Characterization of osteosarcoma subtypes mediated by macrophage-related genes and creation and validation of a risk score system to quantitatively assess the prognosis of osteosarcoma and reflect the tumor microenvironment
Highlight box
Key findings
• We identified 2 osteosarcoma clusters mediated by 22 macrophage score-related genes and constructed a risk score system.
What is known and what is new?
• Osteosarcoma is a highly heterogeneous cancer.
• A new prognostic risk score system based on differentially expressed genes between two heterogeneous clusters provided a quantitative assessment of prognosis and tumor microenvironment in osteosarcoma.
What is the implication, and what should change now?
• Although a risk score system composed of nine genes was created, the regulatory network between these genes and the resulting biological effects remains to be explored.
Introduction
Osteosarcoma is an advanced malignant stromal tumor composed of mesenchymal cells that produce osteoid and immature bone, accounting for 3–6% of childhood cancers and 1% of adult cancers (1,2). The combination of surgery and chemotherapy greatly has improved the prognosis of patients with osteosarcoma, and the overall survival (OS) has reached 70%. However, up to 25% of patients show evidence of metastatic disease at the time of diagnosis, of which the lung is the most common metastasis site (3). For osteosarcoma patients with metastasis or poor response to treatment, the prognosis is not satisfactory, and the OS is only 25% (4,5). No effective treatment has been developed to improve the treatment of osteosarcoma for more than 30 years (6). At present, the difficulty of designing and verifying new therapies in osteosarcoma lies in 2 levels of complexity, the most important of which is that the pathology is highly heterogeneous and there are no obvious targeted events (2,7). However, most immunotherapies have not reached the desired level of success in the treatment of osteosarcoma. Immunotherapy depends on the anti-tumor immunity of immune cells. Tumor immune microenvironment (TIME) has become a recent research hotspot, which provides a new and valuable insight for tumor heterogeneity and the mechanism of tumor progression and metastasis, as well as for improving the prognosis of patients and successful implementation of immunotherapy (8,9). The tumor microenvironment (TME) of osteosarcoma is a very complex and highly dynamic environment composed of osteocytes, stromal cells, vascular cells, and immune cells, embedded in the mineralized extracellular matrix (7,10). Tumor-associated macrophages (TAM) are the most common immune cells in the TME of osteosarcoma, accounting for more than 50% of the immune cells, and may play an important role in tumorigenesis, angiogenesis, immunosuppression, drug resistance, and metastasis (11). In OS, TAM promotes tumor growth and angiogenesis and upregulates the cancer stem cell (CSC)-like phenotype. Paracrine communication between TAM and surrounding mesenchymal stem cells (MSCs) and CSCs in TME plays a key role in supporting the stem cell niche and increasing the malignant behavior of the tumor (12,13). The highly infiltrated TAM in most malignant tumors is mainly M2-type cells, which can promote tumor metastasis. It is worth noting that in high-grade OS, TAMs are characterized by both M1 type and M2 type cells. The more M1/M2 type TAM there is, the lower the probability of metastasis and the longer the survival time of OS patients (14). Macrophages have become the central drug targets for many kinds of cancers, including osteosarcoma, and can be used in clinical practice as a tool for adjuvant cell therapy and immunotherapy (14,15). Nevertheless, the research on the role of TAM in osteosarcoma is still in its infancy, and the TAM-centered treatment strategy still has great research potential (14).
Bioinformatics contributes to the research of targeted therapies for diseases (16,17). In this study, we calculated the activity score of 28 different immune cell types, including macrophages, by single-sample gene set enrichment analysis (ssGSEA), and explored the potential use of macrophage-related genes in the Therapeutically Applicable Research to Generate Effective Treatments (TARGET) database in molecular classification, prognosis, and immunotherapy of osteosarcoma samples, and constructed a prognostic risk score system for osteosarcoma after molecular classification. This study provided insight into the heterogeneous effects of TAM on osteosarcoma TME, and highlighted a potential assessment model for risk stratification, laying the foundation for precision treatment of osteosarcoma. We present the following article in accordance with the TRIPOD reporting checklist (available at https://atm.amegroups.com/article/view/10.21037/atm-22-5613/rc).
Methods
Clinical data collection of osteosarcoma samples
The transcriptome data and corresponding clinicopathological information of osteosarcoma samples were obtained through 2 approaches. The first approach was the pediatric oncology database TARGET, of which 79 osteosarcoma samples were included in our study. The second approach was to download the transcript spectrum of 45 osteosarcoma samples by visiting the osteosarcoma data set GSE21257 obtained from the Gene Expression Omnibus (GEO) database (https://www.ncbi.nlm.nih.gov/geo/). The study was conducted in accordance with the Declaration of Helsinki (as revised in 2013).
