Construction and validation of a prognostic model based on stage-associated signature genes of head and neck squamous cell carcinoma: a bioinformatics study
Highlight box
Key findings
• In this study, a stage-related gene prognosis prediction model with sound prediction performance for HNSCC was established based on the TCGA and GEO databases.
What is known and what is new?
• Most cases of HNSCC with poor prognosis are locally advanced or have regional lymph node metastasis at the time of diagnosis.
• Therefore, early detection and risk stratification are essential to improve HNSCC survival.
What is the implication, and what should change now?
• Our results may offer insights to find possible biomarkers for early detection and predicting the clinicopathological stage and prognosis for HNSCC.
• Larger sample size, prospective studies or even vivo vitro experiments are needed to confirm these findings.
Introduction
Head and neck squamous cell carcinoma (HNSCC), which includes epithelial tumors of the lip, mouth, oropharynx, hypopharynx, and larynx, is the 6th most prevalent malignancy in the world and causes about 350,000 deaths annually (1,2). Because the onset site of HNSCC is more insidious, the early clinical presentation is atypical and difficult to detect. Most newly diagnosed cases of HNSCC are locally advanced, and most patients have regional lymph node metastasis at the time of diagnosis (2,3). These people have a poor prognosis at the time of diagnosis, with significant variability by site and stage (4). Early detection is essential to improve the prognosis of HNSCC. Imaging parameters have important value for the diagnosis of HNSCC. But because of the radiation exposure and complicated procedures, people with not obvious early symptoms will not choose imaging examination during the physical examination. Moreover, separate imaging variables cannot accurately predict the prognosis of patients with HNSCC. Therefore, more convenient methods which are useful for the early diagnosis and predicting prognosis of HNSCC are needed. Metastasis is likely to involve the selection of genetically heterogeneous lineages of cancer cells in the context of an entire organism (5,6). To metastasis cells, it needs to accumulate the expressions of multiple necessary genes to initiate and promote the primary tumor metastasis cascade (7). The identification of these necessary genes will provide novel insights into the molecular basis of cancer metastasis and inform strategies to improve cancer treatment outcomes in humans. Therefore, finding possible biomarkers for predicting the clinicopathological stage and prognosis of HNSCC is critical.
The prognosis of HNSCC patients depends on the anatomical site, lymph node metastasis, and distant metastasis. As surgical treatment of HNSCC affects a patient’s ability to speak, chew, and swallow, the clinical work of head and neck oncological surgeons is complicated by decisions that must balance aesthetics, quality of life, and prognosis (8). A prognostic model for HNSCC stage-associated outcomes is needed to accurately evaluate a patient’s prognosis and formulate a rational treatment plan.
Immunotherapy prevents the suppression of the immune system blocked by the tumor cells, regulates the immune microenvironment, and controls or removes tumor cells. In recent years, the application of immune checkpoint inhibitors has led to breakthroughs in many tumors. For example, nivolumab and pembrolizumab have been approved to treat advanced HNSCC patients with a programed death-ligand 1 (PD-L1)-stain combined positive score (CPS) >20, where CPS is the ratio of the number of all PD-L1-expressing cells to the number of all tumor cells (9,10). Programed death-1 (PD-1)/PD-L1 inhibitor has improved the prognosis of HNSCC and increased the patient response rate and the overall survival (OS) rate (11). However, the efficiency of immunotherapy in HNSCC needs improvement. It makes sense that the immunotherapeutic population in HNSCC are screened according to the stage-relevant genes.
Previous studies have developed and validated analogous models (12,13). Yet, such useful tools are not currently studied in HNSCC on the section of stage-associated genes. Through a comprehensive bioinformatic analysis, this study explored the genes that may affect HNSCC stage and be associated with immune cell infiltration. Prognostic models were constructed to assist HNSCC patients in the development of diagnosis and treatment strategies. We present the following article in accordance with the TRIPOD reporting checklist (available at https://atm.amegroups.com/article/view/10.21037/atm-22-5427/rc).
