Screening of the novel immune-suppressive biomarkers of TMED family and whether knockdown of TMED2/3/4/9 inhibits cell migration and invasion in breast cancer
Introduction
Breast cancer (BC) accounted for 30% of estimated new cases and 15% of deaths of female cancers in 2020 and poses severe threats to women’s lives and health (1). Immune infiltration plays a pivotal role in regulating cancer progress and metastatic dissemination in this solid tumor. However, prognostic biomarkers to assess the tumor immune infiltration are still unavailable in breast cancer.
The transmembrane p24 trafficking protein (TMED) family contains 10 members (TMED1–10) involved in cargo protein transport in the secretory pathway. Among them, TMED2 has been shown to be more highly expressed in sphere-shaped clones of 4T1 murine breast cancer cells than in non-sphere-shaped clones (2). TMED3 and TMED9 have been found to exert pro-tumorigenic effects in human breast cancer (3,4). Meanwhile, the TMED family also have been reported to be involved in several other solid malignancies. The role of TMED3 varies widely depending on the distinct tissue origins; it has been reported as a metastatic suppressor in human colon cancer but as an oncogene in hepatocellular carcinoma, renal cell carcinoma, and lung cancer (5-7). TMED5 was shown to promote malignancy by interaction with WNT7B in cervical cancer (8). Higher TMED9 expression was revealed to predict the poor clinical outcomes of patients in hepatocellular carcinoma and to facilitate colon cancer metastasis via the CNIH4/TGFα/GLI pathway (5,9). Overall, the TMED family members participate in the initiation and progression of numerous tumor types. In addition, aberrant expression of TMED proteins has been shown to cause morphological abnormalities and lead to a broad spectrum of diseases, especially in the dysregulation of immune responses. Loss of TMED1 expression was shown to reduce the production of interleukin 33 (IL-33), which was reported to medicate intra-tumoral immunosuppression in breast cancer (10,11). TMED7 was found to inhibit the immunoadjuvant toll-like receptor 4 (TLR4) pathway, which is essential for radiotherapy and chemotherapy (12,13). However, integrative analysis is still lacking to assess the clinical relevance of TMED family members in breast cancer. Still, their biological roles in the tumor immune microenvironment are currently poorly understood.
In the present study, we screened the prognostic factors of the TMED family in BC and explored their correlation with tumor-immune infiltration using bioinformatics tools. We also validated the expressions of these prognostic factors in human tissue samples and identified their cellular phenotypes and functional properties in breast cancer cell lines. In doing so, we sought to elucidate the diagnostic and prognostic value of the TMED family in breast cancer (14). We present the following article in accordance with the MDAR reporting checklist (available at https://atm.amegroups.com/article/view/10.21037/atm-22-5444/rc).
Methods
Gene expression analysis and ROC analysis
We downloaded five microarray datasets related to breast cancer from the public Gene Expression Omnibus (GEO) database (www.ncbi.nlm.nih.gov/geo/): GSE65212, GSE42568, GSE5364, GSE22820 and GSE45827. The RNA-seq data of invasive breast carcinoma (BRCA) based on 1,097 breast cancer samples and 113 normal breast tissues were acquired in the Genomic Data Commons (GDC) portal (http://portal.gdc.cancer.gov/) and calculated using R software version 4.0.3 (The R Foundation of Statistical Computing, Vienna, Austria). eDatasets above were analyzed using the “Bioinformatics analysis” module on the HOME-for-Researchers website (https://www.home-for-researchers.com/static/index.html#/) and Sangerbox software (http://www.sangerbox.com/tool). Receiver operating characteristic curve (ROC) analysis was performed using the statistic tool of the HOME-for-Researchers website.
The comparison of proteomic data between primary tumor and normal tissue in breast cancer was analyzed using the Clinical Proteomic Tumor Analysis Consortium (CPTAC) module of the University of ALabama at Birmingham CANcer data analysis portal (UALCAN) database (http://ualcan.path.uab.edu/index.html) (15). A P value less than 0.05 was considered statistically significant.
Analyses of clinicopathologic variables and patient outcomes
Targeted expression analysis of the TMED family was conducted using the DNA microarray data of breast tumors and normal tissues from the Breast Cancer Gene-Expression Miner v4.8 (http://bcgenex.ico.unicancer.fr/BC-GEM/GEM-Accueil.php?js=1) (16). The receptor status, nodal status, histological status, Scarff-Bloom-Richardson score (SBR), Nottingham Prognostic Index (NPI) and age were explored. All P values smaller than 0.05 were considered statistically significant.
Kaplan-Meier survival curves were generated using the publicly available database Kaplan-Meier Plotter (KM-Plotter; http://kmplot.com/analysis/) (17). The breast cancer patients were split by the median levels of TMED family members, and the log-rank P values less than 0.05 were considered statistically significant.
Analysis of tumor immunity
We used the “Immune module” of the “Immune Association” part in the web tool Tumor IMmune Estimation Resource 2.0 (TIMER2.0; http://timer.comp-genomics.org/) to assess the associations between TMED expression and tumor-infiltrating immune cells in breast cancer (18). The correlations between TMED expression and landmark genes of immune cells were also evaluated by the “Gene_Corr module” of this website’s “Cancer Exploration” part. The “Purity Adjustment” option was selected according to the website developer’s recommendation. Filter criteria: P value less than 0.05 and |Rho ratio| ≥0.1.
The relationship between key TMED members and the immune checkpoint genes was analyzed and visualized by the “Gene-Immune Analysis” module of SangerBox (http://sangerbox.com/).
A total of 2,483 immune-related genes were obtained from the “Shared Data” module of ImmPort database (https://www.immport.org/home) and then utilized to generate an intersection with the common co-expressed genes of TMED2/3/4/9 by Venn diagram. GeneMANIA software (https://genemania.org/) was queried with default parameters to visualize the protein-protein interaction (PPI) networks between TMED2/3/4/9 and their common co-expressed immune-related genes (19).
Exploration of gene regulatory network
Genomic Profiles of TMED for breast invasive carcinoma [The Cancer Genome Atlas (TCGA); PanCancer Atlas] cohort were generated by querying the “Oncoprint” and “survival analysis” module of cBioPortal database (http://www.cbioportal.org). The co-expressed genes of TMED2/3/4/9 were downloaded from the “Co-expression” module (Filter criteria: P value <0.05 and |Spearman’s Correlation value| ≥0.1), and the common correlated genes of TMED2/3/4/9 were generated by the webtool Venn diagram (http://bioinformatics.psb.ugent.be/webtools/Venn/). The top 200 positively co-expressed genes of TMED2/3/4/9 were separately entered into the Metascape database (http://www.metascape.org) to perform Gene Ontology (GO) enrichment analysis (20), and their common positively co-expressed genes together with TMED2/3/4/9 were submitted into Expression2kinases (X2K) webtool to analyze the upstream regulators, including transcription factors and protein kinases enriched by known PPIs (https://maayanlab.cloud/X2K/) (21).
