Interfering with CALCRL expression inhibits glioma proliferation, promotes apoptosis, and predicts prognosis in low-grade gliomas
Highlight box
Key findings
• Compared with normal tissues,
What is known and what is new?
• Glioma is the most common and aggressive malignant tumor in the central nervous system with poor prognosis.
• The effect of
What is the implication, and what should change now?
• It provides a new biomarker and potential therapeutic target for the prognosis and treatment of glioma.
Introduction
Glioma is the most common and aggressive primary malignant tumor of the central nervous system in adults, accounting for nearly 30% of primary brain tumors and 80% of all malignant brain tumors (1,2). According to the World Health Organization (WHO) grading system, grades II and III are classified as low-grade gliomas (LGGs), with grade IV gliomas being considered glioblastomas (GBMs) (3-5). The 5-year survival rate for patients with LGGs ranges from 49% to 93% (6), and the median overall survival (OS) for patients with grade II and III gliomas is 78.1 and 37.6 months, respectively (7). Although LGGs are inert precursors of GBMs and less aggressive, due to the heterogeneity of clinical manifestations, they still lead to a high incidence rate and poor prognosis, posing challenges to treatment (8,9). The tumor boundary of LGG surgery is difficult to define, complete surgical resection is considered impossible, and local recurrence and malignant progression are inevitable despite a combination of adjuvant radiotherapy and chemotherapy treatments (10-12). Therefore, it is necessary to further explore the exact pathogenesis of LGGs and the molecular mechanisms that affect the malignant biological behavior in order to develop new treatments.
The CALCRL gene, located on chromosome 2q32.1, encodes for a 7-transmembrane G protein-coupled receptor that mediates the pleiotropic effects of calcitonin gene-related peptide (CGRP) and adrenomedullin (ADM), 2 structurally related neuropeptides originally described as potent vasodilators (13). CALCRL is involved in a variety of key biological processes, including cell proliferation, apoptosis, angiogenesis, and inflammation (14-16). Antibody-mediated inhibitors of the CALCRL signaling pathway have shown therapeutic activity in malignant solid tumors, including GBM, melanoma, and prostate tumors (17), and it has also emerged as a novel target for the treatment of migraine (18). Low CALCRL expression has been found in lung adenocarcinoma and to act as a prognostic marker and potential tumor suppressor (19), while high CALCRL expression has been associated with glioma (20). However, thus far, no report exists concerning the expression of CALCRL in broad-spectrum tumors, particularly glioma, and its impact on patient survival. Therefore, the role of CALCRL in glioma needs to be further investigated and its prognostic value in LGGs clarified.
The aim of this study was thus to investigate the expression of CALCRL in glioma and its impact on the malignant behavior of glioma. We analyzed the expression of CALCRL in different tumors using The Cancer Genome Atlas (TCGA) database and the Oncomine database, as well as the differential expression of CALCRL in LGGs and GBMs. The expression of CALCRL in glioma clinical specimens was then verified at the tissue level by immunohistochemical staining. Finally, a CALCRL-knockdown cell model was constructed via lentiviral transfection, and the effects of CALCRL on glioma cell proliferation and apoptosis were investigated. Our results provide preliminary insights into the regulation of CALCRL in glioma and provide a reference for the treatment of glioma. We present the following article in accordance with the MDAR reporting checklist (available at https://atm.amegroups.com/article/view/10.21037/atm-22-5154/rc).
Methods
Data acquisition
With the widespread application of microarrays and high-throughput sequencing, a large amount of genomics data has accumulated in the field of cancer research, in databases like TCGA (https://portal.gdc.cancer.gov/) (21). RNA sequencing (RNA-seq) data of glioma were obtained from TCGA database, while RNA-seq data of normal samples were obtained from the Genotype-Tissue Expression (GTEx) database. Patient data with significantly missing information were excluded. This study complies with the publication guidelines developed by TCGA.
