Classification of solid pulmonary nodules using a machine-learning nomogram based on 18F-FDG PET/CT radiomics integrated clinicobiological features
Introduction
Lung cancer is the leading cause of cancer-related mortality globally, accounting for 1.3 million deaths per year (1). The overall mortality has decreased significantly in recent years due to the improvements in early detection and treatment advances that have extended survival (2). However, with an estimated 1.5 million new pulmonary nodules detected through screening or incidentally requiring follow-up diagnostic procedures annually (3,4), the task of classifying benign pulmonary nodules (BPN) from malignant pulmonary nodules (MPN) to avoid delayed treatment or overdiagnosis presents a great challenge for radiologists and clinicians.
Biopsy is considered the “golden standard” for insight into possible cancerous, but the application of this invasive procedure is limited due to complications after sampling or contraindications for patients with severe cardiopulmonary insufficiency (5). Noninvasive radiologic approaches present alternative options to tissue-based procedures. Computed tomography (CT) and 18F-fluorodeoxyglucose (18F-FDG) positron emission tomography (PET) are most commonly used in clinical practice (6), while PET/CT is more advanced than CT in assessing the malignancy risk of solid pulmonary nodules with higher sensitivity and accuracy values (7,8). However, the high false positive rate (FPR) of PET/CT can not to be ignored because 18F-FDG is not a tumor-specific tracer (9). In addition, there are no completely specific radiologic features or imaging diagnostic criteria for MPNs.
Machine learning-based radiomics is particularly suitable for the assessment and management of pulmonary nodules by providing high-dimensional and valuable data, such as intranodular heterogeneity, which can recognize biomarkers that reduce false-positive results of diagnostic imaging and more accurately distinguish between BPN and MPN (10-12). In addition, radiomics features can be combined with demographic, histologic or proteomic data, which can make a more quantitative risk prediction of lung cancer and more defined clinical decision guidelines (13).
We aimed to construct a machine-learning model that integrated the clinical factors, serum tumor marker, PET/CT metabolic features and radiomics features to distinguish BPN from MPN and provide a visually quantitative nomogram in clinical practice. We present the following article in accordance with the TRIPOD reporting checklist (available at https://atm.amegroups.com/article/view/10.21037/atm-22-2647/rc) (14).
Methods
Patients
We conducted the retrospective analysis of records from patients with a solitary solid pulmonary nodule between January 2017 and December 2020. The study was conducted in accordance with the Declaration of Helsinki (as revised in 2013). The Ethics Committee of Shanghai Proton and Heavy Ion Center (No. 200217EXP-01) approved this retrospective study, and waived the demand for informed consent. The inclusion criteria were as follows: (I) BPN or MPN diagnosed by pathology of curative surgical resection; (II) whole-body 18F-FDG PET/CT less than 2 weeks before surgery; (III) solitary solid pulmonary nodule larger than 1.0 cm in diameter with maximum standardized uptake value (SUVmax) >1.0. The exclusion criteria and patient recruitment process were presented in Figure 1.
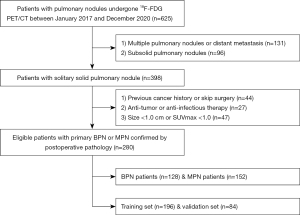
Finally, a total of 280 consecutive patients were enrolled in this study, comprising 128 BPNs and 152 MPNs. Patients were randomly divided into the training set (n=196) and validation set (n=84) according to a 7:3 ratio. Baseline data pertaining to the clinical characteristics and serum tumor marker levels of each patient were reviewed and recorded.
18F-FDG PET/CT examination
All patients were received the 18F-FDG PET/CT on a Biograph 64 PET/CT scanner (Siemens Healthcare, Erlangen, Germany) with a blood glucose level of <8.7 mmol/L fasted for at least 6 h before the scan. After intravenous administration of 5.18 MBq/kg of 18F-FDG, the whole-body scan was operated about 1 h later. First, CT scanning with 3.0 mm slice thickness (120 kVp, 150 mAs, 0.33 seconds per rotation) was performed and reconstructed to a 512×512 matrix (voxel size: 0.98×0.98×3.0 mm3). PET scanning was subsequently performed with 2 min in each bed and reconstructed using the TrueX algorithm (2 iterations, 24 subsets and 2 mm full width at half maximum, matrix size: 200×200, anisotropic voxels: 4.07×4.07×3.0 mm3). The PET images were transformed into SUV units by standardizing the activity concentration into the injected 18F-FDG’ dosage and patients’ weight.
