Bioinformatics analysis of the prognostic biomarkers and predictive accuracy of differentially expressed genes in high-risk multiple myeloma based on Gene Expression Omnibus database mining
Introduction
Multiple myeloma (MM) is an abnormal proliferative malignancy originating from plasma cells, accounting for 10–15% of hematological malignancies. MM is characterized by abnormal infiltration of clonal plasma cells in bone marrow, and is the second most common hematological malignancy after non-Hodgkin’s lymphoma (1-3). In recent years, the diagnosis and treatment of MM patients have consistently improved. However, about 20–30% of MM patients still have progression-free survival (PFS) less than 1.5 years and overall survival (OS) less than 2–3 years (4). These MM patients have severe clinical manifestations, short survival time, are insensitive to standard treatment, and have poor prognosis, and are considered as high-risk MM (5).
The pathogenesis of MM is complex, and cytogenetic abnormalities play a crucial role in the risk stratification of MM. Several cytogenetic abnormalities, such as t(4;14), del(17/17p), t(14;16), and t(14;20), are closely associated with poor prognosis (5,6) . According to the International Myeloma Working Group (IMWG), myeloma cells can be classified as high-risk MM by any of the following cytogenetic abnormalities detected by fluorescence in situ hybridization (FISH): t(4;14), 17p−, t(14;16), 1+q, or 1p− (5,7).
Genetic alterations and tumor-microenvironment interaction play crucial roles in the occurrence and development of MM (3,8,9). The occurrence and development of MM is accompanied by a variety of specific changes in the number or structure of related genes at the cytogenetic level. In addition, accumulating studies have revealed that disordered epigenetic gene mutations play an important role in the pathogenesis of MM (6,10,11). Genetic testing techniques suggest that high-risk MM patients often have more severe clinical symptoms. The study of differentially expressed genes (DEGs) in high-risk MM patients and standard-risk MM patients has deepened our understanding of cytogenetic abnormalities in MM, and helped to identify important biomarkers and rapidly explore effective molecular targets (6,7). For all the prognostic gene signatures having been developed to predict the clinical outcome in patients with MM, however, serious concerns regarding these signatures have diminished their utility in clinical practice. The most striking deficiency of the previous signatures is non-reproducibility in external datasets. In light of the limitations of the current staging system, it is necessary to identify novel biomarkers and establish a prognostic model based on cytogenetic characterization to distinguish good prognosis from poor prognosis patients, thereby improving patients’ final prognosis.
In this study, we downloaded the microarray data of high-risk MM and standard-risk MM from the Gene Expression Omnibus (GEO) database, and obtained the DEGs with significant differences in high-risk MM. Moreover, the genes that might be involved in the development of high-risk MM were screened out, which provides a basis for exploring the pathogenesis, diagnosis, and treatment of high-risk MM. We present the following article in accordance with the STROBE reporting checklist (available at https://atm.amegroups.com/article/view/10.21037/atm-22-2656/rc).
Methods
Microarray datasets
The GEO database (https://www.ncbi.nlm.nih.gov/geo/) in NCBI was used to search the gene expression profiles with the filter criteria of ‘Multiple myeloma, Homo’. After screening, the gene chip GSE87900, which was submitted by Sonneveld et al. (5) and based on GPL570 [HG-U133_plus_2], was downloaded from the GEO database. A total of 180 samples were collected from the gene expression profile, including 24 high-risk MM samples and 156 standard-risk MM samples.
Analysis of DEGs
DEGs of high-risk MM were analyzed using online analytical tools GEO2 R (http://www.ncbi.nlm.nih.gov/geo/geo2r/), which is based on GEOquery (12), and limma (13). With the filter criteria of ‘P<0.05 and |logFC| >1’, DEGs in the GSE87900 chip were screened.
Functional enrichment analysis and regulatory pathway analysis of DEGs in MM
Gene Ontology (GO) functional annotation and Kyoto Encyclopedia of Genes and Genomes (KEGG) signaling pathway analysis were performed for DEGs (P<0.05) using SangerBox (http://sangerbox.com/), which is based on the R software package clusterProfiler (14). GO functional annotation included cell composition (CC), biological process (BP), and molecular function (MF).
Heatmap analysis of DEGs in key pathways
The SangerBox online analysis tool (http://sangerbox.com/) was used for heatmap clustering analysis.
