Effect of Bining decoction on gouty nephropathy: a network pharmacology analysis and preliminary validation of gut microbiota in a mouse model
Highlight box
Key findings
• Combining
What is known and what is new?
• Traditional Chinese Medicine (TCM) formulation formulations can influence disease by mediating the homeostasis of intestinal flora.
• After Intervention of Bining Decoction, BN could increase the richness and diversity of the intestinal flora. BN did play a certain role in gouty nephropathy (GN) structure.
What is the implication, and what should change now?
• After more in-depth research, BN could be recommended for GN treatment in the future.
Introduction
Gouty nephropathy (GN) is a kind of kidney disorder that results from an elevation in uric acid (UA) level in the serum which has long been accumulated in the renal tubules and interstitium (1), causing a reaction in the surrounding macrophages (2). The representative histological features of UA nephropathy are characterized by urate deposits in the renal tubules and interstitium, which can be observed as birefringent, needle-like urate crystals (1). Disease progression may even lead to renal failure. Most patients experience lumbago, nocturia, or waist soreness, and some patients may develop hematuria, edema, hypertension, and other symptoms such as fever. Currently, GN is regarded as an essential public health issue. A recent study estimated that 24% of gout patients exhibit chronic kidney disease (3). According to the statistics, 12–18% of people in southern China experience UA stones (4). The development of modern society has contributed to GN gradually becoming one of the serious threats to human health.
Colchicine, non-steroidal anti-inflammatory drugs (NSAIDs), and non-gut/oral glucocorticoids are recommended as first-line treatments for gout based on the American College of Rheumatology (ACR) guidelines issued in 2020 (5). Nevertheless, no other treatment options are currently available for some clinical patients. NSAIDs are widely used for the treatment of gout attacks, but are not suitable for elderly patients with renal injury and many comorbidities. Meanwhile, colchicine has been prohibited for use in the treatment of gout attacks in patients with renal damage (6).
Therefore, there is a growing need for safe and effective alternatives to substitute drug therapy, and investigational drugs with a wider application range, better efficacy, and lower toxicity, especially those derived from natural products. Herbal medicine is a valuable resource in the exploration of novel drugs to treat various diseases, including GN. Increasing numbers of traditional Chinese medicines (TCM) have been reported to have a protective effect on the kidneys; therefore, mounting attention has been paid to TCM due to their satisfactory and mild activity in treating nephropathy. A study has shown that TCM formulations can influence disease by mediating the homeostasis of intestinal flora (7).
The mechanism of GN is primarily associated with hyperuricemia (HUA) and the deposition of monosodium urate crystals in different parts of the body. Excessive UA deposits place a burden on the kidneys, eventually causing lesions. In the human body, approximately 80% of the total amount of UA is decomposed from nucleic acids, and the rest is generated by purine-rich foods. The human body produces and excretes 600–700 mg of UA per day, 70% of which is excreted by the kidneys, and the remaining 30% is eliminated through the intestines (8). An elevated UA level in blood circulation affects the intestinal environment, resulting in alternations of the gut microbiota. Additional UA will be eliminated by the intestine as compensation for the impairment of the kidney (9). Therefore, the gut microbiota has become a novel target to elucidate the GN pathogenesis and for the further treatment of GN.
Evidence has shown that over 1,000 genera of bacteria can colonize the human gut and form the gut microbiota (10). These bacteria are involved in host metabolism, immunoregulation, as well as maintenance of internal environment homeostasis (11). High-throughput sequencing has achieved tremendous advances in our understanding of the gut microbiome. As previously described, gut microbiota can affect UA levels. Current research has shown that the intestinal flora reduces UA levels due to its participation in purine metabolism and decomposition of UA. For example, a report suggested that the gut microbiota can secrete the oxidative metabolism-associated enzyme xanthine oxidase (12).
Intestinal flora-generated metabolites promote UA excretion by providing energy for intestinal epithelial cells (11). Meanwhile, the gut microbiota also produces short-chain fatty acids (SCFA) by decomposing indigestible carbohydrates ingested by humans (13), which play a part in maintaining homeostasis of the human gastrointestinal tract.
Bining decoction (BN) is a TCM formulation that is derived from the Er Miao San prescription, the action of which is described as dissipating heat and dampness, as well eliminating edema in the State Pharmacopoeia of People’s Republic of China, Danxi’s Experiences in Medicine, and other TCM literature. Clinically, BN is used in the treatment of GN; it provides a breakthrough in the prevention and treatment of GN and improves the quality of life of patients. The ingredients of BN include Dioscoreae Hypoglaucae Rhizoma, Plantaginis Semen, Atractylodes Lancea (Thunb.) DC., Coicis Semen, Pseudobulbus Cremastrae Seu Pleiones, Phellodendri Chinensis Cortex, Chuanxiong Rhizoma, Cyathulae Radix, and Lonicerae Japonicae Caulisand. Atractylodes Lancea (Thunb.) DC. is the principal drug in the decoction, and various active ingredients have been found to act on tumor necrosis factor-α (TNF-α), interleukin-6 (IL-6), IL-1β, transcription factor p65 (RELA), prostaglandin-endoperoxide synthase 2 (PTGS2), as well as recombinant mitogen-activated protein kinase 14 (MAPK14), which has a vital effect on modulating inflammatory cytokines and apoptosis-associated pathways for the improvement of the internal imbalance associated with the disease (14). Meanwhile, it also has the function of removing turbidity and could remove the dampness and heat from urine, supporting the modern pharmacological discovery of reducing UA levels, and promoting UA excretion. As an adjuvant drug, Phellodendri Chinensis Cortex helps the principal drug to dispel dampness and heat, but also works to resolve phlegm, indicating its protective effect on the kidneys. Chuanxiong Rhizoma is considered an adjuvant to promote blood circulation and remove dampness; Cyathulae Radix is regarded as an agent to catalyze the efficacy of the drug. The above drugs are used together to remove pathogenic factors, balance the healthy qi, and simultaneously treat the symptoms and the root causes, thereby jointly removing turbidity and protecting the kidneys. This also demonstrates the treatment based on syndrome differentiation of BN, reflecting the advancement of ancient TCM theory. Nevertheless, how BN mediates GN has not been elucidated thoroughly.
Network pharmacology is a conduit for explaining the complex interactions among biological systems, disease, and drugs. It can identify synergistic functions in disease treatment by analyzing huge datasets and explicating the possible complex bioactivity processes, which may accelerate clinical translation (15). The primary outcome of this study is the mechanism of therapeutic effects of BN on GN which were collected by integrating network pharmacology. The secondary outcomes constitute the treatment effects of BN against GN in mice, which were evaluated by performing biochemical tests [UA, blood urea nitrogen, and creatinine (BUN and Cr)] and evaluating the renal weight index. The last outcome of this study is the signaling pathway prediction of BN in GN by using 16S rRNA sequencing. We present the following article in accordance with the ARRIVE reporting checklist (available a https://atm.amegroups.com/article/view/10.21037/atm-22-5523/rc).