Screening of macrophage-related genes
According to the extracted major hallmarks of 28 different immune cell types (18), the immune cell enrichment fraction of osteosarcoma samples was calculated by ssGSEA method. The correlation between the ssGSEA score of macrophages and all genes in TARGET was calculated using ‘Hmisc’ package (19), genes meeting |R| >0.6 & P<0.05 were chose as macrophage score related genes, and they were inputted into ‘clusterProfiler’ package for Gene Ontology (GO) enrichment and Kyoto Encyclopedia of Genes and Genomes (KEGG) analysis.
Consensus clustering for osteosarcoma samples
The “Hc” clustering algorithm with 500 repetitions and Spearman’s correlation was used as a distance metric for clustering were conducted by ‘ConsensusClusterPlus’ R package (The R Foundation for Statistical Computing, Vienna, Austria), and each iteration included 80% of the samples and minK =2 and maxK =9. The final K was selected according to cumulative distribution function (CDF), the change in the area under the CDF curves, and the consensus heatmap.
Infiltration of cells in immune microenvironment
We chose 3 algorithms to estimate the fraction of infiltrating cells in the immune microenvironment of osteosarcoma. ssGSEA analysis was performed using the ‘gsva’ package in R program Using the “GSVA” package, ssGSEA was conducted to infer infiltration of 28 immune microenvironment cells based on transcriptome data of osteosarcoma samples (20). Correlation analysis was performed using the ‘corrplot’ package in R. The Estimation of STromal and Immune cells in MAlignant Tumor tissues using Expression data (ESTIMATE) algorithm measured the infiltration of stromal and immune cells by calculating stromal score and immune score based on matrix and immune characteristic genes (21). The enrichment pattern of immune microenvironment cells was estimated by quantifying the fraction of leukocyte subsets using the Cell-type Identification by Estimating Relative Subsets of RNA Transcripts (CIBERSORT) tool (22).
Immunotherapy response and drug sensitivity analysis
Immune checkpoint molecules are key regulators of tumor immune response (13). To associate macrophage-associated molecular subtypes with immunotherapy, we examined differences in immune checkpoint gene expression between subtypes. The Tumor Immune Dysfunction and Exclusion (TIDE) is a predictive tool to reflect tumor response to immune checkpoint blockade (ICB) therapy (23). The signature scores of different macrophage-related subtypes were determined by TIDE. The Genomics of Drug Sensitivity in Cancer (GDSC) portal is the largest public source of information on molecular markers of drug sensitivity and drug response in cancer cells (24). The drug response data were collected from GDSC and the half-maximal inhibitory concentration (IC50) values was estimated using the ‘pRRophetic’ package in R. A lower IC50 indicated a higher sensitivity of the drug.
Construction of prognostic models based on differentially expressed genes (DEGs) between macrophage-associated subtypes
Differences between macrophage-related subtypes were analyzed by ‘limma’ package in R, with the criteria of |log2fold change (FC)| >1 as well as false discovery rate (FDR) <0.05. The obtained DEGs was filtered by univariate Cox regression analysis, and least absolute shrinkage and selection operator (LASSO) regression analysis was performed by R ‘glmnet’ package to select the most reliable genes related to the prognosis of osteosarcoma and to create a signature that combines these genes and their coefficients.
Prognostic performance assessment of risk score
The risk model was adopted to assess the risks of osteosarcoma by calculating the risk score for each osteosarcoma sample. Kaplan-Meier survival analysis and receiver operating characteristic (ROC) analysis were implemented to assess the prognostic ability of the risk score using the ‘timeROC’, ‘survival’ packages in R. Univariate and multivariate Cox regression analysis were implemented with the ‘survival’ package in R to determine whether risk score had independent predictive value for the prognosis of osteosarcoma.
Analysis of biological regulatory pathway
The h.all.v7.5.1.entrez.gmt geneset was selected in the Molecular Signatures Database (MSigDB), and GSEA was run in the TARGET and GSE21257 datasets. The cutoff criteria of |normalized enrichment score (NES)| >1.0 and nominal P<0.05 was used to identify the differences of biological regulatory pathways between different macrophage-related subtypes and between different risk groups, and the enrichment results were visualized by R packet ggplot2.
Generation and evaluation of decision tree and nomogram
Decision tree and nomogram are commonly used algorithms to optimize risk stratification. Recursive partitioning analysis was conducted using the ‘rpart’ package in R to generate a survival decision tree based on age, gender, metastasis, and risk score. A nomogram was created using the ‘rms’ package in R based on independent prognostic factors obtained by univariate and multivariate Cox regression analysis, and its survival prediction performance was judged by calibration curve, decision curve analysis (DCA), and ROC analysis.