Methods
Data acquisition
RNA expression profiles and matched clinical pathological information for HNSCC patients were obtained from The Cancer Genome Atlas (TCGA) database (https://portal.gdc.cancer.gov), which was identified as the training cohort. A total of 536 HNSCC samples (comprising 492 tumors and 44 non-tumors) were obtained. Samples with incomplete and duplicated medical data were removed. Finally, 516 samples were eventually subsumed in our study. Among these, 101 samples were from early stage (stages I–II) patients, and the other 341 were from advanced stage (stages III–IV) patients. The age of the enrolled patients ranged from 19 to 90 years, and the OS time of the patients ranged from 1 to 6,417 days. A validation cohort comprised 97 HNSCC samples was gained from the Gene Expression Omnibus (GEO) (https://www.ncbi.nlm.nih.gov/geo/query/acc.cgi?acc=GSE41613). The study was conducted in accordance with the Declaration of Helsinki (as revised in 2013).
Differences in expression of the stage-associated genes
The HNSCC samples were divided into the following two groups: (I) the stages I–II group; and (II) the stages III–IV group. The differential messenger RNA (mRNA) expressions of these two groups were compared using the limma package of R software. The threshold was set as follows: an adjusted P value <0.05, and a log2(fold change) value >1, or a log2(fold change) value <−1. The differentially expressed genes (DEGs) were then visualized in a heatmap.
Protein-protein interaction (PPI) network
The PPI network for stage-associated genes was calculated using the Metascape online database (https://metascape.org/gp/#/main/step1). The hub genes were obtained using the molecular complex detection (MCODE) algorithm.
Enrichment analysis of the core genes
For the screened stage-associated genes, a Gene Ontology (GO) enrichment analysis of the biological processes, cellular components, and molecular functions, and a Kyoto Encyclopedia of Genes and Genomes (KEGG) pathway analysis were conducted using the R package in R (v.4.2.0) software (https://www.r-project.org/). A P value <0.05 was defined as the cut-off value for the enriched functional categories and pathways.
Correlation analysis of the signature genes and tumor-infiltrating immune cells (TIICs)
Using least absolute shrinkage and selection operator (LASSO) regression methods, we identified 13 stage-associated signature genes. A correlation heat map was generated and a Spearman analysis was conducted to analyze the correlations among the signature genes and TIICs. The horizontal coordinates represented the signature genes, the ordinates represented the immune cells, and the correlation coefficients ranged from −1 to 1. The negative and positive values represented the negative and positive correlations, respectively. A P value <0.05 indicated a statistically significant difference.
Prognostic modeling
The representation data of the signature genes were combined with the survival data to construct a prognostic model, and the accuracy of the model was then tested by a receiver operating characteristic (ROC) curve analysis and a separate date. A risk score was obtained for each sample. Based on the median risk score, the samples were categorized into high- and low-risk patient groups, and the prognosis outcomes between the two groups were compared based on the log-rank test using Kaplan-Meier analysis. ROC curves were drawn and area under the curve (AUC) was calculated to evaluate risk scores’ accuracy in predicting the prognosis of HNSCC. Finally, univariate and multivariate Cox regression analyses were conducted to identify whether the risk score was an independent prognostic factor.
Statistical analysis
All statistical analyses were performed using the R (version 4.2.0) software and related R packages. A P value <0.05 indicated a statistically significant difference.
Results
Figure 1 shows the working flow chart for this study.

Analysis of DEGs for early stage and advanced stage HNSCC
The clinical information of the patients in TCGA cohort is set out in Table S1. We first identified genes in TCGA database that were differentially expressed in early stage and advanced stage HNSCC. Two hundred and eighty DEGs were found, of which 230 were upregulated and 50 were downregulated (Figure 2A). The heatmap showed the top 100 genes of the most differential variations (Figure 2B).