Oncomine database analysis
Oncomine (https://www.oncomine.org/resource/login.html) is currently the world’s largest cancer-related gene microarray database and integrated data-mining platform, collecting the most complete spectrum of cancer mutations, associated gene expression profiles, and relevant clinical information (22). In this study, we selected the CALCRL gene as the subject and compared its expression levels in tumor tissues and normal tissues. We set the threshold for gene ranking to “top 10%” and the data type to “all”.
Analysis of the Gene Expression Profiling Interactive Analysis 2 (GEPIA2) database
GEPIA2 (http://gepia.cancer-pku.cn/) is a website for online analysis of cancer expression profiles. The database contains 9,736 tumor samples and 8,587 normal samples from TCGA and GTEx (23). It can provide online analyses of TCGA database. GEPIA2 was used to verify the differential expression of CALCRL in glioma tissue and normal tissue.
Data analysis
Statistical analysis and visualization were performed using the corresponding R package (version 4.0.3). CALCRL in glioma and GTEx did not conform to normal distribution, so the Mann-Whitney test (Wilcoxon rank sum test) was used to compare the expression differences between the tumor group and normal group. The Kruskal-Wallis test is an extension of the Mann-Whitney test and is a type of nonparametric test used for multiple independent samples. The Kruskal-Wallis test was used to compare the differential expression between LGGs, high-grade gliomas, and normal tissue. The diagnostic value of CALCRL for glioma was determined by receiver operating characteristic (ROC) curve. Univariate cox regression analysis was applied, and a forest plot was drawn using the “forestplot” R package to calculate the P value, hazard ratio, and 95% confidence interval (CI). A P value <0.05 was considered statistically significant. The significance levels were as follows: *P<0.05, **P<0.01, and ***P<0.001.
Clinical specimen collection
The human brain tissue specimens used in this experiment were collected from the Neurosurgery Department of the First Affiliated Hospital of Anhui Medical University and included 62 glioma specimens and 10 normal brain tissue specimens. All glioma specimens were primary and were not subject to radiotherapy or chemotherapy before operation. All glioma specimens were resected for the first time, and the diagnosis was confirmed by postoperative pathological testing. Grading the 62 glioma specimens using the WHO’s neuroepithelial tumor criteria (2016 version) yielded the following results: 6 grade I gliomas, 22 grade II, 16 grade III, and 18 grade IV. Furthermore, 10 samples of normal brain tissue were obtained from the brain tissue removed during internal decompression surgery in patients with traumatic brain injury. All specimens were immediately frozen in liquid nitrogen after surgical removal and preserved for use. Informed consent was obtained from the patients for the acquisition and use of all specimens, and the study was approved by the Ethics Committee of the First Affiliated Hospital of Anhui Medical University (No. P2020-12-07). The study was conducted in accordance with the Declaration of Helsinki (as revised in 2013).
Hematoxylin and eosin (HE) staining
Glioma and normal tissues were fixed in 4% paraformaldehyde, dehydrated with alcohol, and embedded in paraffin to prepare tissue wax. The wax blocks were continuously sliced into 5 µm-thick sections, which were then dewaxed in xylene. After being dewaxed, the tissue sections were stained with hematoxylin staining solution, differentiated with 1% hydrochloric acid alcohol for 3 seconds, stained with 1% eosin staining solution for 1 minute, dehydrated, made transparent, sealed, and observed under an optical microscope.
Immunohistochemical staining
After being dewaxed, tissue sections were incubated in 3% hydrogen peroxide solution for 15 minutes to block endogenous peroxidase activity. Sections were fully immersed in antigen repair solution and subjected to heat-mediated antigen repair using an autoclave heated to maximum pressure for 3 minutes, cooled, and rinsed 3 times with phosphate-buffered saline (PBS). Closure solution was added in dropwise fashion. Subsequently, appropriate dilutions of CALCRL (Bioss, Beijing, China) primary antibody were added dropwise to the tissue sections and incubated overnight at 4 ℃. After washing, an appropriate amount of goat anti-rabbit immunoglobin G (IgG) working solution (Bioss) was added dropwise to the sections, DAB (3,3-diaminobenzidine) was developed, and hematoxylin staining was reapplied. The sections were then dehydrated, made transparent, sealed, observed, analyzed, and photographed under a microscope. The staining results were scored simultaneously by 2 experienced pathologists. The staining result score was determined by a combination of staining intensity and percentage of positive cells and calculated according to the following formula: total staining score = intensity score × percentage score.