Statistical analysis
The statistical analysis packages used in this study were all in R. Correlation analysis was conducted by Pearson correlation test. Student’s t-tests and Wilcoxon signed rank test compared the differences in continuous variables between two groups. ANOVA was used to analyze the differences in the distribution of clinical features between two groups. If not specifically mentioned, P<0.05 was considered statistically significant.
Results
Classification of osteosarcoma based on macrophage-related genes
According to the correlation analysis between macrophages score calculated by ssGSEA and gene expression profile in TARGET, 204 macrophage score-related genes were screened. GO analysis revealed that these genes were enriched in a total of 410 GO terms of biological process (BP), 60 GO terms of cellular component (CC), and 20 GO terms of molecular function (MF). The GO terms with the most significant enrichment of 204 genes were neutrophil mediated immunity, major histocompatibility complex (MHC) class II protein complex, and MHC class II protein complex binding (Figure 1A). A total of 204 macrophage score-related genes were also significantly associated with 40 KEGG pathways, most of which were involved in immune regulation, such as allograft rejection, autoimmune thyroid disease, intestinal immune network for immunoglobulin A (IgA) production, antigen processing and presentation, and other KEGG pathways (Figure 1B). A total of 22 prognostic genes were identified by univariate Cox regression analysis of 204 macrophage score-related genes. According to the calculated hazard ratio (HR) values, we determined that BNIP3 and PLCB4 were the risk genes for prognosis, and the other 20 genes were of protective significance to the prognosis (Figure 1C). These 22 genes showed a strong expression correlation (Figure 1D), indicating that there may be a potential relationship between them.
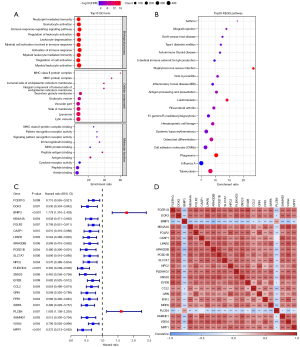
Consensus clustering of osteosarcoma samples in TARGET was performed according to the expression of 22 genes. Osteosarcoma samples from the TARGET dataset were divided into 2 clusters: cluster 1 (C1) and cluster 2 (C2) (Figure 2A-2C). Among the 22 genes, 2 risk genes for the prognosis of osteosarcoma were overexpressed in C2, 20 genes with protective significance for prognosis were overexpressed in C1, and lacking expression in C2 (Figure 2D). According to the same clustering steps, we found that the osteosarcoma samples in the GSE21257 dataset were also divided into 2 subtypes (Table S1). In TARGET, samples belonging to type C1 had more survival advantages than samples belonging to type C2 (Figure 2E). There was also this trend in the survival trend of 2 types of samples in GSE21257 dataset (Figure 2F). By analyzing the distribution of clinical characteristics of the 2 types of osteosarcoma, we statistically found that the distribution of metastatic and vital state showed significant differences between the 2 types of osteosarcoma, and C2 had a higher proportion of metastatic and dead samples (Figure 2G).
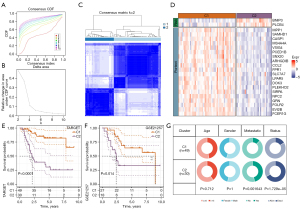
Immune microenvironment of 2 clusters of osteosarcoma
The OS and the expression patterns of 22 macrophage-related genes with prognostic significance of the 2 clusters were different. We analyzed the status of immune cells and the expression of immune molecules in the immune microenvironment of the 2 subtypes in the TARGET dataset. The ESTIMATE algorithm assessed stromal score, immune score, and ESTIMATE score, all of which had significantly higher levels in C1 than in C2 (Figure 3A). Cells involved in anti-tumor immunity, including activated CD4+ T cells and activated CD8+ T cells, central memory CD4+ T cells, central/effector memory CD8+ T cells, gamma delta cells, immature B cell, memory B cells, T follicular helper cell, type 1 T helper cells, type 17 T helper cells, dendritic cell lineage (activated dendritic cell, immature dendritic cell, and plasmacytoid dendritic cell) natural killer cells, as well as cells involved in promoting tumor immunity, including regulatory T cells, macrophages, and myeloid-derived suppressor cells (MDSCs) were found to infiltrate relative to C2 significantly more in C1 (Figure 3B). The expression of a large part of costimulatory checkpoint molecules, such as PDCD1 (PD-1), CTLA4, and CD274 (PD-L1) in C1 was significantly higher than that in C2 (Figure 3C). Therefore, C1 might be an immunological “hot” tumor, and C2 might be an immunological “cold” tumor.