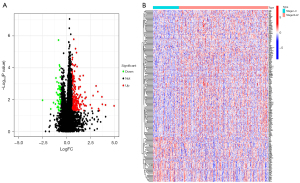
PPI network
In the PPI network that was constructed for the DEGs in the early and advanced stages of HNSCC, 196 were upregulated (red) and 45 were downregulated (green) (Figure 3A). The following 20 network core genes were identified: INA, CPLX2, CXCL10, KCNJ4, GRIK5, LGI3, BRINP1, IL17A, MPPED1, RAB6B, PRR18, APOA1, CRP, SLC22A17, CABP1, ALB, FOXA2, ZCCHC12, SV2A, and ADCY8 (Figure 3B). The expression levels of these 20 genes were statistically significant in both the early and advanced stages of HNSCC. In the advanced stage group, the BRINP1, IL17A, and CXCL10 genes were downregulated, and the INA, CPLX2, KCNJ4, GRIK5, LGI3, MPPED1, RAB6B, PRR18, APOA1, CRP, SLC22A17, CABP1, ALB, FOXA2, ZCCHC12, SV2A, and ADCY8 genes were upregulated (Figure 4).
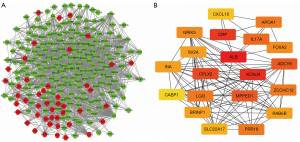
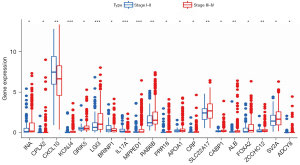
Enrichment analysis results
An enrichment analysis was conducted to investigate the molecular mechanisms of the 20 genes associated with stage in HNSCC. The GO analysis revealed that these genes were important in cellular calcium ion homeostasis, calcium ion homeostasis, and cellular divalent inorganic cation homeostasis (Figure 5A-5I). The KEGG analysis indicated that these 20 genes were important in a number of pathways, such as adenylate cyclase activity and leucine zipper domain binding (Figure 6A,6B).
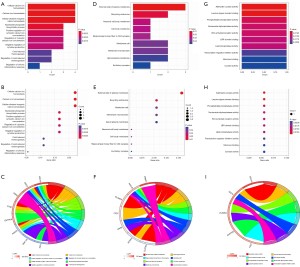
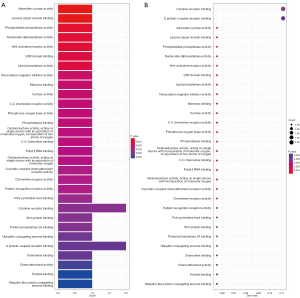
Association of 13 signature genes and immune cell infiltration
The enrichment analysis showed that the 13 genes may affect the pathways of calcium homeostasis, endocytosis, and adenylate cyclase activity. Because their activities are closely related to the immune response (14-16), we investigated the correlation between the 13 genes in the HNSCC samples and TIICs. The ALB gene was positively correlated with mast cells and resting dendritic cells and was negatively correlated with the activation of mast cells and dendritic cells. The FOXA2 and BRINP1 genes were positively correlated with memory CD4 T cells and M0 macrophages, and negatively associated with follicular helper T cells, CD8 T cells, CD4 memory activation T cells, and memory B cells. IL17A and ZCCHC12 expression was positively correlated with regulatory T cells (Tregs) (Figure 7).
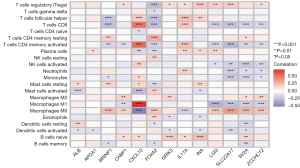
Prognostic model
A LASSO logistic regression analysis was conducted on the gene expression matrices of 13 genes from the HNSCC cohort. The risk scores for the 5 most relevant gene constructs were determined (Figure 8A,8B). The risk score was calculated using the following formula: risk score = (0.193313842865967) × BRINP1 + (−1.00010038505531) × IL17A + (0.175471845859283) × ALB + (0.0945417384137182) × FOXA2 + (0.23890552509693) × ZCCHC12. We performed risk curves and scatter plots to indicate the risk score and survival status of each HNSCC patient. The risk coefficient and the number of dead statuses in the high-risk group were higher than those in the low-risk group (Figure 8C,8D), and the results indicated that mortality was determined by the risk score. The high-risk group showed BRINP1, ALB, FOXA2, and ZCCHC12 expression to be high, while the low-risk group showed IL17A expression to be high (Figure 8E).