Pulmonary nodule segmentation and PET/CT image evaluation
Pulmonary nodules were visualized and segmented on the 18F-FDG PET/CT images using Inveon Research Workplace software (Siemens Healthcare, Erlangen, Germany). The boundaries of nodules were drawn in the axial, coronal, and sagittal PET scans to delineate the volume of interest (VOI) using a threshold of 40% of SUVmax by two experienced nuclear medicine physicians blinded to the pathology and with consensus decision (15). The joint reading of the PET and CT scan was performed in parallel to avoid incorporating the areas of physiological 18F-FDG uptake into VOI and ensure that the accurate anatomical tumor borders were fully reflected.
Six metabolic parameters of 18F-FDG uptake [minimum SUV (SUVmin), SUVmax, SUVmean, SUV standard deviation (SD), metabolic tumor volume (MTV) and total lesion glycolysis (TLG)] that are generally used for the measurement of intratumoral heterogeneity were recorded for each VOI. The SUVmax threshold value of the pulmonary nodule was set at 2.5 to differentiate BPN from MPN according to previous studies (16,17). An SUVmax of ≥2.5 was considered MPN, while an SUVmax of <2.5 was considered BPN.
Quantitative radiomics feature extraction
A total of 157 quantitative radiomics features (100 PET radiomics features and 57 CT radiomics features) were automatically calculated and extracted from the VOI using the Chang Gung Image Texture Analysis (CGITA) (18). The extraction and definition of these radiomics features were consistent with the Imaging Biomarker Standardization Initiative (19), and the details of these features were described in Table S1 of supplementary data.
Feature selection and model development
The optimum features for predicting the histologic subtypes were selected using a machine-learning method [least absolute shrinkage and selection operator (LASSO) regression with 10-fold cross-validation] in the training set (20). The prediction models for distinguishing BPN and MPN patients were constructed by the linear fusion of the selected non-zero features weighted by their coefficients, and the prediction scores (Pre-scores) of each model were calculated for each patient.
Model performance and clinical utility evaluation
The receiver-operator characteristic curve (ROC) analysis and DeLong test were applied to evaluate and compare the performance of these prediction models in the training and validation sets. The area under the curve (AUC) with 95% confidence interval (CI), sensitivity, specificity, accuracy, positive predictive value (PPV), negative predictive value (NPV), FPR and false negative rate (FNR) were calculated for each model. The decision curve analysis (DCA) and clinical impact curve (CIC) were used to evaluate the clinical utility of these models (21).
Nomogram development and validation
To provide a visually quantitative tool for distinguishing BPN from MPN, an individualized nomogram was developed using the factors of the model with the highest efficiency and clinical utility (22). Calibration curves were drawn using 1,000 bootstrap resamples based on the internal (training set) and external (validation set) validity to reflect the agreement between the actual probability and the predicted probability of the nomogram.
Statistical analysis
The statistical analysis was performed on R (version 4.0, http://www.r-project.org) software. Comparisons between the groups were performed using Mann-Whitney U test or independent t-test for continuous variables and χ2 test or Fisher’s exact test for categorical variables. A two-sided P value of <0.05 indicated statistical significance.
Results
Demographic, clinicopathologic and metabolic characteristics of patients
In total, 280 patients comprising 128 BPN and 152 MPN patients (146 males and 134 females, mean age: 59.79±10.26 years, range: 26–84 years) were eventually enrolled in this study. Among the BPN patients, 64 (50.0%) had a final diagnosis of inflammation, 40 (31.3%) of granuloma, 9 (7.0%) of active tuberculosis, 9 (7.0%) of hamartoma and 6 (4.7%) of sclerosing pulmonary cell tumor. Among the MPN patients, the most common histologic subtype was adenocarcinoma (n=77, 50.7%), followed by squamous cell carcinoma (n=59, 38.8%). Rarer cases of small cell lung cancer (n=8, 5.3%), large cell carcinoma (n=6, 3.9%) and sarcomatoid carcinoma (n=2, 1.3%) were reported. The patients’ demographic, clinico-biological and metabolic characteristics were summarized and compared in Table 1.
