Development and validation of a prognostic model and gene co-expression networks for breast carcinoma based on scRNA-seq and bulk-seq data
Highlight box
Key findings
• Constructed gene co-expression networks based on scRNA-seq data of malignant epithelial cells of breast cancer.
• Constructed a well-performed malignant cell-related prognostic model for breast cancer.
• Established a web app for other researchers to get our results.
What is known and what is new?
• There were lots of studies about constructing gene co-expression networks and prognostic models based on bulk RNA-seq which may be noised by the gene expression of other components of the tumor microenvironment.
• Here, we constructed gene co-expression networks and a well performed prognostic model based on scRNA-seq data of malignant cells of breast cancer and evaluated them in multiple data.
What is the implication, and what should change now?
• Our results provide insights into the mechanisms underlying breast carcinoma progression and suggest potential therapeutic strategies for the treatment of this cancer.
Introduction
Breast carcinoma arises from the epithelial cells of the breast and is the most common malignant tumor in women worldwide. A recent study estimated there were about 2.3 million new cases of breast cancer each year globally (1). Various treatment strategies that target specific molecular subtypes of breast carcinoma, such as progesterone receptor-positive (PR+) cells and human epidermal growth factor receptor 2-positive (HER2+) cells, have greatly improved the survival outcomes for about 70–80% of breast cancer patients (2), suggesting that the identification of additional molecular subtypes may also provide better survival in these patients. However, further research is needed to identify prognosis-related biomarkers and the functions and molecular mechanisms of these molecules.
The tumor, node, metastasis staging system of malignant tumors (TNM stage) is universally recognized as a significant predictor of clinical outcomes. However, its predictive power is limited, and increasing evidence suggests that the prognosis of breast cancer patients is not only related to the TNM stage but also the expression levels of key biomarkers. In particular, previous transcriptional analyses that used bulk RNA-seq data found that changes in the levels of SMC4, UBE2C, and JAM2 mRNAs were related to breast cancer prognosis (3-5). Given the heterogeneous composition of the tumor microenvironment (TME), which includes T cells, B cells, and numerous other cell types, coupled with the fact that many different types of cells can affect breast carcinoma progression (6-8), the use of bulk RNA-seq to identify dysregulated genes in breast cancer may generate misleading results. Moreover, analysis of the co-expression networks in breast cancer using bulk RNA-seq, an approach widely used to describe gene interactions and molecular mechanisms, could also produce misleading results.
Bulk RNA-sequencing (Bulk RNA-seq) is the method that detects transcriptomics profiles of biopsies. It shows the average expression of genes across numerous cells (9).
On the other hand, single-cell transcriptional sequencing (scRNA-seq) is a transcriptome technology that can simultaneously quantify gene expression at the genome-wide level in thousands of individual cells (10,11) and provides a solution to help address the above-mentioned issues (screening of tumor-cell-related biomarkers and construction of gene co-expression networks). Thus, scRNA-seq is a powerful tool for studying heterogeneous tissues, such as the TME. More specifically, scRNA-seq can reduce the false-positive identification of differentially expressed genes (DEGs) that may be caused by multiple non-target cell types in the TME, and is well-suited for determining the differences between tumor and normal cells. This new method also facilitates the study of gene-gene relationships, thus enabling the investigation of co-expression networks (12). Several recent scRNA-seq studies have examined the heterogeneous environment of breast carcinoma (11,13), with a particular focus on the tumor immune microenvironment and cancer cell subpopulations. These scRNA-seq datasets are a useful resource for researchers attempting to identify novel breast cancer molecular subtypes and improve prognostic prediction. However, few studies have focused on the transcriptomic signature of malignant epithelial cells in breast carcinoma, especially their gene co-expression network. Moreover, today, most breast cancer treatments are primarily directed against tumor cells. We believe that a prognostic model for tumor cells can better guide treatment strategy. Therefore, we built a prognostic model based on genes that focus on tumor cells screened from scRNA-seq data. This approach has been utilized to study other tumors (14-16); however, breast cancer has not yet been examined.
In this work, we used publicly available breast cancer scRNA-seq data to compare the transcriptomic changes in breast malignant epithelial cells to those of non-malignant epithelial cells and obtained a set of 1,262 genes that are dysregulated in malignant breast epithelial cells. To examine the functions of these dysregulated genes, we constructed co-expression networks for all 1,262 genes in individual malignant epithelial cells using scRNA-seq data and performed pathway enrichment analysis to annotate their functions. We then utilized over 300 published transcriptomics datasets and 31 Clustered Regularly Interspaced Short Palindromic Repeats (CRISPR) screening data to verify the co-expression networks. On this basis, iterative least absolute shrinkage and selection operator (LASSO) Cox analysis was used to conduct the second round of screening, and a collection of breast cancer prognosis-related gene sets consisting of 10 genes was obtained; thus, a new breast cancer patient prognostic stratification strategy was constructed. Finally, we constructed a breast cancer prognostic prediction model with good predictive power based on risk grouping and other clinical characteristics. Our model performed well in both internal corhort and external corhort. Our analyses of malignant breast epithelial cells will provide a characterization of the transcriptomic mechanisms and gene co-expression networks during the progression of breast carcinoma, and may also help identify novel biomarkers and therapeutic targets. We present the following article in accordance with the TRIPOD reporting checklist (available at https://atm.amegroups.com/article/view/10.21037/atm-22-5684/rc).