Patient information
A total of 40 MM patients diagnosed in the Hematology Department of Taian City Central Hospital from January 2017 to July 2019 were included in this study. All patients had de novo MM, had not received any radiotherapy or chemotherapy before sampling. These MM patients were graded according to the Revised International Staging System (R-ISS) and SMART risk stratification (15), and were divided into groups of 20 high-risk MM and 20 standard-risk MM patients. After marrow puncture, the bone marrow samples were transferred to liquid nitrogen immediately, and stored in a refrigerator at −80 ℃ for subsequent detection and analysis. The included MM patients were followed up for 24 months (median follow-up time was 17 months) by outpatient department visits and telephone to calculate the OS of MM patients. The study was conducted in accordance with the Declaration of Helsinki (as revised in 2013). The study was approved by ethics board of The Second Affiliated Hospital of Tianjin Medical University (No. 2021-06-65). Written informed consent was taken from all the patients.
RNA extraction and RT-qPCR detection
Total RNA was extracted from MM tissues with Trizol reagent (Invitrogen, USA). After extraction, the concentration and purity of total RNA were determined by the NanoDrop Lite spectrophotometer (Thermo Scientific, USA). Then, the RNA was reversely transcribed into cDNA with a special reverse transcription kit (Vazyme, China). A fluorescence quantitative reagent (Vazyme, China) was used for RT-qPCR detection. GAPDH was used as the internal reference gene. The 2−∆∆CT method was used to calculated the relative gene expression levels.
Statistical analysis
The data was presented as mean ± SD. The t-test was used to compare differences between the 2 groups. The gene expression level was taken as the index to be measured, and the R-ISS and SMART scoring systems were used as the gold standard for MM diagnosis. The receiver operating characteristic (ROC) curve for predicting the expression level of genes in high-risk MM was plotted to determine the optimal cut-off value and calculate the sensitivity and specificity. According to the optimal critical value determined by the ROC curve, MM patients were divided into a high gene expression group and low gene expression group. The influence of gene expression level on the OS of MM patients was observed after 2 years of follow-up. The survival curve was calculated in each dataset and survival was compared between the two distinguished groups using Kaplan-Meier analysis and the logrank test at a P value of less than <0.05. All statistical analyses were performed using GraphPad Prism 8 software. When P<0.05, the difference was considered statistically significant.
Results
Screening of DEGs in high-risk MM
According to the screening conditions, there were 611 DEGs between high-risk and standard-risk MM in the GSE87900 chip, including 251 up-regulated genes and 360 down-regulated genes. According to the differences in |logFC|, the top 3 up-regulated genes were cancer/testis antigen 1 (CTAG1), cancer/testis antigen 2 (CTAG2), and fibroblast growth factor receptor 3 (FGFR3), and the top 3 down-regulated genes were G1/S-specific cyclin-D1 (CCND1), CCN family member 2 (CTGF), and cannabinoid receptor 1 (CNR1) (Figure 1).
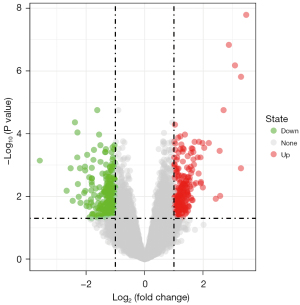
Enrichment analysis of DEGs in high-risk MM
Enrichment analysis showed that the DEGs mainly regulated DNA replication, nuclear division, organelle fission, chromosome segregation, and other processes at the biological level. The changes of DNA replication were the most significant (Figure 2).
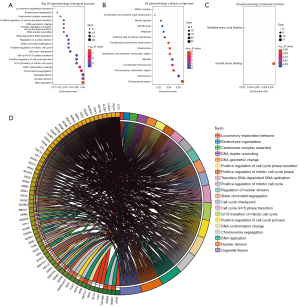
The variation of DEGs during DNA replication in enrichment analysis
In the DNA replication pathway, there were 21 DEGs in the high-risk MM group compared with the standard-risk MM group. According to the differences in multiples |logFC| size sorting, the up-regulated genes were GINS1, RRM2, BRCA1, CDT1, PCNA, GINS3, MCM10, RFC5, CDC7, GINS4, MCM4, BRIP1, CDK1, MCM2, GINS2, DTL, SMC1, BARD1, and MCM6, and the down-regulated genes were NFIB and CDAN1 (Figure 3).
