A narrative review of progress in the application of artificial intelligence in acute respiratory distress syndrome: subtypes and predictive models
Introduction
A large number of basic and clinical studies in the past 50 years have clarified the pathophysiological mechanisms of acute respiratory distress syndrome (ARDS) and proposed new treatments, yet ARDS remains a very common respiratory syndrome in the intensive care unit (ICU) (1). Recently, two large-scale multicenter investigations found that the mortality of patients with ARDS in ICU is as high as 40% (2,3), and complications of varying degrees occur in at least 50% of patients with ARDS (3). This often leads to prolonged hospitalization and increases treatment costs, which then increases the family and social burden.
Big data are immensely large datasets that are difficult to acquire, store, manage, and analyze using traditional database software tools. These are often characterized by a massive data scale, rapid data flow, diverse data types, and low value density. Big data in healthcare consist of a patient’s demographics, laboratory results, waveforms, and imaging findings. These data are all combined to form a data archive (4). Big data analysis in medical treatment should be based on multiple disciplines, such as statistics, bioinformatics, epidemiology, and artificial intelligence (AI), aiming at improving patient care in terms of outcome prediction, diagnosis, and risk classification (5).
Machine learning is a science of AI that uses data or experience to improve the performance of specific algorithms in learning. Machine learning has been widely used in the medical field, mainly for supervised prediction and unsupervised subgroup classification (6). In some cases, compared with traditional methods, AI-supervised methods to construct ARDS prediction models is more accurate and convenient and can predict the diagnosis, survival, and complications of ARDS at an early stage. AI-supervised methods also support clinical decision-making and achieve early prevention, control, and treatment. Because of the heterogeneity and various etiologies of ARDS and pathological changes (7), unsupervised clustering is required to classify patients with ARDS and distinguish different subgroups of clinical manifestations and treatment responses for disease management.
This narrative review aimed to (I) understand the role of AI in improving the management of patients with ARDS; (II) explore the subgroup classification of patients with ARDS via unsupervised clustering; (III) evaluate the use of supervised predictive models for the early detection of ARDS; (IV) focus on research gaps in ARDS prediction; and (V) identify the current problems that could be solved by applying AI in ARDS. We present the following article in accordance with the Narrative Review reporting checklist (available at https://atm.amegroups.com/article/view/10.21037/atm-22-3153/rc).
Methods
This review focused on the application and development of AI for ARDS from December 1, 2011, to August 5, 2022, during which the medical application of AI had rapidly developed. To identify eligible studies and reference lists of primary articles found from initial searches, we further searched the PubMed, Web of Science, Embase, and Medline databases using the following index terms: ((ARDS) AND (machine learning) OR (artificial intelligence)) AND ((diagnosis) OR (prognosis) OR (complications) OR (phenotype)). To take advantage of the breadth and boundaries of interpretations, we included literature from different countries where AI was used to predict the diagnosis or prognosis of ARDS based on the Berlin criteria as well as articles on ARDS subphenotypes. Some studies (8-10) suggested that coronavirus disease 2019 (COVID-19)-related ARDS and typical ARDS differ significantly in multiple ways, such as the injury site, clinical features, time of onset, respiratory system compliance, and management protocols. Articles on COVID-19-related ARDS were excluded. The inclusion criteria were as follows: accessibility of full texts, use of AI and ARDS data in subtype or prediction models, formal publication types, and English language. The exclusion criteria were as follows: articles on COVID-19-related ARDS, duplicate records, no adults in the study population, and review articles. The study selection process is summarized in Table 1.
Table 1
Items | Specification |
---|---|
Date of search | June 8, 2022, to August 5, 2022 |
Databases and other sources searched | PubMed, Web of Science, Embase, and Medline |
Search terms used | Keywords: artificial intelligence, ARDS, machine learning, phenotype, prognosis, diagnosis, and complications |
Timeframe | December 1, 2011, to August 5, 2022 |
Inclusion criteria | Accessibility of full texts, use of AI and ARDS data in subtype or prediction models, formal publication types, and English language |
Exclusion criteria | Articles on COVID-19-related ARDS, duplicate records, no adults in the study population, and review articles |
Selection process | Study selection was performed by Yu Bai, Xu Huang, and Jingen Xia independently. Any disagreements about the inclusion of studies were resolved by discussion |
COVID-19, coronavirus disease 2019; ARDS, acute respiratory distress syndrome; AI, artificial intelligence.
Discussion
Four online research databases were searched: PubMed, Web of Science, Embase, and Medline. On initial screening, the following keywords were used: respiratory distress syndrome, machine learning, artificial intelligence, diagnosis, prognosis, and complications. A total of 1,074 articles were found. Of these, 116 duplicate articles and 91 articles with inaccessible full texts were excluded. Of the remaining 867 articles, 10 articles related to ARDS subtypes and 5 articles related to diagnosis and survival prediction were selected after applying the inclusion and exclusion criteria (Figure 1).