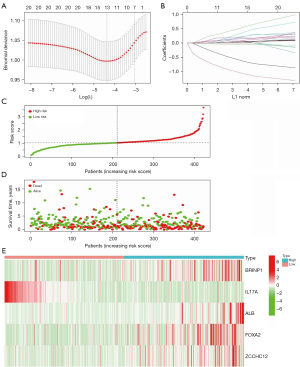
Then log-rank test for the survival outcome differences between the high and low risk groups were performed. The OS of the high-risk group was significantly poorer than that of the low-risk group (P=0.001; Figure 9A). The AUC under the ROC curves in this model at 2, 3, and 6 years were 0.613, 0.629, and 0.638, respectively (Figure 9B). Based on the results of univariate and multivariate Cox regression analyses, the hazard ratio (HR) of the risk score was 2.128 [95% confidence interval (CI), 1.580–2.865] (Figure 9C) and 1.985 (95% CI, 1.462–2.695) (Figure 9D), respectively, (all P<0.001). Univariate Cox analysis in Figure 9C also displayed that the pathological stage and age were risk factors for the prognosis of HNSCC (HR >1; P<0.001). Multivariate Cox analysis in Figure 9D also displayed that the pathological stage and age were independent risk factors for the prognosis of HNSCC (HR >1; P<0.001). These results indicated that the risk score and pathological stage were independent prognostic factors for HNSCC. Moreover, the results showed that the BRINP1, ALB, FOXA2, and ZCCHC12 genes were independent high-risk prognostic factors. IL17A was a favorable prognostic factor according to the multivariate Cox regression analysis based on risk-scoring (Figure 9E).
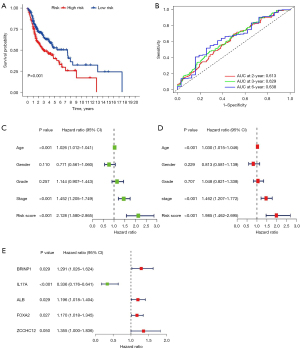
The clinical information of the patients in GEO cohort is set out in Table S1. In the GEO cohort, the different survival outcome between the high-risk group and the low-risk group was consistent with that of the TCGA cohort. The low-risk group showed a better OS (P=0.044; Figure 10A). The AUC in this model at 2, 3, and 6 years were 0.705, 0.698, and 0.669, respectively (Figure 10B). According to the univariate and multivariate analyses, the risk score also served as an independent prognostic factor for HNSCC (HR >1; P<0.005; Figure 10C,10D). This indicated the predictive accuracy of this prognosis prediction models in HNSCC.
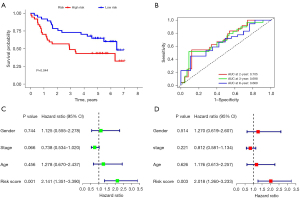
Discussion
Tumor-node-metastasis (TNM) staging is the main clinical staging system for HNSCC. The treatment plans and prognoses of patients are based on TNM staging, as each stage indicates different developing trend of the disease. Early detection of HNSCC has important implications for improving prognosis. But to date, few studies have investigated biomarkers related to HNSCC clinicopathological stage. Our study screened stage-associated genes related to the prognosis of HNSCC and developed prognostic model to provide a basis for early clinical diagnosis and identify new treatment strategies.
