Metabolomic characterization of congenital microtia: a possible analysis for early diagnosis
Highlight box
Key findings
• Serum metabolic biomarkers could distinguish between microtia and controls.
What is known and what is new?
• Metabolic abnormalities are one of the essential risk factors for the abnormity of growth and development.
• The metabolites different between microtia and controls. Meanwhile, these metabolic biomarkers show high sensitivity and specificity for the diagnosis of microtia.
What is the implication, and what should change now?
• Metabolic biomarkers could be used for the early diagnosis of microtia.
Introduction
Congenital microtia is one of the most common congenital craniofacial malformations in children, with an incidence of (0.83–17.40)/10,000 (1-6), which also has a significant familial clustering (7). There are significant regional differences in the incidence of congenital microtia worldwide (1-6). It has been reported that there is a correlation between the occurrence of congenital microtia and parental dietary habits (8,9), and some foods, such as those containing caffeine, have even been considered risk factors for the occurrence of congenital microtia (9). Meanwhile, metabolomic features can affect the development and outcome of a variety of diseases (10-15). Abnormal metabolomics can lead to congenital heart malformations (16-18), diaphragmatic hernia (19), and glaucoma (20). Some metabolomic abnormalities can even serve as biomarkers of disease (21). Normal metabolic pathways were an important guarantee for the normal development of cartilage (22,23), while abnormal metabolic pathways may lead to cartilage tumors or osteoarthritis (24-28). Although there was a study on metabolic mass spectrometry of microtia cartilage (29), it cannot be used for early diagnosis. Abnormal metabolites in the blood were more conducive to early diagnosis. Currently, the relationship between metabolomic abnormalities and blood of congenital microtia remains unclear.
Bone morphogenetic protein 5 (BMP5), as a member of the bone morphogenetic protein family, is involved in the morphogenesis of bone and cartilage, the attachment of soft tissue, and the development of limbs in humans (30). Several studies have found that BMP5 is related to the occurrence of congenital microtia (31-34). Our group has previously demonstrated the possible mechanism of congenital microtia, which may be caused by abnormal lipid metabolism due to the low expression of BMP5, changing mitochondrial function (34). The influence of specific metabolites and possible drug interventions have not been investigated. This study aimed to verify the metabolites with low BMP5 expression through in vitro experiments, and to explore the metabolomic characteristics of congenital microtia with low BMP5 expression, which may help to provide guidance for the prevention of congenital microtia during pregnancy.
In this study, we established cell and patient arrays, including 15 microtia patients and 13 controls, for metabolomic analysis in cell models and further identification in serum samples. We used metabolomic analysis to investigate contributions of BMP5-low expressing cells and normal control (NC) cells signatures and analyzed the metabonomic characteristics to diagnosis of microtia. The data presented here furnish a resource for future early diagnosis of microtia. We present the following article in accordance with the MDAR reporting checklist (available at https://atm.amegroups.com/article/view/10.21037/atm-22-5614/rc).
Methods
Study population
Our study included 28 patients in total, who were grouped as follows: (I) Microtia group (n=15): patients with microtia; (II) Control group (n=13): patients without microtia. The diagnosis of microtia was based on our previous study (7). Cases of acquired auricle deformity was excluded. The study was conducted in accordance with the Declaration of Helsinki (as revised in 2013). All participants and their parents or legal guardians provided written informed consent, and all procedures were approved by the Ethics Committee of Sun Yat-sen Memorial Hospital of Sun Yat-sen University (No. 2019-KY-024).
Metabolite extraction
To extract metabolites from the cell model (siBMP5-2 and siBMP5-3), 2.5 mL of methyl tert-butyl ether (MTBE; Thermo Fisher Scientific, Waltham, MA, USA) was added to 1 mL of the cell extract after thawing at room temperature. Then, the mixture was thoroughly vortexed for 60 seconds, added to 600 µL of water (Merck Millipore, Burlington, MA, USA), shaken for 30 minutes at room temperature, rested for 30 mins, and then centrifuged at 5,000 g for 15 minutes. After that, we transferred 1,000 µL of the lipid extract of the upper layer to a 1.5 mL EP tube, concentrated and dried it under reduced pressure (Labconco Corporation, Kansas City, MO, USA), and used 150 µL of acetonitrile-isopropanol mixture (Thermo Fisher Scientific, USA) for the lipid extract [containing the lipid extract standard PC(15:0/18:1(d7)), TG(15:0/18:1(d7)/15:0), SM(18:1(d9)), LPC(18:1(d7)), PG(15:0/18:1(d7)), ChE(18:1(d7)), LPE(18:1(d7)), and PE(15:0/18:1(d7)) (Avanti, Alabaster, AL, USA)] dissolution.