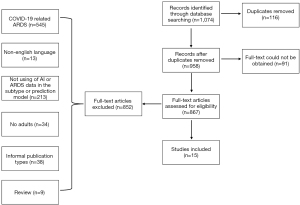
We identified two broad categories of AI applications in ARDS. One category was the use of unsupervised clustering to classify ARDS into different subtypes (Table 2), including the use of clinical indicators combined with biomarkers to classify ARDS as well as the comparison of the responses of different groups to treatments such as positive end-expiratory pressure (PEEP) management, fluid therapy, and statins. The other category included the development of clinical prediction models for diagnosis and survival (Table 3). Complex clinical data were input into the model to obtain more accurate prediction results to guide doctors in their clinical decision-making in early disease stage and reduce the incidence and mortality of ARDS.
Table 2
Author(s) | Year | Method(s) | Dataset(s) | Sample size | Conclusion(s) of study |
---|---|---|---|---|---|
Calfee et al. (11) | 2014 | LCA | ARMA and ALVEOLI (retrospective) | 1,022 | Hyperinflammatory and hypoinflammatory phenotypes were found, and they had different responses to the PEEP strategy |
Famous et al. (12) | 2017 | LCA | FACTT (retrospective) | 1,000 | A two-class subphenotypic model best described the study population; the fluid conservation strategy of the hyperinflammatory phenotype was more conducive to reducing mortality |
Calfee et al. (13) | 2018 | LCA | HARP-2 (retrospective) | 539 | The subphenotypes had features consistent with those previously reported; the hyperinflammatory subphenotype showed improved survival with simvastatin |
Sinha et al. (14) | 2018 | LCA | SAILS (retrospective) | 745 | The subphenotypes had features consistent with those previously reported. No treatment effect was observed with rosuvastatin |
Sinha et al. (15) | 2020 | Random forest, Bootstrapped aggregating, Least absolute shrinkage and selection operator, and nested logistic regression models | ARMA, ALVEOLI, FACTT, and SAILS (retrospective) | 2,737 | Hyper- and hypoinflammatory phenotypes can be accurately identified using a simple classifier model comprising three or four variables |
Sinha et al. (16) | 2020 | Gradient boosted machine algorithm | ARMA, ALVEOLI, FACTT, and SAILS (retrospective) | 2,737 | ARDS phenotype can be accurately identified using a machine learning model based on clinical data |
Maddali et al. (17) | 2022 | Gradient boosted machine algorithm, XGBoost: Extreme Gradient Boosting | VALID, EARLI, and LUNG SAFE (retrospective) | 3,834 | A classifier model using clinical variables alone can accurately assign ARDS subphenotypes in the observation cohort and provide individualized information on PEEP treatment strategies |
Sinha et al. (18) | 2022 | LCA | VALID and EARLI (prospective) | 959 | The previous hyperinflammatory and hypoinflammatory subtypes can be extended to unselected populations of nontraumatic ARDS |
Hashem et al. (19) | 2022 | Wilcoxon rank-sum and Fisher’s exact tests | SAILS (retrospective) | 568 | Inflammatory subphenotypes largely reflect the acute phase of illness and its short-term impact |
Liu et al. (20) | 2021 | K-means | eICU, ALVEOLI, FACTT, and SAILS (retrospective) | 3,675 | Three clinical phenotypes of ARDS were identified and they had different clinical characteristics and outcomes |
ARDS, acute respiratory distress syndrome; LCA, latent class analysis; ARMA, the NHLBI ARDS network’s randomized controlled trials of lower versus higher tidal volume ventilation trial; ALVEOLI, higher versus lower positive end-expiratory pressure trial; PEEP, positive end-expiratory pressure; FACTT, Fluids And Catheters Treatment Trial; HARP-2, a UK multicenter, placebo-controlled randomized trial of simvastatin for ARDS; SAILS, contemporary NHLBI network trial of infection-associated ARDS; VALID, Validating Acute Lung Injury biomarkers for diagnosis; EARLI, Early Assessment of Renal and Lung Injury; eICU, Tele-Health intensive care unit.
Table 3
Author(s) | Year | Method(s) | Dataset(s) | Sample size | Conclusion of study |
---|---|---|---|---|---|
Ding et al. (21) | 2019 | Random forest | Five different centers in Beijing (retrospective) | 296 | The machine learning‑based model showed good predictive ability in Chinese patients with ARDS |
Zeiberg et al. (22) | 2019 | L2 logical regression | Single center (retrospective) | 1,621 | Developed an ARDS prediction model based on EHR data with good discriminative performance |
Brown et al. (23) | 2011 | Classification tree | ARDS network trials (retrospective) | 2,022 | The tree-based classification rule performed similarly to APACHE III in terms of stratifying patients according to hospital mortality |
Huang et al. (24) | 2021 | Random forest | MIMIC-III and eICU databases (retrospective) | 2,235 | The ARDS mortality prediction model was superior to existing traditional ARDS scores |
Zhang (25) | 2019 | Neural network | Forty-four hospitals (retrospective) | 1,071 | The ARDS mortality prediction model was superior to existing traditional ARDS scores |
ARDS, acute respiratory distress syndrome; EHR, electronic health record; APACHE: Acute Physiology and Chronic Health Evaluation; MIMIC, Medical Information Mart for Intensive Care; eICU, Tele-Health intensive care unit.
