Cellular nitrogen compound metabolic process | 0.000495657 |
Organelle | 0.000578882 | |||
Semicircular canal morphogenesis | 0.039896044 | |||
Protein binding transcription factor activity | 0.039896044 | |||
Biosynthetic process | 0.039896044 | |||
KEGG pathway | Lysine degradation | 2.56985E-07 | ||
Thyroid hormone signaling pathway | 5.22514E-05 | |||
Fatty acid degradation | 0.000691094 | |||
MicroRNAs in cancer | 0.001447061 | |||
Base excision repair | 0.001464463 | |||
Fatty acid metabolism | 0.003039675 | |||
alpha-Linolenic acid metabolism | 0.018012771 | |||
RELN | hsa-miR-15b-5p hsa-miR-449a hsa-miR-449b-5p hsa-miR-138-5p hsa-miR-218-5p |
GO terms | Epidermal growth factor receptor signaling pathway | 9.09E-23 |
Immune system process | 5.61E-18 | |||
Innate immune response | 5.55E-11 | |||
Transforming growth factor beta receptor signaling pathway | 2.05E-10 | |||
Notch receptor processing | 0.002526753 | |||
KEGG pathway | Protein processing in endoplasmic reticulum | 1.72E-06 | ||
Hippo signaling pathway | 6.33E-06 | |||
p53 signaling pathway | 0.000162725 | |||
CA3 | hsa-miR-6833-5p hsa-miR-138-5p hsa-miR-6073 hsa-miR-7161-3p hsa-miR-653-3p |
GO terms | Transcription initiation from RNA polymerase II promoter | 1.87E-07 |
Nucleic acid binding transcription factor activity | 0.001057032 | |||
Phosphatidylinositol-mediated signaling | 0.001260273 | |||
Natural killer cell mediated cytotoxicity | 0.034863603 | |||
Negative regulation of cell adhesion mediated by integrin | 0.037682518 | |||
KEGG pathway | RNA degradation | 0.032732342 | ||
Notch signaling pathway | 0.032732342 | |||
IL1B | hsa-miR-328-3p hsa-miR-1291 hsa-miR-6775-3p hsa-miR-6090 hsa-miR-495-3p |
GO terms | Positive regulation of transcription, DNA-templated | 1.75E-09 |
Fc-epsilon receptor signaling pathway | 2.12E-08 | |||
Cell proliferation | 4.83E-07 | |||
Ventricular cardiac muscle cell differentiation | 0.006174478 | |||
Negative regulation of cardiac muscle contraction | 0.035952363 | |||
Heart development | 0.000730859 | |||
Heart morphogenesis | 0.022466387 | |||
Negative regulation of Wnt signaling pathway involved in heart development | 0.043293536 | |||
KEGG pathway | Arrhythmogenic right ventricular cardiomyopathy (ARVC) | 0.015531278 | ||
mTOR signaling pathway | 0.025593018 | |||
PI3K-Akt signaling pathway | 0.039626604 | |||
Thyroid hormone signaling pathway | 0.019206814 | |||
EPHA3 | hsa-miR-33a-3p hsa-miR-325 hsa-miR-1271-5p hsa-miR-548am-5p hsa-miR-548c-5p |
GO terms | Cellular nitrogen compound metabolic process | 5.09E-45 |
Neurotrophin TRK receptor signaling pathway | 2.44E-14 | |||
Immune system process | 3.79E-10 | |||
Toll-like receptor TLR6:TLR2 signaling pathway | 0.014133742 | |||
Negative regulation of intrinsic apoptotic signaling pathway | 0.038997795 | |||
Positive regulation of type I interferon production | 0.044355055 | |||
Canonical Wnt signaling pathway involved in regulation of cell proliferation | 0.044604261 | |||
KEGG pathway | AMPK signaling pathway | 0.006993869 | ||
mTOR signaling pathway | 0.03258834 | |||
p53 signaling pathway | 0.04654045 | |||
Protein processing in endoplasmic reticulum | 0.048226152 | |||
CHGB | hsa-miR-592 hsa-miR-1179 hsa-miR-3132 hsa-miR-942-5p |
GO terms | Notch signaling pathway | 0.000131028 |
Neurotrophin TRK receptor signaling pathway | 0.00174162 | |||
Phosphatidylinositol-mediated signaling | 0.018874782 | |||
Epidermal growth factor receptor signaling pathway | 0.032175918 | |||
Repressing transcription factor binding | 0.034206643 | |||
Myeloid progenitor cell differentiation | 0.034985843 | |||
Myeloid cell homeostasis | 0.042765672 | |||
KEGG pathway | Huntington’s disease | 0.002894388 | ||
Acute myeloid leukemia | 0.025724522 | |||
B3GALT2 | hsa-miR-25-3p hsa-miR-33a-5p hsa-miR-2355-3p hsa-miR-92a-3p hsa-miR-32-5p |
GO terms | Small molecule metabolic process | 7.56E-31 |
Protein binding transcription factor activity | 3.95E-28 | |||
Neurotrophin TRK receptor signaling pathway | 2.11E-21 | |||
Cytoskeletal protein binding | 8.94E-11 | |||
Fibroblast growth factor receptor signaling pathway | 2.83E-08 | |||
Immune system process | 1.10E-06 | |||
Transcription from RNA polymerase II promoter | 6.51E-05 | |||
Transcription coactivator activity | 0.000938201 | |||
Endoplasmic reticulum unfolded protein response | 0.001359258 | |||
KEGG pathway | MAPK signaling pathway | 0.000246814 | ||
FoxO signaling pathway | 0.000246814 | |||