Ultra-high performance liquid chromatography-high resolution mass spectrometry analysis
For the untargeted metabolomics of the polar metabolites, the extracts were analyzed using ultra-high performance liquid chromatography-high resolution mass spectrometry (UPLC-HRMS; Thermo Fisher Scientific, USA). Lipid molecules were separated by chromatography on an Accucore C30 core-shell column (Thermo Fisher Scinetific, USA) at 50 ℃ and eluted with 60% acetonitrile in water (A) and 10% acetonitrile in isopropanol (B) (Thermo Fisher Scientific, USA). The separation gradient was set up as follows: 10% B at first, ramping up to 50% in 5 minutes, then increasing to 100% in 23 minutes, with the final 7 minutes being used for column washing and equilibration at a flow rate of 0.3 mL/min. The Quadrupole-Orbitrap mass spectrometer (Thermo Fisher, USA) was used in conjunction with a heated electrospray ionization source that had the following operating conditions: capillary temperature 320 ℃, heater temperature 355 ℃, sheath gas 45 arb, aux gas 10 arb, and S-Lens RF level 55%. The acquisition mass range was 250–2,000 m/z. For thorough lipid structural annotation, quality control (QC) samples were repeatedly injected to obtain the top 10 data-dependent MS2 spectra (full scan-ddMS2). For the whole tandem mass spectrometry (MS/MS) data capture, a resolution setting of 17,500 FWHM was chosen. The precursor isolation window was set at 1.0 Da; dynamic exclusion, isotope exclusion, and apex trigger were all activated. Utilizing ultra-pure nitrogen as the fragmentation gas, stepped normalized impact energy was used to cause the dissociation of a metabolite during a collision.
Data processing and analysis
MetaboAnalyst (version 5.0, http://www.metaboanalyst.ca/) (27,28), a free online software, was used to analyze the differences in metabolites and metabolite pathways between the siBMP5 and siNC groups. Principal component analysis (PCA) analysis among all QC samples and the other samples could evaluate the quality control throughout UPLC-HRMS analysis. Metabolites with analysis of variance (ANOVA) test P value <0.05 were considered for further investigation. The summit of the volcano plot showed data points with low ANOVA test P values (highly significant); and those with large fold change (FC) appeared toward the left or right of the center. The cutoff value was set at |log2(FC)| >0.58. Orthogonal partial least squares discriminant analysis (OPLS- DA) allowed selection of 15 metabolites with the highest variable influence on projection (VIP) scores (VIP >1.5) which were considered important for group discrimination. Visualizing the top 50 most important differential metabolites ranked by FC intuitively in a hierarchical clustering heatmap demonstrated the proportion of significantly altered metabolite components.
Pathway analysis
In order to identify differential metabolic pathways between the siBMP5 and siNC groups, pathway analysis was performed with MetaboAnalyst 5.0 (35,36). Over-representation was assessed using hypergeometric tests with Holm-Bonferroni-corrected P values. Differential metabolism was regarded to have significantly enhanced metabolic pathways when P<0.05. The pathway impact value threshold was set to >0.10 (37,38), and the pathway topological analysis was based on the relative betweenness centrality (39).
Determination of PC, TG, and choline contents
Serum samples of all the participants were collected and stored at −80 ℃ for later analysis. We used enzyme-linked immunosorbent assay (ELISA) kits (Meimian, Nanjing, China) to quantify the concentration (PC, TG, and choline) of the samples for confirmation of the differential metabolite changes in cell model, following the kit manufacturer’s instructions. On a microplate reader (SpectraMax® absorbance readers, Molecular Devices, Shanghai, China), the optical density was read at 450 nm. All the samples were analyzed in a single step and in duplicate.
Statistical analysis
The data from serum samples were analyzed using SPSS 23.0 software (IBM Corp., Armonk, NY, USA), which were expressed as a mean ± standard deviation via the t-test between two groups. Statistical significance was considered when P<0.05. Meanwhile, receiver operating characteristic (ROC) analysis illustrated the performance of the newly established metabolic biomarker panel by considering sensitivity and specificity of model through SPSS 23.0.
Results
Clinical characteristics
To confirm the metabolomics changes in the cell model, we profiled serum metabolites of 28 participants, which were collected consecutively at random time period, including 15 patients with microtia and 13 controls. The clinical characteristics of these participants are displayed in Table 1.