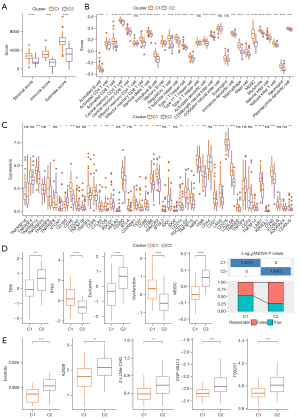
Sensitivity to immunotherapy/antitumor agents in 2 clusters
The infiltration of immune cells and the characteristics of immune molecules in TME reflect the state of immune response and affect the therapeutic effect of immunotherapy. The TIDE score of C1 and C2 samples in the TARGET dataset was evaluated by TIDE and compared by t-test. The TIDE score of C1 was significantly lower than that of C2. Moreover, compared with C2, the exclusion score and MDSC score of C1 were significantly down-regulated, while interferon gamma (IFNG) score and dysfunction score were significantly increased. The response rate of C1 to ICB therapy was significantly higher than that of C2 (Figure 3D). We also tried to evaluate the potential response of 2 osteosarcoma clusters to 5 antitumor agents. By comparing the IC50 values of C1 and C2, we observed that there was a significant difference in the estimated IC50 values of erlotinib, AZ628, Z-LLNle-CHO, CGP-60474, and TGX221 between the 2 clusters, and it was lower in C1, which indicates that C1 might be more sensitive to 5 kinds of antitumor agents (Figure 3E).
Differences in regulatory pathways between 2 osteosarcoma categories
The difference of pathway between C1 and C2 in osteosarcoma dataset was compared. The results revealed that there were significant differences in signal pathways between the 2 clusters. C1 was significantly enriched in many signaling pathways related to immune response, including interferon alpha (IFNA/IFNG) response, IL6/JAK/STAT3 signaling, allograft rejection, inflammatory response, and IL2/STAT5 signaling. Pathways significantly associated with C2 mainly regulate cell cycle progression, such as G2M checkpoint, E2F targets, MYC targets, and mitotic spindle (Figure 4A,4B). In addition, in the 2 osteosarcoma datasets, the NES of C1 vs. C2 in the above immunomodulatory pathways was >1, and the NES in the above cell cycle related signal pathways was negative, indicating that the consistency of the regulation mechanism of C1 vs. C2 in the 2 datasets (Figure 4C).
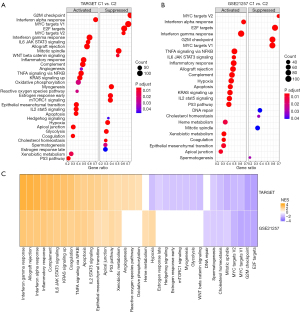
Construction and validation of prognostic risk score system
Since there were different degrees of heterogeneity between the 2 osteosarcoma subclasses based on 22 macrophage score-related genes in clinical phenotype, immune microenvironment characteristics, regulated signal pathways, and sensitivity to immunotherapy/antitumor agents, we were compelled to identify the key genes related to the osteosarcoma prognosis from the DEGs between the 2 subclasses. Difference analysis showed that there were 347 DEGs within the threshold defined by us between the 2 subtypes, of which 131 were prognostic genes of osteosarcoma (Figure 5A). LASSO Cox regression analysis was used to calculate the coefficient of each gene and select 9 genes whose coefficient was not 0 for integration into the prognostic risk score system (Figure 5B,5C). The created prognostic risk score system formula was described as each base factor multiplied by its LASSO coefficient and added. According to the prognostic risk score system, each osteosarcoma sample from the TARGET and GSE21257 datasets was given a risk score, and the risk score was standardized by z-score and further classified into high- and low-risk groups. In the TARGET dataset, the survival results obtained by Kaplan-Meier analysis were significantly different between the high-risk group and the low-risk group. Compared with the low-risk group, the OS time of the high-risk group was shorter, and the survival rate was lower (Figure 5D). The ROC curve obtained from the TARGET dataset showed that prognostic risk score system predicted the area under the curve (AUC) of the sample OS in 1–5 years, and the AUC of each year was 0.82 or more, indicating that the accuracy of prognostic risk score system in predicting the sample OS in the TARGET dataset was very high (Figure 5E). The survival results of patients in the high-risk group and the low-risk group in the GSE21257 dataset were compared, and it was also revealed that the survival results of the high-risk group were at a disadvantage compared with the low-risk group (Figure 5F). The prognostic risk score system predicted that the 3-, 4-, and 5-year AUC of the sample OS in the GSE21257 dataset were equal to or higher than 0.75, and increased year by year, and the AUC reached 0.86 in 5 years (Figure 5G). These results confirmed the potential of risk score system to accurately predict the prognosis of patients with osteosarcoma.
