DNAH7 mutations benefit colorectal cancer patients receiving immune checkpoint inhibitors
Highlight box
Key findings
• This study demonstrated the significance of DNAH7 as a risk factor and a prognostic feature in CRC, and that DNAH7 affects the clinical efficacy of ICIs by affecting the tumor immune microenvironment.
What is known and what is new?
• CRC is a malignant tumor with a high mortality rate. The advent of ICIs has brought satisfactory results in CRC treatment. However, only a small percentage of CRC patients benefit from ICIs.
• This study demonstrated the connection between ICIs and DNAH7 mutations in CRC.
What is the implication, and what should change now?
• This study revealed that DNAH7 may affect the clinical efficacy of ICIs by affecting the tumor immune microenvironment, and DNAH7 mutation may be used as a prognostic indicator and potential target for CRC treatment in clinical practice.
Introduction
Colorectal cancer (CRC) is a prevalent malignancy with nearly 1.4 million new cases each year globally (1). Despite medical advances, the efficacy of diagnosis and treatments remains unsatisfactory (2). In recent years, immunotherapy has been widely used with some success. While immune checkpoint inhibitors (ICIs) are effective in defective mismatch repair (MMR) or highly microsatellite unstable (MSI-H) metastatic CRC, it has not produced good results in patients with good MMR or microsatellite stable (MSS) tumors (3). The progressive accumulation of somatic mutations within cells can lead to the occurrence of CRC (4). Hence, it is important to determine the key somatic mutations of CRC, which may be important markers for the prognosis and prediction of CRC, or may be novel therapeutic targets. Specific mechanisms of DNA repair include polymerase proofreading, base excision repair, and MMR (5).
Axonemal dynein heavy chain 7 (DNAH7) is an inner arm component of human cilia, which is induced during ciliated cell differentiation. There are two kinds of cilia, one is called motile cilia and the other is called primary cilia. Motile cilia are responsible for cleaning, and their absence causes inflammation. As sensing organelles, primary cilia sense mechanical stress, optical, chemical and other signals from the extracellular environment. The abnormal expression of DNAH7 can cause the loss of cilia, and then become a pathogenic factor of tumors. The down-regulation of primary cilia expression is related to the occurrence of colorectal cancer, ovarian cancer, and kidney cancer. BRVF V600E is a gene closely related to the occurrence and development of CRC, which gene sequence has significant sequence homology with the region of a variety of axonal dynein heavy chain proteins, such as DNAH7. Therefore, DNAH7 may be closely related to the occurrence, development and prognosis of CRC. But the role of DNAH7 in CRC has not been thoroughly investigated. Therefore, we tried to research the role of DNAH7 in CRC.
This investigation explored the relationship between DNAH7 gene mutations and prognosis in CRC patients. Analysis of data from The Cancer Genome Atlas (TCGA) revealed that missense mutations were a major component of the overall mutations in CRC. Indeed, missense mutations were the main form of mutation observed in DNAH7. Patients were divided into two groups, one group with DNAH7 mutations and the other group with no DNAH7 mutations. The results showed that DNAH7 mutation is a risk factor for CRCs, and the mutation load and immunogenicity were higher in such patients. Moreover, functional enrichment analysis revealed the distinct pathway between CRC patients with DNAH7 mutations and those with unmutated DNAH7. Furthermore, CRC patients with the DNAH7 mutation benefited more from ICI therapy compared with patients who did not have the DNAH7 mutation. We present the following article in accordance with the TRIPOD reporting checklist (available at https://atm.amegroups.com/article/view/10.21037/atm-22-6166/rc).
Methods
The study was conducted in accordance with the Declaration of Helsinki (as revised in 2013).
Data download
The rectal adenocarcinoma (READ) and colonic adenocarcinoma (COAD) datasets, including 690 colorectal cancer (CRC) samples, and all corresponding clinical data, were obtained from The Cancer Genome Atlas (TCGA) database (http://gdc-portal.nci.nih.gov/). There was no record of survival time for 63 samples, 3 samples had no record of survival status, and 3 samples had no record of gender, and these samples were excluded. Finally, 582 CRC samples and 44 normal control samples were included for analysis. The data of “Masked Mutation” was used as the data of Somatic Mutation of CRCs. VarScan software (6) was used to preprocess the data. The somatic mutation data was visualized by R maftools package (7). The gene expression data (fragments per kilobase per million (FPKM) value) of the patient’s RNA sequencing were downloaded and converted into transcript per million (TPM) values.