Application of unsupervised clustering
Biological subphenotype
The initial biological subphenotype was implemented by Calfee et al. (11) in 2014. A latent class analysis (LCA) was performed using data from two ARDS randomized controlled trials (RCTs) comprising 1,022 patients with ARDS [the National Heart, Lung, and Blood Institute (NHLBI) ARDS network RCT of lower versus higher tidal volume ventilation (ARMA trial) and higher versus lower PEEP in patients with ARDS (ALVEOLI trial)]. In total, 27 clinical variables and 8 plasma biomarkers were included in the LCA for clustering. Finally, an optimal two-class model was determined, and the patients with ARDS were divided into groups of phenotypes 1 and 2; one-third of the patients were assigned to phenotype 2. In phenotype 1, the mortality of the high PEEP strategy was higher than that of the low PEEP strategy. In phenotype 2, the plasma concentrations of interleukin-6 (IL-6), IL-8, soluble tumor necrosis factor receptor-1 (TNFR-1), and plasminogen activator inhibitor-1 were higher than those in phenotype 1, and it demonstrated a higher heart rate and total minute ventilation and lower systolic blood pressure, bicarbonate level, and protein C concentration. Phenotype 2 was also categorized by more severe inflammation, shock, metabolic acidosis, and worse clinical outcomes, and the mortality of the high PEEP group was lower than that of the low PEEP group. The study by Calfee et al. (11) pioneered the use of cluster analysis for categorizing ARDS and represents the beginning of personalized treatment for ARDS, which is more conducive to the individual management of patients with ARDS. ARDS phenotypes (endotypes) represent patient subsets of ARDS defined either by a biologically restricted molecular pathway/mechanism or by differences in treatment response or, rarely, both (26). LCA is used to identify queue clustering by testing the hypothesis that two or more unobserved classes (latent classes) could elucidate the relationship between observed variables in a queue. The main goal of LCA is to identify the most concise set of predictive variables and potential classes for interpreting cohort data. All data points are condition-independent and are generated from one of these unobserved categories. Thus, LCA can be used to identify ARDS subgroups (27-30). In this study, although the 27 clinical variables and 8 plasma biomarkers were not readily available simultaneously and the variables were numerous, their classification can be used as reference for bedside ventilator management (31). However, prospective studies with large samples should further evaluate more precise results.
In 2017, the same team verified the subphenotype of ARDS in the Fluids and Catheters Treatment Trial (FACTT) with a dataset of 1,000 people and assessed whether the subphenotype responded differently to fluid management (12). The authors used LCA to analyze the baseline clinical data and plasma biomarkers and logistic regression to test for an interaction between subphenotype and treatment to determine mortality rates. Results confirmed that a two-class subphenotypic model best described the study population, with phenotype 2 again characterized by higher inflammatory biomarker levels and hypotension. Moreover, a more simplified model, comprising IL-8, bicarbonate, and TNFR-1, accurately classified the subphenotypes. Regarding fluid treatment, the mortality after the fluid-conservative and fluid-liberal strategies in phenotype 1 were 26% and 18%, respectively, whereas those in phenotype 2 were 40% and 50%, respectively. In that study, the authors simplified the classification model to accurately classify patients with ARDS using only three indicators. Results demonstrated that fluid conservation in phenotype 2 was more conducive to reducing mortality, thus validating a significant interaction between hyperinflammatory and hypoinflammatory subgroups and fluid treatment.
In 2018, the same team conducted subphenotypic identification in 539 patients with ARDS in a multicenter, placebo-controlled, randomized trial of simvastatin for ARDS (HARP-2) in the United Kingdom and investigated whether different subgroups responded differently to simvastatin treatment (13). The authors used LCA and found that 65% of patients had the hypoinflammatory subphenotype, whereas 35% of patients had the hyperinflammatory subphenotype. The clinical and biological characteristics of these two subphenotypes were similar to those reported in previous studies (11,12). No difference was noted in the 28-day survival between the placebo and simvastatin groups in HARP-2, but there was a significant difference in survival between different treatments in different subphenotypic groups (P<0.00001). In the hyperinflammatory subtype group, patients taking simvastatin had a significantly higher 28-day survival than those taking placebo (P=0008); a similar pattern was observed at 90-day survival. In the same year, the team used LCA again to identify subtypes in 745 patients with ARDS in a contemporary NHLBI network trial of infection-associated ARDS (SAILS) dataset. Different therapeutic responses of rosuvastatin in subtypes were examined (14). The characteristics of the subphenotype were consistent with those previously reported (11) in four other cohorts, with approximately 40% of the patients classified to have hyperinflammatory subphenotypes. There was no significant difference in efficacy between patients with hyperinflammatory subphenotypes who were randomized to rosuvastatin treatment and those who received the placebo. In both studies, the hyper- and hypoinflammatory ARDS subphenotypes were verified via analysis of simvastatin and rosuvastatin treatment response between two groups of patients. For simvastatin, the 28-day survival rate was higher in the hyperinflammatory group than in the placebo group, whereas for rosuvastatin, there was no difference in the curative effect between groups. The therapeutic response to statins should be investigated further.