Cell culture
The human glioma cell lines (SHG44, SU3, SU2, U87, U251, U343, and A172) were obtained from the Department of Neurosurgery, the First Affiliated Hospital of Anhui Medical University. A complete medium was prepared by adding 10% fetal bovine serum, 1% penicillin, and 1% streptomycin in Dulbecco’s Modified Eagle’s medium (DMEM). The cells were cultured in complete medium in a constant temperature incubator at 37 ℃ with a 5% CO2 atmosphere and saturated humidity. Cells were passaged when the cell fusion rate reached 80% and stably passaged to the third or fourth generation for subsequent cell experiments.
Lentiviral transfection
U87 cells were divided into 3 treatment groups: shCtrl (negative control), shCALCRL#1 (knockdown group 1), and shCALCRL#2 (knockdown group 2). The shCALCRL#1 target sequence was CTTATCTCGCTTGGCATATTC, and the shCALCRL#2 target sequence was TTACCTGATGGGCTGTAATTA. U87 cells were inoculated into 6-well plates, and transfection enhancement reagents and an appropriate amount of virus were added to the 6-well plates. After 16 h of transfection, the cells were changed and incubated for 72 h. The expression of the green fluorescent protein (GFP) was observed under a fluorescent microscope to initially test the transfection efficiency. After a 24-h culture, relevant experiments were carried out.
Quantitative real-time polymerase chain reaction (qRT-PCR)
The cells were lysed by TRIzol and total RNA was isolated. The absorbance value of the extracted RNA was measured by NanoDrop2000 (Thermo Fisher Scientific, Waltham, MA, USA). When 1.8 <OD260/OD280 <2.0, the extracted RNA was considered to be of good purity and could be used for subsequent experiments. Reverse transcription primers (2 µL) and total RNA were added to the p PCR tubes for the reaction to obtain complement DNA (cDNA) according to the kit instructions. The process of configuring the reverse transcription system was carried out on ice. Quantitative real-time PCR (qRT-PCR) was performed using the Ultra SYBR mixture, and the relative expression of genes was finally analyzed by the 2−ΔΔCt method, with GAPDH as the internal reference. The primer sequences used during qRT-PCR were the following: CALCRL forward primer 5'-GCAGCAGCTACCTAGCTTGAA-3' and reverse primer 5'-TTCACGCCTTCTTCCGACTC-3', GAPDH-F: 5'GGCCTCCAAGGAGTAAGAAA, GAPDH-R: 5'GCCCCTCCTGTTATTATGG.
Celigo cell count assay
The cells were digested with trypsin at the logarithmic growth stage and prepared as a single cell suspension. The cell suspension was then homogenously inoculated into 96-well plates containing 100 µL of complete medium at approximately 1.5×103 cells per well, which was followed by incubation at 37 ℃ in a 5% CO2 atmosphere. Cells were examined once daily for 5 days starting the day after plate laying using a Celigo full-field cell scanning analyzer (Nexcelom, Germany). The number of cells in each well was accurately recorded and photographed, and the proliferation curve was finally plotted.
MTT experiments
Single cell suspensions were prepared from cells in the logarithmic growth phase and inoculated uniformly into 96-well plates. Each well contained 100 µL of complete medium and approximately 2×103 cells. After 24 hours, 20 µL of MTT solution at a concentration of 5 mg/mL was added to each well and incubated for 4 h. All liquid in the wells was then aspirated, and 100 µL of methanogenic pellets dissolved in dimethyl sulfoxide (DMSO) was added to each well. The 96-well plate was placed on a shaker and shaken well for 2 to 5 min to completely dissolve the crystals. The 96-well plate was then placed in an enzyme marker, and the absorbance values were measured at optical density (OD) 490 nm. The subsequent data were exported and recorded in detail. The same method was used for 5 consecutive days, with the data being statistically analyzed at the end.