The multi-omics database LinkedOmics was queried for analyzing TMED-related microRNAs (miRNAs; http://www.linkedomics.org/login.php) (22). Specifically, the RNAseq data of BRCA from platform HiSeq RNA and the miRNASeq data from HS miR were chosen as the “Search dataset” and “Target dataset”, respectively. TMED was submitted as the “Attribute” and Pearson Correlation test as the “Statistical Method”. The Function module LinkFinder analyzed TMED associated miRNAs, and the results were downloaded. Then TMED2/3/4/9 and their commonly associated miRNAs were submitted into the Cytoscape software 3.8.2 (https://cytoscape.org/), and the interaction network was visualized based on their positive or negative correlations.
Human tissue samples and cell lines
This study was conducted in accordance with the principles stated in the Declaration of Helsinki (as revised in 2013). Ethical clearance was granted from the First Affiliated Hospital of Nanjing Medical University Medical Science Research Ethics Committee (Ethics code: 2021-SR-308). Breast cancer specimens were harvested from the Department of General Surgery, the First Affiliated Hospital of Nanjing Medical University, and written informed consent was provided by the patients or their next of kin. Surgical specimens were resected, snap-frozen in liquid nitrogen, and deposited in the Biological Sample Bank of Jiangsu Province Hospital. The catalogue number of samples used in this study were 399, 459, 461, 474, 476, 477 and 488. The breast tumor cells MCF-7, ZR-75-1, BT-474, SK-BR-3, MDA-MB-453, MDA-MB-231, BT-549, Hs-578T, HCC1806, and HCC1937 were purchased from the Cell Bank of the Chinese Academy of Sciences (Shanghai, China). For western blot analysis, frozen human breast cancer samples were ground and homogenized in a mortar and pestle under liquid nitrogen and then lysed in radioimmunoprecipitation assay (RIPA) lysis buffer (Solarbio, Beijing, China) supplemented with protease inhibitors phenylmethylsulfonyl fluoride (PMSF; Beyotime, Shanghai, China). Cells were rinsed twice with phosphate-buffered saline (PBS) and lysed in RIPA buffer on ice.
Western blot assay
The lysates were mixed with 5× protein loading buffer (NCM Biotech, Suzhou, China) and denatured at 99 ℃ for 10 minutes. Equivalent amounts of total tissue or cellular proteins were separated on a 12.5% sodium dodecyl sulfate polyacrylamide gel electrophoresis (SDS-PAGE) gel and subsequently transferred onto polyvinylidene fluoride (PVDF) membranes (Millipore, USA) at constant 200 mA for 50 minutes. After blocking with 5% milk in tris-buffered saline with Tween 20 (TBST) for 6 hours at room temperature, the membranes were incubated with primary antibodies overnight at 4 ℃ and corresponding secondary antibodies at room temperature for 1 hour. Finally, membranes were rinsed 3 times in TBST, exposed with enhanced chemiluminescence (ECL) Plus (Yeasen Biotechnology, China), and imaged on Bio-Rad ChemDoc XRS (Bio-Rad, Hercules, CA, USA). Before re-blotting with another antibody, the membranes were stripped by incubating with weak stripping buffer (Beyotime, China) for 15 minutes and re-blocked for 6 hours. Antibodies used were as follows: anti-TMED2 (1:500, Proteintech, Wuhan, China), anti-TMED3 (1:500, Proteintech), anti-TMED4 (1:300, Proteintech), anti-TMED9 (1:500, Proteintech).
Cell culture and siRNA transfection
Most cells were cultured in basic medium with 10% fetal bovine serum (FBS; the specific culture conditions are listed in Table S1). One day before small interfering RNA (siRNA) transfection, cells were plated at 3×105 cells per well in a 6-well plate. Next day, the plated cells with about 30–50% confluence were washed, and fresh complete medium replaced before treatment. A total of 100 pmol of siRNA against TMED2/3/4/9 or negative control siRNA (RiboBio, Guangzhou, China) were added into 125 µL Opti-MEM medium, complexed with 4ul lipofectamine 8000 (Beyotime).
The siRNA target sequences were as follows:
TMED2 siRNA, GGAAGTCCGGGAGAGAATA;
TMED3 siRNA, GTCAGGTGCTACTGTTGAA;
TMED4 siRNA, CTACCAGGATGGCTCTCTT;
TMED9 siRNA, GCTGCTAAAGACAAGTTGA.
The control sequence (siCONTROL non-targeting siRNA) was from RiboBio.
After resting for 20 minutes, the transfection mix was added dropwise to each well in the 6-well plate. The transfected cells were continually cultured for 48 hours before being harvested.
Wound healing and transwell invasion assay
For wound healing assays, a linear scratch was made on the single-cell layer using a pipette tip, and then the cells were incubated in a fresh medium containing 0.5% FBS for 48 hours. Images of the scratch wounds were captured using a phase-contrast microscope at 0, 24, and 48 hours after scratching, and wound areas were measured using the Image J software (National Institutes of Health, Bethesda, MD, USA).
Transwell invasion assay was carried out using the 24-well transwell chamber (Costar, Washington, DC, USA) with a pore size of 8 µm. Briefly, the Matrigel [Becton, Dickinson, and Co. (BD) Biosciences, Franklin lakes, NJ, USA] was diluted 1:9 with serum-free medium and added into the upper chamber in a 24-well plate. After matrix gel solidification, 1×105 cells were added into the chamber, embedded in the medium with 20% FBS immediately. After incubation for 24 hours, the invading cells on the basal side of the chambers were fixed with 4% paraformaldehyde (PFA), dyed with 0.1% crystal violet, and imaged using a Zeiss Axiocam camera (Zeiss, Oberkochen, Germany).
Statistics
The bioinformatics data of the TMED family were generated by the online web tools. The ROC curve was calculated by the website based on R software version 4.0.3. Statistical analysis of cell migration and invasion data was conducted using paired or independent samples t-test. A P value less than 0.05 was considered statistically significant.
Results
TMED2/3/4/9 was highly expressed in breast cancer tissues and had the diagnostic potential to distinguish breast tumors from normal tissues
According to the study workflow (Figure 1), we first analyzed TMED messenger RNA (mRNA) levels in the 5 GEO microarray datasets of breast cancer, and found expression profiles of TMED2/3/4/9 were significantly elevated in tumors relative to normal tissues, whereas TMED6 was remarkably reduced in cancer tissues. Similar results were obtained from the transcriptome data of TCGA BRCA cohort (Figure 2A and Figure S1). Thus, we selected TMED2/3/4/6/9 for further analysis because they showed a consistent trend of changes in both datatypes.