Copy number alteration (CNV) analysis
The Masked Copy Number Segment data of patients were downloaded and the R TCGAbiolinks package was used to analyze the copy number alteration in the DNAH7 gene in CRC samples (8). GenePattern5, GISTIC2.0 analysis was performed on the downloaded CNV segments (9). The default settings were used for the GISTIC2.0 analysis excepted for a few parameters (e.g., confidence level of 0.99; X chromosomes not excluded before analysis). The R package maftools were used to visualized the results of the GISTIC2.0 analysis.
Calculation and correlation analysis of somatic mutation and tumor mutation burden (TMB) score
For each tumor sample, we detected the total number of somatic mutations in the tumor and translated it into the TMB of the sample. The TMB was defined as the number of coded, somatic, base replacement, and insert-deletion mutations per megabyte in the genome used non-synonymous and code transfer insertion deletion less than the 5% detection, obtained from the TCGA dataset. The TMB value was calculated for each sample and the Wilcoxon rank-sum test was used to compare the TMB between the DNAH7 mutated patients and DNAH7 unmutated patients.
Differentially expressed genes (DEGs) and clinical correlation analysis
To analyze the influence of DNAH7 mutation on the occurrence and development of CRC, samples were divided into a mutated group and an unmutated group according to the status of DNAH7 mutation. The overall differences between the two groups of samples were analyzed using principal component analysis (PCA) (10). The R DESeq2 package (11) was used to analyze the DEGs between the two groups, with log fold change (logFC) >1.0 and P<0.05 as the threshold. The results of the differential analysis are displayed in heatmaps and volcano plots.
Functional enrichment analysis and gene set enrichment analysis (GSEA)
Gene Ontology (GO) analysis, including molecular function, biological processes, and cellular components, was conducted for large-scale functional enrichment studies. Kyoto Encyclopedia of Genes and Genomes (KEGG) is a widely used database that stores information about biological pathways, genomes, diseases, and drugs. The ClusterProfiler R software package (12) was used to perform GO annotation analysis and KEGG pathway enrichment analysis of signature genes. A false discovery rate (FDR) <0.05 was considered statistically significant.
GSEA (13) was performed to distinguish the biological processes among different groups of CRC samples, and to explore whether a specific gene set was statistically different between two biological states (14). The MSIgDB database (https://www.gsea-msigdb.org/gsea/msigdb/) was accessed to download the “C2.cp.kegg.v6.2.-Symbols” gene set for GSEA, and an adjusted P value <0.05 was considered statistically significant.
Comparison of immune cell infiltration levels and immune correlation score between two groups
Based on gene expression profiles, ESTIMATE analysis was conducted to quantify immunocompetence (level of immune invasion) of the tumor sample. The immunoactivity and stroma score of each tumor was assessed using the R ESTIMATE package (15). The Mann-Whitney U test was used to compare the level of immune cell infiltration between the two groups of samples.
Screening of hub genes and construction of the protein-protein interaction (PPI) network
The PPI network of selected genes was constructed using the STRING online tool (16) and Cytoscape (V3.7.2) was used to visualize the network model (17). The genes with credibility score greater than 0.4 were selected. The Maximal Clique Centrality (MCC) algorithm was used to determine the nodes in the set in the common expression network, which is the most effective method (18). The Cytoscape cytoHubba plug-in was used to calculate the MCC of each node (18). The genes with the first 8 MCC values were selected as hub genes.
DNA damage related signaling pathway analysis
We evaluated and compared differences in enrichment fractions in DNA damage repair related pathways between DNAH7 mutated and unmutated groups in the CRC cohort. Among them, the gene set related to DNA damage repair pathway was obtained from the Broad Institute’s Molecular Signatures Database (MSigDB) (14).
Clinical prediction model validation of the DNAH7 gene mutation
Univariate and multivariate Cox analysis was used to examine the independent predictive ability of risk scores for overall survival (OS) in patients with the DNAH7 gene mutation combined with clinicopathological features. Subsequently, we constructed survival predictions in patients in the TCGA dataset using nomograms. The Harrell’s Consistency index (C-index) was measured to quantify differentiated performance. By comparing the predicted and observed actual survival rates of the nomogram, a calibration curve was obtained.