To simplify the model, the team used the datasets from RCTs (ARMA, ALVEOLI, and FACTT) as the machine learning and logistic regression model test set (n=2,022), whereas the fourth RCT dataset (SAILS; n=715) was the internal validation set (15). To select the six most important features as classification variables, the LCA-derived subtype was used as a reference, employing machine learning algorithms such as random forest, bootstrapped aggregating, and least absolute shrinkage and selection operator. Nested logistic regression models were then developed. The 28-, 60-, and 90-day mortality and 28-day no-machine ventilation time were assessed in the external validation set [START (START was a phase 2a trial that tested the safety of intravenous human bone marrow-derived mesenchymal stromal cells for moderate to severe ARDS) and HARP2]. The six most important classification variables were IL8, IL 6, protein C, soluble TNFR-1, bicarbonate, and vasopressor use. In the nested logistic regression model, three-variable (IL8, bicarbonate, and protein C) and fourvariable (the aforementioned three variables plus vasopressor use) models performed the best, with accuracies of 94% and 95%, respectively. In the external validation datasets using the three-variable models developed in the derivation dataset, two phenotypes were identified, with distinct clinical features and outcomes consistent with previous findings (11), including differential survivals with simvastatin versus placebo in HARP2 (P=0.023 for survival at 28 days). Thus, phenotypes can be accurately identified using a simple classifier model with three or four variables. This study used larger datasets and machine learning algorithms to screen the most important variables and build the model and then constructed a grouping model with three and four variables, which could accurately identify the phenotype of patients with ARDS. However, although the model is already simplified, it still includes plasma biomarkers, which cannot be quickly obtained at the bedside.
Clinical data subphenotype
Sinha et al. (16) noted that although identifying the ARDS phenotype via plasma biomarkers is a key component, the current lack of their immediate detection is an obstacle to phenotypic clinical implementation. Thus, clinical data must be used to quickly identify phenotypes at the bedside. In the study by Sinha et al. (16), three RCT cohorts were considered as training datasets (ARMA, ALVEOLI, and FACTT; n=2,022) and the fourth as a validation dataset (SAILS; n=745). A classification model was developed using the gradient boosted machine algorithm, which included 24 clinical variables (demographic, vital signs, and laboratory and respiratory variables). In the secondary analysis, the ALVEOLI and FACTT queues were used as verification datasets, and the remaining combined queues constituted the training dataset for each analysis. The performance of the phenotypic model derived from the LCA was thereafter evaluated. In the main analysis, the model accurately classified the phenotypes [area under the curve (AUC) =0.95; 95% confidence interval (CI): 0.94–0.96] in the verification queue. When ALVEOLI (AUC =0.94; 95% CI: 0.92–0.96) and FACTT (AUC =0.94; 95% CI: 0.92–0.95) were used as verification queues, the accuracy of the model was similar, indicating that the ARDS phenotype can be accurately identified using a machine learning model based on off-the-shelf clinical data and can thus can be quickly identified at the bedside. Maddali et al. (17) validated the ARDS subphenotypes in two ARDS observation cohorts using clinical classifier models with readily available clinical variables in 2022. The primary model included only vital signs and laboratory variables, whereas ventilatory variables and demography were added to the secondary model. They also assessed the performance of the primary model in the Early Assessment of Renal and Lung Injury (EARLI) trial using data that were automatically extracted from an electronic health record. Based on their findings, Maddali et al. (17) reported that a classifier model using clinical variables alone could also be used in the ARDS observation cohort to assign ARDS subphenotypes at the bedside. Furthermore, results of the Lung Safe study showed that the mortality rate was lower with high PEEP than with low PEEP in the hyperinflammatory subphenotype group (54% vs. 62%) and it was lower with low PEEP than with high PEEP in the hypoinflammatory subphenotype group (32% vs. 34%).
Phenotypic prospective verification
As noted earlier, Sinha et al. (16) used retrospective data to classify patients with ARDS into two subtypes: hyperinflammatory and hypoinflammatory. Considering the adaptability in the prospective population, they also performed LCA in 2021 using two prospective observation cohorts of patients with ARDS from the Validating Acute Lung Injury biomarkers for diagnosis (VALID) (n=624) and EARLI (n=335) studies (18). Clinical and biological data were used as classification definition variables. To test for consistency with the previous ARDS subtypes (11), the performance metrics of parsimonious classifier models of the previously developed models (IL-8, bicarbonate, protein C, and vasopressor use) were evaluated in EARLI, with the subtypes derived from LCA as the gold standard. The new classification in the two prospective cohorts were consistent with the previously described hyperinflammatory and hypoinflammatory subtypes (AUC =0.92–0.94). Additionally, new biomarkers were found; in the hyperinflammatory subtypes, the levels of matrix metalloproteinase-8 and markers of endothelial injury were significantly increased, while the level of matrix metalloproteinase-9 was significantly decreased. This suggests that the previous hyper- and hypoinflammatory subtypes could be extended to unselected populations of nontraumatic ARDS.