Methods
Collection of BN and GN targets
The Traditional Chinese Medicine Systems Pharmacology Database and Analysis Platform (TCMSP; https://old.tcmsp-e.com) was used to seek the useful active ingredients of BN (16). The keywords were as follows: “Dioscoreae Hypoglaucae Rhizoma”, “Plantaginis Semen”, “Atractylodes Lancea (Thunb.) DC.”, “Coicis Semen”, “Pseudobulbus Cremastrae Seu Pleiones”, “Phellodendri Chinensis Cortex”, “Chuanxiong Rhizoma”, “Cyathulae Radix”, and “Lonicerae Japonicae Caulisand”. The screening conditions were “oral bioavailability (OB) ≥30%, and drug-like (DL) index ≥0.18”. OB refers to the speed and extent at which the drug enters the systemic circulation after oral absorption (17). DL is applied to reflect the similarities of specific groups in compounds with known drug degrees. OB and DL play essential roles in the exploration of the TCM active ingredient. The human genes related to GN were screened from the databases of GeneCards (www.genecards.org/), Online Mendelian Inheritance in Man (www.omim.org/), Genbank (http://www.ncbi.nlm.nih.gov), and DisGeNET (https://www.disgenet.org/home/) using the keywords of “gouty nephropathy”, “uric acid nephropathy”, and “gouty kidney" to search for disease targets. The study was conducted in accordance with the Declaration of Helsinki (as revised in 2013). The UniProt database (www.uniprot.org/) was implemented to convert all the aforesaid target proteins into standardized gene names.
Protein-protein interaction (PPI) network construction
The targets of BN for the GN therapy, identified by mapping the active compound targets to the GN-associated targets, were input into the database of the Search Tool for the Retrieval of Interacting Genes/Proteins (STRING; https://string-db.org) to build a PPI network, which was subsequently analyzed with the organism species restricted to Homo sapiens with a high confidence score more than 0.9. Subsequently, the obtained PPI data were input into Cytoscape 3.7.2 (https://cytoscape.org/), and the top 10 genes were selected by cytoHubba plugin in the Cytoscape (18).
Analysis of signaling pathway enrichment using the Kyoto Encyclopedia of Genes and Genomes (KEGG) database
Next, a signaling pathway enrichment analysis was conducted with the Metascape database (https://metascape.org/gp/index.html) via the KEGG database. Significantly, species was restricted to Homo sapiens. The diagrams for the signaling pathway enrichment were plotted by R 3.5.2. (The R Project for Statistical Computing, Vienna, Austria).
Animals
In the study, n refers to number of animals and the individual mouse was considered the experimental unit within the studies. The sample size calculation was based on a similar experiment reported in the literature and a preliminary experiment conducted under identical conditions to the planned experiment (19). A total of 30 male Institute of Cancer Research (ICR) mice (18±2 g), were provided by the Heilongjiang University of Chinese Medicine (Harbin, China). Animals were kept in polyacrylic cages (temperature: 22±2 ℃, humidity: 50%±5%, 12-h light/dark cycle, and sufficient water and food). Animal experiments were performed under the approval by the Animal Ethics Committee of Heilongjiang University of Chinese Medicine (No. 202009670), in compliance with the Heilongjiang University of Chinese Medicine guidelines for the care and use of animals. A protocol was prepared before the study without registration. For each animal, 3 different investigators were involved as follows: a first investigator (HH) was responsible for animal grouping work. This investigator was the only person aware of the group allocation. A second investigator (YY) was responsible for the intragastrical gavage, whereas a third investigator (YG) (also unaware of treatment) was responsible for data collection and assessment. Based on the 3R principle, the experiment was designed to minimize the use of animals, observe the physiological conditions of experimental animals, and standardize the gavage by the same investigator, thus minimizing animal pain and unnecessary death. At the end of the experiment, the mice were sacrificed by decapitation, and then the animal carcasses were sent to the specified places according to the requirements, which were uniformly processed by the institution.
Chemical reagents
Dioscoreae Hypoglaucae Rhizoma, Plantaginis Semen, Atractylodes Lancea (Thunb.) DC., Coicis Semen, Pseudobulbus Cremastrae Seu Pleiones, Phellodendri Chinensis Cortex, Chuanxiong Rhizoma, Cyathulae Radix, and Lonicerae Japonicae Caulisand were purchased from Heilongjiang Deshunchang Decoction Pieces Co., Ltd. (Harbin, China). The GN mouse model was prepared by combining yeast and adenine (Shanghai Aladdin Biochemical Technology Co., Ltd., Shanghai, China). Other reagents were from domestic reagent companies.
Preparation of BN extract
The decoction was prepared by soaking all botanical drugs [Dioscoreae Hypoglaucae Rhizoma, Plantaginis Semen, Atractylodes Lancea (Thunb.) DC., Coicis Semen, Pseudobulbus Cremastrae Seu Pleiones, Phellodendri Chinensis Cortex, Chuanxiong Rhizoma, Cyathulae Radix, and Lonicerae Japonicae Caulisand] for 30 minutes in water and heating to 100 ℃. Next, the formulation was decocted 2 times, and upon combination, the 2 decoctions obtained were subjected to condensing and refluxing; the compound extract (15.1 g) was prepared as a concentrated liquid by using a freeze dryer (VirTis BenchTop Pro, VirTis Co., Ltd., Gardiner, NY, USA).
Animal experiments
The intragastric gavage (IG) suspension was prepared with adenine and 0.5% sodium carboxymethyl cellulose (CMC-Na) solution. Meanwhile, dry yeast powder was added to the regular feed of mice, with the daily intake of yeast being controlled to 10 g/kg (16). A total of 30 mice were assigned into 3 groups in a random fashion (n=10): the blank group, the model group, and the BN group. Random numbers were generated using the random number generator in Microsoft Excel (Microsoft Corp., Redmond, WA, USA). Mice in the blank group were given 0.5% CMC-Na solution at 100 mg/kg, and those in the other 2 groups were administered with a combined 0.5% CMC-Na (100 mg/kg) and adenine (100 mg/kg) by IG once a day at 8:00 a.m. for 28 consecutive days. The research team monitored animals twice daily. Mouse health was monitored by weight, food and water intake, and general assessment of animal activity, panting, and fur condition. When the modeling began, mice in the BN group were separately administered with BN decoction every afternoon. The IG order of each group was randomized. With the prolongation of the modelling time, the mice in the model group showed signs of weight loss and lethargy. The administration group mice were lighter than the model group, but not as healthy as the control group.
Sample collection
Significant increases of UA, BUN, and Cr indicated that the animal model had been established successfully. The animals were excluded if insertion of the IG needle resulted in perforation of the vessel wall, or if the animal died prematurely, preventing the collection of data. A total of 30 mice were therefore included in the analysis in this study. All of the 30 mice were fasted for 12 hours before the last administration. On the 28th day, the blood from all mice was collected for the analysis of UA, BUN, and Cr. Then, the renal tissues were removed, followed by decapitation and weighing. Next, serum was isolated by centrifugation for 10 minutes at 3,000/min at 4 ℃, and the serum levels of BUN, Cr, and UA were tested using an automatic biochemical analyzer. In addition, fresh stool samples were immediately harvested in frozen pipes (20). All biological samples were kept at −80 ℃ until testing.
Renal weight index
On the 28th day, the 30 mice were euthanized quickly, and the kidney index was calculated as double kidney weight/mouse weight × 100%.
16S rRNA gene sequencing
A total of 3 mice were randomly selected from each group. The 16S rRNA gene sequencing was conducted to elucidate whether BN has an effect on GN in mice. Total bacterial DNA was harvested, amplified, and then sequenced on the MiSeq™ platform (Illumina, San Diego, CA, USA) by Majorbio (Shanghai, China). Bioinformatics analysis was implemented based upon operational taxonomic units (OTUs), and these units were clustered based on the 100% sequence similarity according to Uparse11 (http://www.drive5.com/uparse/). For the α-diversity analysis, Mothur1.30.2 (https://www.mothur.org/wiki/Download_mothur) was utilized for the calculation of the Chao1 Index, Shannon Index, Observed Species Index, Good’s Coverage Index, and Simpson’s Diversity Index. The non-metric multidimensional scaling (NMDS) and principal co-ordinate analysis (PCoA) were employed for β-diversity analysis by the unweighted UniFrac method. All processes were performed on MajorbioCloud (https://cloud.majorbio.com/). PICRUSt2 (http://picrust.githubio/) was utilized for predicting the functionally-enriched signaling pathways in gut metabolism (21).