Regulation of actin cytoskeleton | 0.00173382 | |||
ASB11 | hsa-miR-1275 hsa-miR-5010-5p hsa-miR-4428 hsa-miR-5703 hsa-miR-601 |
GO terms | Cellular protein modification process | 0.022187865 |
Peptidyl-arginine N-methylation | 0.032423696 | |||
Transcription coactivator activity | 0.037743702 | |||
Positive regulation of transporter activity | 0.037743702 | |||
Ligand-dependent nuclear receptor transcription coactivator activity | 0.037743702 | |||
Protein methylation | 0.0378816 | |||
KEGG pathway | TGF-beta signaling pathway | 0.001139909 | ||
CRISPLD1 | hsa-miR-130a-3p hsa-miR-195-3p hsa-miR-4667-5p hsa-miR-3176 hsa-miR-5006-5p |
GO terms | Cellular protein modification process | 6.81E-32 |
Protein binding transcription factor activity | 5.76E-17 | |||
Positive regulation of nuclear-transcribed mRNA catabolic process, deadenylation-dependent decay | 0.020717727 | |||
Positive regulation of transcription, DNA-templated | 0.025856783 | |||
Transcription elongation from RNA polymerase II promoter | 0.026949522 | |||
Respiratory electron transport chain | 0.034573444 | |||
Positive regulation of fibroblast proliferation | 0.041798171 | |||
KEGG pathway | Thyroid hormone signaling pathway | 0.000207147 | ||
TGF-beta signaling pathway | 0.001433381 | |||
Signaling pathways regulating pluripotency of stem cells | 0.011292357 | |||
AMPK signaling pathway | 0.020753543 | |||
mTOR signaling pathway | 0.045549458 |
GO, Gene Ontology; KEGG, Kyoto Encyclopedia of Genes and Genomes; NA, not available.
Immune cell infiltration results
The immune cell subtypes were presented as boxplots histograms while null values of immune cell subgroups in the samples were excluded from the output results (Figure 5). These excluded subtypes were M0 macrophages, CD4+ native T cells, plasma cells, follicular helper T cells, CD4+ activated memory T cells, and resting natural killer cells in AF (Figure 5A,5B). The proportion of native B cells was significantly higher and that of memory B cells was significantly lower in the analysis of the GSE79768 dataset compared with controls (Figure 5A,5B), indicating that B cell activation might be closely associated with persistent AF (P<0.05). In addition to the activation of B cells, macrophage polarization and antigen-presenting cells were related to MVP dynamics, as shown by the significant clustering of dendritic cells and regulatory T cells compared with controls (P<0.05). The proportions of monocytes, activated dendritic cells, activated mast cells, memory B cells, and regulatory T cells were lower in the MVP group compared to the control group. This suggested that the depletion of antigen-presenting cells may play a crucial role in the immune infiltration-driven MVP process.
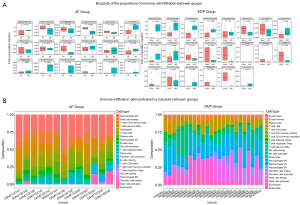
Discussion
MR is an insidious valvular heart disease that affects all ages worldwide, with degeneration (such as MVP) as its main etiology in developed countries and rheumatic changes in developing countries (3,31). According to the population-based Framingham cohort, mild MR was observed in 19% of patients, and aging was characterized as a prominent clinical determinant, with an odds ratio of 1.3 (95% confidence interval: 1.2–1.5) (32). The occurrence of MVP has been reported to be 2.4% according to relatively strict diagnostic criteria, and 1.2% of these patients had AF (33). Notably, degenerative MR, which often requires surgical treatment or percutaneous edge to edge repair, is a consequence of MVP, and is characterized by abnormal systolic valve movement into the left atrium (≥2 mm beyond the saddle-shaped annular level) (34,35). Similar to degenerative MR, AF is also prevalent because of an increase in ventricular volume caused by degenerative MR and a progressively enlarged left atrium (36). Many studies have shown that preoperative and postoperative AF has a major negative effect on the functional remolding of the left ventricle, freedom from late stroke, and survival outcomes in patients with mitral valve surgery (37-40). In addition to the uncertain effects of AF on prognosis, the ideal anticoagulant regimen in patients with AF and MVP awaiting surgical repair or replacement remains controversial (41-43). In patients with AF and MVP, the efficacy of traditional pharmacotherapy has limitations, while surgical treatment is complex and often fraught with technical problems. Therefore, from a mechanistic perspective, a novel, more effective, and less invasive treatment strategy is required.