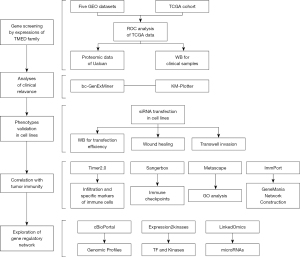
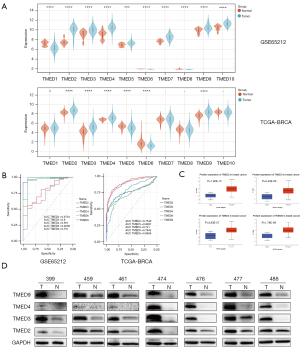
Then, we performed ROC analysis to determine the predictive values of TMED2/3/4/6/9 using mRNA expression data of GSE65212 and TCGA BRCA cohort (Figure 2B), and found that the area under the ROC (AUC) for all candidate TMED members were more than 0.7, indicating that TMED2/3/4//6/9 could distinguish cancer samples from healthy controls {GSE65212 dataset: TMED2: AUC [95% confidence interval (CI)], 0.9734 (0.9483, 0.9986); TMED3: AUC (95% CI), 0.8 (0.6872, 0.9128); TMED4: AUC (95% CI), 0.951 (0.889, 1); TMED6: AUC (95% CI), 0.9238 (0.7787, 1); TMED9: AUC (95% CI), 0.751 (0.6177, 0.8844); TCGA-BRCA dataset: TMED2: AUC (95% CI), 0.7549 (0.7227, 0.7871); TMED3: AUC (95% CI), 0.8997 (0.873, 0.9264); TMED4 AUC (95% CI), 0.741 (0.7088, 0.7732); TMED6 AUC (95% CI), 0.7642 (0.7199, 0.8086); TMED9 AUC (95% CI), 0.8858 (0.8602, 0.9114)}.
To validate the mRNA and protein concordance of candidate TMED members, we queried the proteomic CPTAC data from the UALCAN database (TMED6 data was not available) and confirmed a significant increase in TMED2/3/4/9 protein levels in breast cancer (Figure 2C). Further, we assessed their protein levels in paired cancerous and non-cancerous mammary samples from 7 patients using western blot assay. As expected, higher protein levels of TMED2/3/4/9 were corroborated in most breast cancer tissues compared with paired adjacent normal tissues (Figure 2D).
The correlations between aberrantly expressed TMED2/3/4/9 and clinical variables as well as patient prognosis in breast cancer
To evaluate the clinical and pathological roles of TMED2/3/4/9 in breast cancer, we next performed correlation analysis of TMED2/3/4/9 expression levels with clinical parameters by bc-GenExMiner. Specifically, higher TMED3/4/9 expressions were observed in the >51-year age group than those in the ≤51-year age group. The expression of TMED4 and TMED9 were higher in lymph node-positive patients than lymph node-negative patients, suggesting their potential roles in local invasion and metastasis. Meanwhile, the expression of TMED2 and TMED9 was positively correlated with SBR and NPI, suggesting that high expression of TMED2 and TMED9 in poorly differentiated tumors at diagnosis might indicate an adverse prognosis (Figure 3A).
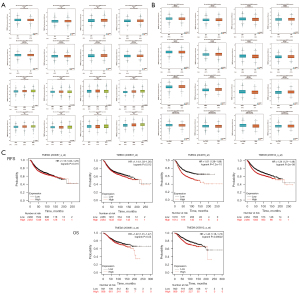
We then examined the differential expression of TMED2/3/4/9 in breast cancer stratified according to the protein level of estrogen receptor (ER), progesterone receptor (PR), human epidermal growth factor receptor-2 (HER-2), and the triple-negative breast cancer (TNBC) status. Briefly, TMED3/9 trended towards higher mRNA expressions in hormone receptor (HR)-negative tumors than HR-positive tumors. Instead, HR-positive tumors expressed relatively high levels of TMED4, respectively. In addition, relative expression of TMED2/3/9 was increased in HER-2 positive tumors compared with HER-2 negative tumors, whereas TMED4 showed an opposite trend. Notably, only TMED9 mRNA level was enriched in TNBCs compared to non-TNBCs, whereas TMED2/3/4 displayed an opposite expression pattern (Figure 3B).
To verify the effect of the TMED family on patient survival, we performed Kaplan-Meier meta-analyses of recurrent-free survival (RFS) and overall survival (OS) using the mRNA chip data of breast cancer in the KM-Plotter database. We found that higher expression of TMED2/3/4/9 correlated with shorter RFS. Furthermore, breast cancer patients with elevated TMED2/9 mRNA levels had a significantly lower OS. In short, a higher mRNA level of TMED2/3/4/9 was correlated with a less favorable prognosis of breast cancer patients (Figure 3C).
Knockdown of TMED2/3/4/9 by siRNA transfection inhibited the migration and invasion of MDA-MB-231 and HCC1937 cells in vitro
To explore the impact of TMED2/3/4/9 on the phenotypes of breast cancer, we first examined the relative expressions of TMED2/3/4/9 in different breast cancer lines (Figure 4A), and further treated MDA-MB-231 and HCC1937 cells by transient transfection of siRNAs, respectively. We confirmed that the protein levels of TMED2/3/4/9 were remarkably suppressed by their corresponding siRNAs (Figure 4B). Next, we analyzed the impacts of transfection by wound-healing assay and found that the migration rates of tumor cells significantly decreased relative to the negative control in both cell lines (Figure 4C). Consistent with the above results, cells that traversed matrix gel and invaded into the lower chamber were remarkably reduced in the experimental group compared to the negative control (Figure 4D). Together, down-regulation of TMED2/3/4/9 suppressed the migratory and invasive abilities of breast cancer cells in vitro.
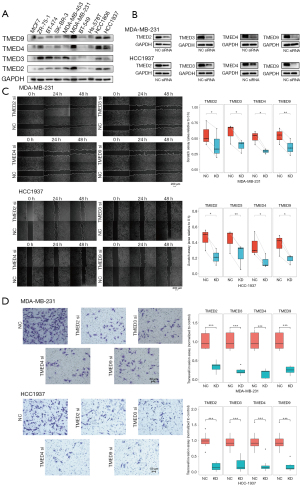
High expressions of TMED2/3/4/9 played essential roles in tumor immunity and indicated the immunosuppressive status in breast cancer.
We queried the TIMER2.0 database to infer immune component abundance depending on the expression of TMED2/3/4/9 in breast cancer (Table 1). Based on most of the algorithms, there was an inverse correlation between their mRNA levels and the infiltration of tumor-suppressive immune cells, including TMED3/9 and CD8+ T cells, TMED2/3/4 and T follicular helper cells, TMED3/4 and dendritic cells (DCs), as well as TMED2/3/4 and natural killer (NK) cells. We also observed the positive associations between TMED3/4 levels and M2-type macrophages infiltrates as well as their negative correlation with M1-type macrophages. However, TMED2/9 was positively correlated with both types of macrophages.
