Exploring the mechanisms underlying the therapeutic effect of the drug pair Rhubarb-Coptis in diabetic nephropathy using network pharmacology and molecular docking analysis
Highlight box
Key findings
• We found that quercetin, berberine, eupatin, epiberberine, and moupinamide can be defined as 5 crucial compounds. STAT3, CTNNB1, PIK3R1, PIK3CA, and TP53 may be the 5 most important therapeutic targets.
What is known and what is new?
• The pathogenesis of DN involves many factors such as abnormal glucose metabolism, oxidative stress, inflammation, and genetic factors, but the specific molecular mechanism is still unclear, and there is no effective treatment.
• The results of this study provide a reference for further exploring the mechanism of Rhubarb-Coptis in the treatment of DN.
What is the implication, and what should change now?
• The effective active components of Rhubarb-Coptis and related targets of DN were screened and predicted by network pharmacology, and we used the molecular docking technology to verify the binding of the selected active substances with the target sites, which provided a basis for the subsequent study of Rhubarb-Coptis and DN.
Introduction
Diabetic nephropathy (DN) is a serious microvascular complication of diabetes (1). On a global scale, the incidence of DN is on the rise in some regions (2), and it has become one of the main causes of renal failure. About 40% of patients with DN eventually progress to end-stage renal disease (ESRD) (3). The main clinical manifestations of DN are proteinuria, edema, and high blood pressure, and the histopathological manifestations are glomerular dilatation, glomerular basement membrane thickening, and podocyte injury, which eventually lead to glomerular sclerosis and tubular interstitial fibrosis, affecting renal function (4,5). The pathogenesis of DN involves many factors such as abnormal glucose metabolism, oxidative stress, inflammation, and genetic factors, but the specific molecular mechanism is still unclear, and there is no effective treatment.
In long-term clinical practice, traditional Chinese medicine (TCM) plays a role in treating DN through multiple approaches, targets, and mechanisms based on the principle of syndrome differentiation and combination of local and global factors. In particular, TCM is effective in regulating blood glucose and lipid metabolism, reducing kidney injury, and other aspects, with less adverse reactions. The medicinal pairing of Rhubarb and Coptis was recorded in the classic TCM text the Treatise on Cold Damage Diseases (Shanghan Lun), and has been used to treat diabetes and DN for more than 1,000 years. Modern pharmacological research displays that Coptis could protect the cardio-cerebrovascular system, regulate blood sugar level, and exert anti-inflammatory and anti-tumor effects (6). Rhubarb can regulate glucose and lipid metabolism, improve insulin resistance (7), inhibit the proliferation of mesangial cells (8), delay renal fibrosis (9), and improve oxidative stress, so as to reduce renal injury (10). However, the mechanism of action of Rhubarb-Coptis in the treatment of DN remains unclear.
Network pharmacology is a new discipline developed on the basis of systems biology theory, it constructs an interaction network of drug, target, pathway, and disease by abstracting the interaction relationship between drug and target into a network model. We could analyze the internal relationship between them by screening the key nodes. It is consistent with the overarching concept of TCM to study disease from a holistic perspective (11). In this paper, the effective active components of Rhubarb-Coptis and related targets of DN were screened and predicted by network pharmacology, and we used the molecular docking technology to verify the binding of the selected active substances with the target sites, which provided a basis for the subsequent study of Rhubarb-Coptis and DN. We present the following article in accordance with the STREGA reporting checklist (available at https://atm.amegroups.com/article/view/10.21037/atm-22-5550/rc).
Methods
Screening of active components of Rhubarb-Coptis
The study was conducted in accordance with the Declaration of Helsinki (as revised in 2013).
The Traditional Chinese Medicine System Pharmacology Database (TCMSP; https://www.tcmsp-e.com/tcmsp.php) is a database of systems pharmacology for drug discovery from herbal medicines (12). Based on the pharmacokinetic parameters: absorption, distribution, metabolism, excretion (ADME), oral bioavailability (OB) ≥30%, and drug-likeness (DL) ≥0.18, compounds were selected. Active compounds with no information on potential targets were excluded.
Screening of drug targets
Targets of potential active components Rhubarb-Coptis were obtained from TCMSP and SwissTargetPrediction databases (http://www.swisstargetprediction.ch/). Then, the UniProtKB search function in the UniProt protein database (https://www.uniprot.org/) was used to standardize genes for related targets with species set as “human” and status set as “reviewed” (13). Compound targets retrieved by TCMSP and SwissTargetPrediction were combined and duplicated genes were deleted to obtain drug target genes.
Determination of DN targets
In order to obtain relevant targets for DN, “diabetic nephropathy” and “diabetic kidney disease” were set as keywords to search 3 disease databases: Online Mendelian Inheritance in Man (OMIM; https://omim.org/) (14), GeneCards (https://www.genecards.org/) (15), and Therapeutic Target Database (TTD) (http://db.idrblab.net/ttd/) (16). The target genes were combined, and duplicated data were removed to obtain candidate targets for the treatment of DN.