Methods
Single-cell data retrieval and processing
The scRNA-seq data in this study were obtained from Gene Expression Omnibus (GEO: GSE161529) (17) and included data from in situ breast carcinoma and control samples (such as adjacent tissues from breast cancer patients and normal breast tissue from patients without breast cancer). Samples from precancerous lesions and lymph nodes were excluded. Scrublet (https://github.com/swolock/scrublet) was used to predict doublets in these data using an expected doublet rate of 0.06 and default parameters otherwise (18). The SoupX R package (https://github.com/constantAmateur/SoupX) was employed to estimate and remove cell-free mRNA contamination in the droplets (19). The study was conducted in accordance with the Declaration of Helsinki (as revised in 2013).
Further quality control was then applied using key metrics, including the number of unique genes detected in each cell (nFeature_RNA >500), the total number of detected molecules (nCount_RNA >1,000), and the percentage of mitochondrial genes in each cell (percent.mt <25%). Seurat’s pipeline (https://github.com/satijalab/seurat/) was used for dimensional reduction and unsupervised clustering after quality control (20). The specific steps were as follows: (I) normalization of the data using the NormalizeData and ScaleData functions of the Seurat package (20); (II) selection of 3,000 hypervariable genes for downstream analysis using the FindVariableFeatures function; and (III) calculation of 30 principal components for dimensionality reduction. Since each sample was processed separately, “batch effect” was a possibility. Thus, Harmony (https://github.com/immunogenomics/harmony) was used to correct for the batch effect between patients (21). RunUMAP and the FindClusters function were used to reduce dimensionality and identify clusters (dims =1:30, resolution =0.3). Finally, epithelial cells were identified using universal markers.
Identification of malignant epithelial cells and DEGs
The evidence for somatic alterations of large-scale chromosomal copy number variants (gains or losses) in single cells was determined using inferCNV with the default parameters (https://github.com/broadinstitute/inferCNV). To distinguish malignant and non-malignant cells in the tumor sample-derived epithelial cells, we performed the following analysis. First, epithelial cells from the standard control samples were annotated a reference. Second, the clustering algorithm was applied to all of the epithelial cells from tumor samples. Third, a second round of dimensional reduction and unsupervised clustering was performed to identify epithelial cell subsets. Finally, cell clusters with high chromosome copy number variations (CNVs) relative to the control (reference) were considered malignant. To be specific, clusters with higher CNVs was defiended as malignant epithelial cells. On the contrast, clusters with lower CNVs was defiended as malignant epithelial cells
Next, we analyzed the differential expression in malignant epithelium relative to the non-malignant epithelium using the FindMarkers function from the Seurat package. Genes were considered to be differentially expressed based on the standard thresholds (avg_log_FC >0.25 or avg_log_FC <−0.25, adjusted P<0.05). Only genes that had higher expression in malignant cells (avg_log_FC >0.25, adjusted P<0.05) were considered to be DEGs and subjected to further co-expression gene analysis.
Co-expression of DEGs
To assess gene co-expression, Spearman’s rank correlation coefficient was determined for all possible combinations of DEGs, and the Benjamini-Hochberg method was used to correct these P values for multiple comparisons. Thus, for each DEG gene i, any other gene whose expression exhibited a significant correlation (adjusted P<0.05) was defined as a co-expressed gene.
Functional enrichment of DEGs
The method of Li et al. (22) was employed for the functional annotation of the DEGs. Briefly, for gene j and another gene i, the rank score (RS) was calculated using the adjusted P value (adjPij), and the correlation coefficient (Rij) was calculated as follows:
Next, all genes were sorted according to the RS and were used in a pre-ranked gene set enrichment analysis (GSEA) to identify potentially related pathways. The fgsea package (https://github.com/ctlab/fgsea) was used for GSEA analysis (23), with a focus on the Hallmark gene sets, which were downloaded from the Sigdb database (https://www.gsea-msigdb.org/gsea/msigdb/). Gene sets with an adjusted P value lower than 0.05 in the GSEA were defined as correlated pathways (24).
Validation of the gene co-expression networks and functional enrichment of the DEGs
The Search-Based Exploration of Expression Compendium (SEEK: https://seek.princeton.edu/seek/) was utilized to validate the gene co-expression networks (25). For the co-expression network of each gene, the top 100 co-expressed genes (due to the input limits of SEEK) with the highest RS values were screened for verification. Only data sets related to breast carcinoma were used for validation. The data sets with P values (provided by SEEK) below 0.05 were considered suitable for co-expression verification.
CRISPR screening data were downloaded from The Biological General Repository for Interaction Datasets (BioGRID) (https://orcs.thebiogrid.org/) (26). The datasets related to breast carcinoma and cell proliferation were used for verification. For each dataset, genes related to cell proliferation were defined according to their original author’s choice. Briefly, a gene whose Hit value was “yes”1 was considered to be related to cell proliferation. Genes that included cell proliferation-related pathways (E2F targets, G2M checkpoint, MYC targets V1, MYC targets V2, P53 pathway, and mitotic spindle; adjusted P value <0.05 and NES >0; screened only in genes that are highly expressed in malignant cells) in their co-expression gene pathway enrichment results were also considered to be related to cell proliferation (27).
Construction of a DEG-based COX prognostic model
Data collection
TGCA-BRCA cohort (internal cohort) bulk RNA-seq data for breast carcinoma were downloaded from the UCSC Xena (http://xena.ucsc.edu/) (28). All of the analyzed tissue samples were from patients who had primary breast carcinoma and were older than 18 years of age. Patients with unknown race, age, TNM stage, or gender were excluded. A standard data collection and filtering workflow was used (Figure 1A). The external cohort data (METABRIC cohort) was downloaded from cBioportal (https://www.cbioportal.org/).