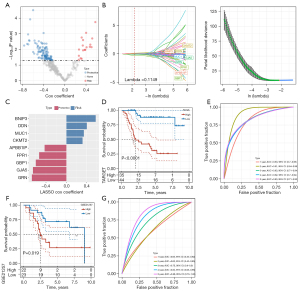
Potential effects of risk score on immune microenvironment infiltration and tumor regulatory pathways
To elucidate the biological relevance of risk score from the perspective of TME infiltration and functional regulation pathways. In the aspect of TME infiltration, the stromal score, immune score, ESTIMATE score and ssGSEA score of 28 immune cells were calculated. The performance of stromal score, immune score, and ESTIMATE score in the low-risk score group was significantly higher than that in the high-risk score group (Figure 6A). There was extensive infiltration of immune cells in low-risk score group, and the infiltration activity scores of activated B cell, activated CD8+ T cell, central memory CD4+ T cell, central memory CD8+ T cell, effector memory CD4+ T cell, effector memory CD8+ T cell, gamma delta T cell, immature B cell, regulatory T cell, T follicular helper cell, type 1 T helper cell, activated dendritic cell, macrophage, MDSC, monocyte, natural killer cell, natural killer T cell, neutrophil, and plasmacytoid dendritic cell were significantly higher than those in the high-risk score group (Figure 6B). There was a significant negative correlation between risk score and the activity score of these immune cells, indicating that the higher the risk score, the worse the immune microenvironment (Figure 6C). From the perspective of functional regulatory pathways, risk score was associated with cell metastasis and a variety of different immune-related signaling pathways, such as the JAK/STAT signaling pathway, cell adhesion molecules (CAMs), complement and coagulation cascades, antigen processing and presentation, autoimmune thyroid disease, allograft rejection, intestinal immune network foe IgA production, natural killer cell-mediated cytotoxicity, cytokine-cytokine receptor interaction, toll-like receptor signaling pathway, hematopoietic cell lineage, chemokine signaling pathway, leukocyte transendothelial migration, T cell receptor signaling pathway, and B cell receptor signaling pathway (Figure 6D). Moreover, compared with the samples with low-risk score, the NES of immune-related signaling pathways of the samples with high-risk score were all negative, indicating that the immune response of the high-risk score group was inactive or suppressed (Figure 6E). These results suggested that risk score may have a certain potential in reflecting immune infiltration and immune response.
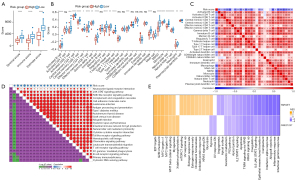
Independence analysis of prognostic risk score system and construction of prognostic decision tree and nomogram
We constructed a decision tree based on the clinical characteristics of osteosarcoma samples and risk score system. Only age, metastasis, and risk score were retained in the decision tree, which divided osteosarcoma samples into 4 subgroups (C1, C2, C3, C4) (Figure 7A). Kaplan-Meier analysis showed that there were significant differences in survival results among the 4 subgroups, in the order from long to short: C1 > C2 > C3 > C4 (Figure 7B). By analyzing the risk distribution in each subgroup, it was obvious that only low risk samples were distributed in C1 and C2, and only high-risk samples were distributed in C3 and C4 (Figure 7C). From the perspective of survival state, there was a significant difference in the proportion of survival samples among the 4 subgroups. The proportion of survival samples in C1 was the highest, and the order of survival ratio from high to low was C1 > C2 > C3 > C4 (Figure 7D).
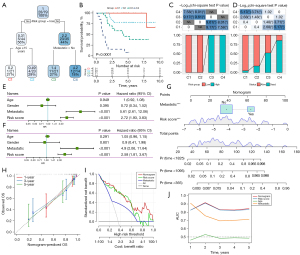
Univariate and multivariate Cox regression analyses were performed to determine the prognostic independence of risk score system and clinical features. Risk score and metastasis remained independent in predicting the prognosis of osteosarcoma (Figure 7E,7F). To provide an accurate and quantitative tool for predicting the prognosis of osteosarcoma, risk score and metastasis were incorporated into the ‘rms’ package to construct a nomogram (Figure 7G). The calibration chart showed that the nomogram was very close to the 1-, 3-, and 5-year OS performance predicted by the best prediction model (Figure 7H). The nomogram surpassed the highest net income of risk score and metastasis, and reached an AUC higher than 0.8 (Figure 7I,7J).