Table 1
Characteristics | Training set (n=196) | Validation set (n=84) | |||||
---|---|---|---|---|---|---|---|
BPN (n=91) | MPN (n=105) | P | BPN (n=37) | MPN (n=47) | P | ||
Sex | 0.57 | <0.01 | |||||
Male | 47 (51.65) | 59 (56.19) | 11 (29.73) | 29 (61.70) | |||
Female | 44 (48.35) | 46 (43.81) | 26 (70.27) | 18 (38.30) | |||
Age (years) | 55.88±10.17† | 63.10±9.97† | <0.01 | 54.89±8.93† | 63.81±7.58† | <0.01 | |
Height (m) | 1.63±0.08† | 1.64±0.08† | 0.43 | 1.62±0.06† | 1.65±0.08† | 0.13 | |
Weight (kg) | 64.85±11.55† | 62.08±9.57† | 0.07 | 60.74±8.27† | 63.81±9.49† | 0.12 | |
BMI | 24.16±3.04† | 22.97±3.06† | 0.01 | 23.08±3.01† | 23.45±2.73† | 0.55 | |
Smoking | 0.04 | <0.01 | |||||
Never | 61 (67.03) | 55 (52.38) | 30 (81.08) | 21 (44.68) | |||
Ever/always | 30 (32.97) | 50 (47.62) | 7 (18.92) | 26 (55.32) | |||
Symptom | 0.25 | 0.40 | |||||
Negative | 43 (47.25) | 41 (39.05) | 17 (45.95) | 26 (55.32) | |||
Positive | 48 (52.75) | 64 (60.95) | 20 (54.05) | 21 (44.68) | |||
Family history | 0.13 | 0.07 | |||||
Negative | 88 (96.70) | 96 (91.43) | 37 (100.00) | 43 (91.49) | |||
Positive | 3 (3.30) | 9 (8.57) | 0 (0.00) | 4 (8.51) | |||
Tumor side | 0.80 | 0.83 | |||||
Right lung | 53 (58.24) | 63 (60.00) | 18 (48.65) | 24 (51.06) | |||
Left lung | 38 (41.76) | 42 (40.00) | 19 (51.35) | 23 (48.94) | |||
Tumor location | 0.13 | <0.01 | |||||
Upper lobe | 54 (59.34) | 52 (49.52) | 14 (37.84) | 33 (70.21) | |||
Middle lobe | 10 (10.99) | 11 (10.48) | 1 (2.70) | 2 (4.26) | |||
Lower lobe | 27 (29.67) | 42 (40.00) | 22 (59.46) | 12 (25.53) | |||
Tumor size (cm) | 3.70±1.41† | 5.47±1.98† | <0.01 | 3.95±1.42† | 4.18±1.77† | 0.15 | |
FERR (ng/mL) | 234.60 (163.00, 376.90)‡ | 218.90 (130.35, 331.35)‡ | 0.02 | 234.60 (87.30, 234.60)‡ | 220.00 (132.00, 316.00)‡ | 0.05 | |
SCCA (ng/mL) | 0.90 (0.68, 1.20)‡ | 1.10 (0.70, 1.70)‡ | 0.21 | 0.80 (0.52, 0.89)‡ | 0.87 (0.62, 1.55)‡ | <0.01 | |
CA199 (U/mL) | 12.03 (8.22, 17.70)‡ | 11.04 (6.35, 17.50)‡ | 0.58 | 12.10 (8.08, 14.09) ‡ | 9.97 (6.07, 17.60) ‡ | 0.82 | |
CEA (ng/mL) | 2.04 (1.43, 2.96)‡ | 3.09 (1.98, 4.88)‡ | <0.01 | 1.70 (0.97, 2.04)‡ | 3.22 (2.08, 4.60)‡ | 0.02 | |
CYFRA21-1 (ng/mL) | 2.48 (2.20, 3.21)‡ | 3.24 (2.41, 5.18)‡ | <0.01 | 2.46 (1.75, 2.95)‡ | 2.86 (1.93, 4.29)‡ | 0.29 | |
NSE (ng/mL) | 10.73 (9.52, 12.09)‡ | 11.24 (10.17, 13.10)‡ | 0.44 | 11.99 (10.16, 12.82)‡ | 11.20 (9.52, 12.60)‡ | 0.48 | |
SUVmax threshold | <0.01 | 0.45 | |||||
<2.5 | 23 (25.27) | 10 (9.52) | 7 (18.92) | 6 (12.77) | |||
≥2.5 | 68 (74.73) | 95 (90.48) | 30 (81.08) | 41 (87.23) | |||
SUVmin | 0.90±0.43† | 1.13±0.59† | <0.01 | 0.81±0.36† | 1.14±0.69† | 0.01 | |
SUVmax | 6.21±5.02† | 8.17±5.08† | <0.01 | 5.94±3.69† | 9.50±7.09† | <0.01 | |
SUVmean | 2.97±2.17† | 4.25±2.76† | <0.01 | 2.72±1.42† | 4.62±3.39† | <0.01 | |
SUV SD | 1.22±1.15† | 1.65±1.11† | <0.01 | 1.16±0.86† | 1.97±1.67† | <0.01 | |
MTV | 13.86±18.51† | 20.51±30.10† | 0.07 | 19.32±33.47† | 18.68±38.77† | 0.94 | |
TLG | 58.13±103.25† | 122.87±245.36† | 0.02 | 72.88±175.68† | 137.33±374.88† | 0.34 |
Data are shown as mean ± standard deviation, median (interquartile range) or number (percentage). P values were the results of univariate analysis and the bold ones indicated statistical significance. BPN, benign pulmonary nodule; MPN, malignant pulmonary nodule; SD, standard deviation; BMI, body mass index; FERR, ferritin; SCCA, squamous cell carcinoma antigen; CA, carbohydrate antigen; CEA, carcinoembryonic antigen; CYFRA21-1, cytokeratin 19 fragment antigen; NSE, neuron specific enolase; SUV, standardized uptake value; MTV, metabolic tumor volume; TLG, total lesion glycolysis.