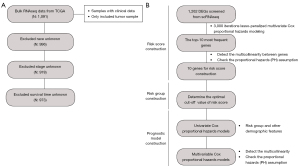
Risk group construction
DEGs from the single-cell RNAseq data were used to construct a breast cancer-related risk group. First, the bulk RNA-seq data for breast carcinoma patients [from The Cancer Genome Atlas (TCGA)] were divided into a training set (60%) and a testing set (40%). In the training set, the iterative LASSO technique was used to screen for characteristic genes in the model. The workflow for risk group construction utilized defined procedures (Figure 1B). Initially, 3,000 iterations were used for variable screening of the DEGs from the scRNA-seq analysis using a LASSO-penalized multivariate Cox proportional hazards model with the three-fold cross-validation set (29). Next, genes in the DEGs were sorted according to their frequency in the 3,000 iterations, and genes with the highest frequency were considered an important signature for the prediction of patient survival. Subsequently, the 10 most frequent genes were selected to construct a risk score with Cox regression. The following equation was applied to calculate the risk score, in which the coefficients were from the Cox regression:
Thereafter, the Variance Inflation Factor (VIF) was used to assess collinearity between genes (threshold: VIF =4) (30). The cox.zph function was employed to check the proportional hazards (PH) assumption (31), and features with P values greater than 0.05 were considered to satisfy the PH assumption. Lastly, receiver operating characteristic (ROC) analysis was performed used to determine the model accuracy and the optimal cut-off value for patient stratification.
Construction and evaluation of the COX prognostic model
We constructed a prognostic model to predict overall survival in breast cancer patients. Univariable Cox regression analysis was performed to screen for prognostic factors and clinical factors (age, gender, stage, race, and risk group). All variables with P values less than 0.05 in the univariable Cox regression analysis were included in the multivariate Cox regression analysis. Collinearity was determined based on the VIF, and the PH assumption of variables in the multivariate Cox regression analysis was confirmed. The area under the curve (AUC) was used to assess the prognostic value of the model at different times in the internal (TCGA cohort) and external (METABRIC cohort) cohorts.
Differences between DEGs in the scRNA-seq and bulk RNA-seq data
The bulk RNA-seq data for breast carcinoma were downloaded from UCSC Xena (http://xena.ucsc.edu/) (28). Tissue samples from carcinomas in situ and normal tissues were retained for further analysis. DeSeq2 (https://github.com/mikelove/DESeq2) was used to screen for DEGs, followed by ashr to remove noise and preserve large differences (32,33). DEGs from bulk RNA-seq were defined using the standard criteria (log2FoldChange <−1 or >1, adjusted P<0.05). Only genes that were highly expressed in malignant breast tissue (log2FoldChange >1, adjusted P<0.05 in seqseq; avg_log_FC >0.25, adjusted P<0.05 in scRNA-seq) were used to compare the bulk RNA-seq DEGs with those of scRNA-seq. Gene Ontology (GO) enrichment analyses of the different DEGs were performed using the clusterProfiler R package (https://guangchuangyu.github.io/software/clusterProfiler/), and the function simplify was applied to reduce the redundancy of the enriched GO terms (34). GO terms with adjusted P values less than 0.05 were considered in the final result.
Shiny App construction
We developed an online application, the Gene co-expression Network in Breast Cancer (GCNBC), based on the shiny framework (https://shiny.rstudio.com/, with the shinydashboard and shiny R packages), whose workflow was described above. The app was then deployed on the shinyapps.io website (https://www.shinyapps.io/). The DT R package was used to present tables, and the igraph package was used to display the figures of the gene co-expression network.
Statistical analysis
The Wilcoxon test was used to analyze the differences between two groups of continuous variables that were non-normally distributed. The chi-square test was used to analyze the variance of categorical variables. The Kaplan-Meier and log-rank tests were used to analyze the survival differences. All data analyses were performed using R version 3.6.3 or Python version 3.8.5. Unless otherwise specified, a P value below 0.05 was considered statistically significant.
Results
Identification of DEGs in malignant epithelial cells
We analyzed the largest breast carcinoma single-cell dataset (GEO: GSE161529) from a public domain, which consisted of 58 samples from in situ carcinomas (n=34) and control tissues (including adjacent tissue from breast cancer patients and normal breast tissue from patients without breast cancer, n=24) from 49 patients. We applied a series of strict quality control procedures to remove cells with low-quality data and low-gene expression, and ultimately examined 273,053 cells (166,569 and 106,484 cells for tumor and non-cancerous tissues, respectively). We then annotated and obtained 169,487 epithelial cells according to the expression levels of well-known epithelial cell markers (KRT family genes and CLDN4), including 104,607 and 64,880 cells for tumor and non-cancerous tissues, respectively (Figure 2A,2B).
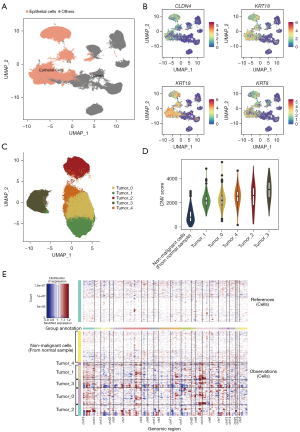
We used inferCNV to distinguish non-malignant epithelial cells from tumor samples. The results indicated that the cell subpopulations from tumor samples were in five clusters (Figure 2C) and that there were large CNVs in all five tumor epithelial cells clusters relative to the normal cells (Figures 2D,2E), which suggested that there were no detectable normal epithelial cells among the tumor samples in these data. We then annotated the epithelial cells as either malignant or non-malignant according to the CNVs, and the results showed there were 104,607 malignant cells and 64,880 non-malignant cells.