Phenotype controversy
Hashem et al. (19) aimed to determine if there were significant clinical differences in the physical, mental health, or cognitive outcomes between patients with hyper- and hypoinflammatory subtypes of sepsis-related ARDS at 6 and 12 months. Although previous studies (11-13) suggested that short-term mortality was significantly lower in the hypoinflammatory subtype group than in the hyperinflammatory subtype group, Hashem et al. (19) found no significant difference in the survival rates above 90 days and no consistent significance in differences in the physical, cognitive, and mental health outcomes between 6 months and 12 months. Therefore, this inflammatory subtype may be of greatest value to trials that focus on short-term mortality and related outcome measurements, rather than on long-term functional outcomes.
Other subphenotypes
In 2021, a study in China used K-means clustering to divide patients with ARDS into three subgroups, departing from the previous classic hyper- and hypoinflammatory subtypes (20). While a previous study (11) of hyper- and hypoinflammatory subtypes used plasma biomarkers as classification variables that could not be quickly obtained at the bedside, the study in China used 21 clinical variables to cluster 5,959 patients with ARDS in the Tele-Health ICU (eICU) database and the ALVEOLI, FACTT, and SAILS datasets. Of the three identified phenotypes, phenotype I (n=1,565; 40%) was associated with fewer laboratory abnormalities, less organ dysfunction, and the lowest hospital mortality (8%). Meanwhile, phenotype II (n=1,032; 32%) was associated with more inflammation and shock, with a higher mortality rate (18%). Phenotype III (n=1,078; 28%) was closely related to renal insufficiency and acidosis and had the highest mortality (22%). In phenotype I, the 60-day mortality of the high PEEP group was higher than that of the low PEEP group, and the ventilator- and ICU-free days were less. The mortality of patients with phenotype II was 22% in the fluid-conservative group and 32% in the fluid-liberal group; meanwhile, in patients with phenotype III, the mortality was 45% in the fluid-conservative group and 36% in the fluid-liberal group. These results were also validated in the three RCT datasets. This triple classification also improves the understanding of ARDS heterogeneity, but it requires verification in prospective studies.
Application of supervised prediction models
Diagnostic model
Ding et al. (21) used a random forest model to predict the risk of ARDS (296 patients, 5 different centers in Beijing). Among the 42 variables (including baseline characteristics and clinical and laboratory parameters) measured on the first day of admission, the minimum hematocrit, glucose, and sodium levels were decreased and the minimum white blood cell count was increased, which could effectively predict the occurrence of ARDS. The model yielded an AUC of 0.82 and a predictive accuracy of 83%. Zeiberg et al. (22) identified 10 predictive (highest weight) and protective (lowest weight) features using clinical data of 1621 patients with moderate hypoxia from a single center, and these characteristics were entered into the L2 logical regression model to establish a diagnostic model for patients with ARDS. The performance of the model in the test set (AUC =0.81, 95% CI: 0.73–0.88) was better than that of the XGBoost model (AUC =0.75, 95% CI: 0.68–0.81).
The studies of Ding et al. (21) and Zeiberg et al. (22) differed in some aspects. Zeiberg et al. (22) included hospitalized patients with hypoxia and non-ICU patients, and the efficacy of the logical regression was better than that of the more complex machine learning model XGBoost, probably due to a low incidence of ARDS (2–3%), resulting in a positive predictive value of only 9%. The AUC values of the models in both studies are not particularly satisfactory, and both used retrospective data without external verification. Therefore, larger prospective studies and external validation are needed to further explore diagnostic models for ARDS.
Survival prognosis model
In 2011, Brown et al. (23) established a survival prediction model for 2022 patients with ARDS from the ARDS Network Trials. Using the classification tree, they identified age (>63 years), blood urea nitrogen (>15 mg/dL), shock, respiratory rate (>21 breaths/min), and minute ventilation (>13.9 L/min) as important predictors of hospital mortality at 90 days. The classification tree showed a similar expected prediction error (28% vs. 26%; P=0.18) and AUC (0.71 vs. 0.73; P=0.71) in the training and validation sets as noted in a model based on Acute Physiology and Chronic Health Evaluation III (APACHE III). Huang et al. (24) established a prediction model of in-hospital, 30-day, and 1-year mortality rates based on the random forest algorithm for 2,235 patients with ARDS in the Medical Information Mart for Intensive Care (MIMIC-III) database, and they verified the model in the eICU database. The AUCs of the random forest model for predicting in-hospital mortality in the MIMIC-III and eICU datasets were 0.905 and 0.736, respectively, which were superior to those of existing traditional scores of ARDS, including the Simplified Acute Physiology Score II (SAPS-II) and Sepsis-Related Organ Failure Assessment scores. Platelet count and lactate level were the strongest predictors of in-hospital mortality. Zhang (25) developed a model based on data of 1,071 patients with ARDS from 44 hospitals to predict the mortality of patients with ARDS. Seven important variables were identified in the model: age, AIDS, leukemia, metastatic tumor, hepatic failure, lowest albumin, and FiO2. A representative neural network model was constructed using forward selection. The AUC of the neural network model evaluated with the validation cohort was 0.821 (95% CI: 0.753–0.888), which was significantly greater than that of the APACHE III score (0.665; 95% CI: 0.590–0.739; P=0.002) but non-significantly greater than that of the logistic regression model (0.743; 95% CI: 0.669–0.817, P=0.130).