Plate clone formation experiments
Single cell suspensions were prepared from cells in the logarithmic growth phase, and 1,000 cells were counted and inoculated in 6-well plates and incubated for 10 min with slow shaking being used to evenly disperse the cells. The cells were incubated at 37 ℃ in a 5% CO2 incubator for 12 days. The cell culture medium was carefully discarded, and the cell clones were fixed using methanol and then stained with crystal violet for 5 min. A cell count of >50 cells was set as 1 valid cell clone. The number of cell clones formed in each well was counted under a microscope, and the subsequent data were statistically analyzed.
Detection of apoptosis by flow cytometry
Single cell suspensions were prepared by taking 1×106 cells at the logarithmic growth stage. Cells were washed twice with PBS and 1 × binding buffer. The cells were then resuspended with 500 µL of 1 × binding buffer and stained for 10 to 15 min at room temperature using the Annexin V staining kit (eBioscience, USA). After this, 5 µL of propidium iodide (PI) was then added to stain the cells. The entire liquid containing the cells was transferred into a flow tube and tested on the flow cytometer (Millipore, France).
Statistical analysis
Data analyses were performed by GraphPad Prism version 8.0.2. All enumeration data were shown as mean ± SD and were analyzed by Student’s t-test. P<0.05 was considered to be statistically significant.
Results
CALCRL was highly expressed in glioma
In order to explore the role of CALCRL in tumor progression, we first analyzed the transcript levels of CALCRL in various tumor tissue types through the Oncomine database. The results showed that the CALCRL gene was generally highly expressed in all tumor types except kidney cancer, lung cancer, and sarcoma tissue (Figure 1A). RNA-seq data from all tumor samples in TCGA database and normal tissue samples in the GTEx database were analyzed, and the transcription levels of CALCRL in various tumors were compared. We found that CALCRL was significantly highly expressed in GBM and LGG (Figure 1B). The Wilcoxon rank sum test was used to calculate the differential expression between glioma and normal tissues. The results showed that there was a significant difference in the expression of CALCRL between glioma and normal tissues (Figure 1C). Analysis of the GEPIA2 database showed that CALCRL expression was significantly upregulated in LGG and GBM compared to normal tissue (Figure 1D,1E).
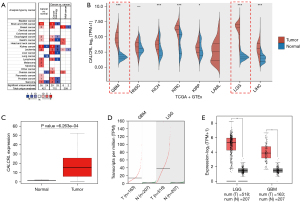
The relationship between CALCRL expression and clinical features of glioma and prediction of prognosis in LGG
We used the R package, “ggplot2”, to statistically analyze the data from TCGA and GTEx. A P value <0.05 was considered statistically significant, and the results indicated that CALCRL expression was higher in LGG compared to GBM (Figure 2A). We determined whether there were significant differences in the expression of CALCRL in different clinical features between WHO grade II and III glioma. The results showed that CALCRL expression remained significantly different in LGG, with higher expression in WHO grade II gliomas compared to grade III gliomas, and decreased expression with increasing glioma grade (Figure 2B). The isocitrate dehydrogenase (IDH) in WHO grade II and III glioma was divided into a wild type (WT) and mutant type (MT). The difference in CALCRL expression between the 2 groups was statistically significant (Figure 2C). The time-dependent ROC curve showed that the area under the curve (AUC) of CALCRL for predicting survival outcome in patients with LGG was 0.979, indicating that CALCRL can be a good predictor of patient survival (Figure 2D). To further explore whether CALCRL is an important prognostic factor for LGG, we performed univariate Cox regression analysis on RNA-seq data from TCGA, and the forest plot showed that CALCRL expression was significantly associated with the prognosis of LGG (Figure 2E).