Feature selection and model development
Originally, 7 clinico-biological markers, 14 PET/CT radiomics features and 26 combination features were selected by the LASSO method in the training set (Figure 2). Subsequently, we developed 3 independent multivariable models using the most valuable 4 clinico-biological features, 7 radiomics features and 12 combination features according to the Akaike information criterion (AIC) for distinguishing BPN from MPN patients. Generally, MPN patients had higher Pre-scores than BPN patients for each model calculated by the following formulas (P<0.01) (Figure 3, Table 2):
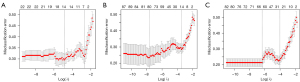
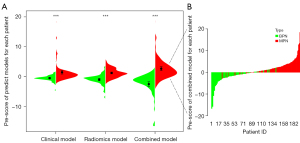
Table 2
Pre-score | BPN (n=91) | MPN (n=105) | P |
---|---|---|---|
Pre-score (clinical model) | −0.59±1.17 | 1.47±3.06 | <0.01 |
Pre-score (radiomics model) | −0.97±1.78 | 1.23±1.51 | <0.01 |
Pre-score (combined model) | −2.49±3.85 | 2.69±3.41 | <0.01 |
Data is presented as mean ± standard deviation. P values were the results of univariate analysis and the bold ones indicated statistical significance. BPN, benign pulmonary nodule; MPN, malignant pulmonary nodule.
Pre-score (Clinical Model) = −4.637 + 0.064 × age (y) − 0.002 × ferritin (FERR, ng/mL) + 0.199 × carcinoembryonic antigen (CEA, ng/mL) + 0.267 × cytokeratin 19 fragment antigen (CYFRA21-1, ng/mL).
Pre-score (Radiomics Model) = 13.614 − 0.371 × PET_Kurtosis − 28.346 × PET_Texture Feature Coding (TFC)_Coarseness − 0.023 × PET_TFC_Mean convergence + 37.651 × PET_Texture Feature Coding Cooccurrence Matrix (TFCCM)_Second angular moment + 119.249 × PET_TFCCM_Code Similarity − 18.044 × CT_Gray Level Size Zone Matrix (GLSZM)_Short zone emphasis + 20.031 × CT_Texture Spectrum (TS)_Max spectrum.
Pre-score (Combined Model) = 9.374 + 1.922 × SUVmax threshold (SUVmax <2.5:0, SUVmax ≥2.5:1) + 0.070 × age (y) + 1.447 × smoking (never: 0, ever/always: 1) − 0.004 × FERR (ng/mL) + 0.283 × CEA (ng/mL) − 0.924 × PET_Kurtosis + 1.166 × PET_Kurtosisbias corrected − 0.021 × PET_TFC_Mean convergence + 34.521 × PET_TFCCM_Second angular moment + 139.095 × PET_TFCCM_Code Similarity − 24.389 × CT_ GLSZM_Short zone emphasis + 21.559 × CT_TS_Max spectrum.