Table 1
Variables | Microtia group (n=15) | Control group (n=13) |
---|---|---|
Age (years) | 10.47±2.53 | 15.75±11.23 |
Gender | ||
Male | 13 (86.67) | 8 (61.54) |
Female | 2 (13.33) | 5 (38.46) |
Affected side | ||
Left side | 5 (33.33) | 0 |
Right side | 10 (66.67) | 0 |
Both sides | 0 | 0 |
Grade of hearing loss | ||
Normal hearing | 1 (6.67) | 11 (84.61) |
Mild hearing loss | 1 (6.67) | 2 (15.38) |
Moderately severe hearing loss | 6 (40.00) | 0 |
Severe hearing loss | 7 (46.66) | 0 |
Data are expressed as mean ± standard deviation or n (%).
Analyses of characteristic metabolites to distinguish between microtia patients with low BMP5 expression and control groups
Through detection of non-targeted metabolite profiles on transfected 293T cell samples, we found 319 metabolites in total, in which TGs (25.705%), PCs (25.392%) and glycerophosphoethanolamines (PEs) (21.630%) accounted for the largest proportion (Figure 1A). According to the PCA analysis (Figure 1B), the QC samples were closely clustered together, confirming that the analytical data was reliable. We identified 190 differential metabolites between siBMP5-2 and siNC control groups, and 255 between siBMP5-3 and siNC groups (ANOVA test P<0.05). Discriminant analysis of the OPLS-DA model revealed distinctive clustering for siBMP5 and siNC groups (Figure S1A,S1B). The performance of OPLS-DA showed no overfitting (Q2 =0.71, R2Y =0.872 in siBMP5-2 vs. siNC groups, and Q2 =0.79, R2Y =0.995 in siBMP5-3 vs. siNC) (Figure S1C,S1D), evaluated by means of cross-validation and permutation test. These findings suggested that metabolites altered distinctly in microtia patients with low BMP5 expression compared to controls.
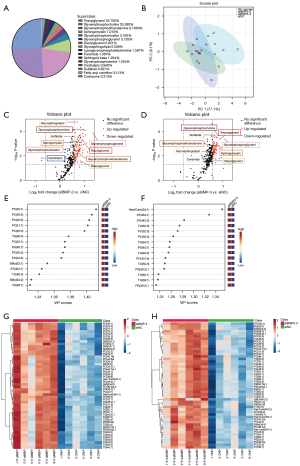
Comparison of characteristic metabolites between groups
We then found the visibly altered metabolites to discriminate between microtia patients and controls. There were 117 (with 115 enriched and 2 depleted metabolites) and 95 (all were enriched) significantly differential metabolites altogether in siBMP5-2 and siBMP5-3 versus the siNC group respectively (adjusted P<0.05 and |log2(FC)| >0.58), which was annotated according to metabolite chemical classes (Figure 1C,1D). These included 8 significantly upregulated metabolites of TG, PC, PE, sphingomyelin, glycerophosphoglycerol, diacylglycerol, glycosphingolipid, and sulfatide. The OPLS-DA model also provided the VIP score (Figure 1E,1F), showing the top 15 metabolites with the highest VIP scores and respective concentrations for each comparison, including TG, PC, PE, sphingomyelin, glycerophosphoglycerol, and glycosphingolipid. Hierarchical clustering heatmaps were constructed based on the differential metabolites correspondingly (P<0.05) (Figure 1G,1H). These results indicated that 8 of these metabolites may play a role in distinguishing between microtia patients with low BMP5 expression and controls.
Pathway analysis
To evaluate potentially altered metabolic pathways, we found that these differential metabolites were enriched in 6 Kyoto Encyclopedia of Genes and Genomes (KEGG) pathways in both groups in comparison, but only glycerophospholipid metabolism showed an important number of effective metabolites matching to this pathway (Tables 2,3 and Figure 2) via over-representation analysis. It displayed the greatest impact value (0.26 in Table 2, 0.20 in Table 3) through topology analysis.
Table 2
Pathway | Totala | Hitsb | Raw P valuec | Holm adjust P valued | Impact valuee |
---|---|---|---|---|---|
Glycerophospholipid metabolism | 36 | 4 | <0.001 | <0.001 | 0.26 |
Linoleic acid metabolism | 5 | 1 | 0.022 | 1.00 | <0.01 |
alpha-Linolenic acid metabolism | 13 | 1 | 0.057 | 1.00 | <0.01 |
GPI-anchor biosynthesis | 14 | 1 | 0.062 | 1.00 | <0.01 |
Glycerolipid metabolism | 16 | 1 | 0.070 | 1.00 | 0.01 |
Arachidonic acid metabolism | 36 | 1 | 0.152 | 1.00 | <0.01 |
a, total is the total numbers of compounds in the pathway. b, hits represents the number of metabolites that matched with the metabolites of the HMDB based on the accurate mass of each MF that differed significantly (adjusted P<0.05 and |log2(FC)| >0.58) in siBMP5 vs. siNC groups. c, the original P value calculated from the over-representation analysis. d, the P value adjusted by Holm-Bonferroni method. e, the impact value is calculated from the pathway topology analysis. GPI, glycosylphosphatidylinositol; HMDB, Human Metabolome Database; MF, metabolomic features.