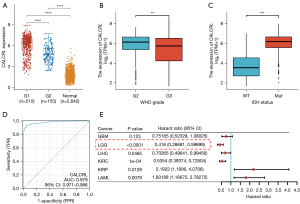
CALCRL expression levels correlated with glioma pathological grade
To clarify the expression of CALCRL in clinical glioma surgical specimens and normal brain tissue, we performed HE staining and immunohistochemical staining on 62 glioma surgical specimens and 10 normal brain tissue specimens. We found that CALCRL expression was low in normal brain tissue; however, in glioma tissue, especially in WHO grade III glioma, CALCRL expression was significantly upregulated and higher compared to that in GBM (Figure 3).
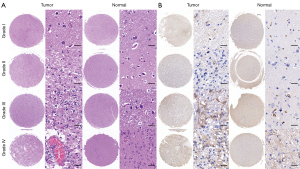
CALCRL expression in glioma cell lines
To assess CALCRL expression in different glioma cell lines, we measured CALCRL messenger RNA (mRNA) levels in 7 glioma cell lines (SHG44, SU3, SU2, U87, U251, U343, and A172) and 2 normal astrocyte lines (HEB and HA1800) by qRT-PCR. The results showed a significantly different expression of CALCRL in the glioma cell lines compared to normal astrocytes (Figure 4A). U87, as the cell line with the highest CALCRL expression, was selected for subsequent CALCRL knockdown experiments. Short hairpin RNA (shRNA) was transfected in U87 cells to interfere with CALCRL expression, and stably transfected cell lines were constructed, with transfection efficiency being verified by qRT-PCR. The results showed that the expression level of CALCRL was reduced after transfection with shRNA, and the difference between the knockdown and control groups was statistically significant (Figure 4B). Specifically, the knockdown efficiency of shCALCRL#1 group was greater than shCALCRL#2, which was thus used in the follow-up experiment, and assign as shCALCRL. The cell morphology and vector fluorescence expression before and after shRNA transfection of U87 cells were observed under fluorescence microscope, and green fluorescence could be observed, indicating successful shRNA transfection (Figure 4C).
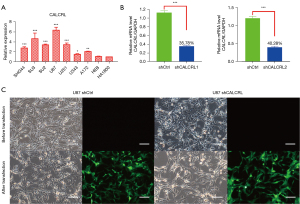
The effect of interference with CALCRL expression on the proliferation of glioma cells
The number of cells was dynamically detected by Celigo imaging for 5 consecutive days. We found that the number of U87 cells in the shCALCRL group was significantly lower than that in the shCtrl group. Further calculation of the cell proliferation rate also revealed that the cell proliferation rate in the shCALCRL group was significantly lower than that in the shCtrl group (Figure 5A,5B). Through cell viability analysis, we found that the number of viable U87 cells in the shCALCRL group was significantly lower than that in the shCtrl group, and further calculation of the cell proliferation rate showed that the cell proliferation rate in the shCALCRL group was significantly lower than that in the shCtrl group (Figure 5C). This finding, in combination with the results of the Celigo assay and MTT assay, indicated that the knockdown of CALCRL gene could inhibit the proliferation of glioma cells.
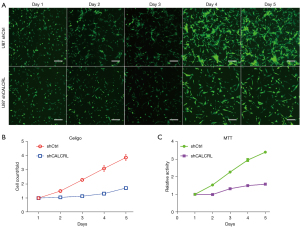
Effect of interference with CALCRL expression on clone formation in glioma cells
The results of the clone formation assay showed that after interference with CALCRL expression in U87 cells, the clone formation rate of cells in the shCALCRL group was significantly decreased compared with that in the shCtrl group, and the difference was statistically significant (Figure 6A-6C). The results indicated that interfering with CALCRL expression could inhibit the clone formation of glioma cells.
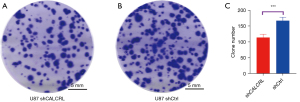
Effect of interference with CALCRL expression on apoptosis in glioma cells
Apoptosis resistance is a major feature of tumor, and promoting apoptosis in tumor cells has become one of the important strategies for antitumor therapy. By using Annexin V staining to assess the relationship between CALCRL gene downregulation and apoptosis in U87 cells.