Discussion
Osteosarcoma is an osteoblast cell line tumor. However, osteoclasts play a crucial role in the pathogenesis of osteosarcoma. Osteoclasts are specialized tissue macrophages that occupy the bone. Monocytes, macrophages are specialized phagocytes (25). Anti-osteoclast drugs have been reported to significantly reduce patient mortality and morbidity by preventing tumor progression and local spread (26). Macrophages represent the main immune components in the microenvironment of osteosarcoma, and the therapy focused on targeting TAM has become a hot topic of immunotherapy (13). The macrophage activator agent mifamurtide targeting immune system is the latest progress in the treatment of osteosarcoma since multidrug chemotherapy. However, the use of antibodies against PD-1/PD-L1 to stimulate the immune system in osteosarcoma remains controversial. Major controversies include the toxicity of antibodies, poor CD8+ T lymphocyte infiltration, no histological evidence of PD-1/PD-L1 in most osteosarcoma samples, and no correlation with tumor outcome to date (7). In response to these disputes, we started with the identification of macrophage-related genes, divided osteosarcoma into 2 molecular categories according to 22 macrophage-related genes, and explored the infiltration of a variety of immune cells in each molecular category, including CD8+ T cells, as well as the expression of immune checkpoints including PD-1/PD-L1 and the response to ICB treatment. Among the 2 molecular subclasses of osteosarcoma, C1 had a significantly longer survival time, and several cells including activated CD8+ T cells, central memory CD8+ T cells, effector memory CD8+ T cells involved in anti-tumor immunity and several cells involved in promoting tumor immunity had significantly higher infiltration. The IFNA/IFNG response (27,28), complement (29,30), and inflammatory response (31) are crucial mechanisms contributing to the efficacy of cancer immunotherapy. Here, all of these mechanisms were active in C1. In addition, a large group of costimulatory immune checkpoint molecules, including PD-1 and PD-L1, were significantly promoted in C1 relative to C2. More importantly, C1 showed a significantly lower TIDE score than C2. This evidence suggested that immunotherapy, including PD-1/PD-L1 antibodies, can be targeted in osteosarcoma.
Although clusters with prognostic advantages and suitable for immunotherapy were identified based on clustering algorithm, an accurate method is needed to quantitatively evaluate osteosarcoma OS and reflect TME and signal transduction. Here, we identified the DEGs between 2 osteosarcoma clusters mediated by macrophage-related genes, and created a prognostic risk score system with a combination of 9 DEGs by machine learning. Some genes in the prognostic risk score system have been studied in different cancers. BNIP3 has been found to be highly expressed in many types of tumors and described as a major independent factor in OS of cancer patients (32). GRN is a growth factor and secreted glycosylated peptide, which is involved in the invasion of colorectal cancer and is a marker of survival in patients (33). DDN has been identified as underexpressed in glioblastoma and is a biomarker candidate for prognosis (34). FPR1 participates in cancer immune surveillance and is regarded as a potential immunopharmacological target for neuroblastoma (35,36). A pan-cancer study showed that APBB1IP could be used as prognostic biomarkers of pan-cancer, and the up-regulation of APBB1IP was associated with increased immune cell infiltration (37). MUC1 drives drug resistance and immune evasion and is described as the main target for the design and development of cancer vaccines (38,39). The GBP1 may be a double-edged sword in cancer environment, which can inhibit cancer cell proliferation in the environment of breast and colorectal cancer, but is hijacked by upstream tumorigenic events in ovarian cancer and glioblastoma, inducing cancer drug resistance and tumor progression (40). In our study, we considered the association between the risk score system of these genes and the prognosis, TME, and biological pathway of osteosarcoma. According to risk score system, osteosarcoma samples can obtain a risk score and be assessed for the risk of death. A higher risk score means a higher risk of death. A risk score system could also monitor TME infiltration in osteosarcoma samples and showed a close relationship with osteosarcoma biology, including metastasis and immunity.
The limitations of this study cannot be ignored. First of all, all data were downloaded from public databases, and the sample size and clinical information were limited. Second, although a risk score system consisting of 9 genes has been created, the regulatory network and biological effects between these genes remain to be explored.
Conclusions
In summary, we identified 2 macrophage-related gene-mediated clusters, which had different degrees of heterogeneity in clinical phenotype, immune microenvironment characteristics, regulated signal pathway, and sensitivity to immunotherapy/antitumor agents. Based on the DEGs between the 2 clusters, a new prognostic risk score system was created to quantitatively evaluate the OS and TME of osteosarcoma. Our research provides a new perspective for identifying cancer subtypes suitable for immunotherapy and a new entry point for the design of personalized therapy.
Acknowledgments
Funding: None.
Footnote
Reporting Checklist: The authors have completed the TRIPOD reporting checklist. Available at https://atm.amegroups.com/article/view/10.21037/atm-22-5613/rc
Conflicts of Interest: All authors have completed the ICMJE uniform disclosure form (available at https://atm.amegroups.com/article/view/10.21037/atm-22-5613/coif). The authors have no conflicts of interest to declare.