Statistical analysis
SPSS 21.0 was used for statistical analysis. The measurement data were expressed as mean ± standard deviation (SD). A One-way analysis of variance (ANOVA) was used for comparison between groups, and SNK method was further used for pairwise comparison, and P<0.05 was considered as statistically significant.
Results
Screened Targets and PPI Networks Associating with BN and GN
The databases yielded 413 pharmacological targets associated with BN upon the removal of the duplicate targets. A total of 1,085 GN-associated targets were available from all the databases, which were plotted to 118 active component targets. As shown in Figure 1A, the targets of BN for GN therapy were analyzed by the Venny 2.1 (https://bioinfogp.cnb.csic.es/tools/venny/), and the STRING database was employed for the visualization of the PPI networks of BN. In the PPI network, more significant interactions were observed by showing with 416 edges and 90 nodes (Figure 1B). Additionally, the core targets were screened out using the cytoHubba plug-in Cytoscape, and the top 10 genes, including mitogen-activated protein kinase (MAPK)1, proto-oncogene tyrosine-protein kinase Src (SRC), heat shock protein HSP 90-alpha (HSP90AA1), tyrosine-protein phosphatase non-receptor type (PTPN)11, transforming protein RhoA (RHOA), MAPK14, estrogen receptor (ESR)1, tyrosine-protein kinase JAK (JAK)2, signal transducer and activator of transcription 1-alpha/beta (STAT)1, and MAPK8 (Figure 1C), were considered as core genes after combining with the calculation method. These genes may be key targets through which BN plays a role in lowering the UA concentrations (22).
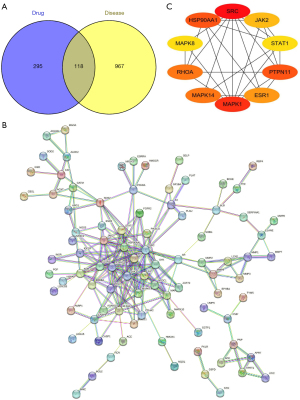
Signaling pathway enrichment using core targets
Subsequently, we performed the pathway enrichment analysis of the aforesaid 10 core genes using the KEGG database (Min Overlap: 3, P value cutoff: 0.01). Finally, 157 metabolic pathways were identified, and the top 20 pathways with the highest enrichment are exhibited in Figure 2.
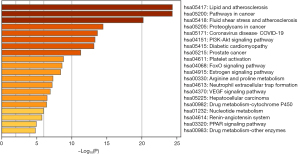
Effect on serum biochemical indexes
As displayed in Figure 3A-3C, we observed that the plasma levels of UA, BUN, and Cr increased significantly. After modeling, mice were euthanized to obtain the kidneys, followed by the calculation of the renal weight index (weight of kidney/ICR mouse weight, mg/g). The comparative findings of the serum levels of UA, BUN, and Cr in each group are displayed in Table 1. According to the results of the one-way ANOVA, we noticed that the serum levels of UA, BUN, and Cr in each group were statistically significant (all P<0.05). Further, the Student-Neuman-Keuls (SNK) method was used to compare the levels of UA, BUN, and Cr in each group, which indicated increased serum levels of UA, BUN, and Cr in the model and the BN groups in comparison to those in the blank group, and the levels of which were lower in the BN group than those in the model group (all P<0.05).
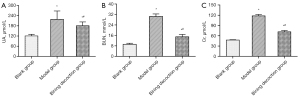
Table 1
Group | n | UA (μmol/L) | BUN (mmol/L) | Cr (μmol/L) |
---|---|---|---|---|
Blank group | 10 | 120.61±9.76 | 9.17±0.85 | 47.56±0.99 |
Model group | 10 | 218.03±49.67* | 30.50±1.92* | 118.24±4.19* |
Bining decoction group | 10 | 180.56±22.63*# | 14.91±1.85*# | 71.64±3.79*# |
F | – | 23.564 | 466.359 | 1177.491 |
P | – | <0.001 | <0.001 | <0.001 |
*, compared with Blank group; #, compared with Model group, all P<0.05. UA, uric acid; BUN, blood urea nitrogen; Cr, creatinine.
Effect on the renal weight index
Table 2 shows the comparative results of the renal indexes of mice in each group. A significant difference was witnessed in the renal weight index in each group based on the results of one-way ANOVA (P<0.05). The results of the SNK method further demonstrated that the renal weight index of mice in the model group and the BN group was elevated when compared to that in the blank group, and the renal weight index was lower in the BN group than that in the model group (P<0.05; Figure 4). It is suggested that BN could decrease the kidney weight index of GN mice.
Table 2
Group | n | Renal weight index (%) |
---|---|---|
Blank group | 10 | 1.12±0.18 |
Model group | 10 | 2.76±0.52* |
Bining decoction group | 10 | 1.65±0.26*# |
F | – | 23.564 |
P | – | <0.001 |
*, compared with Blank group; #, compared with Model group, all P<0.05.
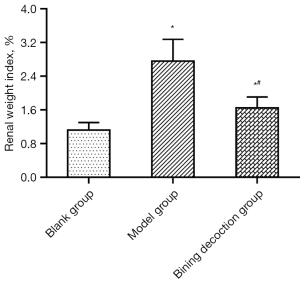
16S rRNA gene sequencing
A growing number of articles have indicated that gut microbiota is regarded as a novel target for personalized drug therapy by impacting host metabolism and maintaining intestinal environment homeostasis (23).
Upon the completion of quality-filtering steps, denoising, and chimera removal, in the case of 100% of the sequence similarity threshold, 316,454 high-quality sequences were gained from 9 samples for further analyses of OTUs of gut microbiota. In the blank group, there were 109 OTUs and 0 unique OTUs; in the model group, 117 OTUs and 0 unique OTUs; in the BN group, 116 OTUs and 1 unique OTUs. These 3 groups possessed 105 OTUs (Figure 5A). The number of OTUs was increased in the model group in contrast to the blank group; the number of OTUs was downregulated in the BN group in comparison to the model group. The slopes of the rank abundance reduced smoothly and flattened eventually, and the wide span of the horizontal coordinates unveiled the rich and uniform species samples (Figure 5B).
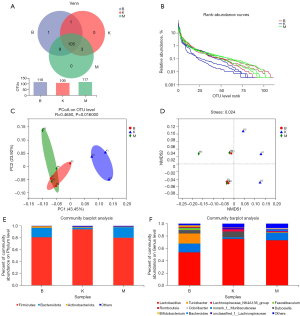
Alpha diversity analysis of gut microbiota
The α-diversity analysis displayed in Table 3 shows an elevation in the Chao Index, Ace Index, Shannon Diversity Index, Good’s Coverage Index, Pd Index, and Sobs Index in the model group when compared with the blank group. This suggests that BN decoction could increase the richness and diversity of the gut microflora. In comparison to the model group, the Chao Index, Ace Index, Shannon Diversity Index, Pd Index, and Sobs Indexes in the BN group were almost increased, which demonstrated that BN could increase the richness and diversity of the intestinal flora. More than 98% of the Good’s Coverage Index suggests high sample coverage and reliable sequencing findings.
Table 3
Group | Ace | Chao | Shannon | Simpson | Good’s coverage | Pd | Sobs |
---|---|---|---|---|---|---|---|
Blank group | 97.080453 | 96.938492 | 1.952064667 | 0.286234667 | 0.999592667 | 10.28976 | 89 |
Model group | 106.942599 | 109.75 | 2.694780667 | 0.149178667 | 0.999760333 | 11.10108 | 103 |
Bining decoction group | 113.5857397 | 116.7333333 | 2.721098333 | 0.142312333 | 0.999688667 | 11.38382667 | 107.6666667 |
Pd, phylogenetic diversity; Sobs, the number of observed OTUs.