The results showed that CALCRL knockdown significantly promoted apoptosis in U87 cells (Figure 7A-7C). Thus, this result suggests that interference with CALCRL expression induces apoptosis in glioma cells.
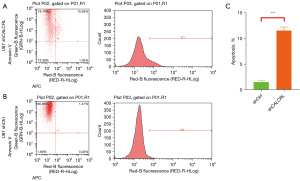
Discussion
Glioma is a highly aggressive malignant brain tumor with the ability to proliferate rapidly, but treating this disease is challenging due the inability to form accurate prognoses and the limited treatment options (24,25). The pathogenesis of glioma is complex due to the expression of aberrant genes that affect cell growth, aggressiveness, and angiogenesis (26). In general, lower-grade tumors grow more slowly and have a more favorable prognosis than do higher-grade tumors. However, pathological grading is not sufficient to predict the prognosis of patients with glioma (27). Therefore, there is a need to find more promising prognostic biomarkers and new therapeutic targets.
CALRL is a G protein-coupled neuropeptide receptor involved in the regulation of calcium ion concentration in cells. CALCRL is upregulated in certain tumor types such as acute myeloid leukemia (AML), Kaposi sarcoma, and Ewing sarcoma (28). High expression of CALCRL has been shown to be associated with poor prognosis in AML. Angenendt et al. found that knockdown of CALCRL significantly inhibited colony formation in human myeloid leukemia cell lines, suggesting that CALCRL may be a potential therapeutic target in AML (13). In addition, Larrue et al. found that CALCRL depletion reduced the frequency of leukemic stem cells in relapse-initiating cells after in vivo chemotherapy (29). CALCRL plays a role in tumor progression by mediating CGRP and ADM. It has been demonstrated that CGRP and ADM exert a crucial action in enhancement of angiogenesis and promotion of tumor cell growth. Among them, autocrine or paracrine CALCRL signaling loops exert this effect (30,31). These results all suggest that CALCRL is closely associated with cancer. However, the exact role of CALCRL in glioma, and more generally in other cancers, remains to be determined.
In the present study, we evaluated the role of the rarely reported on CALCRL gene in glioma. Using TCGA and GTEx databases, we found that CALCRL is overexpressed in glioma, especially in LGG, plays a role in its diagnosis, and is significantly associated with LGG prognosis. Pathological immunohistochemical staining of clinical glioma specimens indicated that CALCRL was overexpressed in LGG compared with GBM. Next, we explored the relationship between CALCRL expression levels and glioma biological properties using the U87 cell line. We knocked down the expression level of CALCRL in the U87 cell line using shRNA transfection and verified the transfection efficiency using qRT-PCR. The results of in vitro experiments showed that knockdown of CALCRL significantly inhibited the proliferation and clone formation of glioma cells while promoting the occurrence of apoptosis. In conclusion, CALCRL expression is associated with malignant biological processes and can be used as a biomarker for glioma. The expression of CALCRL in glioma may be the key to the diagnosis and treatment of glioma.
Conclusions
CALCRL was significantly more highly expressed in LGG compared to normal tissue, and differential expression correlated significantly with LGG prognosis. Glioma cell proliferation was significantly slowed, cloning was reduced, and apoptosis was increased after interference with CALCRL expression. Thus, interference with CALCRL expression significantly inhibited glioma proliferation, promoted apoptosis, and predicted the prognosis of LGG and thus may serve as a potential therapeutic target for glioma.
Acknowledgments
Funding: This study was supported by the Anhui Provincial Natural Science Foundation (No. 2208085MH251), the Anhui Medical University Scientific Research Fund (No. 2021xkj131), and the Basic and Clinical Cooperative Research and Promotion Program of Anhui Medical University (No. 2022xkjT024).
Footnote
Reporting Checklist: The authors have completed the MDAR reporting checklist. Available at https://atm.amegroups.com/article/view/10.21037/atm-22-5154/rc
Data Sharing Statement: Available at https://atm.amegroups.com/article/view/10.21037/atm-22-5154/dss
Conflicts of Interest: All authors have completed the ICMJE uniform disclosure form (available at https://atm.amegroups.com/article/view/10.21037/atm-22-5154/coif). The authors have no conflicts of interest to declare.