Table 1
Immune cells | Algorithms | TMED2 | TMED3 | TMED4 | TMED9 |
---|---|---|---|---|---|
T cell CD8+ | TIMER | 0.233* | 0.08 | 0.146* | −0.024 |
EPIC | 0.214* | −0.075 | 0.237* | −0.112# | |
MCPCOUNTER | −0.098 | −0.152# | −0.084 | −0.031 | |
CIBERSORT | −0.187# | −0.101# | −0.141# | −0.071 | |
CIBERSORT−ABS | −0.121# | −0.162# | −0.089 | −0.047 | |
QUANTISEQ | −0.052 | −0.17# | −0.058 | −0.037 | |
XCELL | −0.012 | −0.174# | −0.039 | −0.068 | |
Subtype | Naive_XCEL | −0.007 | −0.229# | −0.073 | −0.14# |
Central memory_XCEL | 0.024 | −0.154# | −0.055 | −0.065 | |
Effector memory_XCELL | −0.124# | −0.109# | −0.1 | −0.03 | |
T cell follicular helper | CIBERSORT | −0.227# | −0.137# | −0.216# | −0.047 |
CIBERSORT−ABS | −0.133# | −0.203# | −0.13# | −0.019 | |
DC cells | Myeloid dendritic cell_TIMER | −0.173# | −0.066 | −0.093 | 0.13* |
Myeloid dendritic cell_XCELL | −0.045 | −0.151# | −0.152# | 0.015 | |
Myeloid dendritic cell_MCPCOUNTER | 0.004 | −0.182# | 0.039 | −0.103# | |
Myeloid dendritic cell_QUANTISEQ | 0.207* | −0.08 | 0.097 | −0.081 | |
Myeloid dendritic cell activated_XCELL | −0.111# | −0.135# | −0.187# | 0.044 | |
Plasmacytoid dendritic cell_XCELL | −0.012 | −0.069 | −0.179# | 0.07 | |
NK cells | NK cell_EPIC | −0.247# | −0.062 | −0.355# | 0.121* |
NK cell_MCPCOUNTER | 0.017 | −0.238# | 0.01 | −0.066 | |
NK cell_QUANTISEQ | −0.148# | −0.181# | 0.051 | −0.102# | |
NK cell_XCELL | 0.051 | 0.004 | 0.053 | 0.06 | |
NK cell activated_CIBERSORT | −0.221# | −0.062 | −0.198# | 0.001 | |
NK cell activated_CIBERSORT−ABS | −0.199# | −0.109# | −0.175# | 0.02 | |
Macrophage | EPIC | −0.061 | −0.075 | −0.129# | 0.172* |
TIMER | 0.317* | 0.101* | 0.222* | 0.098 | |
XCELL | 0.095 | 0.045 | −0.076 | 0.208* | |
M0_CIBERSORT | −0.04 | 0.032 | −0.108# | 0.148* | |
M0_CIBERSORT−ABS | −0.035 | −0.033 | −0.106# | 0.148* | |
M1_CIBERSORT | 0.128* | −0.112# | −0.064 | −0.015 | |
M1_CIBERSORT−ABS | 0.093 | −0.164# | −0.025 | −0.002 | |
M1_QUANTISEQ | 0.139* | 0.055 | −0.007 | 0.124* | |
M1_XCELL | −0.037 | 0.012 | −0.189# | 0.242* | |
M2_CIBERSORT | 0.187* | 0.189* | 0.172* | 0.139* | |
M2_CIBERSORT−ABS | 0.186* | −0.034 | 0.155* | 0.114* | |
M2_QUANTISEQ | 0.078 | 0.022 | 0.178* | −0.023 | |
M2_XCELL | 0.039 | 0.118* | −0.07 | 0.189* | |
M2_TIDE | −0.116# | 0.097 | 0.03 | −0.086 | |
Macrophage/Monocyte_MCPCOUNTER | 0.122* | −0.088 | −0.017 | 0.109* |
*, represents positive correlation (P<0.05 and Rho >0.1); whereas #, represents negative correlation (P<0.05 and Rho <−0.1). The regular numbers with colorless background indicate not significant. mRNA, messenger RNA; TMED, transmembrane p24 trafficking protein; DC, dendritic cells; NK, natural killer.
Afterward, we analyzed the associations between TMED2/3/4/9 expression and landmark genes of immune cells. As shown in Table 2, TMED2/3/4 was inversely related to hallmarks of 2 major adaptive immune cells, including general T cells (CD3D, CD3E, CD2) and B cells (CD19, CD79A). Consistent with the above immune cell types estimated by multiple algorithms, TMED3 was inversely associated with CD8+ T cells (CD8A, CD8B) and DCs, whereas TMED3/4 was negatively correlated with NK cells. In addition, TMED2/9 positively correlated with specific markers of M2-type macrophages (CD163, MS4A4A, and VSIG4) and tumor-associated macrophages (CCL-2, CD68, IL10).
Table 2
Immune cells | Gene markers | TMED2 | TMED3 | TMED4 | TMED9 |
---|---|---|---|---|---|
T cell (general) | CD3D | −0.113# | −0.195# | −0.161# | −0.009 |
CD3E | −0.053 | −0.207# | −0.102# | −0.052 | |
CD2 | 0.001 | −0.201# | −0.081 | −0.028 | |
CD8+ T cell | CD8A | 0.008 | −0.206# | −0.013 | −0.075 |
CD8B | −0.098 | −0.152# | −0.084 | −0.031 | |
Tex | PD-1 (PDCD1) | −0.173# | −0.19# | −0.126# | 0.058 |
CTLA4 | −0.024 | −0.203# | −0.139# | 0.019 | |
LAG3 | −0.128# | −0.17# | −0.201# | 0.089 | |
TIM-3 (HAVCR2) | 0.186* | −0.061 | 0.042 | 0.137* | |
GZMB | −0.058 | −0.166# | −0.209# | 0.039 | |
B cell | CD19 | −0.132# | −0.143# | −0.106# | −0.048 |
CD79A | −0.101# | −0.149# | −0.109# | −0.073 | |
NK cell | KIR2DL3 | 0.031 | −0.12# | −0.054 | −0.017 |
KIR2DL4 | 0.014 | −0.134# | −0.083 | 0.002 | |
KIR3DL1 | 0.002 | −0.115# | −0.043 | 0.016 | |
KIR3DL2 | −0.027 | −0.135# | −0.109# | −0.041 | |
KIR2DS4 | 0.029 | −0.113# | −0.063 | −0.033 | |
DC | HLA-DPB1 | −0.193# | −0.064 | −0.096 | 0.119* |
HLA-DQB1 | −0.113# | −0.118# | −0.132# | 0.089 | |
HLA-DRA | 0.049 | −0.11# | −0.015 | 0.037 | |
BCDA-1 (CD1C) | −0.109# | −0.111# | 0.015 | −0.121# | |
BDCA-4 (NRP1) | 0.235* | −0.019 | 0.182* | −0.065 | |
TAM | CCL2 | 0.043 | −0.128# | −0.101# | 0.002 |
CD68 | 0.149* | −0.036 | 0.029 | 0.192* | |
IL10 | 0.197* | −0.093 | 0.016 | 0.162* | |
M1 macrophage | IRF5 | 0.056 | −0.05 | 0.15* | 0.18* |
COX2 (PTGS2) | 0.044 | −0.142# | −0.012 | −0.098 | |
M2 macrophage | CD163 | 0.202* | −0.075 | 0.113* | 0.199* |
VSIG4 | 0.092 | −0.031 | 0.072 | 0.133* | |
MS4A4A | 0.17* | −0.085 | 0.056 | 0.118* |
*, represents positive correlation (P<0.05 and Rho >0.1); whereas #, represents negative correlation (P<0.05 and Rho <−0.1). The regular numbers with colorless background indicate not significant. TMED, transmembrane p24 trafficking protein; mRNA, messenger RNA; DC, dendritic cells; NK, natural killer; TAM, tumor associated macrophages.