To investigate the genetic determinants of the progression of MVP, microarrays and high-throughput sequencing have become powerful and important tools for predicting potential molecular and cellular mechanisms (44). Several previous studies have used microarrays and high-throughput sequencing technologies to examine hub genes of MVP or AF and their function in the development of disease. Nevertheless, these studies only focused on identifying hub genes, and did not further investigate the particular potential molecular mechanisms or regulatory interplay network in the occurrence of MVP with or without AF (44-47). This current study not only analyzed the hub genes of AF and MVP, but also examined the corresponding predicted miRNAs based on co-DEGs and the possible immune infiltration.
The GSE79768 dataset related to AF and the GSE109744 dataset related to MVP were analyzed to identify the respective DEGs. Using different Genetic Programming Layer (GPL) platforms, two datasets was found to share 11 common DEGs after background calibration. This finding may help determine the underlying mechanisms in patients with combined MVP and AF. MiRNAs are the most abundant non-coding RNA species that target mRNA recognition to inhibit protein synthesis. Indeed, miRNAs have been intensively studied in terms of its cellular localization and extracellular secretion features (48). Herein, miRNA prediction was performed based on the co-DEGs. Immune infiltration analysis was then conducted using the joint enrichment results of the co-DEGs and miRNAs. The results suggested a role for immune response in the development of MVP combined with AF. Final comprehensive and rigorous results were then achieved by aggregating the co-DEGs and associated diseases, which further indicated that co-DEGs were associated with MVP combined with AF via unbalanced immune homeostasis.
Collectively, the following 11 integrated DEGs were identified: PRG4, GPR34, RELN, CA3, IL1B, EPHA3, CHGB, TCEAL2, B3GALT2, ASB11, and CRISPLD1. Hub gene and module analysis using the CytoHubba and MCODE plugins indicated that the IL1B and TTN genes had the highest combined score in AF DEGs and MVP DEGs, respectively. To note, IL1B was identified as a co-DEG as well as a hub gene. Furthermore, KEGG pathway results showed that AF DEGs were mainly enriched in cytokine-cytokine receptor interactions, inflammatory bowel disease, and graft-versus-host disease. Similar enrichment results were observed in MVP DEGs, with enrichment of dilated cardiomyopathy and hypertrophic cardiomyopathy pathways. In the subsequent analysis of GO terms, we mainly focused on biological processes, and found that AF DEGs were significantly enriched in immune responses, inflammatory responses, and complement activation. The MVP DEGs were enriched in biological processes involving cardiac muscle contraction regulation and intracellular energy metabolism. These findings suggested that immune response was associated with MVP combined with AF. Immune infiltration analysis was then performed with the aid of CIBERSORTx.
Novel biomarkers and the importance of the immune or inflammatory response have been examined in MR and AF in separate studies (44-46,49-51). Driesbaugh et al. showed that upregulation of 5-hydroxytryptamine receptor 2B (5HTR2B) expression by increased 5-hydroxytryptamine (5HT) receptor signaling contributed to the progression of MVP (46). They also found that an antagonist of 5HTR2B was effective in ameliorating mitral valve remolding in vitro. However, Tan and colleagues showed that transforming growth factor-β-driven endothelial-to-mesenchymal transition greatly contributed to the myofibroblast phenotype in mitral valve interstitial cell cultivation, and 5HT signaling was likely to be transforming growth factor-β1-mediated (52). Moreover, Oceandy et al. identified the matrix metalloproteinase 3 promoter as a novel marker associated with an exceedingly adverse outcome in MVP, owing to left ventricular remodeling (53). This suggested the presence of genetic determinants for the severity of MVP. In another study, myocardial pro-inflammatory cytokine expression was detected pre- and postoperatively in patients with chronic MR receiving MV repair surgery (54). Additionally, there was a clear inverse correlation between transforming growth factor-α and left ventricular overload in these patients compared with control subjects (54). Previous study (46) showed that 5HTR2B was upregulated in the MVP group, which is consistent with reports in the literature. Furthermore, the focal adhesion pathway, which regulates cell adhesion, migration, proliferation, and differentiation by interacting with various family kinase, was enriched (55). Similarly, Sabri et al. induced isolated MR by chordal rupture in a canine model and found that β-adrenergic receptor blockade prevented focal adhesion signaling from downregulation induced by volume overload-driven MR (56). Considering that 5HTR2B initiates remodeling activity characterized by increased proliferation and collagen production (57), enhanced 5HT pathway signaling might result in a greater response in remodeling endpoints characterized by proliferation and collagen.