Ethical Statement: The authors are accountable for all aspects of the work in ensuring that questions related to the accuracy or integrity of any part of the work are appropriately investigated and resolved. The study was conducted in accordance with the Declaration of Helsinki (as revised in 2013). Informed consent was obtained from the patients for the acquisition and use of all specimens, and the study was approved by the Ethics Committee of the First Affiliated Hospital of Anhui Medical University (No. P2020-12-07).
Open Access Statement: This is an Open Access article distributed in accordance with the Creative Commons Attribution-NonCommercial-NoDerivs 4.0 International License (CC BY-NC-ND 4.0), which permits the non-commercial replication and distribution of the article with the strict proviso that no changes or edits are made and the original work is properly cited (including links to both the formal publication through the relevant DOI and the license). See: https://creativecommons.org/licenses/by-nc-nd/4.0/.
References
- Rong L, Li N, Zhang Z. Emerging therapies for glioblastoma: current state and future directions. J Exp Clin Cancer Res 2022;41:142. [Crossref] [PubMed]
- Chen Z, Ye N, Teng C, et al. Alternations and Applications of the Structural and Functional Connectome in Gliomas: A Mini-Review. Front Neurosci 2022;16:856808. [Crossref] [PubMed]
- Louis DN, Perry A, Wesseling P, et al. The 2021 WHO Classification of Tumors of the Central Nervous System: a summary. Neuro Oncol 2021;23:1231-51. [Crossref] [PubMed]
- Ostrom QT, Cioffi G, Waite K, et al. CBTRUS Statistical Report: Primary Brain and Other Central Nervous System Tumors Diagnosed in the United States in 2014-2018. Neuro Oncol 2021;23:iii1-iii105. [Crossref] [PubMed]
- Suzuki H, Aoki K, Chiba K, et al. Mutational landscape and clonal architecture in grade II and III gliomas. Nat Genet 2015;47:458-68. [Crossref] [PubMed]
- Liu Y, Liu S, Li G, et al. Association of high-dose radiotherapy with improved survival in patients with newly diagnosed low-grade gliomas. Cancer 2022;128:1085-92. [Crossref] [PubMed]
- Li J, Wang J, Ding Y, et al. Prognostic biomarker SGSM1 and its correlation with immune infiltration in gliomas. BMC Cancer 2022;22:466. [Crossref] [PubMed]
- Chen J, Wang Z, Wang W, et al. SYT16 is a prognostic biomarker and correlated with immune infiltrates in glioma: A study based on TCGA data. Int Immunopharmacol 2020;84:106490. [Crossref] [PubMed]
- Guo Y, Li Y, Li J, et al. DNA Methylation-Driven Genes for Developing Survival Nomogram for Low-Grade Glioma. Front Oncol 2021;11:629521. [Crossref] [PubMed]
- Chen J, Shen S, Li Y, et al. APOLLO: An accurate and independently validated prediction model of lower-grade gliomas overall survival and a comparative study of model performance. EBioMedicine 2022;79:104007. [Crossref] [PubMed]
- Shankar GM, Kirtane AR, Miller JJ, et al. Genotype-targeted local therapy of glioma. Proc Natl Acad Sci U S A 2018;115:E8388-94. [Crossref] [PubMed]
- Wang Z, Cheng W, Zhao Z, et al. Comparative profiling of immune genes improves the prognoses of lower grade gliomas. Cancer Biol Med 2021;19:533-50. [Crossref] [PubMed]
- Angenendt L, Bormann E, Pabst C, et al. The neuropeptide receptor calcitonin receptor-like (CALCRL) is a potential therapeutic target in acute myeloid leukemia. Leukemia 2019;33:2830-41. [Crossref] [PubMed]