Statistical analysis
R software (version 4.0.2) was used for all statistical data analysis. The Mann-Whitney U test was used to compare continuous variables between two groups. The Wilcoxon rank-sum test was used to analysis differences in the non-normally distributed variables, and the independent Student t-test was used to analysis normally distributed variables. The Chi-square test or Fisher’s exact test was applied to compare and estimate the statistical significance between the two groups of categorical variables. Pearson correlation analysis was used to calculate the correlation coefficients of different genes. Survival analysis was performed using the R survival package, and survival differences were analyzed using the Kaplan-Meier survival curve. Differences in survival time between the two groups were evaluated using the log-rank test. Univariate and multivariate Cox analyses were used to determine independent prognostic factors. The receiver operating characteristic (ROC) curve was plotted using the R pROC package (19) and the area under the ROC curve (AUC) was calculated to assess the accuracy of the risk score in estimating prognosis. A P-value <0.05 was defined as statistically significant and all statistical P values were bilateral.
Results
Analysis of the overall degree of mutation in CRC patients
The CRC mutation data were downloaded from TCGA database to explore the frequency of the DNAH7 gene mutation. CRC mutations were predominantly missense mutations involving single nucleotide variants (SNVs), with C>T being the most common (Figure 1A,1B). Patients in the dataset were subsequently divided into a DNAH7 mutated group (Figure 1C) and a DNAH7 unmutated group (Figure 1D). The waterfall plots show the mutation level of related genes. Analysis of amino acid changes in the DNAH7 protein showed that the main mutated forms were missense mutation forms (Figure 1E).
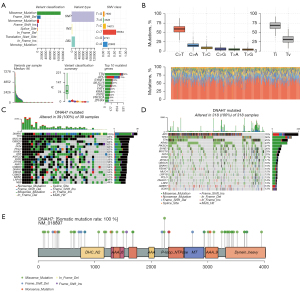
DNAH7 gene copy number alteration (CNV)
Figure 2A shows the CNV heat map of the DNAH7 mutant gene, in which the red color indicates an increase in CNV.
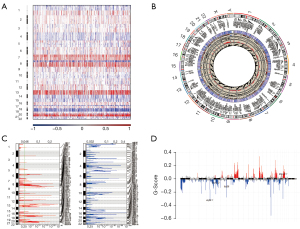
Figure 2B showed the circos diagram of the DNAH7 gene CNV of CRC. Visual analysis of the CNV results of GISTIC2.0 based on CRC queue (Figure 2C,2D) showed that CNV increased the most digits at 13P1.2 in the CRC DNAH7 mutation group, while CNV decreased the most multiples at 16p13.3.
Correlation parsing of biological features of DNAH7 gene mutation level
The expression of the DNAH7 gene was significantly different between the tumor group and the non-tumor group (P=0.003, Figure 3A). There was a significant increase in the TMB value (P<0.001, Figure 3B), and a non-significant difference in the MSI value (P=0.114, Figure 3C).
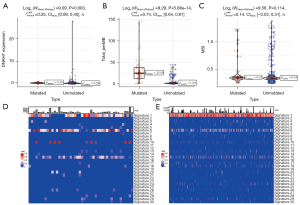
Subsequently, we explored the influence of DNAH7 mutation on different biological features. The results demonstrated that the DNAH7 mutated group was significantly different from the non-mutated group in signatures 1, 6, and 15 (Figure 3D,3E).
Drug susceptibility analysis and pathway analysis of the DNAH7 gene mutation
The influence of gene mutations on drug susceptibility was examined. Various drugs were relevant to DNAH7 gene mutation levels, in particular, the Druggable Genome (Figure 4A). Pathway analysis revealed that DNAH7 mutations were strongly associated with the RTK-RAS pathway and the NOTCH pathway in CRCs (Figure 4B), and related genes in the RTK-RAS pathway (Figure 4C) and NOTCH pathway (Figure 4D) had a high mutation rate in CRC samples.
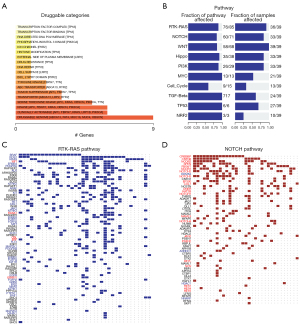
Differential gene expression in patients with DNAH7 mutations
To explore the influence of the DNAH7 gene mutation on the occurrence and development of CRC, patients in the TCGA dataset were divided into a mutated group and an unmutated group based on DNAH7 gene mutation. Differential expression gene analysis demonstrated that there were 1,764 significantly upregulation genes and 583 downregulation genes in the CRC datasets (Figure 5A,5B).