Ethical Statement: The authors are accountable for all aspects of the work in ensuring that questions related to the accuracy or integrity of any part of the work are appropriately investigated and resolved. The study was conducted in accordance with the Declaration of Helsinki (as revised in 2013).
Open Access Statement: This is an Open Access article distributed in accordance with the Creative Commons Attribution-NonCommercial-NoDerivs 4.0 International License (CC BY-NC-ND 4.0), which permits the non-commercial replication and distribution of the article with the strict proviso that no changes or edits are made and the original work is properly cited (including links to both the formal publication through the relevant DOI and the license). See: https://creativecommons.org/licenses/by-nc-nd/4.0/.
References
- Cascini C, Chiodoni C. The Immune Landscape of Osteosarcoma: Implications for Prognosis and Treatment Response. Cells 2021;10:1668. [Crossref] [PubMed]
- Moukengue B, Lallier M, Marchandet L, et al. Origin and Therapies of Osteosarcoma. Cancers (Basel) 2022;14:3503. [Crossref] [PubMed]
- Eaton BR, Schwarz R, Vatner R, et al. Osteosarcoma. Pediatr Blood Cancer 2021;68:e28352. [Crossref] [PubMed]
- Chen C, Xie L, Ren T, et al. Immunotherapy for osteosarcoma: Fundamental mechanism, rationale, and recent breakthroughs. Cancer Lett 2021;500:1-10. [Crossref] [PubMed]
- Gaspar N, Occean BV, Pacquement H, et al. Results of methotrexate-etoposide-ifosfamide based regimen (M-EI) in osteosarcoma patients included in the French OS2006/sarcome-09 study. Eur J Cancer 2018;88:57-66. [Crossref] [PubMed]
- Wedekind MF, Wagner LM, Cripe TP. Immunotherapy for osteosarcoma: Where do we go from here? Pediatr Blood Cancer 2018;65:e27227. [Crossref] [PubMed]
- Corre I, Verrecchia F, Crenn V, et al. The Osteosarcoma Microenvironment: A Complex But Targetable Ecosystem. Cells 2020;9:976. [Crossref] [PubMed]
- Zhu T, Han J, Yang L, et al. Immune Microenvironment in Osteosarcoma: Components, Therapeutic Strategies and Clinical Applications. Front Immunol 2022;13:907550. [Crossref] [PubMed]
- Liu W, Xie X, Qi Y, et al. Exploration of Immune-Related Gene Expression in Osteosarcoma and Association With Outcomes. JAMA Netw Open 2021;4:e2119132. [Crossref] [PubMed]
- Liu Y, Feng W, Dai Y, et al. Single-Cell Transcriptomics Reveals the Complexity of the Tumor Microenvironment of Treatment-Naive Osteosarcoma. Front Oncol 2021;11:709210. [Crossref] [PubMed]
- Huang Q, Liang X, Ren T, et al. The role of tumor-associated macrophages in osteosarcoma progression - therapeutic implications. Cell Oncol (Dordr) 2021;44:525-39. [Crossref] [PubMed]
- Ridge SM, Sullivan FJ, Glynn SA. Mesenchymal stem cells: key players in cancer progression. Mol Cancer 2017;16:31. [Crossref] [PubMed]
- Cersosimo F, Lonardi S, Bernardini G, et al. Tumor-Associated Macrophages in Osteosarcoma: From Mechanisms to Therapy. Int J Mol Sci 2020;21:5207. [Crossref] [PubMed]
- Zhao Y, Zhang B, Zhang Q, et al. Tumor-associated macrophages in osteosarcoma. J Zhejiang Univ Sci B 2021;22:885-92. [Crossref] [PubMed]
- Ngambenjawong C, Gustafson HH, Pun SH. Progress in tumor-associated macrophage (TAM)-targeted therapeutics. Adv Drug Deliv Rev 2017;114:206-21. [Crossref] [PubMed]
- Ning W, Li S, Tsering J, et al. Protective Effect of Triphala against Oxidative Stress-Induced Neurotoxicity. Biomed Res Int 2021;2021:6674988. [Crossref] [PubMed]
- Ning W, Jiang X, Sun Z, et al. Identification of the potential biomarkers involved in the human oral mucosal wound healing: a bioinformatic study. BioMed Research International 2021;2021:6695245.