Model performance and clinical utility evaluation
All the prediction models were significantly associated with the pathological subtypes of solid pulmonary nodules, while the SUVmax Threshold approach displayed the poorest performance with the lowest AUC, specificity and accuracy values in the two sets (P<0.05). However, the SUVmax Threshold approach had the highest sensitivity value among these prediction models. The DeLong test showed that the Combined Model held the highest AUC and best prediction specificity, accuracy, PPV and NPV for discriminating BPN from MPN among the 3 models in both the training and validation sets (AUCs of 0.91 and 0.94, respectively) (Figure 4A,4B, Table 3). In addition, the FPR of the Combined Model in the diagnosis of MPN was remarkably decreased compared to the other 3 classifiers (SUVmax Threshold approach, Clinical Model and Radiomics Model). In addition, when the threshold probability was greater than 15%, the Combined Model was demonstrated to be the most reliable tool for assessing the malignancy risk in patients with solid pulmonary nodules according to the DCA and CIC (Figure 4C,4D).
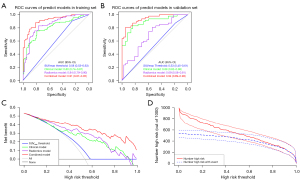
Table 3
Models | AUC (95% CI) | SEN (%) | SPE (%) | ACC (%) | PPV (%) | NPV (%) | FPR (%) | FNR (%) |
---|---|---|---|---|---|---|---|---|
Training set | ||||||||
SUVmax threshold | 0.58 (0.53–0.63) | 90.48 | 25.27 | 60.20 | 58.28 | 69.70 | 74.73 | 9.52 |
Clinical model | 0.80 (0.74–0.87) | 75.24 | 79.12 | 77.04 | 80.61 | 73.47 | 20.88 | 24.76 |
Radiomics model | 0.84 (0.79–0.90) | 82.86 | 76.92 | 80.10 | 80.56 | 79.55 | 23.08 | 17.14 |
Combined model | 0.91 (0.87–0.95) | 87.62 | 81.32 | 84.69 | 84.40 | 85.06 | 18.68 | 12.38 |
Validation set | ||||||||
SUVmax threshold | 0.53 (0.45–0.61) | 87.23 | 18.92 | 57.14 | 57.75 | 53.85 | 81.08 | 12.77 |
Clinical model | 0.89 (0.82–0.96) | 82.98 | 83.78 | 83.33 | 86.67 | 79.49 | 16.22 | 17.02 |
Radiomics model | 0.69 (0.58–0.81) | 80.85 | 54.05 | 69.05 | 69.09 | 68.97 | 45.95 | 19.15 |
Combined model | 0.94 (0.89–0.98) | 80.85 | 94.59 | 86.90 | 95.00 | 79.55 | 5.41 | 19.15 |
AUC, area under the receiver operating curve; CI, confidence interval; SEN, sensitivity; SPE, specificity; ACC, accuracy; PPV, positive predictive value; NPV, negative predictive value; FPR, false positive rate; FNR, false negative rate; SUV, standardized uptake value.
Nomogram development and validation
Thus, we constructed a machine-learning nomogram consisting of the Combined Model’s risk factors for visualization according to the above results (Figure 5A), and a good consistency between the predictions and the actual observations was validated by the calibration curves (Figure 5B,5C).
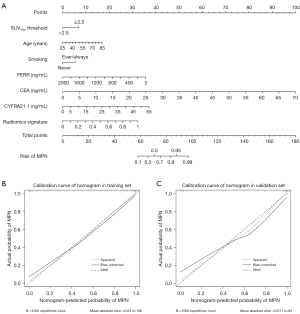
Discussion
In this study, we successfully developed and validated a machine-learning nomogram consisting of clinico-biological factors, tumor markers and 18F-FDG PET/CT radiomics features, which demonstrated an excellent performance in noninvasively distinguishing between BPN and MPN patients.
The relationship between the clinical factors selected in the nomogram (age and smoking status) and lung cancer risk has previously been well established: an accelerating increase in risk associated with advancing age and smoking (1,23). The serum tumor markers such as CEA and CYFRA21-1 also contribute to the diagnosis of lung cancer, and MPN patients generally have significantly higher levels of those markers than BPN patients (24,25). Serum FERR, a non-specific tumor marker, is highly expressed in benign lung diseases, which may be the result of inflammation and oxidative stress (26,27). This study’s results were corresponded to the above reports’ conclusions.