Beta diversity analysis of gut microbiota
For the β-diversity analysis, PCoA and NMDS were performed based on the actual sequence variants (ASVs) (Figure 5C,5D). The findings revealed that the microflora of the mice was significantly separated in the model group versus the blank group, indicating a significant difference in the β-diversity between the 2 groups. The BN group exhibited the same tendency as that of the blank group, which disclosed that BN failed to reverse the GN structural alteration. However, BN did play a certain role in GN structure.
Taxonomic composition analysis of gut microbiota
Through the taxonomy analysis displayed in Figure 5E,5F, it can be observed that at the phylum level, Firmicutes and Bacteroidota were the most essential phyla, followed by Actinobacteriota and others. These 5 phyla belong to the dominant phyla and occupy a very high portion of the whole phyla. Gut microbiota sequencing analysis showed the abundance of Faecalibaculum, Romboutsia, Bifidobacterium, Bacteroides, Odoribacter, Lachnospiraceae_NK4A136_group, unclassified_f__Lachnospiraceae, Roseburia, norank_f__Lachnospiraceae, Lactobacillus, Dubosiella, norank_f__Muribaculaceae, and Turicibacter in the BN group had changed significantly.
As shown in Figure 6A-6C, Firmicutes (94.03–79.92%), Bacteroidota (3.01–17.79%), and Actinobacteriota (1.40–0.94%),The ratio of Firmicutes/Bacteroidetes in the blank group and the model group was 0.31 and 0.04, respectively, implying that the dominant flora structure of mice in the model and blank groups has changed significantly; the Firmicutes/Bacteroidetes ratio in the BN group was P<0.05 compared with the model group, implying that BN could modulate the mouse’ dominant flora.
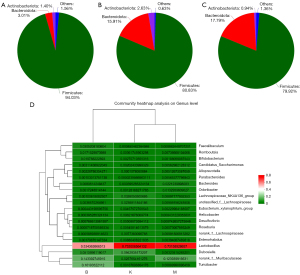
From the subordinate level, by performing a cluster analysis of the absolutely-dominant bacterial genera in the top 20 positions, we discovered and analyzed the abundance of various bacterial genera, namely, Faecalibaculum, Romboutsia, Bifidobacterium, Candidatus_Saccharimonas, Alloprevotella, Parabacteroides, Bacteroides, Odoribacter, Lachnospiraceae_NK4A136_group, unclassified_f__Lachnospiraceae, Eubacterium_xylanophilum_group, Helicobacter, Desulfovibrio, Roseburia, norank_f__Lachnospiraceae, Enterorhabdus, Lactobacillus, Dubosiella, norank_f__Muribaculaceae, and Turicibacter (Figure 6D).
Metabolic pathway prediction in microbiota sample communities
In this study, to identify the metabolic pathway of BN for treating GN, the treatment group was analyzed by using picurst2, and 272 signaling pathways were identified by enrichment analysis (Table 4).
Table 4
Pathway | Description |
---|---|
ko01100 | Metabolic pathways |
ko01110 | Biosynthesis of secondary metabolites |
ko01120 | Microbial metabolism in diverse environments |
ko01230 | Biosynthesis of amino acids |
ko03010 | Ribosome |
ko02010 | ABC transporters |
ko01200 | Carbon metabolism |
ko00230 | Purine metabolism |
ko00010 | Glycolysis/gluconeogenesis |
ko00500 | Starch and sucrose metabolism |
ko02020 | Two-component system |
ko00520 | Amino sugar and nucleotide sugar metabolism |
ko02024 | Quorum sensing |
ko00240 | Pyrimidine metabolism |
ko00970 | Aminoacyl-tRNA biosynthesis |
ko00620 | Pyruvate metabolism |
ko03440 | Homologous recombination |
ko02060 | PTS |
ko00052 | Galactose metabolism |
ko00550 | Peptidoglycan biosynthesis |
ko00270 | Cysteine and methionine metabolism |
ko03430 | Mismatch repair |
ko00250 | Alanine, aspartate and glutamate metabolism |
ko00260 | Glycine, serine and threonine metabolism |
ko00030 | Pentose phosphate pathway |
ko00680 | Methane metabolism |
ko00051 | Fructose and mannose metabolism |
ko03030 | DNA replication |
ko00190 | Oxidative phosphorylation |
ko00720 | Carbon fixation pathways in prokaryotes |
ko00300 | Lysine biosynthesis |
ko03060 | Protein export |
ko00640 | Propanoate metabolism |
ko03018 | RNA degradation |
ko00561 | Glycerolipid metabolism |
ko00900 | Terpenoid backbone biosynthesis |
ko00564 | Glycerophospholipid metabolism |
ko04112 | Cell cycle-caulobacter |
ko03070 | Bacterial secretion system |
ko00710 | Carbon fixation in photosynthetic organisms |
ko00650 | Butanoate metabolism |
ko03410 | Base excision repair |
ko01501 | Beta-lactam resistance |
ko00670 | One carbon pool by folate |
ko05230 | Central carbon metabolism in cancer |
ko01212 | Fatty acid metabolism |
ko00061 | Fatty acid biosynthesis |
ko03420 | Nucleotide excision repair |
ko00730 | Thiamine metabolism |
ko04922 | Glucagon signaling pathway |
ko00630 | Glyoxylate and dicarboxylate metabolism |
ko04066 | HIF-1 signaling pathway |
ko00195 | Photosynthesis |
ko00770 | Pantothenate and CoA biosynthesis |
ko00760 | Nicotinate and nicotinamide metabolism |
ko00983 | Drug metabolism-other enzymes |
ko00020 | Citrate cycle (TCA cycle) |
ko00220 | Arginine biosynthesis |
ko00450 | Selenocompound metabolism |
ko00521 | Streptomycin biosynthesis |
ko01210 | 2-oxocarboxylic acid metabolism |
ko01502 | Vancomycin resistance |
ko01503 | CAMP resistance |
ko00480 | Glutathione metabolism |
ko00790 | Folate biosynthesis |
ko00780 | Biotin metabolism |
ko03020 | RNA polymerase |
ko05111 | Biofilm formation-Vibrio cholerae |
ko00040 | Pentose and glucuronate interconversions |
ko00330 | Arginine and proline metabolism |
ko04122 | Sulfur relay system |
ko00400 | Phenylalanine, tyrosine and tryptophan biosynthesis |
ko01220 | Degradation of aromatic compounds |
ko01523 | Antifolate resistance |
ko00473 | D-alanine metabolism |
ko05150 | Staphylococcus aureus infection |
ko00460 | Cyanoamino acid metabolism |
ko00430 | Taurine and hypotaurine metabolism |
ko00261 | Monobactam biosynthesis |
ko00350 | Tyrosine metabolism |
ko00471 | D-glutamine and D-glutamate metabolism |
ko00071 | Fatty acid degradation |
ko02026 | Biofilm formation-Escherichia coli |
ko00920 | Sulfur metabolism |
ko00600 | Sphingolipid metabolism |
ko04212 | Longevity regulating pathway-worm |
ko00280 | Valine, leucine and isoleucine degradation |
ko00511 | Other glycan degradation |
ko00910 | Nitrogen metabolism |
ko00523 | Polyketide sugar unit biosynthesis |
ko05132 | Salmonella infection |
ko05152 | Tuberculosis |
ko00625 | Chloroalkane and chloroalkene degradation |
ko00860 | Porphyrin and chlorophyll metabolism |
ko00290 | Valine, leucine and isoleucine biosynthesis |
ko00333 | Prodigiosin biosynthesis |
ko00130 | Ubiquinone and other terpenoid-quinone biosynthesis |
ko05418 | Fluid shear stress and atherosclerosis |
ko00750 | Vitamin B6 metabolism |
ko00626 | Naphthalene degradation |
ko00740 | Riboflavin metabolism |
ko00362 | Benzoate degradation |
ko04626 | Plant-pathogen interaction |
ko05134 | Legionellosis |
ko00660 | C5-branched dibasic acid metabolism |
ko00310 | Lysine degradation |
ko04727 | GABAergic synapse |