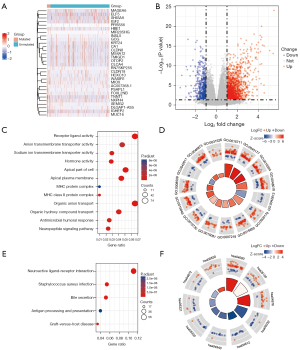
Functional enrichment analysis was performed and the GO results (Table 1) showed that the differentially expressed genes were strongly related to biological processes such as receptor ligand activity, apical part of the cell, and organic anion transport (Figure 5C,5D). KEGG analysis (Table 2) suggested that differentially expressed genes mainly affected pathways such as bile secretion and neuroactive ligand-receptor interaction (Figure 5E,5F).
Table 1
Ontology | ID | Description | P value | p.adjust | q value |
---|---|---|---|---|---|
BP | GO:0015711 | Organic anion transport | 8.02e–14 | 4.01e–10 | 3.68e–10 |
BP | GO:0015850 | Organic hydroxy compound transport | 1.63e–10 | 4.08e–07 | 3.74e–07 |
BP | GO:0007218 | Neuropeptide signaling pathway | 3.39e–10 | 5.65e–07 | 5.18e–07 |
BP | GO:0019730 | Antimicrobial humoral response | 6.06e–10 | 5.77e–07 | 5.29e–07 |
CC | GO:0045177 | Apical part of cell | 7.39e–12 | 3.60e–09 | 2.89e–09 |
CC | GO:0016324 | Apical plasma membrane | 3.38e–11 | 8.24e–09 | 6.62e–09 |
CC | GO:0042613 | MHC class II protein complex | 1.12e–10 | 1.81e–08 | 1.46e–08 |
CC | GO:0042611 | MHC protein complex | 6.89e–08 | 8.39e–06 | 6.75e–06 |
MF | GO:0048018 | Receptor ligand activity | 1.09e–11 | 9.09e–09 | 7.44e–09 |
MF | GO:0005179 | Hormone activity | 5.92e–11 | 2.47e–08 | 2.02e–08 |
MF | GO:0015081 | Sodium ion transmembrane transporter activity | 2.07e–09 | 5.74e–07 | 4.70e–07 |
MF | GO:0008509 | Anion transmembrane transporter activity | 9.74e–09 | 2.03e–06 | 1.66e–06 |
GO, gene ontology; DEGs, differentially expressed genes; BP, biological process; CC, cellular component; MF, molecular function.
Table 2
ID | Description | P value | p.adjust | q value |
---|---|---|---|---|
hsa04080 | Neuroactive ligand-receptor interaction | 1.22e–11 | 1.75e–09 | 1.39e–09 |
hsa05150 | Staphylococcus aureus infection | 1.46e–11 | 1.75e–09 | 1.39e–09 |
hsa04976 | Bile secretion | 1.79e–11 | 1.75e–09 | 1.39e–09 |
hsa05332 | Graft-versus-host disease | 1.55e–10 | 1.14e–08 | 9.05e–09 |
hsa04612 | Antigen processing and presentation | 3.78e–08 | 2.22e–06 | 1.77e–06 |
KEGG, Kyoto Encyclopedia of Genes and Genomes; DEGs, differentially expressed genes.
GSEA (Figure 6A-6F) revealed that pathways such as transport of small molecules, keratinization, asthma, autoimmune thyroid disease, allograft rejection, and others were significantly enriched in DNAH7 mutant tissues (Table 3).
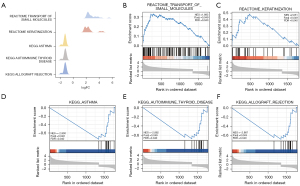
Table 3
ID | enrichmentScore | P value | p.adjust | q value |
---|---|---|---|---|
REACTOME_TRANSPORT_OF_SMALL_MOLECULES | 0.33217744 | 0.00104493 | 0.04933586 | 0.03994807 |
REACTOME_KERATINIZATION | 0.48623374 | 0.00120482 | 0.04933586 | 0.03994807 |
WP_ALLOGRAFT_REJECTION | −0.4744149 | 0.00537634 | 0.04933586 | 0.03994807 |
REACTOME_INTERFERON_SIGNALING | −0.75628843 | 0.00520833 | 0.04933586 | 0.03994807 |
KEGG_SYSTEMIC_LUPUS_ERYTHEMATOSUS | −0.40111294 | 0.0047619 | 0.04933586 | 0.03994807 |
KEGG_ANTIGEN_PROCESSING_AND_PRESENTATION | −0.71254582 | 0.0047619 | 0.04933586 | 0.03994807 |