For the relationship between TMED2/3/4/9 and the immune checkpoint genes, we noted that TMED2/3/4/9 showed negative correlations with a range of immune checkpoint genes such as PD-1 and CTLA4, which might indicate the immunosuppressive status in breast cancer (Figure 5A).
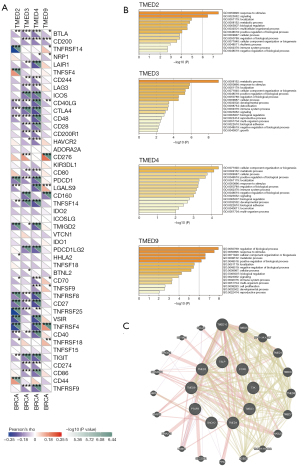
Based on GO enrichment analysis of the top 200 co-expressed genes of TMED2/3/4/9, we found that each member of TMED2/3/4/9 participated in the immune system process (Figure 5B). We also identified CCR6, TXK, TSLP, BACH2, and PTGFR as the common co-expressed immune-related genes of TMED2/3/4/9, their prognostic values in breast cancer (Figures S2,S3 and Tables S2-S4) and the interactome network generated by GeneMANIA elucidated their central roles in the gene regulatory network (Figure 5C).
Exploration of genomic profiles, upstream regulators, and related miRNAs of TMED2/3/4/9
To investigate genetic alterations of TMED2/3/4/9 in breast cancer samples, we focused on the genomic profiles of TMED2/3/4/9 in the selected TCGA PanCancer Atlas breast cancer cohort. The genetic alteration percent of TMED2/3/4/9 in 994 samples was 7%, 5%, 10%, and 9%, respectively, and their variability was dominated by high expressions of mRNA (Figure 6A). Further survival analysis indicated that the patients in the altered group had shorter progression free survival (PFS), OS, and disease free survival (DFS) compared to patients in the unaltered group (Figure 6B).
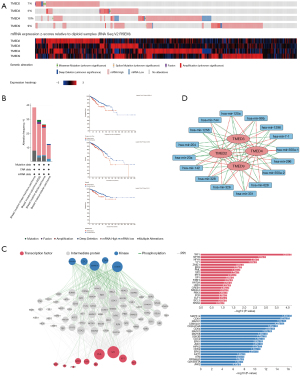
To probe the upstream regulatory network, we performed kinases and transcription factors enrichment analysis by submitting TMED2/3/4/9 and their common 54 positively correlated genes to X2K. As shown in Figure 6C, TAF1, NFYB, NFYA, YY1, ZMIZ1, ERG, PML, SP2, IRF3, and SP1 were identified as the top 10 transcription factors, and MAPK14, CDK1, MAPK1, CSNK2A1, CK2ALPHA, CDK4, MAPK3, MAPK8, GSK3B, and CDC2 as the top 10 protein kinases.
Given the importance of miRNAs in post-transcriptional regulation, we explored TMED2/3/4/9 associated miRNAs in breast cancer by extracting the data from LinkedOmics. Firstly, TMED2/3/4/9 correlated miRNAs were acquired, respectively, and then their common miRNAs were determined by further overlapping (Figure S4). As shown in the interaction network visualized by Cytoscape (Figure 6D), TMED2/3/4/9 shared the common related miRNAs including hsa-mir-1258, hsa-mir-125a, hsa-mir-1296, hsa-mir-142, hsa-mir-204, hsa-mir-20a, hsa-mir-296, hsa-mir-326, hsa-mir-328, hsa-mir-331, hsa-mir-550a-1, hsa-mir-550a-2, hsa-mir-628, hsa-mir-7-1, hsa-mir-744, and hsa-mir-99b. Among them, only hsa-mir-1258 and hsa-mir-204 negatively correlated with all of the 4 members, whereas the other miRNAs positively related to some TMEDs only occasionally. In brief, aberrant expression of TMED2/3/4/9 was determined at the gene level and transcriptional level and interacted with a seizes of epigenetic regulators such as miRNAs.
Discussion
The TMED family members serve as important regulators of the vesicular trafficking of proteins in the cytoplasm. They mainly participate in the anterograde transport of coatomer II (COP II) coated vesicles as well as the retrograde transport of coatomer I (COP I) coated vesicles between the endoplasmic reticulum and Golgi apparatus. TMED7 could also aid the delivery of TLR4 to the plasma membrane (23). Additionally, TMED10 forms a protein channel in the endoplasmic reticulum to facilitate the entry of cytosolic proteins lacking signal peptides by an unconventional protein secretion (24). It has been shown that dysregulation of TMED family members leads to morphological defects of the mouse embryo and participated in Alzheimer’s disease, Mucin 1 kidney disease, and tumors in humans (25-27). Previous studies have revealed that the TMED family proteins influence the malignant progression of several cancers, including the pro-tumor effects of TMED2, TMED3, and TMED9 on breast cancer, but it has remained unclear whether they could be used as ideal biomarkers for this tumor type. Increasing evidence has suggested that the tumor microenvironment has an important impact on the invasion and metastasis of solid tumors. To date, reports of TMED members on tumor immune infiltration have remained unavailable. Hence, we aimed to probe the roles of the whole TMED family in breast cancer, particularly their modulations on tumor immune responses. In this study, we observed that TMED2/3/4/9 was highly expressed in breast cancer tissues at both mRNA and protein levels. We verified the high expression of TMED2/3/9 in paired human breast cancer samples which were consistent with the previous studies (2-4). To our knowledge, our findings regarding the high expression of TMED4 in breast cancer have not been previously reported. We also showed the diagnostic value of TMED2/3/4/9 mRNA levels by ROC and the coincidence of their expression patterns at the protein level.