ko04621 | NOD-like receptor signaling pathway |
ko04217 | Necroptosis |
ko00340 | Histidine metabolism |
ko03013 | RNA transport |
ko00562 | Inositol phosphate metabolism |
ko03320 | PPAR signaling pathway |
ko00627 | Aminobenzoate degradation |
ko00525 | Acarbose and validamycin biosynthesis |
ko04724 | Glutamatergic synapse |
ko00603 | Glycosphingolipid biosynthesis-globo and isoglobo series |
ko05340 | Primary immunodeficiency |
ko04931 | Insulin resistance |
ko04070 | Phosphatidylinositol signaling system |
ko00380 | Tryptophan metabolism |
ko04016 | MAPK signaling pathway-plant |
ko00121 | Secondary bile acid biosynthesis |
ko00410 | Beta-alanine metabolism |
ko00072 | Synthesis and degradation of ketone bodies |
ko02040 | Flagellar assembly |
ko02030 | Bacterial chemotaxis |
ko04146 | Peroxisome |
ko00998 | Biosynthesis of various secondary metabolites-part 2 |
ko00120 | Primary bile acid biosynthesis |
ko00311 | Penicillin and cephalosporin biosynthesis |
ko04940 | Type I diabetes mellitus |
ko00982 | Drug metabolism-cytochrome P450 |
ko00980 | Metabolism of xenobiotics by cytochrome P450 |
ko00830 | Retinol metabolism |
ko00540 | Lipopolysaccharide biosynthesis |
ko00524 | Neomycin, kanamycin and gentamicin biosynthesis |
ko01524 | Platinum drug resistance |
ko04141 | Protein processing in endoplasmic reticulum |
ko01054 | Nonribosomal peptide structures |
ko00053 | Ascorbate and aldarate metabolism |
ko00908 | Zeatin biosynthesis |
ko00332 | Carbapenem biosynthesis |
ko05010 | Alzheimer disease |
ko04152 | AMPK signaling pathway |
ko01055 | Biosynthesis of vancomycin group antibiotics |
ko04930 | Type II diabetes mellitus |
ko05130 | Pathogenic Escherichia coli infection |
ko05165 | Human papillomavirus infection |
ko05203 | Viral carcinogenesis |
ko03008 | Ribosome biogenesis in eukaryotes |
ko05205 | Proteoglycans in cancer |
ko05146 | Amoebiasis |
ko05206 | MicroRNAs in cancer |
ko00360 | Phenylalanine metabolism |
ko04917 | Prolactin signaling pathway |
ko05120 | Epithelial cell signaling in Helicobacter pylori infection |
ko04142 | Lysosome |
ko05200 | Pathways in cancer |
ko00622 | Xylene degradation |
ko00621 | Dioxin degradation |
ko01051 | Biosynthesis of ansamycins |
ko00440 | Phosphonate and phosphinate metabolism |
ko00966 | Glucosinolate biosynthesis |
ko04910 | Insulin signaling pathway |
ko00940 | Phenylpropanoid biosynthesis |
ko00572 | Arabinogalactan biosynthesis-mycobacterium |
ko04213 | Longevity regulating pathway-multiple species |
ko00791 | Atrazine degradation |
ko04934 | Cushing syndrome |
ko05211 | Renal cell carcinoma |
ko00531 | Glycosaminoglycan degradation |
ko00401 | Novobiocin biosynthesis |
ko04964 | Proximal tubule bicarbonate reclamation |
ko04214 | Apoptosis-fly |
ko04216 | Ferroptosis |
ko00785 | Lipoic acid metabolism |
ko02025 | Biofilm formation-pseudomonas aeruginosa |
ko00361 | Chlorocyclohexane and chlorobenzene degradation |
ko00960 | Tropane, piperidine and pyridine alkaloid biosynthesis |
ko04918 | Thyroid hormone synthesis |
ko04138 | Autophagy-yeast |
ko05016 | Huntington disease |
ko04973 | Carbohydrate digestion and absorption |
ko04972 | Pancreatic secretion |
ko00405 | Phenazine biosynthesis |
ko04714 | Thermogenesis |
ko00513 | Various types of N-glycan biosynthesis |
ko04920 | Adipocytokine signaling pathway |
ko01055 | Glycosphingolipid biosynthesis-ganglio series |
ko04930 | Isoquinoline alkaloid biosynthesis |
ko05130 | PI3K-Akt signaling pathway |
ko04622 | RIG-I-like receptor signaling pathway |
ko04612 | Antigen processing and presentation |
ko04915 | Estrogen signaling pathway |
ko04657 | IL-17 signaling pathway |
ko04914 | Progesterone-mediated oocyte maturation |
ko05215 | Prostate cancer |
ko04659 | Th17 cell differentiation |
ko04068 | FoxO signaling pathway |
ko04211 | Longevity regulating pathway |
ko05014 | ALS |
ko00633 | Nitrotoluene degradation |
ko05231 | Choline metabolism in cancer |
ko00590 | Arachidonic acid metabolism |
ko05145 | Toxoplasmosis |
ko04013 | MAPK signaling pathway-fly |
ko00903 | Limonene and pinene degradation |
ko04361 | Axon regeneration |
ko04072 | Phospholipase D signaling pathway |
ko00981 | Insect hormone biosynthesis |
ko00643 | Styrene degradation |
ko00510 | N-glycan biosynthesis |
ko05133 | Pertussis |
ko04210 | Apoptosis |
ko05131 | Shigellosis |
ko04974 | Protein digestion and absorption |
ko00944 | Flavone and flavonol biosynthesis |
ko00140 | Steroid hormone biosynthesis |
ko01053 | Biosynthesis of siderophore group nonribosomal peptides |
ko00565 | Ether lipid metabolism |
ko00592 | Alpha-Linolenic acid metabolism |
ko04011 | MAPK signaling pathway-yeast |
ko00591 | Linoleic acid metabolism |
ko05020 | Prion diseases |
ko04932 | NAFLD |
ko05012 | Parkinson disease |
ko00623 | Toluene degradation |
ko00472 | D-arginine and D-ornithine metabolism |
ko04260 | Cardiac muscle contraction |
ko04614 | Renin-angiotensin system |
ko00281 | Geraniol degradation |
ko01040 | Biosynthesis of unsaturated fatty acids |
ko00930 | Caprolactam degradation |
ko04113 | Meiosis-yeast |
ko05225 | Hepatocellular carcinoma |
ko05017 | Spinocerebellar ataxia |
ko00571 | LAM biosynthesis |
ko05204 | Chemical carcinogenesis |
ko04978 | Mineral absorption |
ko04215 | Apoptosis-multiple species |
ko05210 | Colorectal cancer |
ko05169 | Epstein-Barr virus infection |
ko05161 | Hepatitis B |
ko05160 | Hepatitis C |
ko05168 | Herpes simplex virus 1 infection |
ko05163 | Human cytomegalovirus infection |
ko05170 | Human immunodeficiency virus 1 infection |
ko05164 | Influenza A |
ko05167 | Kaposi sarcoma-associated herpesvirus infection |
ko05162 | Measles |
ko05222 | Small cell lung cancer |
ko05416 | Viral myocarditis |
ko04115 | p53 signaling pathway |
ko05143 | African trypanosomiasis |
ko05142 | Chagas disease (American trypanosomiasis) |
ko03050 | Proteasome |
ko03450 | Non-homologous end-joining |
ko00906 | Carotenoid biosynthesis |
ko04919 | Thyroid hormone signaling pathway |
ko00642 | Ethylbenzene degradation |
ko05034 | Alcoholism |
ko05031 | Amphetamine addiction |
ko00965 | Betalain biosynthesis |
ko05030 | Cocaine addiction |
ko04728 | Dopaminergic synapse |
ko04928 | Parathyroid hormone synthesis, secretion and action |
ko00624 | Polycyclic aromatic hydrocarbon degradation |
ko04726 | Serotonergic synapse |
ko04071 | Sphingolipid signaling pathway |
ko00984 | Steroid degradation |
ko04723 | Retrograde endocannabinoid signaling |
ABC, ATP-binding cassette; PTS, phosphotransferase system; CoA, co-ordinate analysis; TCA, tricarboxylic acid; CAMP, cationic antimicrobial peptide; NOD, nucleotide-binding and oligomerization domain; ALS, amyotrophic lateral sclerosis; MAPK, mitogen-activated protein kinase; NAFLD, non-alcoholic fatty liver disease; LAM, lipoarabinomannan; BN, Bining decoction; GN, gouty nephropathy.