KEGG_NATURAL_KILLER_CELL_MEDIATED_CYTOTOXICITY | −0.59114226 | 0.00454545 | 0.04933586 | 0.03994807 |
KEGG_GRAFT_VERSUS_HOST_DISEASE | −0.70690146 | 0.00454545 | 0.04933586 | 0.03994807 |
KEGG_LEISHMANIA_INFECTION | −0.75571668 | 0.00434783 | 0.04933586 | 0.03994807 |
KEGG_VIRAL_MYOCARDITIS | −0.60687597 | 0.00429185 | 0.04933586 | 0.03994807 |
REACTOME_INTERFERON_GAMMA_SIGNALING | −0.75529543 | 0.00429185 | 0.04933586 | 0.03994807 |
KEGG_TYPE_I_DIABETES_MELLITUS | −0.691749 | 0.00420168 | 0.04933586 | 0.03994807 |
REACTOME_MHC_CLASS_II_ANTIGEN_PRESENTATION | −0.75487465 | 0.00420168 | 0.04933586 | 0.03994807 |
WP_EBOLA_VIRUS_PATHWAY_ON_HOST | −0.6430013 | 0.00395257 | 0.04933586 | 0.03994807 |
KEGG_ALLOGRAFT_REJECTION | −0.68643059 | 0.00395257 | 0.04933586 | 0.03994807 |
KEGG_AUTOIMMUNE_THYROID_DISEASE | −0.68017463 | 0.003663 | 0.04933586 | 0.03994807 |
KEGG_ASTHMA | −0.75319622 | 0.003367 | 0.04933586 | 0.03994807 |
GSEA, gene set enrichment analysis.
Construction of the related regulatory network and the PPI network map
A PPI network of the DEGs was constructed using the STRING database and the interaction relationship between genes was imported into the Cytoscape software to obtain Figure 7A. The top ten genes from the PPI network were selected as hub genes via the Cytoscape’s cytoHubba plug-in and the MCC algorithm (Figure 7B). Figure 7C shows the co-expression heat map of the top10 key genes and DNAH7. Figure 7D shows the molecular correlation between the top10 key genes and DNAH7, among which, AQP8, MS4A12, GUCA2B, and ZG16 have the strongest correlation with DNAH7.
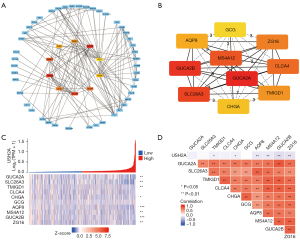
The effect of the DNAH7 gene mutation on immunological characteristics of colorectal cancer patients
We further investigated the influence of the DNAH7 gene mutation on the immunological characteristics of CRC. In the DNAH7 mutated group, the ESTIMATE scores, immune scores, and stromal scores were significantly increased (P<0.001; Figure 8A-8C), but tumor purity was significantly decreased (P<0.001; Figure 8D).
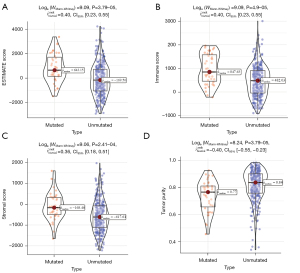
Correlation analysis of the DNAH7 gene mutation and clinical features
The clinical correlation of DNAH7 gene mutations was analyzed. According to the clinicopathological features, both univariate and multivariate Cox regression analyses demonstrated that the level of DNAH7 mutation was a hazard factor for CRC (P=0.014 and 0.007, and Figure 9A and Figure 9B, respectively) (Table 4). In the CRC data sets, DNAH7 mutation had a significant impact on patient prognosis (P=0.014, Figure 9C). To further clarify the influence of DNAH7 gene mutation on CRC, we incorporated the DNAH7 gene mutation and clinicopathological features into the model and constructed a nomogram (Figure 9D) to predict OS of CRC patients. The decision curve analysis (DCA) showed that the rosette map of DNAH7 gene mutation had a good prediction effect (Figure 9E). The calibration curve showed a good consistency between the estimated OS values and the actual observed values at 1-, 3- and 5-year through nomogram predicted survival probability (Figure 9F).