Differential expression analysis indicated that there were 1,262 dysregulated genes in the malignant cells relative to the normal cells (adjusted P<0.05, absolute average logFC >0.25). Among these, 615 genes exhibited a high expression in malignant cells and 647 genes had high expression in normal cells (Figure 3A, available online: https://cdn.amegroups.cn/static/public/atm-22-5684-1.xlsx).
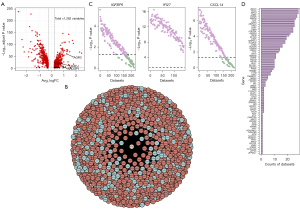
Construction and verification of DEGs in the gene co-expression network
We then examined the possible molecular mechanisms of these 1,262 DEGs by constructing co-expression networks of these DEGs in malignant epithelial cells at a single-cell resolution using Spearman correlation analysis (see our web app GCNBC, https://prognosticpredictor.shinyapps.io/GCNBC/, Figure 3B). Among these, we observed a strong correlation in the expressions of AGR3 and ESR1 (rho = 0.369, adjusted P<0.001), which is consistent with a previous study that reported this correlation in ER+ breast carcinoma cell lines (35). We also observed that ADAR, whose inactivation can lead to a weak IFN response (36), was co-expressed with IF16 in malignant epithelial cells (rho = 0.142, adjusted P<0.001).
Next, we characterized the signaling pathways in which these DEGs functioned in breast cancer epithelium using functional enrichment analysis for each DEG based on its co-expression network (available online: https://cdn.amegroups.cn/static/public/atm-22-5684-2.xlsx). The co-expression networks and functional analyses provided valuable information for studying the function of the dysregulated genes.
We then verified the co-expression networks. We first validated the co-expression of genes in these networks by testing them in more than 300 breast-related transcriptomics data using SEEK (https://seek.princeton.edu/seek/), a bioinformatics data portal that analyzes gene co-expression relationships based on the GEO datasets. For this analysis, we selected the three DEGs with the largest average logFC (IGFBP5, IFI27, and CXCL14; available online: https://cdn.amegroups.cn/static/public/atm-22-5684-1.xlsx) using the top 100 co-expressed genes ranked by RS (due to the input limitations of SEEK). The results indicated co-expression of the tested DEGs and the corresponding 100 genes in most breast cancer-related datasets (70.6% for IGFBP5, 100% for IFI27, and 72.9% for CXCL14; Figure 3C), which support the reliability of our co-expression networks.
We then verified the functional annotation of the co-expression networks. Among the 1,262 epithelial genes related to malignancy, we analyzed the association between 163 genes and cell proliferation based on the functional annotation of their co-expression networks (available online: https://cdn.amegroups.cn/static/public/atm-22-5684-3.xlsx). We validated the association of these 163 genes with cell proliferation using the published CRISPR screening data from BioGRID. CRISPR screening is a large-scale genetic loss-of-function experimental approach that can provide experimental evidence of key genes and identify a specific phenotype in a specific cell line (37). There were 31 CRISPR screening studies related to breast cancer and cell proliferation, and 81 of the 163 genes we analyzed were correlated with proliferation in at least one of the CRISPR screens (Figure 3D). These CRISPR screening results provide experimental support for the validity of the functional annotation of our co-expression networks and support the reliability of our functional annotation results.
Prognostic model based on single-cell DEGs
To evaluate the potential use of DEGs for prognostic prediction and risk stratification, we screened the 1,262 DEGs in 973 breast carcinoma patients from TCGA (including 584 in the training set and 389 in the testing set, Table 1) using the iterative LASSO technique. Since there was a large decline in frequency between the tenth and eleventh most frequent genes (Figure S1), we examined 10 candidate genes (MAL2, BAMBI, ALDH3B2, TMEM14B, MTRNR2L12, SDCBP2, DAB2, TARS, CSTA, and NDUFB1) to define the individual risk score using multivariable Cox regression analysis (Figure 4A). The results indicated that none of these genes were statistically significant, which is consistent with the PH assumption (Figure S2A,S2B). Thus, we defined the risk score using the following equation:
Table 1
Characteristic | TCGA cohort | METABRIC cohort (N=1,764) | ||
---|---|---|---|---|
Training set (N=584) | Test set (N=389) | Overall (N=973) | ||
Race | ||||
White | 447 (76.5) | 291 (74.8) | 738 (75.8) | – |
Black or African American | 96 (16.4) | 80 (20.6) | 176 (18.1) | – |
Others | 41 (7.0) | 18 (4.6) | 59 (6.1) | – |
Age, years | 59 [26, 90] | 55 [27, 90] | 58 [26, 90] | 61 [51, 70] |
TNM stage | ||||
I | 106 (18.2) | 70 (18.0) | 176 (18.1) | 630 (35.7) |
II | 338 (57.9) | 220 (56.6) | 558 (57.3) | 979 (55.5) |
III | 131 (22.4) | 92 (23.7) | 223 (22.9) | 144 (8.16) |
IV | 9 (1.5) | 7 (1.8) | 16 (1.6) | 11 (0.62) |
Data are presented as n (%) or median [25 percentile, 75 percentile]. TCGA, The Cancer Genome Atlas; METABRIC, Molecular Taxonomy of Breast Cancer International Consortium; TNM stage, the tumor, node, metastasis staging system of malignant tumors.

We also classified patients into low- or high-risk groups according to the optimal cut-off value for this risk score.