Integrating 16S rRNA gene sequencing and network pharmacology analysis
Combining 16S rRNA gene sequencing and network pharmacology analysis, similar signaling pathways which were followed included “Pathways in cancer” and “Adipocytokine signaling pathway”.
According to the core genes from both the signaling pathways and network pharmacology, we plotted an integrated network map of BN decoction for GN therapy. As demonstrated in Figure 7, the “Pathways in cancer” had the most significant node, which exhibited the strongest association with JAK2.
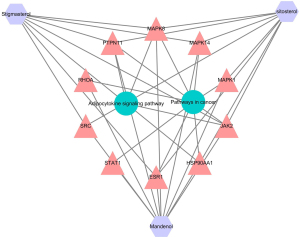
Discussion
GN is a frequent complication of HUA that is caused by the deposition of urate crystals in the kidneys, and its subsequent inflammatory reaction impairs renal function. In this study, ICR mice were given a larger number of urate precursors to accumulate UA in the kidney and damage the nephron for the model establishment. Similar to a previous study, this model was also induced by adenine and yeast powder (24). A higher BUN level is considered one of the most important biochemical indexes for high UA or injury to the kidney (25). Currently, the model is easy to operate and repeat operations.
Revealed by the network pharmacology analysis, the “Pathways in cancer” had the most essential node, which had the strongest correlation with JAK2. These findings suggested that the possible molecular mechanism of BN on GN may be the inhibition of the JAK/STAT pathway.
Recent works have suggested that JAK/STAT signaling, NLRP3 inflammasome signaling, NF-κB signaling, Toll-like receptor signaling, and cGAS-STING signaling control inflammation in cells (26). The JAK/STAT signaling pathway is part of the “pathways in cancer”. Among them, all members of the JAK/STAT signaling are observed in the injured kidney (27,28). This pathway consists of 3 parts: signal factor receptor, JAK, and STAT protein. JAKs are comprised of 4 members, namely JAK1, JAK2, JAK3, and TYK2. Among these, the JAK2 linkage to inflammatory processes is most evident, as it serves as a signaling transmitter downstream of the main cytokine receptors (29,30). The STAT protein, the downstream target of the JAKs family, is responsible for the signal transduction to the nucleus. Most cytokine-initiated immune responses depend on STAT proteins (31). There are 7 numbers for the STAT protein, including STAT1-4, STAT5a, STAT5b, and STAT6. Moreover, multiple cytokines associated with the JAK/STAT information pathway activate specific STAT proteins. The NLRP3 inflammasome is an intracellular supramolecular complex consisting of sensor molecules, the adaptor apoptosis-associated speck-like protein containing a CARD (ASC), as well as the effector protease caspase 1. NLRP3 inflammasome and the successive caspase 1 are activated by reactive oxygen species (ROS), K+ efflux, oxidized mitochondrial DNA release, and lysosomal disruption-induced ion fluxes, thereby executing inflammatory factors production and cell death (32). The type I interferon (IFN) receptors have been found to regulate Caspase-11 via the JAK/STAT signaling pathway and the caspase-1 activation is dependent on the expression of Caspase-11. Activated caspase 1 cleaves both pro-IL-1 and pro-IL-18 for the production of mature IL-1 and IL-18. Meanwhile, it also cleaves and activates the cytoplasmic gasdermin proteins, contributing to cell swelling, cell membrane rupture, cytoplasm efflux, and eventually pyroptosis (33). The inflammatory factors outflow from the gasdermin-contained holes (34), inducing inflammation and injury. Furthermore, recent articles have disclosed that the JAK/STAT signaling pathway could be regarded as a potential target for rheumatoid arthritis (RA) therapy. Recently, novel JAK/STAT inhibitors have been approved by the Food and Drug Administration (FDA) for RA therapy. However, their associated risk and high cost limit their applications for broader use. These restrictions provide a basis for the exploration of novel JAK/STAT inhibitors of natural origin with improved safety, tolerability, and cost-effectiveness (35). One of the aims of investigating the BN is to confirm that the JAK/STAT signaling pathway is closely implicated in the pathological process of GN.
Based on the gut-kidney axis theory, the human gut microbiome is in equilibrium under normal circumstances, and Firmicutes and Bacteroidetes are the dominating bacterial phyla (36). These 2 dominating bacterial phyla were found to occupy more than 95% of the total gut microbiota, which is similar to the findings of other experiments in China and internationally (37). Additionally, Bacteroidetes and Firmicutes differed significantly at the phylum level; both of them were the dominant bacterial at the genus level in the GN model group.
Following previous research findings, the abundance of Firmicutes phylum was decreased and the Bacteroides phylum abundance was elevated in the model group when compared with the blank group (38). Bacteroides was the dominant species that distinguished the intestinal flora between the model and the blank groups. Also, the serum uremic toxins levels were positively correlated with the relative abundance of the Bacteroides genus (39), suggesting that these bacteria play a part in the GN progression. In the model group, the Lactobacillus and Bacteroides abundances were the dominant bacteria. The correlation analysis revealed that Lachnospiraceae was reduced significantly in the model group, which was in line with the previous articles, implying the effectiveness of SCFAs in kidney function (40).
In a chronic kidney disease study, the normal gut microbiome showed an enrichment in Blautia, Bacteroides, Turicibacter, Escherichia-Shigella, and Lachnospiraceae (41), which was similar to our study to some extent. These biota are correlated to systemic inflammation, intestinal barrier integrity, and renal fibrosis (42,43).
Some active ingredients in TCM are capable of impeding the activity of XOD and diminishing the production of UA (44-47). Lactobacillus, Bacteroides, and Turicibacter became the dominant bacteria after the BN intervention. Intestinal bacteria have been shown to decompose UA. Many symbiotic bacteria in the gut, such as Lactobacillus, can express allantoicase and uricase, which participate in UA breakdown (48). Besides, Lactobacillus can also reduce the purine absorption in the gut, preventing the increase of serum uric acid (SUA) and further causing HUA (49). Furthermore, Turicibacter has been found to correlate to intestinal butyric acid, thereby exerting significant anti-inflammatory effects (50).