Validation of key predictive genes in the DNA replication pathway
Bone marrow tissues collected from 20 high-risk MM patients and 20 standard-risk MM patients were further analyzed by RT-qPCR (the clinical characteristics of MM patients are listed in supplemental document Table S1). According to the differences in multiples |logFC| size sorting, the levels of the top 10 DEGs were verified by RT-qPCR (the primer sequences are listed in supplemental document Table S2). The results showed that only the relative expression levels of CDC7 and PCNA were significantly different between the high-risk MM group and the standard-risk MM group (Figure 4).
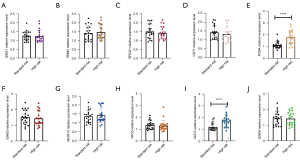
Value analysis of CDC7 and PCNA in high-risk MM
The area under the curve predicted by CDC7 in high-risk MM was 0.825 (P=0.0004), the optimal cut-off value was 1.145, the sensitivity was 95%, and the specificity was 75%. The area under the curve predicted by PCNA in high-risk MM was 0.8488 (P=0.0002), the optimal cut-off value was 1.305, the sensitivity was 85%, and the specificity was 80% (Figure 5).
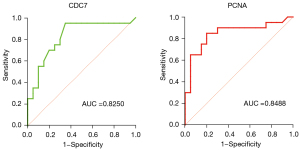
Survival relative to CDC7 and PCNA in MM patients
A total of 40 MM patients were divided into a high gene expression group and low gene expression group by the ROC cut-off value. The Kaplan-Meier survival curve showed that patients with high expression of CDC7 had a lower 2-year OS (P<0.05, Figure 6A). Similarly, patients with high PCNA expression had a lower 2-year OS (P<0.05, Figure 6B).
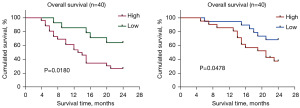
Discussion
In this study, 611 DEGs were screened from the GEO dataset of high-risk MM. Through GO and KEGG pathway enrichment analysis, these differential genes were significantly enriched in DNA replication-related functions and pathways. Moreover, CDC7 and PCNA were screened out by RT-qPCR. These 2 key genes are involved in the regulation of DNA replication and other mechanisms, and may be involved in the regulation of cytogenetic abnormalities in the occurrence and development of MM. ROC analysis and Kaplan-Meier survival curve analysis demonstrated that CDC7 and PCNA have guiding significance in the diagnosis and evaluation of the OS of high-risk MM patients.
The main mechanism of MM involves cyclin D, an important mediator of cell cycle regulation. Cyclin D leads to direct or indirect loss of control, such as malignant behaviors, through non-random primary translocation involving IgH/L sites (16). In this process, the cyclin-dependent kinases family (CDKs) plays an important biological role. Cell division cycle 7 (CDC7) protein is an important S phase kinase, and is a member of the cell division cycle protein family. Overactivation of CDKs during G1 phase can drive cells into S phase and initiate DNA replication from multiple sources throughout chromosomes (17). CDC7 is directly involved in the activation of replicating DNA helicase, the minichromosome maintenance (MCM) complex, and the formation of active replication forks (18). Previous studies have confirmed that inhibition of CDC7 expression can induce reversible cell cycle arrest in normal cells, and induce p53-independent apoptosis in various cancer cells (19,20). Therefore, abnormal CDC7 expression has an important effect on the stability of cell cycle regulation mechanisms. In our study, the expression level of CDC7 was significantly correlated with the disease stage and the clinical prognosis of MM. Patients with high expression of CDC7 often had severe clinical symptoms and poor prognosis.