Previously, little attention has been paid to the histopathological importance of TMED2/3/4/9 in breast cancer. However, we found that upregulated TMED2/3/4/9 mRNA levels correlated a range of pathological parameters, which might explain the inverse associations between TMED2/3/4/9 expression and patient survival. For example, higher levels of TMED4/9 were observed in node-positive groups and increased TMED2/9 expressions in high-grade tumors. We also found that TMED2/3/4/9 levels varied enormously among different molecular subtypes in breast cancer. It might confirm that a high degree of tumoral heterogeneity had a considerable impact on the expression pattern of TMED2/3/4/9 in this tumor. Noticeably, despite the positive correlation between TMED3 mRNA level and HR status consistent with previous studies, its positive relation to HER-2 status contradicted Zhang et al.’s observations in tissue microarray (28). Moreover, the negative relation between TMED3 mRNA level and TNBC status was not observed in Pei et al.’s study (3). These inconsistencies might be attributed to the variance of specimen types and sample size, for the data analyzed in this study were gene expression data from DNA microarrays of 11,359 samples which was quite different from previous formalin-fixed paraffin-embedded (FFPE) tissue samples. For the impacts of TMED2/3/4/9 on the phenotypes of breast cancer cells, we observed that downregulation of their protein levels attenuated the migratory and invasive capacities of tumor cells in vitro. Consistent results were obtained in previous studies on TMED3 and TMED9 in breast cancer, and we observed similar effects of TMED2 and TMED4, which had never been reported before. The oncologic roles of TMED2/3/9 were also supported by similar results from the research on other tumors, including hepatocellular carcinoma, ovarian cancer, lung cancer, and chordoma (6,7,9,29-31). Interestingly, the opposed function of TMED9 against the metastatic suppressor effect of TMED3 in colon cancer might be caused by the distinct roles of the same gene based on the different genetic background (5).
Treatment for high-risk early TNBC with pembrolizumab has been determined to be a promising approach. The Food and Drug Administration (FDA) has approved it for use as (neo)adjuvant therapy for patients with this disease (32). Immune checkpoint inhibitor (ICB) responses are greatly influenced by the tumor microenvironment. Cells of the lymphoid and myeloid types make up the immune microenvironment of breast cancer. The lymphocytes in the body include CD8+ T cells, CD4+ T cells, T regulatory cells, B cells, and CD38+ lymphocytes, while myeloid cells are mainly composed of macrophages, granulocytes, and dendritic cells (33). CD8+ T cells and NK cells were more abundant in tumors that responded to PD-1 inhibitors than in nonresponsive tumors, and macrophages were less abundant (34). Additionally, tumors sensitive to ICB had an increased number of B cells and tertiary lymphoid structures (35). In spite of this, the role of the tumor microenvironment in modulating immune checkpoint inhibitor responses is still unclear. Therefore, identifying immunotherapy targets and biomarkers is critical to improving the effectiveness of therapy (36).
Although the requirement of TMED1 for innate immune signaling and the potentiating effects of TMED2 on anti-virus immunity, the roles of the TMED family on tumor immunity have not yet been clarified (11,37). Thus, we conducted multi-dimensional analysis on the TMED family, including their correlations with infiltrated immune cells and immune-cell specific markers. Importantly, our findings revealed that TMED2/3/4/9 could serve as potential biomarkers indicative of the immunosuppressive state in breast cancer, for their relative abundance inversely correlated with the infiltration of tumor antigen-presenting cells such as DCs and T follicular helper cells, and the tumor-killing effector cells including M1-type macrophages, CD8+ T cells, and NK cells (38-44). Meanwhile, TMED3/4 expression was positively related to M2-type macrophages, which significantly contributed to tumor invasion, metastasis, and immune escape (45). Paradoxically, TMED2/9 was positively correlated with both types of macrophages but was only positively related to specific markers of M2-type macrophages. This discordance might be due to 2 different analytical approaches, and we tended to believe their combined results, that is, the positive correlation between TMED2/9 and M2-type macrophages rather than M1-type macrophages. Notably, TMED2/3/4/9 potentially emerged as a novel indicator in the field of immunotherapy for their negative correlations with numerous immune checkpoint genes, including the well-known PD-1 and CTLA4 (46,47). For the low response rates of breast cancer to immunotherapy, biomarkers like TMED2/3/4/9 that could distinguish immune-active or immune-suppressive status at the tumor site were helpful to identify the targeted population who might benefit from immunotherapy (48,49). Furthermore, we found that the co-expressed genes of TMED2/3/4/9 were enriched into the immune-related pathway, suggesting the important roles of TMED2/3/4/9 in tumor immunity.
We also investigated the potential underlying mechanisms of abnormal TMED2/3/4/9 expression at the genomic and transcriptional level as well as post-transcriptional level. Our findings suggested that genetic alterations of TMED2/3/4/9 had a considerable impact on patient survival, and their aberrant expression might be the integrated regulation effect of numerous kinases, transcription factors, and miRNAs. Interestingly, their common negatively correlated hsa-mir-1258 and hsa-mir-204 were tumor suppressors regulating the metastasis or immune microenvironment remodeling in breast cancer (50,51).
There were some shortcomings in the current study. First, due to the insufficient sample size for protein-level validation, more clinical samples should be collected to evaluate TMED2/3/4/9 expressions by different assays, including western blot and immunohistochemistry (IHC) staining. Importantly, to stratify patients with breast cancer, the correlations between IHC scores of TMED2/3/4/9 in tissues with other pathological parameters will help to find out the best cutoff value to predict the patients’ outcomes. Second, the present works on TMED2/3/4/9’s impact on tumor immunity and regulatory network were predominantly based on bioinformatic analysis. More experiments are needed to unravel the complex underlying mechanisms.
Conclusions
In summary, we conducted an integrative analysis of the roles of the TMED family in breast cancer by bioinformatic data-mining and experimental validation. We identified TMED2/3/4/9 as the potential immune-suppressive biomarkers in this tumor. Further study should focus on confirming our findings by larger sample-sized specimens at the protein level, and the precise mechanism of their relationships with different types of immune-suppressive cells need to be elucidated.
Acknowledgments
The authors would like to gratefully acknowledge all of the patients and their families who contributed specimens to the study, and the surgical staff who assisted in the collection of specimens.