As a functional imaging modality, 18F-FDG PET/CT can provide additional metabolic information that enables more accurate characterization of pulmonary nodules that are indeterminate on CT images, which frequently alters the clinical management strategies (16,28). Malignant tissues consist of metabolically active cells that usually have a higher uptake of glucose than benign ones, which can be measured semiquantitatively using SUV values: the risk of malignancy increases with SUV values (29,30). In this study, all SUV values of MPN were significantly higher than those of BPN, which was consistent with the above reports. Despite SUV values being frequently correlated with the biological behaviors of malignant tissues, the definitive threshold SUV values for malignancy diagnosis have not yet been identified (31). Previous studies have adopted 2.5 as a threshold SUVmax to discriminate between patients with BPN and MPN and obtained different results (17,32). In this study, a SUVmax of 2.5 was applied to classify the nodule subtypes and performed poorly in both the training and validation sets (AUCs of 0.58 and 0.53, respectively). The above results indicated that using the threshold SUVmax values to differentiate BPN from MPN was unrealistic.
Radiomics based on the 18F-FDG PET/CT has been demonstrated to have potential in assessing the lung cancer risk and could optimize the end-to-end diagnosis-treatment-follow-up chain (33). Previous studies found that PET/CT radiomics could differentiate BPN from MPN patients with AUCs >0.8 or an accuracy value of 80.40% (34,35). However, these studies did not validate the results in another independent (internal or external) dataset. In this study, the Radiomics Model performed better in differentiating BPN from MPN in the training set (AUC of 0.84, accuracy of 80.10%, respectively) than in the validation set (AUC of 0.69, accuracy of 69.05%, respectively).
Furthermore, we combined the radiomics data with clinico-biological features. The Combined Model established in this study, which consisted of the SUVmax Threshold approach, 4 clinico-biological markers and 7 PET/CT radiomics parameters, not only significantly improved the prediction efficiency for MPN compared to each factor alone and performed better than the aforementioned studies (AUCs of 0.91 and 0.94, accuracy of 84.69% and 86.90%, respectively), but also remarkably decreased the FPR values in both the training and validation sets (FPRs of 18.68% and 5.41%, respectively). This discrepancy may be related to the fact that intranodular heterogeneity can be evaluated more comprehensively by combining with the multiscale characteristics (36).
According to the above results, we constructed a quantitative nomogram that integrated clinico-biologico-radiological features to provide a noninvasive and accurate tool for assessing the malignancy risk and guiding clinical decisions for patients with solid pulmonary nodule (37). When the probability of lung cancer was >15%, the nomogram could add more benefit than either the treat-all-patients as BPN or the treat-all-patients as MPN, which was more valuable for the current trend toward personalized medicine (38).
There were some limitations of this study. Firstly, this retrospective study was designed in a single center, which could lead to the sample selection bias. It is necessary to design another prospective study incorporating multiple centers to further validate the performance and generalization ability of the models developed in this study. Secondly, we excluded patients with multiple solid nodules, or small nodules with faint 18F-FDG uptake to ensure the relationship between baselined clinico-biologico-radiological features and single pathological subtype and the image data quality. The prediction model used for these patients will be continually explored in future work. Thirdly, the supervised classification approach was used to identify the key features for predicting the target variable in this study. However, the latent relations between different feature types did not been explored. How to integrate features obtained across imaging, molecular and clinical modalities more reasonably to improve the differentiation ability and clarify the potential biological characteristics of tumor will be an important direction for future work.
In conclusion, a machine-learning nomogram was developed and validated in our study, which could improve the diagnostic efficiency and reduce the FPR in the noninvasive differentiation of BPN from MPN patients.
Acknowledgments
Funding: This study was supported by Shanghai Sailing Program (No. 21YF1444300).
Footnote
Reporting Checklist: The authors have completed the TRIPOD reporting checklist. Available at https://atm.amegroups.com/article/view/10.21037/atm-22-2647/rc
Data Sharing Statement: Available at https://atm.amegroups.com/article/view/10.21037/atm-22-2647/dss
Peer Review File: Available at https://atm.amegroups.com/article/view/10.21037/atm-22-2647/prf
Conflicts of Interest: All authors have completed the ICMJE uniform disclosure form (available at https://atm.amegroups.com/article/view/10.21037/atm-22-2647/coif). The authors have no conflicts of interest to declare.
Ethical Statement: The authors are accountable for all aspects of the work in ensuring that questions related to the accuracy or integrity of any part of the work are appropriately investigated and resolved. The study was conducted in accordance with the Declaration of Helsinki (as revised in 2013). The study was approved by the Ethics Committee of Shanghai Proton and Heavy Ion Center
Open Access Statement: This is an Open Access article distributed in accordance with the Creative Commons Attribution-NonCommercial-NoDerivs 4.0 International License (CC BY-NC-ND 4.0), which permits the non-commercial replication and distribution of the article with the strict proviso that no changes or edits are made and the original work is properly cited (including links to both the formal publication through the relevant DOI and the license). See: https://creativecommons.org/licenses/by-nc-nd/4.0/.