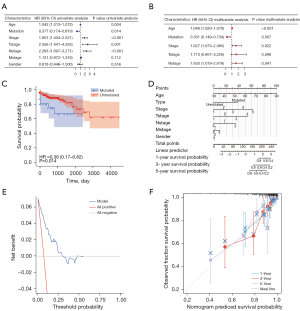
Table 4
Characteristics | Total (N) | Univariate analysis | Multivariate analysis | |||
---|---|---|---|---|---|---|
Hazard ratio (95% CI) | P value | Hazard ratio (95% CI) | P value | |||
Age | 314 | 1.042 (1.013–1.072) | 0.004* | 1.049 (1.020–1.079) | <0.001* | |
Type | 314 | – | – | – | – | |
Mutated | 33 | Reference | – | – | – | |
Unmutated | 281 | 0.377 (0.174–0.819) | 0.014* | 0.331 (0.149–0.734) | 0.007* | |
Stage | 314 | 1.901 (1.434–2.521) | <0.001* | 1.627 (1.073–2.465) | 0.022* | |
T stage | 314 | 2.556 (1.441–4.535) | 0.001* | 1.710 (0.907–3.226) | 0.098 | |
N stage | 314 | 2.293 (1.607–3.271) | <0.001* | 1.633 (1.019–2.616) | 0.041* | |
M stage | 314 | 1.131 (0.972–1.316) | 0.112 | – | – | |
Gender | 314 | 0.818 (0.446–1.500) | 0.516 | – | – |
*, P<0.05. OS, overall survival; TCGA, The Cancer Genome Atlas; CI, confidence interval; T, tumor; N, node; M, metastasis.
Discussion
CRC is the most common digestive system malignant tumor, with a high mortality rate. Although there are comprehensive treatment methods such as surgical operation, iatrochemistry, targeting, and so on, the efficacy is not ideal, and distant metastasis occurs frequently. The 5-year survival in patients with metastatic CRC is less than 20% (20).
Recently, studies have suggested that immunotherapy is beneficial to CRC because of the high immunogenicity conferred by the high mutational load of CRC. Immunotherapy has been shown to be effective in metastatic CRC with deficient MMR or high microsatellite instability (MSI-H) (3,21). Unfortunately, there is currently no effective predictive biomarker for the effectiveness of immunotherapy in CRC patients. Therefore, it is important to identify the key somatic mutations and improve the efficacy of immunotherapy. The clinical data and mutation data was obtained from a TCGA CRC cohort and examined. Survival analysis indicated that DNAH7 mutated patients had better immunotherapy prognosis compared to DNAH7 unmutated patients, suggesting that ICIs are effective in DNAH7 mutated CRC. We further demonstrated that DNAH7 likely affects the efficacy of ICIs by acting on the tumor immune microenvironment.
The study revealed that DNAH7 mutations were the major type of somatic mutations in CRC, with missense mutations being the most common. The DNAH7 mutated group had a greater increase in copy number alteration compared to the DNAH7 unmutated group, and the TMB level was significantly increased in DNAH7 mutated patients. Furthermore, significant changes were detected in the mutated DNAH7 gene at signature 1, 6, and 15 (Figure 3D,3E).
In addition, we demonstrated that various drugs were associated with the level of DNAH7 gene mutation, such as clinically actionable, kinase, serine threonine kinase, and especially the druggable genome (Figure 4A). Oncogenic signaling pathway analysis revealed that CRC samples with DNAH7 mutations had a high number of related genes that were mutated in the RTK-RAS pathway (Figure 4C) and NOTCH pathway (Figure 4D). RAS is a key protein in the regulation of cell proliferation and is commonly found in cancers. A previous study reported that the RTK-RAS pathway is related to the pathogenesis of CRC (22) and mutations in the RTK-RAS pathway led to decreased relapse free survival (RFS) in CRC patients (23). The Cancer Genome Atlas Pan-Cancer Analysis Project examined 123 patients with non-MSI, highly metastatic CRC, and found significant changes in the RTK-RAS, Notch, Wnt, p53, PI3K, TGF-β, and Myc pathways (24). These changes in the RTK-RAS and Notch pathway have been shown to be strongly related to poor OS in patients whose colorectal liver metastases were resected (25).
The effect of the DNAH7 gene mutation on the occurrence and development of tumors was examined using patients from the TCGA-CRC cohort. A total of 1,764 upregulation genes and 583 downregulation genes were identified in the CRC data sets (Figure 5A,5B). Additionally, GO analysis revealed that the differentially expressed genes were enriched in biological processes including receptor ligand activity, apical part of the cell, and organic anion transport (Figure 5C,5D). KEGG analysis showed that the differentially expressed genes prominently affected neuroactive ligand-receptor interaction, Staphylococcus aureus infection, bile secretion, and other pathways (Figure 5E,5F).