Given that tumor stage, patient age, and risk group were related to prognosis in the univariate Cox analysis (Table 2), we examined these three factors in the prognostic model using multivariate Cox analysis. The results showed that risk group was an independent prognostic factor (Table 2, Figure 4B). In addition, our model performed well in the training and testing sets at all of the tested follow-up times (Figure 4C, Table 3), with AUC values ranging from 0.801 to 0.857 (training set) and 0.736 to 0.897 (test set). Furthermore, the model was tested on an external cohort (Table 1). The results showed that our model also performed well in the external cohort, with AUC values ranging from 0.639 to 0.693 (Figure 4D, Table 3).
Table 2
Characteristic | Univariate Cox regression | Multivariate Cox regression | |||||
---|---|---|---|---|---|---|---|
HR (95% CI) | P | HR (95% CI) | P# | VIF | P* | ||
Age | 1.04 (1.02, 1.06) | <0.001 | 1.04 (1.02, 1.06) | <0.001 | 1.02 | 0.51 | |
Race | |||||||
White | Ref | Ref | – | – | – | – | |
Black or African American | 0.98 (0.54, 1.77) | 0.937 | – | – | – | – | |
Others | 0.71 (0.17, 2.93) | 0.64 | – | – | – | – | |
Risk group | |||||||
Low risk | Ref | Ref | Ref | Ref | 1.01 | 0.53 | |
High risk | 4.06 (2.52, 6.53) | <0.001 | 4.28 (2.65, 6.91) | <0.001 | – | – | |
TNM stage | |||||||
I | Ref | Ref | Ref | Ref | 1.04 | 0.22 | |
II | 1.49 (0.76, 2.9) | 0.245 | 1.8 (0.92, 3.53) | 0.088 | – | – | |
III | 2.45 (1.19, 5.06) | 0.015 | 3.2 (1.54, 6.64) | 0.002 | – | – | |
IV | 14.98 (5.46, 41.12) | <0.001 | 20.99 (7.52, 58.57) | <0.001 | – | – |
#, P value from multivariate Cox regression. *, P value from the proportional hazards assumption. HR, hazard ratio; CI, confidence interval; VIF, variance inflation factor; TNM stage, the tumor, node, metastasis staging system of malignant tumors.
Table 3
Cohort | Timepoint | AUC (95% CI) |
---|---|---|
TCGA_Train | 1 | 0.857 (0.744, 0.95) |
TCGA_Train | 3 | 0.852 (0.788, 0.922) |
TCGA_Train | 5 | 0.801 (0.736, 0.867) |
TCGA_Train | 10 | 0.832 (0.739, 0.917) |
TCGA_Test | 1 | 0.897 (0.811, 0.977) |
TCGA_Test | 3 | 0.772 (0.688, 0.854) |
TCGA_Test | 5 | 0.736 (0.664, 0.828) |
TCGA_Test | 10 | 0.787 (0.634, 0.97) |
METABRIC | 1 | 0.693 (0.609, 0.812) |
METABRIC | 3 | 0.654 (0.624, 0.687) |
METABRIC | 5 | 0.660 (0.632, 0.685) |
METABRIC | 10 | 0.639 (0.665, 0.665) |
AUC, area under curve; TCGA, The Cancer Genome Atlas; METABRIC, Molecular Taxonomy of Breast Cancer International Consortium; CI, confidence interval.
Comparison of the DEGs from scRNA-seq and bulk RNA-seq
To determine the value of using single-cell analysis, we compared the transcriptional changes of gene expression in breast cancer using scRNA-seq and bulk RNA-seq. The results showed that most of the genes (n=18,194) were detected both in scRNA-seq and bulk RNA-seq data; however, the DEGs in these datasets differed significantly, and there were only 182 common DEGs (Figure 5A). Moreover, 2,599 DEGs that were identified using bulk RNA-seq data were not identified as DEGs in the scRNA-seq data. These genes included some marker genes of cell types other than epithelial cells (available online: https://cdn.amegroups.cn/static/public/atm-22-5684-4.xlsx), such as CD3D (T-cell marker) and CD19 (B-cell marker). Consistently, the GO results of the 2,599 DEGs suggested their involvement in various non-malignant-cell-related processes, such as regulatory T-cell differentiation and neural precursor cell proliferation (Figure 5B). Thus, we believe that the complex cell composition of bulk carcinoma tissue leads to confounding bias when performing bulk RNA-seq data analysis. In contrast, the DEGs identified using high-quality scRNA-seq data provided more specific and accurate descriptions of DEGs in breast carcinoma epithelial cells.
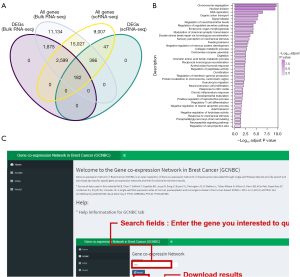
GCNBC web apps
All of our results are publicly available via an interactive web application (Figure 5C), Gene Co-expression Network in Breast Cancer (GCNBC; https://prognosticpredictor.shinyapps.io/GCNBC/). The menu on the left of the web app provides options including “Home” (introduction and tutorial), “GCNBC” (access portal to our gene co-expression network results), “GSEA” (our GSEA functional enrichment analysis results), and “Model” (our prognostic models). Users can get the predictive results by puting the gene expression and clinical information into the “Model” module in our web app.