The combination of network pharmacology and the holistic view of TCM syndrome differentiation and treatment is a methodology with dynamic analysis and holistic view. Through the TCM database website and computer software, the diagnosis and treatment methods of TCM diseases are combined with the compatibility of TCM. The network pharmacology method is used to analyze various active ingredients of TCM or compound prescriptions, and to explore the mechanism of action of compound prescriptions in the treatment of diseases. It solves the difficulties in modern Chinese medicine research and improves the scientific and technological level of modern Chinese medicine research. In this paper, the empirical prescription BN is used as the research object of network pharmacology to predict the key targets and pathways of BN in the treatment of GN. However, it should be noted that network pharmacology technology is only a qualitative prediction of drug components and targets, to determine its clear pharmacological effects still need to be verified by animal experiments or even clinical trials. The in-depth and reasonable application of network pharmacology in the study of the utility mechanism and material basis of TCM compound is worth looking forward. Up to now, wo found no research on GN combining network pharmacology and gut microbiota.
To date, further results with a larger sample size are warranted to validate the findings from the limited sample size in this study. Additionally, a 24-hour urine composition analysis for ICR mice was not performed in this study. Finally, the SCFAs were not conducted with the collected fecal samples due to financial constraints, and a metabonomic analysis was also lacking. Our group will launch in-depth research to address these issues in future and hope to offer a novel avenue for UA-induced nephropathy.
Conclusions
In this study, network pharmacology analysis combined with a 16S rRNA sequencing approach was performed to reveal the gut microbiota composition in GN, and the effect of BN on GN (Figure 8).
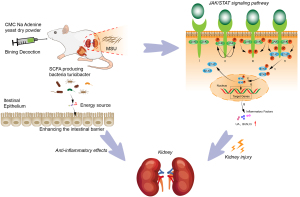
We speculated that BN functioned in GN therapy by inhibiting the JAK/STAT signaling pathway, increasing the beneficial bacteria Turicibacter associated with intestinal butyric acid, which could enhance the intestinal barrier, and exert an anti-inflammatory effect. There have been few Turicibacter-related articles in recent studies. Our findings highlight that BN should be recommended for GN treatment in the development of novel chemopreventive or chemotherapeutic agents.
Acknowledgments
Funding: This work was supported by grants from the Heilongjiang Natural Science Foundation Joint Guidance Project of China (No. LH2019H115 to Ying Tong).
Footnote
Reporting Checklist: The authors have completed the ARRIVE reporting checklist. Available at https://atm.amegroups.com/article/view/10.21037/atm-22-5523/rc
Data Sharing Statement: Available at https://atm.amegroups.com/article/view/10.21037/atm-22-5523/dss
Conflicts of Interest: All authors have completed the ICMJE uniform disclosure form (available at https://atm.amegroups.com/article/view/10.21037/atm-22-5523/coif). YT reports that this work was supported by grants from the Heilongjiang Natural Science Foundation Joint Guidance Project of China (No. LH2019H115). The other authors have no conflicts of interest to declare.
Ethical Statement: The authors are accountable for all aspects of the work in ensuring that questions related to the accuracy or integrity of any part of the work are appropriately investigated and resolved. The study was conducted in accordance with the Declaration of Helsinki (as revised in 2013). Animal experiments were performed under the approval by the Animal Ethics Committee of Heilongjiang University of Chinese Medicine (No. 202009670), in compliance with the Heilongjiang University of Chinese Medicine guidelines for the care and use of animals.
Open Access Statement: This is an Open Access article distributed in accordance with the Creative Commons Attribution-NonCommercial-NoDerivs 4.0 International License (CC BY-NC-ND 4.0), which permits the non-commercial replication and distribution of the article with the strict proviso that no changes or edits are made and the original work is properly cited (including links to both the formal publication through the relevant DOI and the license). See: https://creativecommons.org/licenses/by-nc-nd/4.0/.
References
- Mei Y, Dong B, Geng Z, et al. Excess Uric Acid Induces Gouty Nephropathy Through Crystal Formation: A Review of Recent Insights. Front Endocrinol (Lausanne) 2022;13:911968. [Crossref] [PubMed]
- Towiwat P, Chhana A, Dalbeth N. The anatomical pathology of gout: a systematic literature review. BMC Musculoskelet Disord 2019;20:140. [Crossref] [PubMed]
- Roughley MJ, Belcher J, Mallen CD, et al. Gout and risk of chronic kidney disease and nephrolithiasis: meta-analysis of observational studies. Arthritis Res Ther 2015;17:90. [Crossref] [PubMed]
- Ma Q, Fang L, Su R, et al. Uric acid stones, clinical manifestations and therapeutic considerations. Postgrad Med J 2018;94:458-62. [Crossref] [PubMed]
- FitzGerald JD, Dalbeth N, Mikuls T, et al. 2020 American College of Rheumatology Guideline for the Management of Gout. Arthritis Care Res (Hoboken) 2020;72:744-60. [Crossref] [PubMed]
- Bardin T, Richette P. Impact of comorbidities on gout and hyperuricaemia: an update on prevalence and treatment options. BMC Med 2017;15:123. [Crossref] [PubMed]
- Li Y, Xu W, Zhang F, et al. The Gut Microbiota-Produced Indole-3-Propionic Acid Confers the Antihyperlipidemic Effect of Mulberry-Derived 1-Deoxynojirimycin. mSystems 2020;5:00313-20. [Crossref] [PubMed]
- Yanai H, Adachi H, Hakoshima M, et al. Molecular Biological and Clinical Understanding of the Pathophysiology and Treatments of Hyperuricemia and Its Association with Metabolic Syndrome, Cardiovascular Diseases and Chronic Kidney Disease. Int J Mol Sci 2021;22:9221. [Crossref] [PubMed]
- Dalbeth N, Merriman TR, Stamp LK. Gout. Lancet 2016;388:2039-52. [Crossref] [PubMed]
- Gilbert JA, Blaser MJ, Caporaso JG, et al. Current understanding of the human microbiome. Nat Med 2018;24:392-400. [Crossref] [PubMed]
- Yin H, Liu N, Chen J. The Role of the Intestine in the Development of Hyperuricemia. Front Immunol 2022;13:845684. [Crossref] [PubMed]
- Crane JK, Naeher TM, Broome JE, et al. Role of host xanthine oxidase in infection due to enteropathogenic and Shiga-toxigenic Escherichia coli. Infect Immun 2013;81:1129-39. [Crossref] [PubMed]
- Topping DL, Clifton PM. Short-chain fatty acids and human colonic function: roles of resistant starch and nonstarch polysaccharides. Physiol Rev 2001;81:1031-64. [Crossref] [PubMed]
- Li C, Wang C, Guo Y, et al. Research on the effect and underlying molecular mechanism of Cangzhu in the treatment of gouty arthritis. Eur J Pharmacol 2022;927:175044. [Crossref] [PubMed]
- Yang M, Lao L. Emerging Applications of Metabolomics in Traditional Chinese Medicine Treating Hypertension: Biomarkers, Pathways and More. Front Pharmacol 2019;10:158. [Crossref] [PubMed]