Determination of DN targets of the active components
Screened targets of the active components of Rhubarb-Coptis and DN targets were imported into a Venn diagram webtool (http://bioinformatics.psb.ugent.be/webtools/Venn/) for analysis, and the intersection was taken to obtain the potential targets of Rhubarb-Coptis in the treatment of DN.
Construction of a network of drug-components-target-disease
In order to construct the network of drug-components-target-disease, we used Cytoscape 3.8.2 software (https://cytoscape.org/) to ligate active components and their associated targets. And then, we used Network Analyzer for topological analysis to highlight the key active components and targets of Rhubarb-Coptis in the treatment of DN.
Construction of a protein-protein interaction network
For the purpose of studying the interactions between the active components of Rhubarb-Coptis and their target proteins, we used the interaction database platform Search Tool for the Retrieval of Interacting Genes/Proteins (STRING) v.11.0 (https://string-db.org/) (17) to search for drug-disease intersection target genes, and a protein-protein interaction (PPI) network was constructed. STRING is a multifunctional data platform that can provide PPI evaluation and integration (18). In the database search, the protein species was set as “Homo sapiens”, the minimum interaction threshold was 0.9, and isolated nodes were removed to construct a PPI network. After that, Cytoscape 3.8.2 software was used to visualize the interaction network.
Statistical analysis
Gene Ontology (GO) biological process enrichment analysis
The Database for Annotation, Visualization, and Integrated Discovery (DAVID; https://david.ncifcrf.gov/) is a comprehensive functional annotation tool that can be used not only for gene differential analysis, but also for pathway enrichment (19,20). The common targets of Rhubarb-Coptis and DN were analyzed by GO enrichment analysis through the DAVID database, and the main biological functions of Rhubarb-Coptis in the treatment of DN were analyzed. The enrichment results were visualized by R language, and the results were expressed as bubble charts.
Kyoto Encyclopedia of Genes and Genomes (KEGG) pathway enrichment analysis
The common targets of Rhubarb-Coptis and DN were imported into R language (The R Foundation for Statistical Computing, Vienna, Austria) to obtain the enrichment results of KEGG pathways, and the key pathways were analyzed with the help of R language.
Molecular docking
(I) Design and processing of small molecules: the 3-dimensional (3D) structure of small molecule MOL 2 format was downloaded from TCMSP database according to the MOL ID number of small molecule, and ChemBio3D Ultra 14.0 (PerkinElmer Informatics, Waltham, MA, USA) was imported for energy minimization. The Minimum RMS Gradient was set to 0.001, and the small molecule was saved as MOL 2 format. The optimized small molecules were imported into AutoDockTools-1.5.6 for hydrogenation, charge calculation, charge distribution, and rotatable bond setting, and then saved as “pdbqt” format. (II) Preparation and handling of proteins: The protein structure of the target was downloaded from the Protein Data Bank (PDB) database. Pymol 2.3.0 (https://pymol.org/2/) was used to remove the protein crystal water and original ligand, and the protein structure was imported into AutoDockTools-1.5.6 for hydrogenation, charge calculation, charge distribution, atom type designation, and was saved as “pdbqt” format. (III) Autodock Vina V1.1.2 software (https://vina.scripps.edu/) was used for molecular docking to evaluate the affinity of the compounds with the target proteins. Further, PyMOL2.3.0 and LIGPLOT V 2.2.4 were used to analyze the interaction mode of the docking results.
Results
Screening of active components of Rhubarb-Coptis
“Rhubarb” and “Coptis” were entered into TCMSP database for retrieval. A total of 140 active components were retrieved, including 92 components of Rhubarb and 48 components of Coptis. Based on ADME, with OB ≥30% and DL ≥0.18 as screening conditions, 30 active ingredients were screened, including 16 of Rhubarb and 14 of Coptis (Table 1).