Atrial electrophysiology and structural components can be regulated by inflammation, leading to increased vulnerability and susceptibility to AF alone. Therefore, inflammatory and immune mediators, which may play an important role in the development of AF, have become a focus of research because the inflammatory process is a potential therapeutic target. A previous study established a novel pathophysiological role for cardiomyocyte NOD-like receptor thermal protein domain associated protein 3 inflammasome signaling associated with the progression of AF (58). Additionally, therapeutic knockdown of NOD-like receptor thermal protein domain associated protein 3 suppressed AF development in a mouse model. Goette et al. collected right atrial appendages from 16 patients with sinus rhythm and 16 with chronic AF for reverse-transcription polymerase chain reaction analysis and determined the expression of multiple cytokines in atrial tissues (59). In addition to cytokines, various chemokines, epicardial adipose tissue, and collagen involved in fibrotic remodeling are closely associated with AF (60-63). In this current study, the PPI network of AF showed that the cytokines, IL18 and IL1B, ranked high as hub genes, which further verified the association between the development of AF and activated inflammation.
Interestingly, among the co-DEGs, IL1B was identified as a DEG and a hub gene regulating molecular function and immune reactions. Galeone et al. measured serum concentrations of soluble growth stimulation expressed gene 2, which is a member of the interleukin-1 receptor family, in 20 patients with MR (64). They found that higher soluble growth stimulation expressed gene 2 concentrations were associated with satisfactory postoperative outcomes and a better left ventricular ejection fraction. Similarly, Lu et al. performed next-generation sequencing of miRNAs to predict target genes in 40 patients with rheumatic mitral valve disease (65). They found a higher inflammatory response, as measured by elevated IL1B and IL1R1 expression, in the mitral valve disease group compared to the control group. In an in vitro study, mitral valvular interstitial cells were cultured in collagen and polyethylene glycol scaffolds with cytokines designed to mimic the heart valve microenvironment (66). This latter study showed that IL1B as a pro-inflammatory cytokine, suppressed myofibroblasts and fibrosis via the canonical nuclear factor-κB signaling pathway (66). A plausible explanation for this finding is that endothelial-to-mesenchymal transition was induced in the presence of mitral valve disease, which was characterized by valvular endothelial cell transformation and activated myofibroblasts. This process could reverse the activation of inflammation stimuli by overexpressing IL1B through feedback compensation. Despite the indistinct mechanism of IL1B participating in the progress of mitral valve disease, the crosstalk between macrophages and atrial myocytes in AF has been shown in multiple studies. Sun et al. demonstrated that IL1B inhibited atrial myocyte quaking protein expression in a chronic inflammation mouse model and alleviated the incidence of AF (67).
The immune response participates in disease progression through multiple cellular components and complex cascade reactions. Technical methods for sorting specific cells from harvested tissue include immunohistochemical staining and flow cytometry. Nevertheless, immunohistochemistry is only a semi-quantitative method, and flow cytometry may be limited by sample content. In contrast, CIBERSORTx, which uses deep deconvolution and enumeration of individual cell subsets, provides accurate cell heterogeneity from nearly any tissue RNA mixture. Recently, CIBERSORTx was used to provide novel insights into the deconvolution of immune infiltrates in acute myocarditis (68) and the tumor microenvironment (69,70). To the best of our knowledge, this current report is the first to use CIBERSORTx to determine immune cell infiltration in MVP with AF. Emerging evidence has suggested that T cells are sufficient to induce inflammation, and do not require the presence of cross-reactive antibodies to trigger rheumatic valvulitis. Previous studies on patients with end-stage rheumatic mitral valve disease have identified mononuclear cells, with mainly CD4+ helper T cells, CD8+ T cells (71), macrophages, and B cells (72). However, the proportions of CD4+ and CD8+ T cells appear to fluctuate dynamically in different stages of rheumatic mitral valve disease, as shown by the opposing trends in acute rheumatic fever and rheumatic heart disease (71). Our results further support this perspective. We found that the number of CD4+ naïve T cells was significantly higher in the MVP group than in the control group, while CD8+ T cells showed the reverse trend. The numbers of circulating T follicular helper cells increased with an increase in memory B cells, suggesting a negative association in patients with rheumatic heart disease (73). Notably, our results showed a similar finding in circulating T follicular helper cells and memory B cells, suggesting a prominent role of circulating T follicular helper cells and humoral reactivity in mitral valve disease. Further in-depth studies on the mechanisms of the immune-inflammatory response may contribute to the development of molecular targeted therapies for patients with MVP complicated by AF.
Despite the identification of an elaborate network between the DEGs and miRNAs, there were several limitations to this study. First, the results of the microarray analysis should be interpreted with caution. Second, the reads of gene expression may not fully represent the level of protein expression. Therefore, bioinformatic analysis based on the read count, not experimental data from polymerase chain reaction and western blot assays, should be validated by in vitro studies, in vivo studies, and clinical trials with a long-term follow-up. Finally, the raw data lacked corresponding information on patient prognosis, which may reveal new research perspectives when integrated with the results. However, this study integrated dataset information and only excluded specimens from the right atrium to distinguish the relationship of MVP with AF. More reliable DEGs need to be identified through extensive and strict screening criteria and successive analysis. Nonetheless, this current investigation provides a deeper understanding and contributes to the development of future treatments in the field of competing endogenous RNA regulatory interactions and immune infiltration.