In our study, differences in the expression levels of 280 genes were explored using early and advanced stage HNSCC group samples. Among the 280 DEGs, 50 were downregulated, and 230 were upregulated. In addition, a PPI network of these genes was conducted, and 5 signature genes were identified (i.e., BRINP1, IL17A, ALB, FOXA2, and ZCCHC12). BRINP1 suppresses cell-cycle progression during peripheral neuronal differentiation in non-neuronal cells and may have tumor-suppressive effects (17). A previous study found that BRINP1 methylation in gastric cancer is independent of microsatellite instability, which improves the survival outcomes of patients (18). IL17A has an immunomodulatory function when it recruits the adaptor proteins ACT1 and TRAF6 to activate signaling pathways, including mitogen-activated protein kinase and nuclear factor kappa B (NF-κB) (19). Research has shown that IL17A engages in anti-microbial host defense, epithelial cell repair, and regeneration by stimulating the expression of tight junction proteins in colorectal cancer (20). Serum ALB is an indicator of nutritional status, and low levels are associated with poor survival outcomes in various cancers (21,22). FOXA2 has an important regulatory function in tumor development (23-25). Wang et al. revealed that FOXA2, as an oncogene, promotes the proliferation, migration, and invasion of colon cancer (26). In vitro studies have shown that FOXA2 levels in breast cancer are positively correlated with cell proliferation (27). FOXA2 binds to the obtained enhancer to activate liver-specific gene transcription to drive colorectal cancer liver metastasis (28). In addition, FOXA2 activates ZEB2 to promote the invasion and development of esophageal cancer cells (29). The ZCCHC12 gene is involved in lymph node metastasis and participates in the development of thyroid cancer (30). But the underlying biological mechanisms of the BRINP1, IL17A, ALB, FOXA2, and ZCCHC12 genes in HNSCC need further study.
In our study, GO analysis revealed that cellular calcium ion homeostasis were enriched. Studies have shown that calcium ions homeostasis is closely related to intracellular energy metabolism (31,32). Tumor cells are more sensitive to Ca2+ (33). Liu et al. (34) reported a tumor-targeting “calcium ion nanogenerator” to reverse drug resistance by inducing intracellular Ca2+ bursting. But whether the stage-related genes take part in the HNSCC development through the calcium ions homeostasis needs to be further verified by later experiments.
TIICs, which are crucial components of the tumor microenvironment, are intimately linked to the incidence, progression, and metastasis of tumors (35,36). Gao et al. described a positive feedback loop between cancer cells and macrophages that can increase HNSCC cell migration and invasion (37). A systematic review and meta-analysis confirmed the prognostic benefit of CD8+ T cell infiltration in HNSCC patients and showed that FoxP3+ tumor-infiltrating lymphocyte (TIL) contributed to improved OS (38). Immunosuppressive Tregs are present in the immune infiltration of the skin in squamous cell carcinoma, and cause ineffective anti-tumor immune responses, which in turn promote tumor development and metastasis (39). In the present study, immune cell infiltration was closely correlated with the 13 key genes of INA, CXCL10, RIK5, LGI3, BRINP1, IL17A, APOA1, SLC22A17, CABP1, ALB, FOXA2, ZCCHC12, and SV2A. This suggests that the function of these 13 genes in immune infiltration may differ at different stages of HNSCC.
The 5 most relevant genes in this study (i.e., BRINP1, IL17A, ALB, FOXA2, and ZCCHC12) were used to construct the prognostic model. The correlation between these genes and the prognosis of patients with HNSCC also explored. As these genes are closely associated to immune cell infiltration, immunotherapy may prevent the progression of HNSCC.
Additionally, we also studied the association between risk score and clinical factors and the prognosis of patients with HNSCC. Univariate multivariate Cox analysis showed that pathological stage, age, and risk score were risk factors, and pathological stage and risk score were independent risk factors for the prognosis of HNSCC in the TCGA cohort. While in the GEO cohort, risk score was a significantly and independently factor for the prognosis of HNSCC. According to the AUC values in the results of the TCGA set, the AUC values at 2, 3, and 6 years were higher than 0.6, and it was validated in GEO set. So this model is a feasible study due to the sound predictive accuracy. The HRs of the novel risk variables included in our model reflected those reported in a previous study (12).