Table 3
Pathway | Totala | Hitsb | Raw P valuec | Holm adjust P valued | Impact valuee |
---|---|---|---|---|---|
Glycerophospholipid metabolism | 36 | 2 | 0.003 | 0.26 | 0.20 |
Linoleic acid metabolism | 5 | 1 | 0.013 | 1.00 | <0.01 |
alpha-Linolenic acid metabolism | 13 | 1 | 0.033 | 1.00 | <0.01 |
Glycosylphosphatidylinositol (GPI)-anchor biosynthesis | 14 | 1 | 0.036 | 1.00 | <0.01 |
Glycerolipid metabolism | 16 | 1 | 0.041 | 1.00 | 0.01 |
Arachidonic acid metabolism | 36 | 1 | 0.090 | 1.00 | <0.01 |
a, total is the total numbers of compounds in the pathway. b, hits represents the number of metabolites that matched with the metabolites of the HMDB based on the accurate mass of each MF that differed significantly (adjusted P<0.05 and |log2(FC)| >0.58) in siBMP5 vs. siNC groups. c, the original P value calculated from the over-representation analysis. d, the P value adjusted by Holm-Bonferroni method. e, the impact value is calculated from the pathway topology analysis. GPI, glycosylphosphatidylinositol; HMDB, Human Metabolome Database; MF, metabolomic features.
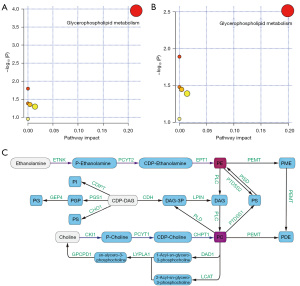
Patients with microtia have multiple metabolite abnormalities
In order to verify the abnormal metabolite changes found in the previous metabolomics, we used ELISA to analyze the contents of PC, TG, and choline in the serum of microtia and control groups. We detected PC (microtia: 41.20±18,06, NC: 25.23±6.922, P=0.0059, P<0.01), TG (microtia: 29.51±12.25, NC: 16.35±9.779, P=0.0045, P<0.01), choline (microtia: 234.1±33.26, NC: 202.3±43.64, P=0.0378, P<0.05) in the serum of participants, as shown in Figure 3A-3C. Then, we used these data from 28 participants to assess the diagnostic performance of 2 biomarkers (PC and TG) in a cell model. Significantly, the biomarker panel was able to distinguish patients with microtia from controls with an area under the curve (AUC) of 89.74% [95% confidence interval (CI): 78.29–100.00%], sensitivity of 93.33%, and specificity of 76.92% (Figure 3D), which confirmed that this biomarker panel was an efficient diagnostic approach of microtia.
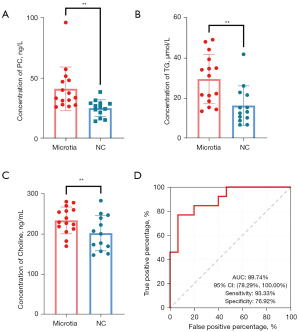
Discussion
Although microtia does not affect the patient’s survival, it can cause inconvenience to the patient’s life and psychology (40-43). Microtia is currently mostly diagnosed after birth. According to reports, although the ears of some patients with microtia can be restored to their normal shape after birth, most patients require surgical treatment (44-47), which is a hugely harmful intervention for patients. Microtia-based diagnostic assays, however, continue to face challenges in their development and clinical application. Commercially available molecular testing is still lacking. Although certain tests have demonstrated decent performance when analyzed on a single institutional cohort, variations in the performance of the majority of these tests have been noted when evaluated in multiple independent cohorts (48-51). Furthermore, these tests are very expensive and require tissue samples to be taken during surgery, which presents problems for both the economy and clinical practice. A recent study illustrated the importance of bioactive lipids in mesoderm differentiation (52). It can even be determined that metabolic abnormalities will affect the development of mesoderm (53,54). Since auricular cells originate from mesoderm parotid arch cells, the metabolic state caused by mesodermal cell changes can alter cell development in the form of metabolic reprogramming derivatives or secreted molecules (53,54). At the same time, the early detection of metabolic abnormalities is crucial to preventing microtia since metabolites are the products of biochemical reactions in the organ and the molecules that are closest to the phenotype. In our study, we discovered lots of different metabolites and 6 enriched pathways of metabolites through metabolomic analysis of cell samples. In addition, the corresponding abnormal metabolites were verified in the serum of patients with microtia and controls. We discovered that the combination of PC and TG provide an AUC of 89.74%, sensitivity of 93.33%, and specificity of 76.92%, that could distinguish microtia patients from controls. These findings may indicate that metabolites may be endogenously generated in microtia and may serve as biomarkers for identifying the underlying cause of microtia as well as for early detection and prevention of the condition during mesoderm development. The limitations of this study include the relatively small number of discovery samples, the lack of validation cohorts, and the temporary absence of metabolomic signatures during embryonic development. Further investigations are required to confirm the origin of circulating metabolites and to identify whether they have a crucial contribution in the development of microtia.