- Bindea G, Mlecnik B, Tosolini M, et al. Spatiotemporal dynamics of intratumoral immune cells reveal the immune landscape in human cancer. Immunity 2013;39:782-95. [Crossref] [PubMed]
- Ma X, Zhang L, Huang D, et al. Quantitative radiomic biomarkers for discrimination between neuromyelitis optica spectrum disorder and multiple sclerosis. J Magn Reson Imaging 2019;49:1113-21. [Crossref] [PubMed]
- Hänzelmann S, Castelo R, Guinney J. GSVA: gene set variation analysis for microarray and RNA-seq data. BMC Bioinformatics 2013;14:7. [Crossref] [PubMed]
- Yoshihara K, Shahmoradgoli M, Martínez E, et al. Inferring tumour purity and stromal and immune cell admixture from expression data. Nat Commun 2013;4:2612. [Crossref] [PubMed]
- Gentles AJ, Newman AM, Liu CL, et al. The prognostic landscape of genes and infiltrating immune cells across human cancers. Nat Med 2015;21:938-45. [Crossref] [PubMed]
- Jiang P, Gu S, Pan D, et al. Signatures of T cell dysfunction and exclusion predict cancer immunotherapy response. Nat Med 2018;24:1550-8. [Crossref] [PubMed]
- Yang W, Soares J, Greninger P, et al. Genomics of Drug Sensitivity in Cancer (GDSC): a resource for therapeutic biomarker discovery in cancer cells. Nucleic Acids Res 2013;41:D955-61. [Crossref] [PubMed]
- Kelleher FC, O'Sullivan H. Monocytes, Macrophages, and Osteoclasts in Osteosarcoma. J Adolesc Young Adult Oncol 2017;6:396-405. [Crossref] [PubMed]
- Behzatoglu K. Osteoclasts in Tumor Biology: Metastasis and Epithelial-Mesenchymal-Myeloid Transition. Pathol Oncol Res 2021;27:609472. [Crossref] [PubMed]
- Critchley-Thorne RJ, Simons DL, Yan N, et al. Impaired interferon signaling is a common immune defect in human cancer. Proc Natl Acad Sci U S A 2009;106:9010-5. [Crossref] [PubMed]
- Du W, Frankel TL, Green M, et al. IFNγ signaling integrity in colorectal cancer immunity and immunotherapy. Cell Mol Immunol 2022;19:23-32. [Crossref] [PubMed]
- Kolev M, Markiewski MM. Targeting complement-mediated immunoregulation for cancer immunotherapy. Semin Immunol 2018;37:85-97. [Crossref] [PubMed]
- Kolev M, Towner L, Donev R. Complement in cancer and cancer immunotherapy. Arch Immunol Ther Exp (Warsz) 2011;59:407-19. [Crossref] [PubMed]
- Chen M, Linstra R, van Vugt MATM. Genomic instability, inflammatory signaling and response to cancer immunotherapy. Biochim Biophys Acta Rev Cancer 2022;1877:188661. [Crossref] [PubMed]
- Gorbunova AS, Yapryntseva MA, Denisenko TV, et al. BNIP3 in Lung Cancer: To Kill or Rescue? Cancers (Basel) 2020;12:3390. [Crossref] [PubMed]
- Klupp F, Kahlert C, Franz C, et al. Granulin: An Invasive and Survival-Determining Marker in Colorectal Cancer Patients. Int J Mol Sci 2021;22:6436. [Crossref] [PubMed]
- Li Q, Aishwarya S, Li JP, et al. Gene Expression Profiling of Glioblastoma to Recognize Potential Biomarker Candidates. Front Genet 2022;13:832742. [Crossref] [PubMed]
- Vacchelli E, Le Naour J, Kroemer G. The ambiguous role of FPR1 in immunity and inflammation. Oncoimmunology 2020;9:1760061. [Crossref] [PubMed]
- Liu M, Zhao J, Chen K, et al. G protein-coupled receptor FPR1 as a pharmacologic target in inflammation and human glioblastoma. Int Immunopharmacol 2012;14:283-8. [Crossref] [PubMed]
- Ge Q, Li G, Chen J, et al. Immunological Role and Prognostic Value of APBB1IP in Pan-Cancer Analysis. J Cancer 2021;12:595-610. [Crossref] [PubMed]
- Kufe DW. MUC1-C in chronic inflammation and carcinogenesis; emergence as a target for cancer treatment. Carcinogenesis 2020;41:1173-83. [Crossref] [PubMed]
- Gao T, Cen Q, Lei H. A review on development of MUC1-based cancer vaccine. Biomed Pharmacother 2020;132:110888. [Crossref] [PubMed]
- Honkala AT, Tailor D, Malhotra SV. Guanylate-Binding Protein 1: An Emerging Target in Inflammation and Cancer. Front Immunol 2019;10:3139. [Crossref] [PubMed]
(English Language Editor: J. Jones)