However, our study had a number of limitations. First, the samples of the database all came from the public data and the size was limited. This may lead to some potential errors or biases in this model. Larger sample size or even prospective studies are needed to confirm these findings. Second, the underlying biological mechanisms of the BRINP1, IL17A, ALB, FOXA2, and ZCCHC12 genes affecting the prognosis of HNSCC require vivo and vitro experiments. We are considering further research on this in the future.
Conclusions
In this study, a prognostic model was constructed and validated based on stage-related genes of HNSCC. ROC curve analysis indicated that the model had favorable performance. The novel stage-associated signature (BRINP1, IL17A, ALB, FOXA2, and ZCCHC12) is a valuable independent prognostic marker and is associated with immune cell infiltration in HNSCC, which may help to develop diagnosis and therapeutic strategies for HNSCC in the future.
Acknowledgments
Funding: This study was supported by funding from the Fujian Provincial Health Technology Project, China (Nos. 2018-1-13 to LC and 2021QNA046 to LC), the Natural Science Foundation of Fujian Province, China (Nos. 2021J0585 to LC and 2022J011054 to LC), the Joint Funds for the Innovation of Science and Technology, Fujian Province, China (Nos. 2020Y9040 to CC and 2021Y9227 to YC), and Fujian Provincial Clinical Research Center for Cancer Radiotherapy and Immunotherapy (No. 2020Y2012 to CC).
Footnote
Reporting Checklist: The authors have completed the TRIPOD reporting checklist. Available at https://atm.amegroups.com/article/view/10.21037/atm-22-5427/rc
Conflicts of Interest: All authors have completed the ICMJE uniform disclosure form (available at https://atm.amegroups.com/article/view/10.21037/atm-22-5427/coif). LC reports funding support from the Fujian Provincial Health Technology Project, China (Nos. 2018-1-13 and 2021QNA046), and the Natural Science Foundation of Fujian Province, China (Nos. 2021J0585 and 2022J011054). YC reports funding support from the Joint Funds for the Innovation of Science and Technology, Fujian Province, China (No. 2021Y9227). CBC reports funding support from the Joint Funds for the Innovation of Science and Technology, Fujian Province, China (No. 2020Y9040), and Fujian Provincial Clinical Research Center for Cancer Radiotherapy and Immunotherapy (No. 2020Y2012). The other authors have no conflicts of interest to declare.
Ethical Statement: The authors are accountable for all aspects of the work in ensuring that questions related to the accuracy or integrity of any part of the work are appropriately investigated and resolved. The study was conducted in accordance with the Declaration of Helsinki (as revised in 2013).
Open Access Statement: This is an Open Access article distributed in accordance with the Creative Commons Attribution-NonCommercial-NoDerivs 4.0 International License (CC BY-NC-ND 4.0), which permits the non-commercial replication and distribution of the article with the strict proviso that no changes or edits are made and the original work is properly cited (including links to both the formal publication through the relevant DOI and the license). See: https://creativecommons.org/licenses/by-nc-nd/4.0/.