Conclusions
The co-DEGs identified herein, namely, PRG4, GPR34, RELN, CA3, IL1B, EPHA3, CHGB, TCEAL2, B3GALT2, ASB11, and CRISPLD1, may be associated with MR and AF. The top five miRNAs for each co-DEG may be potential biomarkers or therapeutic targets for MVP with AF, especially miR-3121-3p, miR-4428, miR-380-3p, miR-889-3p, miR-1205, and miR-96-5p. Finally, immune infiltration analysis of immune cell subtypes suggested that the depletion of antigen-presenting cells and the activation of an autoimmune response may play a role in the mechanism of MVP with AF.
Acknowledgments
Funding: This work was supported by The Fundamental Research Funds for the Central Universities (2019PT350005), the National Natural Science Foundation of China (81970444), Beijing Municipal Science and Technology Project (Z201100005420030), the National High Level Talents Special Support Plan (2020-RSW02), and the Sanming Project of Medicine in Shenzhen (SZSM202011013).
Footnote
Reporting Checklist: The authors have completed the STREGA reporting checklist. Available at https://atm.amegroups.com/article/view/10.21037/atm-22-4595/rc
Conflicts of Interest: All authors have completed the ICMJE uniform disclosure form (available at https://atm.amegroups.com/article/view/10.21037/atm-22-4595/coif). The authors have no conflicts of interest to declare.
Ethical Statement: The authors are accountable for all aspects of the work in ensuring that questions related to the accuracy or integrity of any part of the work are appropriately investigated and resolved. The study was conducted in accordance with the Declaration of Helsinki (as revised in 2013).
Open Access Statement: This is an Open Access article distributed in accordance with the Creative Commons Attribution-NonCommercial-NoDerivs 4.0 International License (CC BY-NC-ND 4.0), which permits the non-commercial replication and distribution of the article with the strict proviso that no changes or edits are made and the original work is properly cited (including links to both the formal publication through the relevant DOI and the license). See: https://creativecommons.org/licenses/by-nc-nd/4.0/.
References
- Nkomo VT, Gardin JM, Skelton TN, et al. Burden of valvular heart diseases: a population-based study. Lancet 2006;368:1005-11. [Crossref] [PubMed]
- Nishimura RA, Otto CM, Bonow RO, et al. 2017 AHA/ACC Focused Update of the 2014 AHA/ACC Guideline for the Management of Patients With Valvular Heart Disease: A Report of the American College of Cardiology/American Heart Association Task Force on Clinical Practice Guidelines. Circulation 2017;135:e1159-95. [Crossref] [PubMed]
- Enriquez-Sarano M, Akins CW, Vahanian A. Mitral regurgitation. Lancet 2009;373:1382-94. [Crossref] [PubMed]
- Flint N, Raschpichler M, Rader F, et al. Asymptomatic Degenerative Mitral Regurgitation: A Review. JAMA Cardiol 2020;5:346-55. [Crossref] [PubMed]
- Faletra FF, Demertzis S, Pedrazzini G, et al. Three-dimensional transesophageal echocardiography in degenerative mitral regurgitation. J Am Soc Echocardiogr 2015;28:437-48. [Crossref] [PubMed]
- Deferm S, Bertrand PB, Verbrugge FH, et al. Atrial Functional Mitral Regurgitation: JACC Review Topic of the Week. J Am Coll Cardiol 2019;73:2465-76. [Crossref] [PubMed]
- Grayburn PA, Sannino A, Packer M. Proportionate and Disproportionate Functional Mitral Regurgitation: A New Conceptual Framework That Reconciles the Results of the MITRA-FR and COAPT Trials. JACC Cardiovasc Imaging 2019;12:353-62. [Crossref] [PubMed]
- Ling LH, Enriquez-Sarano M, Seward JB, et al. Clinical outcome of mitral regurgitation due to flail leaflet. N Engl J Med 1996;335:1417-23. [Crossref] [PubMed]
- Avierinos JF, Brown RD, Foley DA, et al. Cerebral ischemic events after diagnosis of mitral valve prolapse: a community-based study of incidence and predictive factors. Stroke 2003;34:1339-44. [Crossref] [PubMed]
- Varghese R, Itagaki S, Anyanwu AC, et al. Predicting early left ventricular dysfunction after mitral valve reconstruction: the effect of atrial fibrillation and pulmonary hypertension. J Thorac Cardiovasc Surg 2014;148:422-7. [Crossref] [PubMed]