The abovementioned models were developed using clinical data that are easily available in clinical practice, making them convenient and quick to use. While Brown et al. (23) created a survival prognosis model, a complex machine learning model was not used, but prognosis was predicted using a relatively simple classification tree, with a moderate AUC of 0.7. Huang et al. (24) used the MIMIC-III and eICU databases and developed a random forest model to predict ARDS mortality, but some variables could not be directly obtained from the two public databases. Zhang et al. (25) also developed a neural network model to predict mortality in patients with ARDS, but it did not undergo external validation and the AUC values indicated that the models had moderate predictive ability. All prediction models discussed so far were constructed using retrospective data and may require further prospective external validation to confirm their performance.
Risk of bias (ROB) and applicability
We used the PROBAST criteria to assess the ROB in the prediction model (Table 4). To assess the intensity of ROB, all models in the predictor domain were rated as low bias. One study in the participant domain was rated as highly biased, while another study was unable to identify bias in outcomes. The ROB in the analysis domain is generally high, and the high ROB usually originates from project 4.3, which evaluated whether all enrolled participants were included in the study. Moreover, 4.5 evaluated whether the selection of predictors based on univariable analysis was avoided. In terms of model applicability, all models had good overall applicability, and the consistency of study participants, predictors, and outcomes with the review questions was high.
Table 4
Study | ROB | Applicability | Overall | ||||||||
---|---|---|---|---|---|---|---|---|---|---|---|
Participants | Predictors | Outcomes | Analysis | Participants | Predictors | Outcome | ROB | Applicability | |||
Ding et al. (21) | + | + | + | − | + | + | + | − | + | ||
Zeiberg et al. (22) | + | + | + | − | + | + | + | − | + | ||
Brown et al. (23) | + | + | ? | − | + | + | + | − | + | ||
Huang et al. (24) | − | + | + | − | + | + | + | − | + | ||
Zhang (25) | + | + | + | − | + | + | + | − | + |
+ indicates low ROB/low concern regarding applicability; − indicates high ROB/high concern regarding applicability; ? indicates unclear ROB/unclear concern regarding applicability. ROB, risk of bias.
Prospects for the future
In recent years, the number of studies using AI for medical treatment has rapidly increased. The emergence and application of AI resulted in notable developments in medical treatment, which have been helpful in clinical decision-making; however, there are also some limitations. Van de Sande et al. (32) reviewed the maturity of AI in the current ICU setting, the research methods employed in published studies, and the ROB in these studies. They found that 476 (96.4%) studies were retrospective, 8 (1.6%) were prospective, and 10 (2%) used a clinical design. Of the 10 studies that used a clinical design, 5 (1%) were non-RCTs and 5 (1%) were RCTs. The most common research objective was predicting complications [110 studies (22.2%)] and mortality [102 studies (20.6%)], followed by improving prognostic models/risk scoring systems [91 studies (18.4%)] and classification subgroups [57 studies (11.7%)]. The median sample size for all retrospective studies was 1,010 (median sample size of 968 for internally validated studies and 1,528 for external validation). In addition, the median sample size of all prospective observation and clinical studies was much smaller than that of retrospective studies. Data from more than 100,000 patients were analyzed in 10 studies (2%). Among retrospective studies, 142 (28.7%) studies analyzed data of 100–1,000 patients. For the preparation level for AI in the study, the technical preparation levels introduced by the National Aeronautics and Space Administration was used: problem identification (level 1), proposal of solution (level 2), model prototyping and development (levels 3 and 4), model validation (level 5), real-time testing (level 6), workflow integration (level 7), clinical testing (level 8), and integration in clinical practice (level 9). Van de Sande et al. (32) found that 441 studies (89.3%) scored ≤4 on the readiness scale, 35 studies (7.1%) were externally validated (level 5), and 10 studies (2%) clinically evaluated the model performance (level 8). However, none was integrated into clinical practice (level 9), indicating the need for studies to be introduced into clinical practice and not remain in the clinical evaluation stage. Finally, the ROB in all 467 retrospective studies was assessed using the PROBAST criteria, revealing that 378 (80.9%) of the 467 studies had a high ROB, which most often arises from the “participants section” (item 1.1: whether inappropriate data sources are used) and the “analysis section” (item 4.1: whether sufficient patients are included and item 4.3: whether all participants included are involved in the analysis). These items led to the risk of high deviation.