References
- Siegel RL, Miller KD, Fuchs HE, et al. Cancer Statistics, 2021. CA Cancer J Clin 2021;71:7-33. [Crossref] [PubMed]
- Johnson DE, Burtness B, Leemans CR, et al. Head and neck squamous cell carcinoma. Nat Rev Dis Primers 2020;6:92. [Crossref] [PubMed]
- Chow LQM. Head and Neck Cancer. N Engl J Med 2020;382:60-72. [Crossref] [PubMed]
- Zolkind P, Lee JJ, Jackson RS, et al. Untreated head and neck cancer: Natural history and associated factors. Head Neck 2021;43:89-97. [Crossref] [PubMed]
- Gupta GP, Massagué J. Cancer metastasis: building a framework. Cell 2006;127:679-95. [Crossref] [PubMed]
- Nguyen DX, Massagué J. Genetic determinants of cancer metastasis. Nat Rev Genet 2007;8:341-52. [Crossref] [PubMed]
- Fidler IJ. The pathogenesis of cancer metastasis: the 'seed and soil' hypothesis revisited. Nat Rev Cancer 2003;3:453-8. [Crossref] [PubMed]
- Lang J, Gao L, Guo Y, et al. Comprehensive treatment of squamous cell cancer of head and neck: Chinese expert consensus 2013. Future Oncol 2014;10:1635-48. [Crossref] [PubMed]
- Saba NF, Blumenschein G Jr, Guigay J, et al. Nivolumab versus investigator's choice in patients with recurrent or metastatic squamous cell carcinoma of the head and neck: Efficacy and safety in CheckMate 141 by age. Oral Oncol 2019;96:7-14. [Crossref] [PubMed]
- Szturz P, Vermorken JB. Translating KEYNOTE-048 into practice recommendations for head and neck cancer. Ann Transl Med 2020;8:975. [Crossref] [PubMed]
- Galvis MM, Borges GA, Oliveira TB, et al. Immunotherapy improves efficacy and safety of patients with HPV positive and negative head and neck cancer: A systematic review and meta-analysis. Crit Rev Oncol Hematol 2020;150:102966. [Crossref] [PubMed]
- Wu L, Zhou Y, Guan Y, et al. Seven Genes Associated With Lymphatic Metastasis in Thyroid Cancer That Is Linked to Tumor Immune Cell Infiltration. Front Oncol 2021;11:756246. [Crossref] [PubMed]
- Zhang Y, Luo X, Yu J, et al. An Immune Feature-Based, Three-Gene Scoring System for Prognostic Prediction of Head-and-Neck Squamous Cell Carcinoma. Front Oncol 2021;11:739182. [Crossref] [PubMed]
- Hawkins TE, Roes J, Rees D, et al. Immunological development and cardiovascular function are normal in annexin VI null mutant mice. Mol Cell Biol 1999;19:8028-32. [Crossref] [PubMed]
- Massullo P, Sumoza-Toledo A, Bhagat H, et al. TRPM channels, calcium and redox sensors during innate immune responses. Semin Cell Dev Biol 2006;17:654-66. [Crossref] [PubMed]
- Salmon D, Vanwalleghem G, Morias Y, et al. Adenylate cyclases of Trypanosoma brucei inhibit the innate immune response of the host. Science 2012;337:463-6. [Crossref] [PubMed]
- Kawano H, Nakatani T, Mori T, et al. Identification and characterization of novel developmentally regulated neural-specific proteins, BRINP family. Brain Res Mol Brain Res 2004;125:60-75. [Crossref] [PubMed]
- Sepulveda JL, Gutierrez-Pajares JL, Luna A, et al. High-definition CpG methylation of novel genes in gastric carcinogenesis identified by next-generation sequencing. Mod Pathol 2016;29:182-93. [Crossref] [PubMed]
- Monin L, Gaffen SL. Interleukin 17 Family Cytokines: Signaling Mechanisms, Biological Activities, and Therapeutic Implications. Cold Spring Harb Perspect Biol 2018;10:a028522. [Crossref] [PubMed]
- Lee JS, Tato CM, Joyce-Shaikh B, et al. Interleukin-23-Independent IL-17 Production Regulates Intestinal Epithelial Permeability. Immunity 2015;43:727-38. [Crossref] [PubMed]
- Lim WS, Roh JL, Kim SB, et al. Pretreatment albumin level predicts survival in head and neck squamous cell carcinoma. Laryngoscope 2017;127:E437-42. [Crossref] [PubMed]