References
- Siegel RL, Miller KD, Fuchs HE, et al. Cancer statistics, 2022. CA Cancer J Clin 2022;72:7-33. [Crossref] [PubMed]
- Horeweg N, van der Aalst CM, Thunnissen E, et al. Characteristics of lung cancers detected by computer tomography screening in the randomized NELSON trial. Am J Respir Crit Care Med 2013;187:848-54. [Crossref] [PubMed]
- Gould MK, Tang T, Liu IL, et al. Recent Trends in the Identification of Incidental Pulmonary Nodules. Am J Respir Crit Care Med 2015;192:1208-14. [Crossref] [PubMed]
- MacMahon H, Naidich DP, Goo JM, et al. Guidelines for Management of Incidental Pulmonary Nodules Detected on CT Images: From the Fleischner Society 2017. Radiology 2017;284:228-43. [Crossref] [PubMed]
- de Margerie-Mellon C, de Bazelaire C, de Kerviler E. Image-guided biopsy in primary lung cancer: Why, when and how. Diagn Interv Imaging 2016;97:965-72. [Crossref] [PubMed]
- Vlahos I, Stefanidis K, Sheard S, et al. Lung cancer screening: nodule identification and characterization. Transl Lung Cancer Res 2018;7:288-303. [Crossref] [PubMed]
- Kim TJ, Kim CH, Lee HY, et al. Management of incidental pulmonary nodules: current strategies and future perspectives. Expert Rev Respir Med 2020;14:173-94. [Crossref] [PubMed]
- Li W, Pang H, Liu Q, et al. The role of F-18-FDG PET or F-18-FDG-PET/CT in the evaluation of solitary pulmonary nodules. Eur J Radiol 2015;84:2032-7. [Crossref] [PubMed]
- Maiga AW, Deppen SA, Mercaldo SF, et al. Assessment of Fluorodeoxyglucose F18-Labeled Positron Emission Tomography for Diagnosis of High-Risk Lung Nodules. JAMA Surg 2018;153:329-34. [Crossref] [PubMed]
- Lambin P, Rios-Velazquez E, Leijenaar R, et al. Radiomics: extracting more information from medical images using advanced feature analysis. Eur J Cancer 2012;48:441-6. [Crossref] [PubMed]
- Thawani R, McLane M, Beig N, et al. Radiomics and radiogenomics in lung cancer: A review for the clinician. Lung Cancer 2018;115:34-41. [Crossref] [PubMed]
- Liu A, Wang Z, Yang Y, et al. Preoperative diagnosis of malignant pulmonary nodules in lung cancer screening with a radiomics nomogram. Cancer Commun (Lond) 2020;40:16-24. [Crossref] [PubMed]
- Bi WL, Hosny A, Schabath MB, et al. Artificial intelligence in cancer imaging: Clinical challenges and applications. CA Cancer J Clin 2019;69:127-57. [Crossref] [PubMed]
- Collins GS, Reitsma JB, Altman DG, et al. Transparent Reporting of a multivariable prediction model for Individual Prognosis or Diagnosis (TRIPOD): the TRIPOD statement. Ann Intern Med 2015;162:55-63. [Crossref] [PubMed]
- Bashir U, Azad G, Siddique MM, et al. The effects of segmentation algorithms on the measurement of 18F-FDG PET texture parameters in non-small cell lung cancer. EJNMMI Res 2017;7:60. [Crossref] [PubMed]
- Christensen JA, Nathan MA, Mullan BP, et al. Characterization of the solitary pulmonary nodule: 18F-FDG PET versus nodule-enhancement CT. AJR Am J Roentgenol 2006;187:1361-7. [Crossref] [PubMed]
- Sim YT, Goh YG, Dempsey MF, et al. PET-CT evaluation of solitary pulmonary nodules: correlation with maximum standardized uptake value and pathology. Lung 2013;191:625-32. [Crossref] [PubMed]
- Fang YH, Lin CY, Shih MJ, et al. Development and evaluation of an open-source software package "CGITA" for quantifying tumor heterogeneity with molecular images. Biomed Res Int 2014;2014:248505. [Crossref] [PubMed]
- Zwanenburg A, Vallières M, Abdalah MA, et al. The Image Biomarker Standardization Initiative: Standardized Quantitative Radiomics for High-Throughput Image-based Phenotyping. Radiology 2020;295:328-38. [Crossref] [PubMed]