Discussion
ScRNA-seq technology is a new method that allows researchers to examine the heterogeneity of cancer cells within a tumor, discover novel potential therapeutic biomarkers, and study the co-expression relationships between genes at single-cell resolutions. We performed a comprehensive analysis of breast carcinoma datasets at the level of single cells by examining 169,487 breast epithelial cells (including 104,607 malignant epithelial cells and 6,484 non-malignant epithelial cells) to characterize the transcriptomic changes of epithelial cells in breast carcinoma. Through this analysis, we identified 1,262 genes that form a signature for breast carcinoma and then described their functions and co-expression networks. Notably, several novel genes that were highly expressed in breast cancer cells—H2AFJ and ARL61P1—have established functions in the resistance to cell proliferation in glioblastoma multiforme (38) and cervical cancer (39). Some of the DEGs identified herein were also highly expressed in breast carcinoma cells, such as TFF3 (40) and AGR3 (35,41,42). Previous research suggests that TFF3 promotes angiogenesis in breast cancer (43), and AGR3 promotes the proliferation and migration of malignant breast cancer cells and functions in the resistance of breast carcinoma to tamoxifen (35,41,42). These results confirm the robustness of our analytic pipeline and enabled the identification of DEGs that play important roles in the development of breast carcinoma. Moreover, our comparison of DEGs from the scRNA-seq and bulk RNA-seq data demonstrated the advantages of studying transcriptomic changes using scRNA-seq data.
Although single-cell sequencing technology can detect tumor-related genes and characterize their co-expression networks, most current scRNA-seq research of breast carcinoma focuses on the tumor immune microenvironment or the detection of heterogeneous clusters among tumor cells. Large-scale single-cell data can elucidate malignancy-related genes in breast cancer and their co-expression networks well. Our study is the first to present the co-expression networks of 1,262 DEGs in breast carcinoma epithelial cells at a single-cell resolution. We then performed a functional annotation analysis of these networks to annotate the function of the dysregulated genes. Although we did not experimentally validate these co-expression networks or their functional annotations, a secondary analysis of the data from public co-expression datasets (SEEK) and public CRISPR screens showed consistent results. Our findings provide an important foundation and insights for subsequent research. Finally, we provided a user-friendly web application so that other researchers can access our results, including the co-expression networks and functional annotation results. The findings presented here improve our understanding of the mechanisms of breast carcinoma at the level of individual cells and provide new insights for the development of targeted therapies.
Numerous studies have constructed prognostic models for cancer based on scRNA-seq and bulk RNA-seq datasets in other tumors; however, none have been developed for breast cancer (14-16,43). Using the iterative LASSO method, we identified 10 genes that had good prognostic value and could be used to reliably stratify different populations (44). We then utilized these results to develop a prognostic model for breast cancer, expressed as a risk score. Our model not only performed well in both the internal and external cohorts, which indicated that it has tremendous prognostic ability. This reflects the ability of our gene set to be used for patient stratification and prognostic prediction. Previous studies reported that six of the 10 genes included in our risk model play roles in breast tumor promotion or suppression: CALML5, BAMBI, QPRT, CLDN7, TARS, and NDUFB1 (44-49). Three of the 10 genes (NDUFB10, SERPINA3, and PHLDB2I) were not previously identified as related to breast cancer but were reportedly related to other cancers (50-52). Furthermore, one of the 10 genes (MAL2) was reported to mediate the endocytosis and degradation of MHC-I complexes, which could lead to immune evasion of breast cancer cells (53). Our gene co-expression networks showed that the functions of these genes might be broader than previously thought. For instance, we found that BAMBI was related to tumor growth and oxidative phosphorylation (available online: https://cdn.amegroups.cn/static/public/atm-22-5684-2.xlsx). More work is needed to examine the underlying mechanisms of these 10 genes in breast carcinoma. However, despite our model’s strengths, there are still limitations. For instance, we did not analyze patients with missing information, and this model should be validated using a greater number of samples.
Conclusions
In this study, we performed a comprehensive analysis of breast carcinoma using single-cell gene transcription data. We identified a set of potential genes that contribute to breast carcinoma tumorigenesis and tumor development and can be used for prognostic prediction. We also annotated these genes and constructed co-expression networks based on the single-cell data of malignant epithelial cells. Finally, we built a well-performing prognostic model of breast cancer based on scRNA-seq and bulk RNA-seq, which can also be used for prognostic prediction. Taken together, our results provide insights into the mechanisms underlying breast carcinoma progression and suggest potential therapeutic strategies for the treatment of this cancer.
Acknowledgments
Funding: This work was supported by the Basic and Applied Basic Research Fund of Guangdong Province (No. 2022A1515012387).
Footnote
Reporting Checklist: The authors have completed the TRIPOD reporting checklist. Available at https://atm.amegroups.com/article/view/10.21037/atm-22-5684/rc
Conflicts of Interest: All authors have completed the ICMJE uniform disclosure form (available at https://atm.amegroups.com/article/view/10.21037/atm-22-5684/coif). The authors have no conflicts of interest to declare.
Ethical Statement: The authors are accountable for all aspects of the work in ensuring that questions related to the accuracy or integrity of any part of the work are appropriately investigated and resolved. The study was conducted in accordance with the Declaration of Helsinki (as revised in 2013).
Open Access Statement: This is an Open Access article distributed in accordance with the Creative Commons Attribution-NonCommercial-NoDerivs 4.0 International License (CC BY-NC-ND 4.0), which permits the non-commercial replication and distribution of the article with the strict proviso that no changes or edits are made and the original work is properly cited (including links to both the formal publication through the relevant DOI and the license). See: https://creativecommons.org/licenses/by-nc-nd/4.0/.