Protein, microRNA (miRNA) and other types of circulating diagnostic indicators such as messenger RNA (mRNA) are also suggested for distinguishing between microtia and healthy people (55-61). Studies have been conducted on the differences between the metabolites of auricle cartilage, but the changes in the metabolites of the auricle cannot indicate the occurrence of microtia (29). Since metabolites are the products of metabolic activities in the body, changes in the transcriptome (mRNA, miRNA), and proteome (proteins) due to pathophysiological modifications, might be reflected in the metabolome. Metabolites are the cornerstone of cell function. However, the field of metabolomics is still not mature, and it is easy to be interfered by upstream organisms (62,63). Although the diagnosis of congenital diseases such as microtia has obvious advantages, there are also shortcomings such as unstable results and difficult to obtain samples. This study has some limitations, such as small sample size and lack of functional students of key metadata. We will further increase the sample size and supplement the research of functional students of key metadata in the future. In addition, our metabolic biomarker panel demonstrated higher diagnostic performance in terms of both sensitivity and specificity, even before the auricle is produced, as compared to other types of circulating biomarkers.
Conclusions
In conclusion, by metabolomics profiling, we were able to determine which metabolites were significantly altered between patients with microtia and controls. We specifically showed that a newly developed panel of serum metabolic biomarkers could distinguish between patients with microtia and controls with high sensitivity and specificity, which was superior to other circulating biomarkers in the early diagnosis of microtia. Therefore, this study could provide a basis for a new metabolic biomarker panel for early diagnosis of microtia.
Acknowledgments
Funding: This work was supported by grants from the National Natural Science Foundation of China (Nos. 82171160, 81801132, and 81971190); the National Natural Science Foundation of Guangdong (No. 2019A1515010902); the Science and Technology Program of Guangzhou (No. 201903010075); the National Key R&D Program of China (No. 2020YFB020003); and GD Frontier & Key Tech Innovation Program (Nos. 2018B030337001 and 2019B020228001).
Footnote
Reporting Checklist: The authors have completed the MDAR reporting checklist Available at https://atm.amegroups.com/article/view/10.21037/atm-22-5614/rc
Data Sharing Statement: Available at https://atm.amegroups.com/article/view/10.21037/atm-22-5614/dss
Conflicts of Interest: All authors have completed the ICMJE uniform disclosure form (available at https://atm.amegroups.com/article/view/10.21037/atm-22-5614/coif). All authors report that the study was supported by grants from the National Natural Science Foundation of China (Nos. 82171160, 81801132, and 81971190); the National Natural Science Foundation of Guangdong (No. 2019A1515010902); the Science and Technology Program of Guangzhou (No. 201903010075); the National Key R&D Program of China (No. 2020YFB020003); and GD Frontier & Key Tech Innovation Program (Nos. 2018B030337001 and 2019B020228001). The authors have no other conflicts of interest to declare
Ethical Statement: The authors are accountable for all aspects of the work in ensuring that questions related to the accuracy or integrity of any part of the work are appropriately investigated and resolved. The study was conducted in accordance with the Declaration of Helsinki (as revised in 2013). Written informed consent was provided by all the participants and their parents or legal guardians for participation in and publication of this study. All procedures were approved by the Ethics Committee of Sun Yat-sen Memorial Hospital of Sun Yat-sen University (No. 2019-KY-024).
Open Access Statement: This is an Open Access article distributed in accordance with the Creative Commons Attribution-NonCommercial-NoDerivs 4.0 International License (CC BY-NC-ND 4.0), which permits the non-commercial replication and distribution of the article with the strict proviso that no changes or edits are made and the original work is properly cited (including links to both the formal publication through the relevant DOI and the license). See: https://creativecommons.org/licenses/by-nc-nd/4.0/.
References
- Mastroiacovo P, Corchia C, Botto LD, et al. Epidemiology and genetics of microtia-anotia: a registry based study on over one million births. J Med Genet 1995;32:453-7. [Crossref] [PubMed]
- Castilla EE, Orioli IM. Prevalence rates of microtia in South America. Int J Epidemiol 1986;15:364-8. [Crossref] [PubMed]
- Du J, Zhuang H, Chai J, et al. Psychological status of congenital microtia patients and relative influential factors: analysis of 410 cases. Zhonghua Yi Xue Za Zhi 2007;87:383-7.