- Coutinho GF, Garcia AL, Correia PM, et al. Negative impact of atrial fibrillation and pulmonary hypertension after mitral valve surgery in asymptomatic patients with severe mitral regurgitation: a 20-year follow-up. Eur J Cardiothorac Surg 2015;48:548-55; discussion 555-6. [Crossref] [PubMed]
- Keßler M, Pott A, Mammadova E, et al. Atrial Fibrillation Predicts Long-Term Outcome after Transcatheter Edge-to-Edge Mitral Valve Repair by MitraClip Implantation. Biomolecules 2018;8:152. [Crossref] [PubMed]
- Arora S, Vemulapalli S, Stebbins A, et al. The Prevalence and Impact of Atrial Fibrillation on 1-Year Outcomes in Patients Undergoing Transcatheter Mitral Valve Repair: Results From the Society of Thoracic Surgeons/American College of Cardiology Transcatheter Valve Therapy Registry. JACC Cardiovasc Interv 2019;12:569-78. [Crossref] [PubMed]
- Jabs A, von Bardeleben RS, Boekstegers P, et al. Effects of atrial fibrillation and heart rate on percutaneous mitral valve repair with MitraClip: results from the TRAnscatheter Mitral valve Interventions (TRAMI) registry. EuroIntervention 2017;12:1697-705. [Crossref] [PubMed]
- Shah S, Raj V, Abdelghany M, et al. Impact of atrial fibrillation on the outcomes of transcatheter mitral valve repair using MitraClip: a systematic review and meta-analysis. Heart Fail Rev 2021;26:531-43. [Crossref] [PubMed]
- Rabkin E, Aikawa M, Stone JR, et al. Activated interstitial myofibroblasts express catabolic enzymes and mediate matrix remodeling in myxomatous heart valves. Circulation 2001;104:2525-32. [Crossref] [PubMed]
- Guy TS, Hill AC. Mitral valve prolapse. Annu Rev Med 2012;63:277-92. [Crossref] [PubMed]
- Sticht C, De La Torre C, Parveen A, et al. miRWalk: An online resource for prediction of microRNA binding sites. PLoS One 2018;13:e0206239. [Crossref] [PubMed]
- Tokar T, Pastrello C, Rossos AEM, et al. mirDIP 4.1-integrative database of human microRNA target predictions. Nucleic Acids Res 2018;46:D360-70. [Crossref] [PubMed]
- Shirdel EA, Xie W, Mak TW, et al. NAViGaTing the micronome--using multiple microRNA prediction databases to identify signalling pathway-associated microRNAs. PLoS One 2011;6:e17429. [Crossref] [PubMed]
- Chen Y, Wang X. miRDB: an online database for prediction of functional microRNA targets. Nucleic Acids Res 2020;48:D127-31. [Crossref] [PubMed]
- Li JH, Liu S, Zhou H, et al. starBase v2.0: decoding miRNA-ceRNA, miRNA-ncRNA and protein-RNA interaction networks from large-scale CLIP-Seq data. Nucleic Acids Res 2014;42:D92-7. [Crossref] [PubMed]
- Paraskevopoulou MD, Georgakilas G, Kostoulas N, et al. DIANA-microT web server v5.0: service integration into miRNA functional analysis workflows. Nucleic Acids Res 2013;41:W169-73. [Crossref] [PubMed]
- Reczko M, Maragkakis M, Alexiou P, et al. Functional microRNA targets in protein coding sequences. Bioinformatics 2012;28:771-6. [Crossref] [PubMed]
- Vlachos IS, Zagganas K, Paraskevopoulou MD, et al. DIANA-miRPath v3.0: deciphering microRNA function with experimental support. Nucleic Acids Res 2015;43:W460-W466. [Crossref] [PubMed]
- Shannon P, Markiel A, Ozier O, et al. Cytoscape: a software environment for integrated models of biomolecular interaction networks. Genome Res 2003;13:2498-504. [Crossref] [PubMed]
- Chin CH, Chen SH, Wu HH, et al. cytoHubba: identifying hub objects and sub-networks from complex interactome. BMC Syst Biol 2014;8:S11. [Crossref] [PubMed]
- Bader GD, Hogue CW. An automated method for finding molecular complexes in large protein interaction networks. BMC Bioinformatics 2003;4:2. [Crossref] [PubMed]
- Davis AP, Grondin CJ, Johnson RJ, et al. Comparative Toxicogenomics Database (CTD): update 2021. Nucleic Acids Res 2021;49:D1138-43. [Crossref] [PubMed]
- Carbon S, Ireland A, Mungall CJ, et al. AmiGO: online access to ontology and annotation data. Bioinformatics 2009;25:288-9. [Crossref] [PubMed]
- Klein AL, Burstow DJ, Tajik AJ, et al. Age-related prevalence of valvular regurgitation in normal subjects: a comprehensive color flow examination of 118 volunteers. J Am Soc Echocardiogr 1990;3:54-63. [Crossref] [PubMed]
- Singh JP, Evans JC, Levy D, et al. Prevalence and clinical determinants of mitral, tricuspid, and aortic regurgitation (the Framingham Heart Study) Am J Cardiol 1999;83:897-902. [Crossref] [PubMed]