By establishing the PPI network and the interaction relationship, we identified the top 10 key genes, among which, AQP8, MS4A12, GUCA2B, and ZG16 have the strongest correlation with DNAH7. The expression of these latter genes is associated with the OS of CRC patients (26,27). The DNAH7 gene mutation, which was first mentioned in male infertility, causes asthenospermia syndrome and is a susceptibility gene for testicular cancer (28,29). Therefore, the DNAH7 gene may be a novel biological marker for CRC. Analysis of the effects of DNAH7 gene mutation on the immunological characteristics of CRC revealed that the ESTIMATE score, immune score, and stromal score were all elevated, while tumor purity decreased significantly in DNAH7 mutated CRC. Finally, both univariate and multivariate Cox regression analysis demonstrated that DNAH7 gene mutation level was a risk factor for CRC. A longer OS was observed in DNAH7 mutant patients than unmuntant DNAH7 patients. The OS benefit was approximately 131 months (about 3,932 days) in DNAH7 mutant patients. Therefore, DNAH7 mutation has good predictive value for the OS of CRC patients.
There were some limitations to this study. First, although we included two independent cohort studies with clinical and mutational data, there was a lack of relevant clinical studies. Second, while the functional pathways of the DNAH7 mutation were analyzed, further validation in vitro and in vivo is required. Third, there may have been batch differences that could not be avoided nor removed during analysis due to the large number of data sets.
Conclusions
The results herein demonstrated that CRC with DNAH7 mutations benefited more from ICI therapy than CRC without the DNAH7 mutation. Furthermore, the DNAH7 mutated group had a higher mutation load and immunogenicity compared to the unmutated group. Indeed, DNAH7 mutation may be a potential prognostic indicator and/or therapeutic target for the clinical treatment of CRC.
Acknowledgments
Funding: The study was supported by grants from the TCM Science and Technology Development Program of Jiangsu Province (Grant No. YB201957), the Natural Science Foundation of Jiangsu Province (Grant No. BK20190236), and the National Natural Science Foundation of China (Grant No. 82104956).
Footnote
Reporting Checklist: The authors have completed the TRIPOD reporting checklist. Available at https://atm.amegroups.com/article/view/10.21037/atm-22-6166/rc
Conflicts of Interest: All authors have completed the ICMJE uniform disclosure form (available at https://atm.amegroups.com/article/view/10.21037/atm-22-6166/coif). The authors have no conflicts of interest to declare.
Ethical Statement: The authors are accountable for all aspects of the work in ensuring that questions related to the accuracy or integrity of any part of the work are appropriately investigated and resolved. The study was conducted in accordance with the Declaration of Helsinki (as revised in 2013).
Open Access Statement: This is an Open Access article distributed in accordance with the Creative Commons Attribution-NonCommercial-NoDerivs 4.0 International License (CC BY-NC-ND 4.0), which permits the non-commercial replication and distribution of the article with the strict proviso that no changes or edits are made and the original work is properly cited (including links to both the formal publication through the relevant DOI and the license). See: https://creativecommons.org/licenses/by-nc-nd/4.0/.
References
- Lewandowska A, Rudzki G, Lewandowski T, et al. Risk Factors for the Diagnosis of Colorectal Cancer. Cancer Control 2022;29:10732748211056692. [Crossref] [PubMed]
- Manzoor S, Muhammad JS, Maghazachi AA, et al. Autophagy: A Versatile Player in the Progression of Colorectal Cancer and Drug Resistance. Front Oncol 2022;12:924290. [Crossref] [PubMed]
- Ciardiello D, Vitiello PP, Cardone C, et al. Immunotherapy of colorectal cancer: Challenges for therapeutic efficacy. Cancer Treat Rev 2019;76:22-32. [Crossref] [PubMed]
- Lei H, Tao K. Somatic mutations in colorectal cancer are associated with the epigenetic modifications. J Cell Mol Med 2020;24:11828-36. [Crossref] [PubMed]
- Díaz-Gay M, Alexandrov LB. Unraveling the genomic landscape of colorectal cancer through mutational signatures. Adv Cancer Res 2021;151:385-424. [Crossref] [PubMed]