- Shaw GM, Carmichael SL, Kaidarova Z, et al. Epidemiologic characteristics of anotia and microtia in California, 1989-1997. Birth Defects Res A Clin Mol Teratol 2004;70:472-5. [Crossref] [PubMed]
- Canfield MA, Langlois PH, Nguyen LM, et al. Epidemiologic features and clinical subgroups of anotia/microtia in Texas. Birth Defects Res A Clin Mol Teratol 2009;85:905-13. [Crossref] [PubMed]
- Forrester MB, Merz RD. Descriptive epidemiology of anotia and microtia, Hawaii, 1986-2002. Congenit Anom (Kyoto) 2005;45:119-24. [Crossref] [PubMed]
- Zhang HS, Chen SJ, Zeng HC, et al. Characteristics of 43 multiple auricular deformity case families and auricle morphology in 463 microtia patients in South China. Ann Transl Med 2020;8:496. [Crossref] [PubMed]
- Ma C, Shaw GM, Scheuerle AE, et al. Association of microtia with maternal nutrition. Birth Defects Res A Clin Mol Teratol 2012;94:1026-32. [Crossref] [PubMed]
- Browne ML, Hoyt AT, Feldkamp ML, et al. Maternal caffeine intake and risk of selected birth defects in the National Birth Defects Prevention Study. Birth Defects Res A Clin Mol Teratol 2011;91:93-101. [Crossref] [PubMed]
- Fan Y, Li Y, Chen Y, et al. Comprehensive Metabolomic Characterization of Coronary Artery Diseases. J Am Coll Cardiol 2016;68:1281-93. [Crossref] [PubMed]
- Yu S, Liu C, Hou Y, et al. Integrative metabolomic characterization identifies plasma metabolomic signature in the diagnosis of papillary thyroid cancer. Oncogene 2022;41:2422-30. [Crossref] [PubMed]
- Detour J, Bund C, Behr C, et al. Metabolomic characterization of human hippocampus from drug-resistant epilepsy with mesial temporal seizure. Epilepsia 2018;59:607-16. [Crossref] [PubMed]
- Blanco FJ, Ruiz-Romero C. Metabolomic characterization of metabolic phenotypes in OA. Nat Rev Rheumatol 2012;8:130-2. [Crossref] [PubMed]
- Wang LB, Karpova A, Gritsenko MA, et al. Proteogenomic and metabolomic characterization of human glioblastoma. Cancer Cell 2021;39:509-528.e20. [Crossref] [PubMed]
- Shen B, Yi X, Sun Y, et al. Proteomic and Metabolomic Characterization of COVID-19 Patient Sera. Cell 2020;182:59-72.e15. [Crossref] [PubMed]
- Yu M, Sun S, Yu J, et al. Discovery and Validation of Potential Serum Biomarkers for Pediatric Patients with Congenital Heart Diseases by Metabolomics. J Proteome Res 2018;17:3517-25. [Crossref] [PubMed]
- Troisi J, Cavallo P, Richards S, et al. Noninvasive screening for congenital heart defects using a serum metabolomics approach. Prenat Diagn 2021;41:743-53. [Crossref] [PubMed]
- Cedars A, Manlhiot C, Ko JM, et al. Metabolomic Profiling of Adults with Congenital Heart Disease. Metabolites 2021;11:525. [Crossref] [PubMed]
- Piersigilli F, Syed M, Lam TT, et al. An omic approach to congenital diaphragmatic hernia: a pilot study of genomic, microRNA, and metabolomic profiling. J Perinatol 2020;40:952-61. [Crossref] [PubMed]
- Chen X, Chen Y, Wang L, et al. Metabolomics of the aqueous humor in patients with primary congenital glaucoma. Mol Vis 2019;25:489-501.