- Freed LA, Levy D, Levine RA, et al. Prevalence and clinical outcome of mitral-valve prolapse. N Engl J Med 1999;341:1-7. [Crossref] [PubMed]
- Levine RA, Triulzi MO, Harrigan P, et al. The relationship of mitral annular shape to the diagnosis of mitral valve prolapse. Circulation 1987;75:756-67. [Crossref] [PubMed]
- Lee AP, Hsiung MC, Salgo IS, et al. Quantitative analysis of mitral valve morphology in mitral valve prolapse with real-time 3-dimensional echocardiography: importance of annular saddle shape in the pathogenesis of mitral regurgitation. Circulation 2013;127:832-41. [Crossref] [PubMed]
- Tang Z, Fan YT, Wang Y, et al. Mitral Annular and Left Ventricular Dynamics in Atrial Functional Mitral Regurgitation: A Three-Dimensional and Speckle-Tracking Echocardiographic Study. J Am Soc Echocardiogr 2019;32:503-13. [Crossref] [PubMed]
- Alexiou C, Doukas G, Oc M, et al. The effect of preoperative atrial fibrillation on survival following mitral valve repair for degenerative mitral regurgitation. Eur J Cardiothorac Surg 2007;31:586-91. [Crossref] [PubMed]
- Pimor A, Galli E, Vitel E, et al. Predictors of post-operative cardiovascular events, focused on atrial fibrillation, after valve surgery for primary mitral regurgitation. Eur Heart J Cardiovasc Imaging 2019;20:177-84. [PubMed]
- Yoo JS, Kim JB, Jung SH, et al. Impact of the maze procedure and postoperative atrial fibrillation on progression of functional tricuspid regurgitation in patients undergoing degenerative mitral repair. Eur J Cardiothorac Surg 2013;43:520-5. [Crossref] [PubMed]
- Balachandran P, Schaff HV, Lahr BD, et al. Preoperative left atrial volume index is associated with postoperative outcomes in mitral valve repair for chronic mitral regurgitation. J Thorac Cardiovasc Surg 2020;160:661-672.e5. [Crossref] [PubMed]
- Vinereanu D, Wang A, Mulder H, et al. Outcomes in anticoagulated patients with atrial fibrillation and with mitral or aortic valve disease. Heart 2018;104:1292-9. [Crossref] [PubMed]
- Nakagami H, Yamamoto K, Ikeda U, et al. Mitral regurgitation reduces the risk of stroke in patients with nonrheumatic atrial fibrillation. Am Heart J 1998;136:528-32. [Crossref] [PubMed]
- Miyasaka Y, Tsuji H, Tokunaga S, et al. Mild mitral regurgitation was associated with increased prevalence of thromboembolic events in patients with nonrheumatic atrial fibrillation. Int J Cardiol 2000;72:229-33. [Crossref] [PubMed]
- Tsai FC, Chen YL, Yen KC, et al. Gene Expression Changes of Humans with Primary Mitral Regurgitation and Reduced Left Ventricular Ejection Fraction. Int J Mol Sci 2021;22:3454. [Crossref] [PubMed]
- Larupa Santos J, Rodríguez I. Investigating gene-microRNA networks in atrial fibrillation patients with mitral valve regurgitation. PLoS One 2020;15:e0232719. [Crossref] [PubMed]
- Driesbaugh KH, Branchetti E, Grau JB, et al. Serotonin receptor 2B signaling with interstitial cell activation and leaflet remodeling in degenerative mitral regurgitation. J Mol Cell Cardiol 2018;115:94-103. [Crossref] [PubMed]
- Zheng J, Chen Y, Pat B, et al. Microarray identifies extensive downregulation of noncollagen extracellular matrix and profibrotic growth factor genes in chronic isolated mitral regurgitation in the dog. Circulation 2009;119:2086-95. [Crossref] [PubMed]
- Bäck M, Pizarro R, Clavel MA. Biomarkers in Mitral Regurgitation. Prog Cardiovasc Dis 2017;60:334-41. [Crossref] [PubMed]
- Parwani P, Avierinos JF, Levine RA, et al. Mitral Valve Prolapse: Multimodality Imaging and Genetic Insights. Prog Cardiovasc Dis 2017;60:361-9. [Crossref] [PubMed]
- Zou R, Zhang D, Lv L, et al. Bioinformatic gene analysis for potential biomarkers and therapeutic targets of atrial fibrillation-related stroke. J Transl Med 2019;17:45. [Crossref] [PubMed]
- Li W, Wang L, Wu Y, et al. Weighted gene co-expression network analysis to identify key modules and hub genes associated with atrial fibrillation. Int J Mol Med 2020;45:401-16. [PubMed]
- Tan K, Markby G, Muirhead R, et al. Evaluation of canine 2D cell cultures as models of myxomatous mitral valve degeneration. PLoS One 2019;14:e0221126. [Crossref] [PubMed]