Funding: This research was supported by National Natural Science Foundation of China (No. 81902987); National Natural Science Foundation of China under Grant (No. 81872365); and the Jiangsu Province Postgraduate Cultivation Innovation Project-Graduate Research and Practice Innovation Program (No. KYCX21 1713).
Footnote
Reporting Checklist: The authors have completed the MDAR reporting checklist. Available at https://atm.amegroups.com/article/view/10.21037/atm-22-5444/rc
Data Sharing Statement: Available at https://atm.amegroups.com/article/view/10.21037/atm-22-5444/dss
Conflicts of Interest: All authors have completed the ICMJE uniform disclosure form (available at https://atm.amegroups.com/article/view/10.21037/atm-22-5444/coif). The authors have no conflicts of interest to declare.
Ethical Statement: The authors are accountable for all aspects of the work in ensuring that questions related to the accuracy or integrity of any part of the work are appropriately investigated and resolved. This study was conducted in accordance with the principles stated in the Declaration of Helsinki (as revised in 2013). Ethical clearance was granted from the First Affiliated Hospital of Nanjing Medical University Medical Science Research Ethics Committee (Ethics code: 2021-SR-308). Written informed consent was provided by the patients or their next of kin.
Open Access Statement: This is an Open Access article distributed in accordance with the Creative Commons Attribution-NonCommercial-NoDerivs 4.0 International License (CC BY-NC-ND 4.0), which permits the non-commercial replication and distribution of the article with the strict proviso that no changes or edits are made and the original work is properly cited (including links to both the formal publication through the relevant DOI and the license). See: https://creativecommons.org/licenses/by-nc-nd/4.0/.
References
- Siegel RL, Miller KD, Jemal A. Cancer statistics, 2020. CA Cancer J Clin 2020;70:7-30. [Crossref] [PubMed]
- Lin X, Liu J, Hu SF, et al. Increased expression of TMED2 is an unfavorable prognostic factor in patients with breast cancer. Cancer Manag Res 2019;11:2203-14. [Crossref] [PubMed]
- Pei J, Zhang J, Yang X, et al. TMED3 promotes cell proliferation and motility in breast cancer and is negatively modulated by miR-188-3p. Cancer Cell Int 2019;19:75. [Crossref] [PubMed]
- Ju G, Xu C, Zeng K, et al. High expression of transmembrane P24 trafficking protein 9 predicts poor prognosis in breast carcinoma. Bioengineered 2021;12:8965-79. [Crossref] [PubMed]
- Mishra S, Bernal C, Silvano M, et al. The protein secretion modulator TMED9 drives CNIH4/TGFα/GLI signaling opposing TMED3-WNT-TCF to promote colon cancer metastases. Oncogene 2019;38:5817-37. [Crossref] [PubMed]
- Zheng H, Yang Y, Han J, et al. TMED3 promotes hepatocellular carcinoma progression via IL-11/STAT3 signaling. Sci Rep 2016;6:37070. [Crossref] [PubMed]
- Xie A, Xu X, Kuang P, et al. TMED3 promotes the progression and development of lung squamous cell carcinoma by regulating EZR. Cell Death Dis 2021;12:804. [Crossref] [PubMed]
- Yang Z, Sun Q, Guo J, et al. GRSF1-mediated MIR-G-1 promotes malignant behavior and nuclear autophagy by directly upregulating TMED5 and LMNB1 in cervical cancer cells. Autophagy 2019;15:668-85. [Crossref] [PubMed]
- Yang YC, Chien MH, Lai TC, et al. Proteomics-based identification of TMED9 is linked to vascular invasion and poor prognoses in patients with hepatocellular carcinoma. J Biomed Sci 2021;28:29. [Crossref] [PubMed]
- Jovanovic IP, Pejnovic NN, Radosavljevic GD, et al. Interleukin-33/ST2 axis promotes breast cancer growth and metastases by facilitating intratumoral accumulation of immunosuppressive and innate lymphoid cells. Int J Cancer 2014;134:1669-82. [Crossref] [PubMed]
- Connolly DJ, O'Neill LA, McGettrick AF. The GOLD domain-containing protein TMED1 is involved in interleukin-33 signaling. J Biol Chem 2013;288:5616-23. [Crossref] [PubMed]
- Doyle SL, Husebye H, Connolly DJ, et al. The GOLD domain-containing protein TMED7 inhibits TLR4 signalling from the endosome upon LPS stimulation. Nat Commun 2012;3:707. [Crossref] [PubMed]
- Apetoh L, Ghiringhelli F, Tesniere A, et al. Toll-like receptor 4-dependent contribution of the immune system to anticancer chemotherapy and radiotherapy. Nat Med 2007;13:1050-9. [Crossref] [PubMed]
- Aber R, Chan W, Mugisha S, et al. Transmembrane emp24 domain proteins in development and disease. Genet Res (Camb) 2019;101:e14. [Crossref] [PubMed]
- Chandrashekar DS, Bashel B, Balasubramanya SAH, et al. UALCAN: A Portal for Facilitating Tumor Subgroup Gene Expression and Survival Analyses. Neoplasia 2017;19:649-58. [Crossref] [PubMed]
- Jézéquel P, Gouraud W, Ben Azzouz F, et al. bc-GenExMiner 4.5: new mining module computes breast cancer differential gene expression analyses. Database (Oxford) 2021;2021:baab007. [Crossref] [PubMed]
- Győrffy B. Survival analysis across the entire transcriptome identifies biomarkers with the highest prognostic power in breast cancer. Comput Struct Biotechnol J 2021;19:4101-9. [Crossref] [PubMed]
- Li T, Fu J, Zeng Z, et al. TIMER2.0 for analysis of tumor-infiltrating immune cells. Nucleic Acids Res 2020;48:W509-14. [Crossref] [PubMed]
- Warde-Farley D, Donaldson SL, Comes O, et al. The GeneMANIA prediction server: biological network integration for gene prioritization and predicting gene function. Nucleic Acids Res 2010;38:W214-20. [Crossref] [PubMed]
- Zhou Y, Zhou B, Pache L, et al. Metascape provides a biologist-oriented resource for the analysis of systems-level datasets. Nat Commun 2019;10:1523. [Crossref] [PubMed]
- Clarke DJB, Kuleshov MV, Schilder BM, et al. eXpression2Kinases (X2K) Web: linking expression signatures to upstream cell signaling networks. Nucleic Acids Res 2018;46:W171-9. [Crossref] [PubMed]