- León X, Pardo L, Sansa A, et al. Prognostic significance of albumin levels prior to treatment in patients with head and neck squamous cell carcinoma. Acta Otorrinolaringol Esp 2020;71:204-11. (Engl Ed). [Crossref] [PubMed]
- Wang DH, Tiwari A, Kim ME, et al. Hedgehog signaling regulates FOXA2 in esophageal embryogenesis and Barrett's metaplasia. J Clin Invest 2014;124:3767-80. [Crossref] [PubMed]
- Yamashita H, Amponsa VO, Warrick JI, et al. On a FOX hunt: functions of FOX transcriptional regulators in bladder cancer. Nat Rev Urol 2017;14:98-106. [Crossref] [PubMed]
- Liu M, Lee DF, Chen CT, et al. IKKα activation of NOTCH links tumorigenesis via FOXA2 suppression. Mol Cell 2012;45:171-84. [Crossref] [PubMed]
- Wang B, Liu G, Ding L, et al. FOXA2 promotes the proliferation, migration and invasion, and epithelial mesenchymal transition in colon cancer. Exp Ther Med 2018;16:133-40. [Crossref] [PubMed]
- Perez-Balaguer A, Ortiz-Martínez F, García-Martínez A, et al. FOXA2 mRNA expression is associated with relapse in patients with Triple-Negative/Basal-like breast carcinoma. Breast Cancer Res Treat 2015;153:465-74. [Crossref] [PubMed]
- Teng S, Li YE, Yang M, et al. Tissue-specific transcription reprogramming promotes liver metastasis of colorectal cancer. Cell Res 2020;30:34-49. [Crossref] [PubMed]
- Gao H, Yan Z, Sun H, et al. FoXA2 promotes esophageal squamous cell carcinoma progression by ZEB2 activation. World J Surg Oncol 2021;19:286. [Crossref] [PubMed]
- Wang O, Zheng Z, Wang Q, et al. ZCCHC12, a novel oncogene in papillary thyroid cancer. J Cancer Res Clin Oncol 2017;143:1679-86. [Crossref] [PubMed]
- Xu L, Tong G, Song Q, et al. Enhanced Intracellular Ca(2+) Nanogenerator for Tumor-Specific Synergistic Therapy via Disruption of Mitochondrial Ca(2+) Homeostasis and Photothermal Therapy. ACS Nano 2018;12:6806-18. [Crossref] [PubMed]
- Kleiner D. The effect of Zn2+ ions on mitochondrial electron transport. Arch Biochem Biophys 1974;165:121-5. [Crossref] [PubMed]
- Pesakhov S, Nachliely M, Barvish Z, et al. Cancer-selective cytotoxic Ca2+ overload in acute myeloid leukemia cells and attenuation of disease progression in mice by synergistically acting polyphenols curcumin and carnosic acid. Oncotarget 2016;7:31847-61. [Crossref] [PubMed]
- Liu J, Zhu C, Xu L, et al. Nanoenabled Intracellular Calcium Bursting for Safe and Efficient Reversal of Drug Resistance in Tumor Cells. Nano Lett 2020;20:8102-11. [Crossref] [PubMed]
- Fridman WH, Galon J, Dieu-Nosjean MC, et al. Immune infiltration in human cancer: prognostic significance and disease control. Curr Top Microbiol Immunol 2011;344:1-24. [Crossref] [PubMed]
- Steven A, Seliger B. The Role of Immune Escape and Immune Cell Infiltration in Breast Cancer. Breast Care (Basel) 2018;13:16-21. [Crossref] [PubMed]
- Gao L, Wang FQ, Li HM, et al. CCL2/EGF positive feedback loop between cancer cells and macrophages promotes cell migration and invasion in head and neck squamous cell carcinoma. Oncotarget 2016;7:87037-51. [Crossref] [PubMed]
- de Ruiter EJ, Ooft ML, Devriese LA, et al. The prognostic role of tumor infiltrating T-lymphocytes in squamous cell carcinoma of the head and neck: A systematic review and meta-analysis. Oncoimmunology 2017;6:e1356148. [Crossref] [PubMed]
- Lai C, August S, Behar R, et al. Characteristics of immunosuppressive regulatory T cells in cutaneous squamous cell carcinomas and role in metastasis. Lancet 2015;385:S59. [Crossref] [PubMed]
(English Language Editor: L. Huleatt)