- Larráyoz IM, Martínez-Herrero S, García-Sanmartín J, et al. Adrenomedullin and tumour microenvironment. J Transl Med 2014;12:339. [Crossref] [PubMed]
- Russell FA, King R, Smillie SJ, et al. Calcitonin gene-related peptide: physiology and pathophysiology. Physiol Rev 2014;94:1099-142. [Crossref] [PubMed]
- Davis RB, Kechele DO, Blakeney ES, et al. Lymphatic deletion of calcitonin receptor-like receptor exacerbates intestinal inflammation. JCI Insight 2017;2:e92465. [Crossref] [PubMed]
- Angenendt L, Wöste M, Mikesch JH, et al. Calcitonin receptor-like (CALCRL) is a marker of stemness and an independent predictor of outcome in pediatric AML. Blood Adv 2021;5:4413-21. [Crossref] [PubMed]
- Dodick DW. Migraine. Lancet 2018;391:1315-30. [Crossref] [PubMed]
- Lu M, Fan X, Liao W, et al. Identification of significant genes as prognostic markers and potential tumor suppressors in lung adenocarcinoma via bioinformatical analysis. BMC Cancer 2021;21:616. [Crossref] [PubMed]
- Han T, Zuo Z, Qu M, et al. Comprehensive Analysis of Inflammatory Response-Related Genes, and Prognosis and Immune Infiltration in Patients With Low-Grade Glioma. Front Pharmacol 2021;12:748993. [Crossref] [PubMed]
- Zhang L, Peng R, Sun Y, et al. Identification of key genes in non-small cell lung cancer by bioinformatics analysis. PeerJ 2019;7:e8215. [Crossref] [PubMed]
- Xie XW, Jiang SS, Li X. CLEC3B as a Potential Prognostic Biomarker in Hepatocellular Carcinoma. Front Mol Biosci 2020;7:614034. [Crossref] [PubMed]
- Tang Z, Kang B, Li C, et al. GEPIA2: an enhanced web server for large-scale expression profiling and interactive analysis. Nucleic Acids Res 2019;47:W556-60. [Crossref] [PubMed]
- Wu J, Tang X, Yu X, et al. TMEM60 Promotes the Proliferation and Migration and Inhibits the Apoptosis of Glioma through Modulating AKT Signaling. J Oncol 2022;2022:9913700. [Crossref] [PubMed]
- Zheng X, Wang Y, Wang D, et al. PSMC2 is overexpressed in glioma and promotes proliferation and anti-apoptosis of glioma cells. World J Surg Oncol 2022;20:84. [Crossref] [PubMed]
- Zhang F, Cai HB, Liu HZ, et al. High Expression of CISD2 in Relation to Adverse Outcome and Abnormal Immune Cell Infiltration in Glioma. Dis Markers 2022;2022:8133505. [Crossref] [PubMed]
- Li F, Zhang Y, Wang N, et al. Evaluation of the Prognosis of Neuroglioma Based on Dynamic Magnetic Resonance Enhancement. World Neurosurg 2020;138:663-71. [Crossref] [PubMed]
- Xing C, Yin H, Yao ZY, et al. Prognostic Signatures Based on Ferroptosis- and Immune-Related Genes for Cervical Squamous Cell Carcinoma and Endocervical Adenocarcinoma. Front Oncol 2021;11:774558. [Crossref] [PubMed]
- Larrue C, Guiraud N, Mouchel PL, et al. Adrenomedullin-CALCRL axis controls relapse-initiating drug tolerant acute myeloid leukemia cells. Nat Commun 2021;12:422. [Crossref] [PubMed]
- Toda M, Suzuki T, Hosono K, et al. Neuronal system-dependent facilitation of tumor angiogenesis and tumor growth by calcitonin gene-related peptide. Proc Natl Acad Sci U S A 2008;105:13550-5. [Crossref] [PubMed]
- Chen P, Huang Y, Bong R, et al. Tumor-associated macrophages promote angiogenesis and melanoma growth via adrenomedullin in a paracrine and autocrine manner. Clin Cancer Res 2011;17:7230-9. [Crossref] [PubMed]
(English Language Editor: J. Gray)