- Vasaikar SV, Straub P, Wang J, et al. LinkedOmics: analyzing multi-omics data within and across 32 cancer types. Nucleic Acids Res 2018;46:D956-63. [Crossref] [PubMed]
- Liaunardy-Jopeace A, Bryant CE, Gay NJ. The COP II adaptor protein TMED7 is required to initiate and mediate the delivery of TLR4 to the plasma membrane. Sci Signal 2014;7:ra70. [Crossref] [PubMed]
- Zhang M, Liu L, Lin X, et al. A Translocation Pathway for Vesicle-Mediated Unconventional Protein Secretion. Cell 2020;181:637-652.e15. [Crossref] [PubMed]
- Jerome-Majewska LA, Achkar T, Luo L, et al. The trafficking protein Tmed2/p24beta(1) is required for morphogenesis of the mouse embryo and placenta. Dev Biol 2010;341:154-66. [Crossref] [PubMed]
- Shin JH, Park SJ, Jo DS, et al. Down-regulated TMED10 in Alzheimer disease induces autophagy via ATG4B activation. Autophagy 2019;15:1495-505. [Crossref] [PubMed]
- Dvela-Levitt M, Kost-Alimova M, Emani M, et al. Small Molecule Targets TMED9 and Promotes Lysosomal Degradation to Reverse Proteinopathy. Cell 2019;178:521-535.e23. [Crossref] [PubMed]
- Zhang X, Luo Y, Li Q. TMED3 Promotes Proliferation and Migration in Breast Cancer Cells by Activating Wnt/β-Catenin Signaling. Onco Targets Ther 2020;13:5819-30. [Crossref] [PubMed]
- Zhang X, Hao HH, Zhuang HW, et al. Circular RNA circ_0008305 aggravates hepatocellular carcinoma growth through binding to miR-186 and inducing TMED2. J Cell Mol Med 2022;26:1742-53. [Crossref] [PubMed]
- Shi-Peng G, Chun-Lin C, Huan W, et al. TMED2 promotes epithelial ovarian cancer growth. Oncotarget 2017;8:94151-65. [Crossref] [PubMed]
- Yang J, Huang H, Xiao D, et al. Knockdown of TMED3 inhibits cell viability and migration and increases apoptosis in human chordoma cells. Int J Oncol 2021;58:15. [Crossref] [PubMed]
- Schmid P, Cortes J, Dent R, et al. Event-free Survival with Pembrolizumab in Early Triple-Negative Breast Cancer. N Engl J Med 2022;386:556-67. [Crossref] [PubMed]
- Danenberg E, Bardwell H, Zanotelli VRT, et al. Breast tumor microenvironment structures are associated with genomic features and clinical outcome. Nat Genet 2022;54:660-9. [Crossref] [PubMed]
- Salemme V, Centonze G, Cavallo F, et al. The Crosstalk Between Tumor Cells and the Immune Microenvironment in Breast Cancer: Implications for Immunotherapy. Front Oncol 2021;11:610303. [Crossref] [PubMed]
- Riaz N, Havel JJ, Makarov V, et al. Tumor and Microenvironment Evolution during Immunotherapy with Nivolumab. Cell 2017;171:934-949.e16. [Crossref] [PubMed]
- Hollern DP, Xu N, Thennavan A, et al. B Cells and T Follicular Helper Cells Mediate Response to Checkpoint Inhibitors in High Mutation Burden Mouse Models of Breast Cancer. Cell 2019;179:1191-1206.e21. [Crossref] [PubMed]
- Sun MS, Zhang J, Jiang LQ, et al. TMED2 Potentiates Cellular IFN Responses to DNA Viruses by Reinforcing MITA Dimerization and Facilitating Its Trafficking. Cell Rep 2018;25:3086-3098.e3. [Crossref] [PubMed]
- Michea P, Noël F, Zakine E, et al. Adjustment of dendritic cells to the breast-cancer microenvironment is subset specific. Nat Immunol 2018;19:885-97. [Crossref] [PubMed]
- Gu-Trantien C, Loi S, Garaud S, et al. CD4+ follicular helper T cell infiltration predicts breast cancer survival. J Clin Invest 2013;123:2873-92. [Crossref] [PubMed]
- Noël G, Fontsa ML, Garaud S, et al. Functional Th1-oriented T follicular helper cells that infiltrate human breast cancer promote effective adaptive immunity. J Clin Invest 2021;131:e139905. [Crossref] [PubMed]
- Ahirwar DK, Charan M, Mishra S, et al. Slit2 Inhibits Breast Cancer Metastasis by Activating M1-Like Phagocytic and Antifibrotic Macrophages. Cancer Res 2021;81:5255-67. [Crossref] [PubMed]
- Ali HR, Provenzano E, Dawson SJ, et al. Association between CD8+ T-cell infiltration and breast cancer survival in 12,439 patients. Ann Oncol 2014;25:1536-43. [Crossref] [PubMed]
- Byrne A, Savas P, Sant S, et al. Tissue-resident memory T cells in breast cancer control and immunotherapy responses. Nat Rev Clin Oncol 2020;17:341-8. [Crossref] [PubMed]
- Muntasell A, Rojo F, Servitja S, et al. NK Cell Infiltrates and HLA Class I Expression in Primary HER2(+) Breast Cancer Predict and Uncouple Pathological Response and Disease-free Survival. Clin Cancer Res 2019;25:1535-45. [Crossref] [PubMed]
- Chen Y, Zhang S, Wang Q, et al. Tumor-recruited M2 macrophages promote gastric and breast cancer metastasis via M2 macrophage-secreted CHI3L1 protein. J Hematol Oncol 2017;10:36. [Crossref] [PubMed]
- Allard B, Pommey S, Smyth MJ, et al. Targeting CD73 enhances the antitumor activity of anti-PD-1 and anti-CTLA-4 mAbs. Clin Cancer Res 2013;19:5626-35. [Crossref] [PubMed]
- Karyampudi L, Lamichhane P, Scheid AD, et al. Accumulation of memory precursor CD8 T cells in regressing tumors following combination therapy with vaccine and anti-PD-1 antibody. Cancer Res 2014;74:2974-85. [Crossref] [PubMed]
- Voorwerk L, Slagter M, Horlings HM, et al. Immune induction strategies in metastatic triple-negative breast cancer to enhance the sensitivity to PD-1 blockade: the TONIC trial. Nat Med 2019;25:920-8. [Crossref] [PubMed]
- Vranic S, Cyprian FS, Gatalica Z, et al. PD-L1 status in breast cancer: Current view and perspectives. Semin Cancer Biol 2021;72:146-54. [Crossref] [PubMed]
- Zhang L, Sullivan PS, Goodman JC, et al. MicroRNA-1258 suppresses breast cancer brain metastasis by targeting heparanase. Cancer Res 2011;71:645-54. [Crossref] [PubMed]
- Hong BS, Ryu HS, Kim N, et al. Tumor Suppressor miRNA-204-5p Regulates Growth, Metastasis, and Immune Microenvironment Remodeling in Breast Cancer. Cancer Res 2019;79:1520-34. [Crossref] [PubMed]
(English Language Editor: J. Jones)