- Koboldt DC, Zhang Q, Larson DE, et al. VarScan 2: somatic mutation and copy number alteration discovery in cancer by exome sequencing. Genome Res 2012;22:568-76. [Crossref] [PubMed]
- Mayakonda A, Lin DC, Assenov Y, et al. Maftools: efficient and comprehensive analysis of somatic variants in cancer. Genome Res 2018;28:1747-56. [Crossref] [PubMed]
- Colaprico A, Silva TC, Olsen C, et al. TCGAbiolinks: an R/Bioconductor package for integrative analysis of TCGA data. Nucleic Acids Res 2016;44:e71. [Crossref] [PubMed]
- Reich M, Liefeld T, Gould J, et al. GenePattern 2.0. Nat Genet 2006;38:500-1. [Crossref] [PubMed]
- Le S, Josse J, Husson F. FactoMineR: An R package for multivariate analysis. Journal of Statistical Software 2008;25:1-18. [Crossref]
- Love MI, Huber W, Anders S. Moderated estimation of fold change and dispersion for RNA-seq data with DESeq2. Genome Biol 2014;15:550. [Crossref] [PubMed]
- Yu G, Wang LG, Han Y, et al. clusterProfiler: an R package for comparing biological themes among gene clusters. OMICS 2012;16:284-7. [Crossref] [PubMed]
- Hänzelmann S, Castelo R, Guinney J. GSVA: gene set variation analysis for microarray and RNA-seq data. BMC Bioinformatics 2013;14:7. [Crossref] [PubMed]
- Subramanian A, Tamayo P, Mootha VK, et al. Gene set enrichment analysis: a knowledge-based approach for interpreting genome-wide expression profiles. Proc Natl Acad Sci U S A 2005;102:15545-50. [Crossref] [PubMed]
- Yoshihara K, Shahmoradgoli M, Martínez E, et al. Inferring tumour purity and stromal and immune cell admixture from expression data. Nat Commun 2013;4:2612. [Crossref] [PubMed]
- Szklarczyk D, Gable AL, Lyon D, et al. STRING v11: protein-protein association networks with increased coverage, supporting functional discovery in genome-wide experimental datasets. Nucleic Acids Res 2019;47:D607-13. [Crossref] [PubMed]
- Shannon P, Markiel A, Ozier O, et al. Cytoscape: a software environment for integrated models of biomolecular interaction networks. Genome Res 2003;13:2498-504. [Crossref] [PubMed]
- Chin CH, Chen SH, Wu HH, et al. cytoHubba: identifying hub objects and sub-networks from complex interactome. BMC Syst Biol 2014;8:S11. [Crossref] [PubMed]
- Robin X, Turck N, Hainard A, et al. pROC: an open-source package for R and S+ to analyze and compare ROC curves. BMC Bioinformatics 2011;12:77. [Crossref] [PubMed]
- Biller LH, Schrag D. Diagnosis and Treatment of Metastatic Colorectal Cancer: A Review. JAMA 2021;325:669-85. [Crossref] [PubMed]
- Le DT, Kim TW, Van Cutsem E, et al. Phase II Open-Label Study of Pembrolizumab in Treatment-Refractory, Microsatellite Instability-High/Mismatch Repair-Deficient Metastatic Colorectal Cancer: KEYNOTE-164. J Clin Oncol 2020;38:11-9. [Crossref] [PubMed]
- Löffler MW, Kowalewski DJ, Backert L, et al. Mapping the HLA Ligandome of Colorectal Cancer Reveals an Imprint of Malignant Cell Transformation. Cancer Res 2018;78:4627-41. [Crossref] [PubMed]
- Lee DW, Han SW, Cha Y, et al. Association between mutations of critical pathway genes and survival outcomes according to the tumor location in colorectal cancer. Cancer 2017;123:3513-23. [Crossref] [PubMed]
- Huang YH, Lin PC, Su WC, et al. Association between Altered Oncogenic Signaling Pathways and Overall Survival of Patients with Metastatic Colorectal Cancer. Diagnostics (Basel) 2021;11:2308. [Crossref] [PubMed]
- Kawaguchi Y, Kopetz S, Kwong L, et al. Genomic Sequencing and Insight into Clinical Heterogeneity and Prognostic Pathway Genes in Patients with Metastatic Colorectal Cancer. J Am Coll Surg 2021;233:272-284.e13. [Crossref] [PubMed]
- Ebadfardzadeh J, Kazemi M, Aghazadeh A, et al. Employing bioinformatics analysis to identify hub genes and microRNAs involved in colorectal cancer. Med Oncol 2021;38:114. [Crossref] [PubMed]
- Xu H, Ma Y, Zhang J, et al. Identification and Verification of Core Genes in Colorectal Cancer. Biomed Res Int 2020;2020:8082697. [Crossref] [PubMed]
- Wei X, Sha Y, Wei Z, et al. Bi-allelic mutations in DNAH7 cause asthenozoospermia by impairing the integrality of axoneme structure. Acta Biochim Biophys Sin (Shanghai) 2021;53:1300-9. [Crossref] [PubMed]
- Paumard-Hernández B, Calvete O, Inglada Pérez L, et al. Whole exome sequencing identifies PLEC, EXO5 and DNAH7 as novel susceptibility genes in testicular cancer. Int J Cancer 2018;143:1954-62. [Crossref] [PubMed]
(English Language Editor: J. Teoh)