Proliferating cell nuclear antigen (PCNA) was first discovered and named by Matsumoto et al. in the serum of patients with systemic lupus erythematosus (SLE) in 1987 (21). In the mid-1980s, PCNA was thought to be involved in DNA replication based on its staining patterns throughout the cell cycle (22). PCNA, an important participant in DNA replication and repair, forms a homotrimeric ring that embraces and slides along DNA to anchor DNA polymerase and other DNA editing enzymes (23). Moreover, PCNA interacts with regulatory proteins through PCNA interacting protein box (PIP-box) (24). Previous studies have confirmed that PCNA is closely related to the occurrence, development, staging, and prognosis of tumors. Silencing PCNA can inhibit the proliferation and induce apoptosis of various cancer cells, such as osteosarcoma, cervical cancer, laryngeal cancer, and non-small cell lung cancer cells. Overexpression of PCNA can promote the proliferation of tumor cells and indicates the degree of malignancy of tumors (25). In addition, studies found that the higher the expression level of PCNA, the worse the prognosis of patients. PCNA plays its role by regulating the cell cycle and DNA replication (26-28). Our study revealed that PCNA was highly expressed in high-risk MM patients and was closely associated with poor prognosis in MM patients.
Conclusions
In our study, DEGs between high-risk MM patients and standard-risk MM patients were analyzed by bioinformatics technology. DNA replication was found to play an important role in the occurrence and development of high-risk MM. In addition, 2 key genes related to DNA replication, CDC7 and PCNA, can be used as biomarkers for diagnostic and prognostic assessments of MM patients.
Acknowledgments
Funding: This work was supported by funds from the National Natural Science Foundation of China, the role and molecular mechanism of SETD5 in the homeostasis of hematopoietic stem cell and occurrence and progression of leukemia (No. 82170117).
Footnote
Reporting Checklist: The authors have completed the STROBE reporting checklist. Available at https://atm.amegroups.com/article/view/10.21037/atm-22-2656/rc
Data Sharing Statement: Available at https://atm.amegroups.com/article/view/10.21037/atm-22-2656/dss
Conflicts of Interest: All authors have completed the ICMJE uniform disclosure form (available at https://atm.amegroups.com/article/view/10.21037/atm-22-2656/coif). The authors have no conflicts of interest to declare.
Ethical Statement: The authors are accountable for all aspects of the work in ensuring that questions related to the accuracy or integrity of any part of the work are appropriately investigated and resolved. The study was conducted in accordance with the Declaration of Helsinki (as revised in 2013). The study was approved by ethics board of The Second Affiliated Hospital of Tianjin Medical University (No. 2021-06-65). Written informed consent was taken from all the patients.
Open Access Statement: This is an Open Access article distributed in accordance with the Creative Commons Attribution-NonCommercial-NoDerivs 4.0 International License (CC BY-NC-ND 4.0), which permits the non-commercial replication and distribution of the article with the strict proviso that no changes or edits are made and the original work is properly cited (including links to both the formal publication through the relevant DOI and the license). See: https://creativecommons.org/licenses/by-nc-nd/4.0/.
References
- Joshua DE, Bryant C, Dix C, et al. Biology and therapy of multiple myeloma. Med J Aust 2019;210:375-80. [Crossref] [PubMed]
- Liu W, Liu J, Song Y, et al. Mortality of lymphoma and myeloma in China, 2004-2017: an observational study. J Hematol Oncol 2019;12:22. [Crossref] [PubMed]
- Wang S, Xu L, Feng J, et al. Prevalence and Incidence of Multiple Myeloma in Urban Area in China: A National Population-Based Analysis. Front Oncol 2019;9:1513. [Crossref] [PubMed]
- Joseph NS, Gentili S, Kaufman JL, et al. High-risk Multiple Myeloma: Definition and Management. Clin Lymphoma Myeloma Leuk 2017;17S:S80-7. [Crossref] [PubMed]