References
- Sung H, Ferlay J, Siegel RL, et al. Global Cancer Statistics 2020: GLOBOCAN Estimates of Incidence and Mortality Worldwide for 36 Cancers in 185 Countries. CA Cancer J Clin 2021;71:209-49. [Crossref] [PubMed]
- Harbeck N, Penault-Llorca F, Cortes J, et al. Breast cancer. Nat Rev Dis Primers 2019;5:66. [Crossref] [PubMed]
- Ma RM, Yang F, Huang DP, et al. The Prognostic Value of the Expression of SMC4 mRNA in Breast Cancer. Dis Markers 2019;2019:2183057. [Crossref] [PubMed]
- Kariri Y, Toss MS, Alsaleem M, et al. Ubiquitin-conjugating enzyme 2C (UBE2C) is a poor prognostic biomarker in invasive breast cancer. Breast Cancer Res Treat 2022;192:529-39. [Crossref] [PubMed]
- Peng Y, Li H, Fu Y, et al. JAM2 predicts a good prognosis and inhibits invasion and migration by suppressing EMT pathway in breast cancer. Int Immunopharmacol 2022;103:108430. [Crossref] [PubMed]
- Chung W, Eum HH, Lee HO, et al. Single-cell RNA-seq enables comprehensive tumour and immune cell profiling in primary breast cancer. Nat Commun 2017;8:15081. [Crossref] [PubMed]
- Wu SZ, Roden DL, Wang C, et al. Stromal cell diversity associated with immune evasion in human triple-negative breast cancer. EMBO J 2020;39:e104063. [Crossref] [PubMed]
- Azizi E, Carr AJ, Plitas G, et al. Single-Cell Map of Diverse Immune Phenotypes in the Breast Tumor Microenvironment. Cell 2018;174:1293-308.e36. [Crossref] [PubMed]
- Li X, Wang C-Y. From bulk, single-cell to spatial RNA sequencing. International Journal of Oral Science 2021;13:36. [Crossref] [PubMed]
- Klein AM, Mazutis L, Akartuna I, et al. Droplet barcoding for single-cell transcriptomics applied to embryonic stem cells. Cell 2015;161:1187-201. [Crossref] [PubMed]
- Macosko EZ, Basu A, Satija R, et al. Highly Parallel Genome-wide Expression Profiling of Individual Cells Using Nanoliter Droplets. Cell 2015;161:1202-14. [Crossref] [PubMed]
- Trapnell C. Defining cell types and states with single-cell genomics. Genome Res 2015;25:1491-8. [Crossref] [PubMed]
- Chen W, Morabito SJ, Kessenbrock K, et al. Single-cell landscape in mammary epithelium reveals bipotent-like cells associated with breast cancer risk and outcome. Commun Biol 2019;2:306. [Crossref] [PubMed]
- Chen K, Liu X, Liu W, et al. Development and validation of prognostic and diagnostic model for pancreatic ductal adenocarcinoma based on scRNA-seq and bulk-seq datasets. Hum Mol Genet 2022;31:1705-19. [Crossref] [PubMed]
- Zheng P, Zhang H, Jiang W, et al. Establishment of a Prognostic Model of Lung Adenocarcinoma Based on Tumor Heterogeneity. Front Mol Biosci 2022;9:807497. [Crossref] [PubMed]
- Chen Z, Wang Y, Li D, et al. Single-Cell RNA Sequencing Revealed a 3-Gene Panel Predicted the Diagnosis and Prognosis of Thyroid Papillary Carcinoma and Associated With Tumor Immune Microenvironment. Front Oncol 2022;12:862313. [Crossref] [PubMed]
- Pal B, Chen Y, Vaillant F, et al. A single-cell RNA expression atlas of normal, preneoplastic and tumorigenic states in the human breast. EMBO J 2021;40:e107333. [Crossref] [PubMed]
- Wolock SL, Lopez R, Klein AM. Scrublet: Computational Identification of Cell Doublets in Single-Cell Transcriptomic Data. Cell Syst 2019;8:281-91.e9. [Crossref] [PubMed]
- Young MD, Behjati S. SoupX removes ambient RNA contamination from droplet-based single-cell RNA sequencing data. Gigascience 2020;9:giaa151. [Crossref] [PubMed]
- Stuart T, Butler A, Hoffman P, et al. Comprehensive Integration of Single-Cell Data. Cell 2019;177:1888-1902.e21. [Crossref] [PubMed]
- Korsunsky I, Millard N, Fan J, et al. Fast, sensitive and accurate integration of single-cell data with Harmony. Nat Methods 2019;16:1289-96. [Crossref] [PubMed]
- Li Y, Jiang T, Zhou W, et al. Pan-cancer characterization of immune-related lncRNAs identifies potential oncogenic biomarkers. Nat Commun 2020;11:1000. [Crossref] [PubMed]
- Korotkevich G, Sukhov V, Budin N, et al. Fast gene set enrichment analysis. 2021:060012.
- Subramanian A, Tamayo P, Mootha VK, et al. Gene set enrichment analysis: a knowledge-based approach for interpreting genome-wide expression profiles. Proc Natl Acad Sci U S A 2005;102:15545-50. [Crossref] [PubMed]
- Zhu Q, Wong AK, Krishnan A, et al. Targeted exploration and analysis of large cross-platform human transcriptomic compendia. Nat Methods 2015;12:211-4, 3 p following 214.
- Oughtred R, Rust J, Chang C, et al. The BioGRID database: A comprehensive biomedical resource of curated protein, genetic, and chemical interactions. Protein Sci 2021;30:187-200. [Crossref] [PubMed]
- Liberzon A, Birger C, Thorvaldsdóttir H, et al. The Molecular Signatures Database (MSigDB) hallmark gene set collection. Cell Syst 2015;1:417-25. [Crossref] [PubMed]
- Goldman MJ, Craft B, Hastie M, et al. Visualizing and interpreting cancer genomics data via the Xena platform. Nat Biotechnol 2020;38:675-8. [Crossref] [PubMed]
- Sveen A, Ågesen TH, Nesbakken A, et al. ColoGuidePro: a prognostic 7-gene expression signature for stage III colorectal cancer patients. Clin Cancer Res 2012;18:6001-10. [Crossref] [PubMed]
- Belsley DA. A Guide to using the collinearity diagnostics. Computer Science in Economics and Management 1991;4:33-50.