Therefore, in the future, we can consider introducing machine learning into prospective studies and bedside testing of ARDS. High-quality models should be selected to provide useful tools for clinical risk screening. Although a high ROB has been noted in some models, this does not negate their predictive value. Therefore, the medical staff should comprehensively consider the predictive performance of the model, availability of predictors, convenience of outcome measurement, and applicable object of the model to select an appropriate model. Additionally, we have some considerations: First, support for these ARDS subgroups or predictive models is currently limited to a few specific populations. Therefore, it would be useful to replicate and validate these findings in ARDS populations from other international RCTs or in prospective studies with other large samples. Whether these ARDS subgroups and predictive models can be identified in unselected observational cohorts should also be clarified. Second, determining whether the subgroup allocation and prediction model of ARDS changes over time is essential, as this may affect the timing of interventions. Finally, there is currently no consensus for clustering and prediction models in machine learning in the field of medicine. Thus, a unified reporting standard should be established to standardize research and writing.
Conclusions
In this review, we primarily focused on the research application of AI in ARDS, which mainly included two aspects: ARDS subgroup classification and ARDS diagnosis and survival prediction. Individualized treatment of ARDS has become possible due to the discovery of hyper- and hypoinflammatory subtypes in patients with ARDS, and diagnosis and survival prediction are essential in disease management. The emergence of AI and its medical applications may be useful in clinical decision-making, but their reliability and generalizability to populations should be clarified in prospective studies by introducing AI and machine learning and conducting bedside testing in larger populations to create a more stable and time-resilient model. A unified standard for conducting and reporting machine learning studies in medicine should be established.
Acknowledgments
We thank DINGDING translation (www.dingdingfanyi.com) for its assistance during the preparation of the manuscript.
Funding: This work was supported by The National Key Research and Development Program of China (No. 2022YFC2504401); The National Key Research and Development Program of China (No. 2020YFC2003700) and the National High Level Hospital Clinical Research Funding (No. 2022-NHLHCRFLX-01-01).
Footnote
Reporting Checklist: The authors have completed the Narrative Review reporting checklist. Available at https://atm.amegroups.com/article/view/10.21037/atm-22-3153/rc
Peer Review File: Available at https://atm.amegroups.com/article/view/10.21037/atm-22-3153/prf
Conflicts of Interest: All authors have completed the ICMJE uniform disclosure form (available at https://atm.amegroups.com/article/view/10.21037/atm-22-3153/rc). All authors reported that this study was funded by The National Key Research and Development Program of China (No. 2022YFC2504401); The National Key Research and Development Program of China (No. 2020YFC2003700) and the National High Level Hospital Clinical Research Funding (No. 2022-NHLHCRFLX-01-01). The authors have no other conflicts of interest to declare.
Ethical Statement: The authors are accountable for all aspects of the work in ensuring that questions related to the accuracy or integrity of any part of the work are appropriately investigated and resolved.
Open Access Statement: This is an Open Access article distributed in accordance with the Creative Commons Attribution-NonCommercial-NoDerivs 4.0 International License (CC BY-NC-ND 4.0), which permits the non-commercial replication and distribution of the article with the strict proviso that no changes or edits are made and the original work is properly cited (including links to both the formal publication through the relevant DOI and the license). See: https://creativecommons.org/licenses/by-nc-nd/4.0/.
References
- Meyer NJ, Gattinoni L, Calfee CS. Acute respiratory distress syndrome. Lancet 2021;398:622-37. [Crossref] [PubMed]
- Bellani G, Laffey JG, Pham T, et al. Epidemiology, Patterns of Care, and Mortality for Patients With Acute Respiratory Distress Syndrome in Intensive Care Units in 50 Countries. JAMA 2016;315:788-800. [Crossref] [PubMed]
- Huang X, Zhang R, Fan G, et al. Incidence and outcomes of acute respiratory distress syndrome in intensive care units of mainland China: a multicentre prospective longitudinal study. Crit Care 2020;24:515. [Crossref] [PubMed]
- Price WN 2nd, Cohen IG. Privacy in the age of medical big data. Nat Med 2019;25:37-43. [Crossref] [PubMed]
- Zhang Z, Navarese EP, Zheng B, et al. Analytics with artificial intelligence to advance the treatment of acute respiratory distress syndrome. J Evid Based Med 2020;13:301-12. [Crossref] [PubMed]
- Deo RC. Machine Learning in Medicine. Circulation 2015;132:1920-30. [Crossref] [PubMed]
- Sinha P, Calfee CS. Phenotypes in acute respiratory distress syndrome: moving towards precision medicine. Curr Opin Crit Care 2019;25:12-20. [Crossref] [PubMed]
- Li X, Ma X. Acute respiratory failure in COVID-19: is it “typical” ARDS? Crit Care 2020;24:198. [Crossref] [PubMed]
- Bos LDJ. COVID-19-related acute respiratory distress syndrome: not so atypical. Am J Respir Crit Care Med 2020;202:622-4.