- Qian G, Xu L, Qin J, et al. Leukocyte proteomics coupled with serum metabolomics identifies novel biomarkers and abnormal amino acid metabolism in Kawasaki disease. J Proteomics 2021;239:104183. [Crossref] [PubMed]
- Blazek AD, Nam J, Gupta R, et al. Exercise-driven metabolic pathways in healthy cartilage. Osteoarthritis Cartilage 2016;24:1210-22. [Crossref] [PubMed]
- Zhang S, Chuah SJ, Lai RC, et al. MSC exosomes mediate cartilage repair by enhancing proliferation, attenuating apoptosis and modulating immune reactivity. Biomaterials 2018;156:16-27. [Crossref] [PubMed]
- Arra M, Swarnkar G, Ke K, et al. LDHA-mediated ROS generation in chondrocytes is a potential therapeutic target for osteoarthritis. Nat Commun 2020;11:3427. [Crossref] [PubMed]
- López-Garrido L, Bañuelos-Hernández AE, Pérez-Hernández E, et al. Metabolic profiling of serum in patients with cartilage tumours using (1) H-NMR spectroscopy: A pilot study. Magn Reson Chem 2020;58:65-76. [Crossref] [PubMed]
- Silagi ES, Schipani E, Shapiro IM, et al. The role of HIF proteins in maintaining the metabolic health of the intervertebral disc. Nat Rev Rheumatol 2021;17:426-39. [Crossref] [PubMed]
- Zheng L, Zhang Z, Sheng P, et al. The role of metabolism in chondrocyte dysfunction and the progression of osteoarthritis. Ageing Res Rev 2021;66:101249. [Crossref] [PubMed]
- Mobasheri A, Rayman MP, Gualillo O, et al. The role of metabolism in the pathogenesis of osteoarthritis. Nat Rev Rheumatol 2017;13:302-11. [Crossref] [PubMed]
- Yang J, He L. Mass Spectrometric Characterization of Metabolites in Ear Cartilage. Ann Plas Surg 2020;85:76-82. [Crossref] [PubMed]
- Suutarla S, Rautio J, Ritvanen A, et al. Microtia in Finland: comparison of characteristics in different populations. Int J Pediatr Otorhinolaryngol 2007;71:1211-7. [Crossref] [PubMed]
- King JA, Marker PC, Seung KJ, et al. BMP5 and the molecular, skeletal, and soft-tissue alterations in short ear mice. Dev Biol 1994;166:112-22. [Crossref] [PubMed]
- Bailón-Plaza A, Lee AO, Veson EC, et al. BMP-5 deficiency alters chondrocytic activity in the mouse proximal tibial growth plate. Bone 1999;24:211-6. [Crossref] [PubMed]
- Mailhot G, Yang M, Mason-Savas A, et al. BMP-5 expression increases during chondrocyte differentiation in vivo and in vitro and promotes proliferation and cartilage matrix synthesis in primary chondrocyte cultures. J Cell Physiol 2008;214:56-64. [Crossref] [PubMed]
- Qiu YY, Zhang HS, Tang Y, et al. Mitochondrial dysfunction resulting from the down-regulation of bone morphogenetic protein 5 may cause microtia. Ann Transl Med 2021;9:418. [Crossref] [PubMed]
- Pang Z, Zhou G, Chong J, et al. Comprehensive Meta-Analysis of COVID-19 Global Metabolomics Datasets. Metabolites 2021;11:44. [Crossref] [PubMed]
- Pang Z, Chong J, Zhou G, et al. MetaboAnalyst 5.0: narrowing the gap between raw spectra and functional insights. Nucleic Acids Res 2021;49:W388-96. [Crossref] [PubMed]
- Liao W, Tan G, Zhu Z, et al. Combined metabonomic and quantitative real-time PCR analyses reveal systems metabolic changes in Jurkat T-cells treated with HIV-1 Tat protein. J Proteome Res 2012;11:5109-23. [Crossref] [PubMed]
- Wang X, Yang B, Sun H, et al. Pattern recognition approaches and computational systems tools for ultra performance liquid chromatography-mass spectrometry-based comprehensive metabolomic profiling and pathways analysis of biological data sets. Anal Chem 2012;84:428-39. [Crossref] [PubMed]
- Aittokallio T, Schwikowski B. Graph-based methods for analysing networks in cell biology. Brief Bioinform 2006;7:243-55. [Crossref] [PubMed]
- Bradford PS, Akinosun M, Danielson KK, et al. Child and Caregiver Perspectives Towards Facial Appearance in Children With Microtia With or Without Craniofacial Microsomia Using a Newly Developed Likert Scale. J Craniofac Surg 2020;31:1583-7. [Crossref] [PubMed]
- Johns AL, Im DD, Lewin SL. Early Familial Experiences With Microtia: Psychosocial Implications for Pediatric Providers. Clin Pediatr (Phila) 2018;57:775-82. [Crossref] [PubMed]
- Zhou Y, Li X, Zhang M, et al. Effect of Multimedia Health Education on Psychological Burden, Quality of Life Ability, and Self-Efficacy of Congenital Microtia. Comput Math Methods Med 2022;2022:1482865. [Crossref] [PubMed]
- Johns AL, Lucash RE, Im DD, et al. Pre and post-operative psychological functioning in younger and older children with microtia. J Plast Reconstr Aesthet Surg 2015;68:492-7. [Crossref] [PubMed]
- Zhang TY, Bulstrode N, Chang KW, et al. International Consensus Recommendations on Microtia, Aural Atresia and Functional Ear Reconstruction. J Int Adv Otol 2019;15:204-8. [Crossref] [PubMed]
- Boahene KD, Owusu JA. Advances in microtia reconstruction. Operative Techniques in Otolaryngology-Head and Neck Surgery 2017;28:133-136.