- Ru J, Li P, Wang J, et al. TCMSP: a database of systems pharmacology for drug discovery from herbal medicines. J Cheminform 2014;6:13. [Crossref] [PubMed]
- Liu H, Wang J, Zhou W, et al. Systems approaches and polypharmacology for drug discovery from herbal medicines: an example using licorice. J Ethnopharmacol 2013;146:773-93. [Crossref] [PubMed]
- Zheng Y, Zeng X, Chen P, et al. Integrating Pharmacology and Gut Microbiota Analysis to Explore the Mechanism of Citri Reticulatae Pericarpium Against Reserpine-Induced Spleen Deficiency in Rats. Front Pharmacol 2020;11:586350. [Crossref] [PubMed]
- Zhao H, Gao Q, Kong LZ, et al. Study on Network Pharmacological Analysis and Preliminary Validation to Understand the Mechanisms of Plantaginis Semen in Treatment of Gouty Nephropathy. Evid Based Complement Alternat Med 2020;2020:8861110. [Crossref] [PubMed]
- Zhen Z, Xia L, You H, et al. An Integrated Gut Microbiota and Network Pharmacology Study on Fuzi-Lizhong Pill for Treating Diarrhea-Predominant Irritable Bowel Syndrome. Front Pharmacol 2021;12:746923. [Crossref] [PubMed]
- Yu X, Jiang W, Kosik RO, et al. Gut microbiota changes and its potential relations with thyroid carcinoma. J Adv Res 2022;35:61-70. [Crossref] [PubMed]
- Alam MS, Rahaman MM, Sultana A, et al. Statistics and network-based approaches to identify molecular mechanisms that drive the progression of breast cancer. Comput Biol Med 2022;145:105508. [Crossref] [PubMed]
- Wang Y, Tong Q, Shou JW, et al. Gut Microbiota-Mediated Personalized Treatment of Hyperlipidemia Using Berberine. Theranostics 2017;7:2443-51. [Crossref] [PubMed]
- Yang L, Chang B, Guo Y, et al. The role of oxidative stress-mediated apoptosis in the pathogenesis of uric acid nephropathy. Ren Fail 2019;41:616-22. [Crossref] [PubMed]
- Wang N, Li P, Pan J, et al. Bacillus velezensis A2 fermentation exerts a protective effect on renal injury induced by Zearalenone in mice. Sci Rep 2018;8:13646. [Crossref] [PubMed]
- Yuan Q, Tang B, Zhang C. Signaling pathways of chronic kidney diseases, implications for therapeutics. Signal Transduct Target Ther 2022;7:182. [Crossref] [PubMed]
- Brosius FC 3rd, He JC. JAK inhibition and progressive kidney disease. Curr Opin Nephrol Hypertens 2015;24:88-95. [Crossref] [PubMed]
- Fragiadaki M, Lannoy M, Themanns M, et al. STAT5 drives abnormal proliferation in autosomal dominant polycystic kidney disease. Kidney Int 2017;91:575-86. [Crossref] [PubMed]
- Koschmieder S, Mughal TI, Hasselbalch HC, et al. Myeloproliferative neoplasms and inflammation: whether to target the malignant clone or the inflammatory process or both. Leukemia 2016;30:1018-24. [Crossref] [PubMed]
- Hasselbalch HC. Perspectives on chronic inflammation in essential thrombocythemia, polycythemia vera, and myelofibrosis: is chronic inflammation a trigger and driver of clonal evolution and development of accelerated atherosclerosis and second cancer? Blood 2012;119:3219-25. [Crossref] [PubMed]
- Zhang S, Gan X, Qiu J, et al. IL-10 derived from Hepatocarcinoma cells improves human induced regulatory T cells function via JAK1/STAT5 pathway in tumor microenvironment. Mol Immunol 2021;133:163-72. [Crossref] [PubMed]
- Mangan MSJ, Olhava EJ, Roush WR, et al. Targeting the NLRP3 inflammasome in inflammatory diseases. Nat Rev Drug Discov 2018;17:588-606. [Crossref] [PubMed]
- Cuevas S, Pelegrín P. Pyroptosis and Redox Balance in Kidney Diseases. Antioxid Redox Signal 2021;35:40-60. [Crossref] [PubMed]
- Shi J, Gao W, Shao F. Pyroptosis: Gasdermin-Mediated Programmed Necrotic Cell Death. Trends Biochem Sci 2017;42:245-54. [Crossref] [PubMed]
- Kour G, Choudhary R, Anjum S, et al. Phytochemicals targeting JAK/STAT pathway in the treatment of rheumatoid arthritis: Is there a future? Biochem Pharmacol 2022;197:114929. [Crossref] [PubMed]
- Wexler AG, Goodman AL. An insider's perspective: Bacteroides as a window into the microbiome. Nat Microbiol 2017;2:17026. [Crossref] [PubMed]
- Ruan D, Fouad AM, Fan QL, et al. Dietary L-arginine supplementation enhances growth performance, intestinal antioxidative capacity, immunity and modulates gut microbiota in yellow-feathered chickens. Poult Sci 2020;99:6935-45. [Crossref] [PubMed]
- Ye G, Zhou M, Yu L, et al. Gut microbiota in renal transplant recipients, patients with chronic kidney disease and healthy subjects. Nan Fang Yi Ke Da Xue Xue Bao 2018;38:1401-8. [PubMed]
- Kikuchi M, Ueno M, Itoh Y, et al. Uremic Toxin-Producing Gut Microbiota in Rats with Chronic Kidney Disease. Nephron 2017;135:51-60. [Crossref] [PubMed]
- Pan L, Han P, Ma S, et al. Abnormal metabolism of gut microbiota reveals the possible molecular mechanism of nephropathy induced by hyperuricemia. Acta Pharm Sin B 2020;10:249-61. [Crossref] [PubMed]
- Xu X, Wang H, Guo D, et al. Curcumin modulates gut microbiota and improves renal function in rats with uric acid nephropathy. Ren Fail 2021;43:1063-75. [Crossref] [PubMed]
- Chen L, Chen DQ, Liu JR, et al. Unilateral ureteral obstruction causes gut microbial dysbiosis and metabolome disorders contributing to tubulointerstitial fibrosis. Exp Mol Med 2019;51:1-18. [Crossref] [PubMed]
- Feng YL, Cao G, Chen DQ, et al. Microbiome-metabolomics reveals gut microbiota associated with glycine-conjugated metabolites and polyamine metabolism in chronic kidney disease. Cell Mol Life Sci 2019;76:4961-78. [Crossref] [PubMed]
- Yang Q, Wang Q, Deng W, et al. Anti-hyperuricemic and anti-gouty arthritis activities of polysaccharide purified from Lonicera japonica in model rats. Int J Biol Macromol 2019;123:801-9. [Crossref] [PubMed]
- Adachi SI, Kondo S, Sato Y, et al. Anti-hyperuricemic effect of isorhamnetin in cultured hepatocytes and model mice: structure-activity relationships of methylquercetins as inhibitors of uric acid production. Cytotechnology 2019;71:181-92. [Crossref] [PubMed]
- Cheng LC, Murugaiyah V, Chan KL. Flavonoids and phenylethanoid glycosides from Lippia nodiflora as promising antihyperuricemic agents and elucidation of their mechanism of action. J Ethnopharmacol 2015;176:485-93. [Crossref] [PubMed]
- Lü JM, Yao Q, Chen C. 3,4-Dihydroxy-5-nitrobenzaldehyde (DHNB) is a potent inhibitor of xanthine oxidase: a potential therapeutic agent for treatment of hyperuricemia and gout. Biochem Pharmacol 2013;86:1328-37. [Crossref] [PubMed]
- Crane JK. Role of host xanthine oxidase in infection due to enteropathogenic and Shiga-toxigenic Escherichia coli. Gut Microbes 2013;4:388-91. [Crossref] [PubMed]
- Yamada N, Iwamoto C, Kano H, et al. Evaluation of purine utilization by Lactobacillus gasseri strains with potential to decrease the absorption of food-derived purines in the human intestine. Nucleosides Nucleotides Nucleic Acids 2016;35:670-6. [Crossref] [PubMed]
- Wu M, Yang S, Wang S, et al. Effect of Berberine on Atherosclerosis and Gut Microbiota Modulation and Their Correlation in High-Fat Diet-Fed ApoE-/- Mice. Front Pharmacol 2020;11:223. [Crossref] [PubMed]
(English Language Editor: J. Jones)