- Chen LY, Li JT, Chang MM. Cancer Diagnosis and Disease Gene Identification via Statistical Machine Learning. Curr Bioinform 2020;15:956-62. [Crossref]
- Kerr KF, Brown MD, Zhu K, et al. Assessing the Clinical Impact of Risk Prediction Models With Decision Curves: Guidance for Correct Interpretation and Appropriate Use. J Clin Oncol 2016;34:2534-40. [Crossref] [PubMed]
- Balachandran VP, Gonen M, Smith JJ, et al. Nomograms in oncology: more than meets the eye. Lancet Oncol 2015;16:e173-80. [Crossref] [PubMed]
- Oberg M, Jaakkola MS, Woodward A, et al. Worldwide burden of disease from exposure to second-hand smoke: a retrospective analysis of data from 192 countries. Lancet 2011;377:139-46. [Crossref] [PubMed]
- Yang G, Xiao Z, Tang C, et al. Recent advances in biosensor for detection of lung cancer biomarkers. Biosens Bioelectron 2019;141:111416. [Crossref] [PubMed]
- Okamura K, Takayama K, Izumi M, et al. Diagnostic value of CEA and CYFRA 21-1 tumor markers in primary lung cancer. Lung Cancer 2013;80:45-9. [Crossref] [PubMed]
- Wang X, Zhang Y, Sun L, et al. Evaluation of the clinical application of multiple tumor marker protein chip in the diagnostic of lung cancer. J Clin Lab Anal 2018;32:e22565. [Crossref] [PubMed]
- Chen Z, Zhu B, Ou C, et al. Serum ferritin and primary lung cancer. Oncotarget 2017;8:92643-51. [Crossref] [PubMed]
- Groheux D, Quere G, Blanc E, et al. FDG PET-CT for solitary pulmonary nodule and lung cancer: Literature review. Diagn Interv Imaging 2016;97:1003-17. [Crossref] [PubMed]
- Boellaard R, Delgado-Bolton R, Oyen WJ, et al. FDG PET/CT: EANM procedure guidelines for tumour imaging: version 2.0. Eur J Nucl Med Mol Imaging 2015;42:328-54. [Crossref] [PubMed]
- Bai B, Bading J, Conti PS. Tumor quantification in clinical positron emission tomography. Theranostics 2013;3:787-801. [Crossref] [PubMed]
- Ziai P, Hayeri MR, Salei A, et al. Role of Optimal Quantification of FDG PET Imaging in the Clinical Practice of Radiology. Radiographics 2016;36:481-96. [Crossref] [PubMed]
- Divisi D, Barone M, Bertolaccini L, et al. Standardized uptake value and radiological density attenuation as predictive and prognostic factors in patients with solitary pulmonary nodules: our experience on 1,592 patients. J Thorac Dis 2017;9:2551-9. [Crossref] [PubMed]
- Anan N, Zainon R, Tamal M. A review on advances in F-18-FDG PET/CT radiomics standardisation and application in lung disease management. Insights into Imaging 2022;13:22. [Crossref] [PubMed]
- Albano D, Gatta R, Marini M, et al. Role of 18F-FDG PET/CT Radiomics Features in the Differential Diagnosis of Solitary Pulmonary Nodules: Diagnostic Accuracy and Comparison between Two Different PET/CT Scanners. J Clin Med 2021;10:5064. [Crossref] [PubMed]
- Palumbo B, Bianconi F, Palumbo I, et al. Value of Shape and Texture Features from 18F-FDG PET/CT to Discriminate between Benign and Malignant Solitary Pulmonary Nodules: An Experimental Evaluation. Diagnostics (Basel) 2020;10:696. [Crossref] [PubMed]
- Lv W, Yuan Q, Wang Q, et al. Radiomics Analysis of PET and CT Components of PET/CT Imaging Integrated with Clinical Parameters: Application to Prognosis for Nasopharyngeal Carcinoma. Mol Imaging Biol 2019;21:954-64. [Crossref] [PubMed]
- Salem A, Asselin MC, Reymen B, et al. Targeting Hypoxia to Improve Non-Small Cell Lung Cancer Outcome. J Natl Cancer Inst 2018;110:14-30. [Crossref] [PubMed]
- Rocco G, Morabito A, Leone A, et al. Management of non-small cell lung cancer in the era of personalized medicine. Int J Biochem Cell Biol 2016;78:173-9. [Crossref] [PubMed]