- Gibson PG, Qin L, Puah SH. COVID-19 acute respiratory distress syndrome (ARDS): clinical features and differences from typical pre-COVID-19 ARDS. Med J Aust 2020;213:54-56.e1. [Crossref] [PubMed]
- Calfee CS, Delucchi K, Parsons PE, et al. Subphenotypes in acute respiratory distress syndrome: latent class analysis of data from two randomised controlled trials. Lancet Respir Med 2014;2:611-20. [Crossref] [PubMed]
- Famous KR, Delucchi K, Ware LB, et al. Acute Respiratory Distress Syndrome Subphenotypes Respond Differently to Randomized Fluid Management Strategy. Am J Respir Crit Care Med 2017;195:331-8. [Crossref] [PubMed]
- Calfee CS, Delucchi KL, Sinha P, et al. Acute respiratory distress syndrome subphenotypes and differential response to simvastatin: secondary analysis of a randomised controlled trial. Lancet Respir Med 2018;6:691-8. [Crossref] [PubMed]
- Sinha P, Delucchi KL, Thompson BT, et al. Latent class analysis of ARDS subphenotypes: a secondary analysis of the statins for acutely injured lungs from sepsis (SAILS) study. Intensive Care Med 2018;44:1859-69. [Crossref] [PubMed]
- Sinha P, Delucchi KL, McAuley DF, et al. Development and validation of parsimonious algorithms to classify acute respiratory distress syndrome phenotypes: a secondary analysis of randomised controlled trials. Lancet Respir Med 2020;8:247-57. [Crossref] [PubMed]
- Sinha P, Churpek MM, Calfee CS. Machine Learning Classifier Models Can Identify Acute Respiratory Distress Syndrome Phenotypes Using Readily Available Clinical Data. Am J Respir Crit Care Med 2020;202:996-1004. [Crossref] [PubMed]
- Maddali MV, Churpek M, Pham T, et al. Validation and utility of ARDS subphenotypes identified by machine-learning models using clinical data: an observational, multicohort, retrospective analysis. Lancet Respir Med 2022;10:367-77. [Crossref] [PubMed]
- Sinha P, Delucchi KL, Chen Y, et al. Latent class analysis-derived subphenotypes are generalisable to observational cohorts of acute respiratory distress syndrome: a prospective study. Thorax 2022;77:13-21. [Crossref] [PubMed]
- Hashem MD, Hopkins RO, Colantuoni E, et al. Six-month and 12-month patient outcomes based on inflammatory subphenotypes in sepsis-associated ARDS: secondary analysis of SAILS-ALTOS trial. Thorax 2022;77:22-30. [Crossref] [PubMed]
- Liu X, Jiang Y, Jia X, et al. Identification of distinct clinical phenotypes of acute respiratory distress syndrome with differential responses to treatment. Crit Care 2021;25:320. [Crossref] [PubMed]
- Ding XF, Li JB, Liang HY, et al. Predictive model for acute respiratory distress syndrome events in ICU patients in China using machine learning algorithms: a secondary analysis of a cohort study. J Transl Med 2019;17:326. [Crossref] [PubMed]
- Zeiberg D, Prahlad T, Nallamothu BK, et al. Machine learning for patient risk stratification for acute respiratory distress syndrome. PLoS One 2019;14:e0214465. [Crossref] [PubMed]
- Brown LM, Calfee CS, Matthay MA, et al. A simple classification model for hospital mortality in patients with acute lung injury managed with lung protective ventilation. Crit Care Med 2011;39:2645-51. [Crossref] [PubMed]
- Huang B, Liang D, Zou R, et al. Mortality prediction for patients with acute respiratory distress syndrome based on machine learning: a population-based study. Ann Transl Med 2021;9:794. [Crossref] [PubMed]
- Zhang Z. Prediction model for patients with acute respiratory distress syndrome: use of a genetic algorithm to develop a neural network model. PeerJ 2019;7:e7719. [Crossref] [PubMed]
- Shimatani T, Ohshimo S, Shime N. Next Step to Understanding Subphenotypes of Acute Respiratory Distress Syndrome. Am J Respir Crit Care Med 2017;196:795-6. [Crossref] [PubMed]
- Lazarsfeld PF, Henry NW. Latent structure analysis. Boston, MA: Houghton Mifflin; 1968.
- Dean N, Raftery AE. Latent Class Analysis Variable Selection. Ann Inst Stat Math 2010;62:11-35. [Crossref] [PubMed]
- van Smeden M, Naaktgeboren CA, Reitsma JB, et al. Latent class models in diagnostic studies when there is no reference standard--a systematic review. Am J Epidemiol 2014;179:423-31. [Crossref] [PubMed]
- Rindskopf D, Rindskopf W. The value of latent class analysis in medical diagnosis. Stat Med 1986;5:21-7. [Crossref] [PubMed]
- Reilly JP, Meyer NJ. Pattern recognition in ARDS: a crucial first step toward personalised treatment. Lancet Respir Med 2014;2:594-5. [Crossref] [PubMed]
- van de Sande D, van Genderen ME, Huiskens J, et al. Moving from bytes to bedside: a systematic review on the use of artificial intelligence in the intensive care unit. Intensive Care Med 2021;47:750-60. [Crossref] [PubMed]