- Oceandy D, Yusoff R, Baudoin FM, et al. Promoter polymorphism of the matrix metalloproteinase 3 gene is associated with regurgitation and left ventricular remodelling in mitral valve prolapse patients. Eur J Heart Fail 2007;9:1010-7. [Crossref] [PubMed]
- Oral H, Sivasubramanian N, Dyke DB, et al. Myocardial proinflammatory cytokine expression and left ventricular remodeling in patients with chronic mitral regurgitation. Circulation 2003;107:831-7. [Crossref] [PubMed]
- Ilić D, Furuta Y, Kanazawa S, et al. Reduced cell motility and enhanced focal adhesion contact formation in cells from FAK-deficient mice. Nature 1995;377:539-44. [Crossref] [PubMed]
- Sabri A, Rafiq K, Seqqat R, et al. Sympathetic activation causes focal adhesion signaling alteration in early compensated volume overload attributable to isolated mitral regurgitation in the dog. Circ Res 2008;102:1127-36. [Crossref] [PubMed]
- Balachandran K, Bakay MA, Connolly JM, et al. Aortic valve cyclic stretch causes increased remodeling activity and enhanced serotonin receptor responsiveness. Ann Thorac Surg 2011;92:147-53. [Crossref] [PubMed]
- Yao C, Veleva T, Scott L Jr, et al. Enhanced Cardiomyocyte NLRP3 Inflammasome Signaling Promotes Atrial Fibrillation. Circulation 2018;138:2227-42. [Crossref] [PubMed]
- Goette A, Arndt M, Röcken C, et al. Calpains and cytokines in fibrillating human atria. Am J Physiol Heart Circ Physiol 2002;283:H264-72. [Crossref] [PubMed]
- Abe I, Teshima Y, Kondo H, et al. Association of fibrotic remodeling and cytokines/chemokines content in epicardial adipose tissue with atrial myocardial fibrosis in patients with atrial fibrillation. Heart Rhythm 2018;15:1717-27. [Crossref] [PubMed]
- Lavall D, Selzer C, Schuster P, et al. The mineralocorticoid receptor promotes fibrotic remodeling in atrial fibrillation. J Biol Chem 2014;289:6656-68. [Crossref] [PubMed]
- Chen Y, Surinkaew S, Naud P, et al. JAK-STAT signalling and the atrial fibrillation promoting fibrotic substrate. Cardiovasc Res 2017;113:310-20. [Crossref] [PubMed]
- Li B, Po SS, Zhang B, et al. Metformin regulates adiponectin signalling in epicardial adipose tissue and reduces atrial fibrillation vulnerability. J Cell Mol Med 2020;24:7751-66. [Crossref] [PubMed]
- Galeone A, Lessana A, Mascolo E, et al. Interleukin-1 receptor-related protein ST2 and mitral valve repair outcome in patients with chronic degenerative mitral regurgitation. Thorac Cardiovasc Surg 2014;62:47-51. [PubMed]
- Lu Q, Sun Y, Duan Y, et al. Comprehensive microRNA profiling reveals potential augmentation of the IL1 pathway in rheumatic heart valve disease. BMC Cardiovasc Disord 2018;18:53. [Crossref] [PubMed]
- Zhu AS, Mustafa T, Connell JP, et al. Tumor necrosis factor alpha and interleukin 1 beta suppress myofibroblast activation via nuclear factor kappa B signaling in 3D-cultured mitral valve interstitial cells. Acta Biomater 2021;127:159-68. [Crossref] [PubMed]
- Sun Z, Zhou D, Xie X, et al. Cross-talk between macrophages and atrial myocytes in atrial fibrillation. Basic Res Cardiol 2016;111:63. [Crossref] [PubMed]
- Kawada JI, Takeuchi S, Imai H, et al. Immune cell infiltration landscapes in pediatric acute myocarditis analyzed by CIBERSORT. J Cardiol 2021;77:174-8. [Crossref] [PubMed]
- Huang R, Mao M, Lu Y, et al. A novel immune-related genes prognosis biomarker for melanoma: associated with tumor microenvironment. Aging (Albany NY) 2020;12:6966-80. [Crossref] [PubMed]
- Lin X, Deng J, Deng H, et al. Comprehensive Analysis of the Immune Microenvironment in Checkpoint Inhibitor Pneumonitis. Front Immunol 2021;12:818492. [Crossref] [PubMed]
- Toor D, Vohra H. Immune responsiveness during disease progression from acute rheumatic fever to chronic rheumatic heart disease. Microbes Infect 2012;14:1111-7. [Crossref] [PubMed]
- Kemeny E, Grieve T, Marcus R, et al. Identification of mononuclear cells and T cell subsets in rheumatic valvulitis. Clin Immunol Immunopathol 1989;52:225-37. [Crossref] [PubMed]
- Liu Z, Wang Y, Li F, et al. Circulating follicular T helper cells and humoral reactivity in rheumatic heart disease. Life Sci 2020;245:117390. [Crossref] [PubMed]
(English Language Editor: J. Teoh)