- Behar BJ, Mackay DR. Brent technique for microtia reconstruction. Operative Techniques in Otolaryngology-Head and Neck Surgery 2017;28:77-83.
- Justicz N, Dusseldorp JR, Shaye D. Firmin technique for microtia reconstruction. Operative Techniques in Otolaryngology-Head and Neck Surgery 2017;28:90-96.
- Nikiforova MN, Mercurio S, Wald AI, et al. Analytical performance of the ThyroSeq v3 genomic classifier for cancer diagnosis in thyroid nodules. Cancer 2018;124:1682-90. [Crossref] [PubMed]
- Steward DL, Carty SE, Sippel RS, et al. Performance of a Multigene Genomic Classifier in Thyroid Nodules With Indeterminate Cytology: A Prospective Blinded Multicenter Study. JAMA Oncol 2019;5:204-12. Correction in JAMA Oncol 2019;5:271. [Crossref] [PubMed]
- Klubo-Gwiezdzinska J, Wartofsky L. The Role of Molecular Diagnostics in the Management of Indeterminate Thyroid Nodules. J Clin Endocrinol Metab 2018;103:3507-10. [Crossref] [PubMed]
- Nishino M, Nikiforova M. Update on Molecular Testing for Cytologically Indeterminate Thyroid Nodules. Arch Pathol Lab Med 2018;142:446-57. [Crossref] [PubMed]
- Zhang B, He L, Liu Y, et al. Prostaglandin E(2) Is Required for BMP4-Induced Mesoderm Differentiation of Human Embryonic Stem Cells. Stem Cell Reports 2018;10:905-19. [Crossref] [PubMed]
- Song C, Xu F, Ren Z, et al. Elevated Exogenous Pyruvate Potentiates Mesodermal Differentiation through Metabolic Modulation and AMPK/mTOR Pathway in Human Embryonic Stem Cells. Stem Cell Reports 2019;13:338-51. [Crossref] [PubMed]
- Zhou Y, Fujisawa I, Ino K, et al. Metabolic suppression during mesodermal differentiation of embryonic stem cells identified by single-cell comprehensive gene expression analysis. Mol Biosyst 2015;11:2560-7. [Crossref] [PubMed]
- Wei G. Bioinformatics analysis of microRNA comprehensive regulatory network in congenital microtia. Int J Pediatr Otorhinolaryngol 2015;79:1727-31. [Crossref] [PubMed]
- Zhang L, Lin L, Song YP, et al. Differential expression of long noncoding RNAs in congenital microtia. Gene Expr Patterns 2017;25-26:131-41. [Crossref] [PubMed]
- Si N, Zhang Z, Meng X, et al. Generation of an induced pluripotent stem cell line from a congenital microtia patient with 4p16.1 microduplication involving the long-range enhancer of HMX1. Stem Cell Res 2021;53:102357. [Crossref] [PubMed]
- Guo P, Ji Z, Jiang H, et al. Identification of a novel CYP26A1 mutation in a Chinese family with congenital microtia. Int J Pediatr Otorhinolaryngol 2020;139:110488. [Crossref] [PubMed]
- Si N, Meng X, Lu X, et al. Identification of loss-of-function HOXA2 mutations in Chinese families with dominant bilateral microtia. Gene 2020;757:144945. [Crossref] [PubMed]
- Chen X, Xu Y, Li C, et al. Key Genes Identified in Nonsyndromic Microtia by the Analysis of Transcriptomics and Proteomics. ACS Omega 2022;7:16917-27. [Crossref] [PubMed]
- Li C, Hao S, Wang H, et al. MicroRNA expression profiling and target genes study in congenital microtia. Int J Pediatr Otorhinolaryngol 2013;77:483-7. [Crossref] [PubMed]
- Schrimpe-Rutledge AC, Codreanu SG, Sherrod SD, et al. Untargeted Metabolomics Strategies-Challenges and Emerging Directions. J Am Soc Mass Spectrom 2016;27:1897-905. [Crossref] [PubMed]
- Pang H, Jia W, Hu Z. Emerging Applications of Metabolomics in Clinical Pharmacology. Clin Pharmacol Ther 2019;106:544-56. [Crossref] [PubMed]
(English Language Editor: J. Jones)