- Sonneveld P, Avet-Loiseau H, Lonial S, et al. Treatment of multiple myeloma with high-risk cytogenetics: a consensus of the International Myeloma Working Group. Blood 2016;127:2955-62. [Crossref] [PubMed]
- Rajkumar SV, Dimopoulos MA, Palumbo A, et al. International Myeloma Working Group updated criteria for the diagnosis of multiple myeloma. Lancet Oncol 2014;15:e538-48. [Crossref] [PubMed]
- Fonseca R, Bergsagel PL, Drach J, et al. International Myeloma Working Group molecular classification of multiple myeloma: spotlight review. Leukemia 2009;23:2210-21. [Crossref] [PubMed]
- Wallington-Beddoe CT, Mynott RL. Prognostic and predictive biomarker developments in multiple myeloma. J Hematol Oncol 2021;14:151. [Crossref] [PubMed]
- Rajkumar SV. Multiple myeloma: 2022 update on diagnosis, risk stratification, and management. Am J Hematol 2022;97:1086-107. [Crossref] [PubMed]
- López-Corral L, Gutiérrez NC, Vidriales MB, et al. The progression from MGUS to smoldering myeloma and eventually to multiple myeloma involves a clonal expansion of genetically abnormal plasma cells. Clin Cancer Res 2011;17:1692-700. [Crossref] [PubMed]
- Dimopoulos K, Gimsing P, Grønbæk K. The role of epigenetics in the biology of multiple myeloma. Blood Cancer J 2014;4:e207. [Crossref] [PubMed]
- Davis S, Meltzer PS. GEOquery: a bridge between the Gene Expression Omnibus (GEO) and BioConductor. Bioinformatics 2007;23:1846-7. [Crossref] [PubMed]
- Ritchie ME, Phipson B, Wu D, et al. limma powers differential expression analyses for RNA-sequencing and microarray studies. Nucleic Acids Res 2015;43:e47. [Crossref] [PubMed]
- Yu G, Wang LG, Han Y, et al. clusterProfiler: an R package for comparing biological themes among gene clusters. OMICS 2012;16:284-7. [Crossref] [PubMed]
- Palumbo A, Avet-Loiseau H, Oliva S, et al. Revised International Staging System for Multiple Myeloma: A Report From International Myeloma Working Group. J Clin Oncol 2015;33:2863-9. [Crossref] [PubMed]
- Jiang Y, Zhang C, Lu L, et al. The Prognostic Role of Cyclin D1 in Multiple Myeloma: A Systematic Review and Meta-Analysis. Technol Cancer Res Treat 2022; [Crossref]
- Blow JJ, Gillespie PJ. Replication licensing and cancer--a fatal entanglement? Nat Rev Cancer 2008;8:799-806. [Crossref] [PubMed]
- Sclafani RA, Holzen TM. Cell cycle regulation of DNA replication. Annu Rev Genet 2007;41:237-80. [Crossref] [PubMed]
- Montagnoli A, Tenca P, Sola F, et al. Cdc7 inhibition reveals a p53-dependent replication checkpoint that is defective in cancer cells. Cancer Res 2004;64:7110-6. [Crossref] [PubMed]
- Montagnoli A, Valsasina B, Croci V, et al. A Cdc7 kinase inhibitor restricts initiation of DNA replication and has antitumor activity. Nat Chem Biol 2008;4:357-65. [Crossref] [PubMed]
- Matsumoto K, Moriuchi T, Koji T, et al. Molecular cloning of cDNA coding for rat proliferating cell nuclear antigen (PCNA)/cyclin. EMBO J 1987;6:637-42. [Crossref] [PubMed]
- Madsen P, Celis JE. S-phase patterns of cyclin (PCNA) antigen staining resemble topographical patterns of DNA synthesis. A role for cyclin in DNA replication? FEBS Lett 1985;193:5-11. [Crossref] [PubMed]
- Strzalka W, Ziemienowicz A. Proliferating cell nuclear antigen (PCNA): a key factor in DNA replication and cell cycle regulation. Ann Bot 2011;107:1127-40. [Crossref] [PubMed]
- González-Magaña A, Blanco FJ. Human PCNA Structure, Function and Interactions. Biomolecules 2020;10:570. [Crossref] [PubMed]
- Alexandrakis MG, Passam FH, Pappa CA, et al. Expression of proliferating cell nuclear antigen (PCNA) in multiple myeloma: its relationship to bone marrow microvessel density and other factors of disease activity. Int J Immunopathol Pharmacol 2004;17:49-56. [Crossref] [PubMed]
- Park SH, Kim SJ, Myung K, et al. Characterization of subcellular localization of eukaryotic clamp loader/unloader and its regulatory mechanism. Sci Rep 2021;11:21817. [Crossref] [PubMed]
- Thomas H, Nasim MM, Sarraf CE, et al. Proliferating cell nuclear antigen (PCNA) immunostaining--a prognostic factor in ovarian cancer? Br J Cancer 1995;71:357-62. [Crossref] [PubMed]
- Zhu S, Wang P, Li D. Effect of miR-548c-3p on proliferation and apoptosis of laryngeal carcinoma cells via targeting PCNA gene. Chin Arch Otolaryngol Head Neck Surg 2018;8:705-9.