- Grambsch PM, Therneau TMJB. Proportional hazards tests and diagnostics based on weighted residuals. 1994;81:515-26.
- Love MI, Huber W, Anders S. Moderated estimation of fold change and dispersion for RNA-seq data with DESeq2. Genome Biol 2014;15:550. [Crossref] [PubMed]
- Stephens M. False discovery rates: a new deal. Biostatistics 2017;18:275-94. [PubMed]
- Wu T, Hu E, Xu S, et al. clusterProfiler 4.0: A universal enrichment tool for interpreting omics data. Innovation (Camb) 2021;2:100141. [Crossref] [PubMed]
- Obacz J, Sommerova L, Sicari D, et al. Extracellular AGR3 regulates breast cancer cells migration via Src signaling. Oncol Lett 2019;18:4449-56. [Crossref] [PubMed]
- Herzner AM, Khan Z, Van Nostrand EL, et al. ADAR and hnRNPC deficiency synergize in activating endogenous dsRNA-induced type I IFN responses. J Exp Med 2021;218:e20201833. [Crossref] [PubMed]
- Shalem O, Sanjana NE, Hartenian E, et al. Genome-scale CRISPR-Cas9 knockout screening in human cells. Science 2014;343:84-7. [Crossref] [PubMed]
- Yao J, Weremowicz S, Feng B, et al. Combined cDNA array comparative genomic hybridization and serial analysis of gene expression analysis of breast tumor progression. Cancer Res 2006;66:4065-78. [Crossref] [PubMed]
- Guo F, Liu Y, Li Y, et al. Inhibition of ADP-ribosylation factor-like 6 interacting protein 1 suppresses proliferation and reduces tumor cell invasion in CaSki human cervical cancer cells. Mol Biol Rep 2010;37:3819-25. [Crossref] [PubMed]
- Lau WH, Pandey V, Kong X, et al. Trefoil Factor-3 (TFF3) Stimulates De Novo Angiogenesis in Mammary Carcinoma both Directly and Indirectly via IL-8/CXCR2. PLoS One 2015;10:e0141947. [Crossref] [PubMed]
- Jiang R, Sun Y, Chen X, et al. Estrogen-regulated AGR3 activates the estrogen receptor signaling pathway to promote tamoxifen resistance in breast cancer. Breast Cancer Res Treat 2021;190:203-11. [Crossref] [PubMed]
- Xu Q, Shao Y, Zhang J, et al. Anterior Gradient 3 Promotes Breast Cancer Development and Chemotherapy Response. Cancer Res Treat 2020;52:218-45. [Crossref] [PubMed]
- Jiang A, Wang J, Liu N, et al. Integration of Single-Cell RNA Sequencing and Bulk RNA Sequencing Data to Establish and Validate a Prognostic Model for Patients With Lung Adenocarcinoma. Front Genet 2022;13:833797. [Crossref] [PubMed]
- Debald M, Schildberg FA, Linke A, et al. Specific expression of k63-linked ubiquitination of calmodulin-like protein 5 in breast cancer of premenopausal patients. J Cancer Res Clin Oncol 2013;139:2125-32. [Crossref] [PubMed]
- Fusella F, Seclì L, Busso E, et al. The IKK/NF-κB signaling pathway requires Morgana to drive breast cancer metastasis. Nat Commun 2017;8:1636. [Crossref] [PubMed]
- Ősz Á, Lánczky A, Győrffy B. Survival analysis in breast cancer using proteomic data from four independent datasets. Sci Rep 2021;11:16787. [Crossref] [PubMed]
- Yue Z, Shusheng J, Hongtao S, et al. Silencing DSCAM-AS1 suppresses the growth and invasion of ER-positive breast cancer cells by downregulating both DCTPP1 and QPRT. Aging (Albany NY) 2020;12:14754-74. [Crossref] [PubMed]
- Zhou Z, Sun B, Huang S, et al. Roles of aminoacyl-tRNA synthetase-interacting multi-functional proteins in physiology and cancer. Cell Death Dis 2020;11:579. [Crossref] [PubMed]
- Zhang Y, Tian J, Qu C, et al. Overexpression of SERPINA3 promotes tumor invasion and migration, epithelial-mesenchymal-transition in triple-negative breast cancer cells. Breast Cancer 2021;28:859-73. [Crossref] [PubMed]
- Ellinger J, Poss M, Brüggemann M, et al. Systematic Expression Analysis of Mitochondrial Complex I Identifies NDUFS1 as a Biomarker in Clear-Cell Renal-Cell Carcinoma. Clin Genitourin Cancer 2017;15:e551-62. [Crossref] [PubMed]
- Shangguan L, Ti X, Krause U, et al. Inhibition of TGF-β/Smad signaling by BAMBI blocks differentiation of human mesenchymal stem cells to carcinoma-associated fibroblasts and abolishes their protumor effects. Stem Cells 2012;30:2810-9. [Crossref] [PubMed]
- Zeng Z, Cheng J, Ye Q, et al. A 14-Methylation-Driven Differentially Expressed RNA as a Signature for Overall Survival Prediction in Patients with Uterine Corpus Endometrial Carcinoma. DNA Cell Biol 2020;39:975-91. [Crossref] [PubMed]
- Dersh D, Yewdell JW. Immune MAL2-practice: breast cancer immunoevasion via MHC class I degradation. J Clin Invest 2021;131:144344. [Crossref] [PubMed]
(English Language Editor: A. Kassem)