Table 1
Mol ID | Compound | Drug | OB (%) | DL |
---|---|---|---|---|
MOL002235 | EUPATIN | Rhubarb | 50.8 | 0.41 |
MOL002251 | Mutatochrome | Rhubarb | 48.64 | 0.61 |
MOL002259 | Physciondiglucoside | Rhubarb | 41.65 | 0.63 |
MOL002260 | Procyanidin B-5,3'-O-gallate | Rhubarb | 31.99 | 0.32 |
MOL002268 | Rhein | Rhubarb | 47.07 | 0.28 |
MOL002276 | Sennoside E_qt | Rhubarb | 50.69 | 0.61 |
MOL002280 | Torachrysone-8-O-beta-D-(6'-oxayl)-glucoside | Rhubarb | 43.02 | 0.74 |
MOL002281 | Toralactone | Rhubarb | 46.46 | 0.24 |
MOL002288 | Emodin-1-O-beta-D-glucopyranoside | Rhubarb | 44.81 | 0.8 |
MOL002293 | Sennoside D_qt | Rhubarb | 61.06 | 0.61 |
MOL002297 | Daucosterol_qt | Rhubarb | 35.89 | 0.7 |
MOL002303 | Palmidin A | Rhubarb | 32.45 | 0.65 |
MOL000358 | Beta-sitosterol | Rhubarb | 36.91 | 0.75 |
MOL000471 | Aloe-emodin | Rhubarb | 83.38 | 0.24 |
MOL000554 | gallic acid-3-O-(6'-O-galloyl)-glucoside | Rhubarb | 30.25 | 0.67 |
MOL000096 | (-)-catechin | Rhubarb | 49.68 | 0.24 |
MOL001454 | Berberine | Coptis | 36.86 | 0.78 |
MOL013352 | Obacunone | Coptis | 43.29 | 0.77 |
MOL002894 | Berberrubine | Coptis | 35.74 | 0.73 |
MOL002897 | Epiberberine | Coptis | 43.09 | 0.78 |
MOL002903 | (R)-Canadine | Coptis | 55.37 | 0.77 |
MOL002904 | Berlambine | Coptis | 36.68 | 0.82 |
MOL002907 | Corchoroside A_qt | Coptis | 104.95 | 0.78 |
MOL000622 | Magnograndiolide | Coptis | 63.71 | 0.19 |
MOL000762 | Palmidin A | Coptis | 35.36 | 0.65 |
MOL000785 | Palmatine | Coptis | 64.6 | 0.65 |
MOL000098 | Quercetin | Coptis | 46.43 | 0.28 |
MOL001458 | Coptisine | Coptis | 30.67 | 0.86 |
MOL002668 | Worenine | Coptis | 45.83 | 0.87 |
MOL008647 | Moupinamide | Coptis | 86.71 | 0.26 |
OB, oral bioavailability; DL, drug-likeness.
Determination of targets of Rhubarb-Coptis active components and active component-target network construction
The TCMSP database and SwissTargetPrediction database were used to obtain 806 targets of active components of Rhubarb and Coptis including 312 targets of Rhubarb and 494 targets of Coptis, and 609 potential targets of the drug were finally obtained by deleting repetitive targets and eliminating inactive targets. This was imported into The UniProt database for gene name standardization, and then Cytoscape 3.8.2 software was used to construct the “Rhubarb, Coptis - active ingredient-target” network diagram (Figure 1).
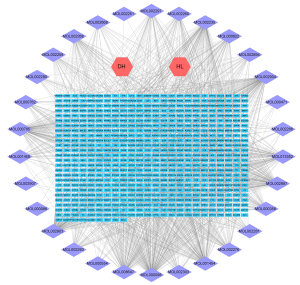
Prediction results of targets related to DN
We used “Diabetic kidney disease” and “Diabetic nephropathy” as the key words. The OMIM, GeneCards, and TTD databases were searched, and 1,107 disease targets were obtained after the deduplication.
Drug-disease intersection targets
Venn analysis was performed on 609 targets of active components of Rhubarb-Coptis and 1,107 targets related to diabetic nephropathy. After intersection of the two, 98 drug-disease cross targets were obtained, and Venn diagram was drawn (Figure 2).
Construction and analysis of drug-component-target-disease network
A total of 30 potential active components of Rhubarb-Coptis and 98 common drug-disease targets were input into Cytoscape software, and isolated components that had no intersection with the targets were deleted to draw the “drug-ingredient-target-disease” network diagram (Figure 3). In the figure, yellow represents drugs, purple represents 28 active components in Rhubarb-Coptis, 2 active component targets have no intersection with disease targets and are deleted, blue represents 98 common targets, and red represents disease.
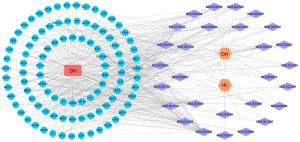
PPI network construction and topology analysis
A total of 98 common drug-disease targets were entered into the STRING database for retrieval, and a PPI network of protein interaction was constructed. The network had 98 nodes and 326 edges, and the average node degree was 6.65, with the PPI enrichment P value of <1.0e-1.6 (Figure 4A). The results of STRING analysis were imported into Cytoscape 3.8.2 software, and Network Analyzer was used for topology analysis. The node size was positively correlated with the degree value, the greater the degree of nodes, the more biological functions the node had in the network. The network was constructed as shown in Figure 4B. The dark and large nodes in the figure are the core targets. The first 20 targets were selected as the key targets of Rhubarb-Coptis compatibility in the treatment of DN, as shown in Table 2 and Figure 5. The 10 most-connected targets were signal transducer and activator of transcription 3 (STAT3), Catenin beta-1 (CTNNB1), phosphatidylinositol 3-kinase regulatory subunit alpha (PIK3R1), Phosphatidylinositol 4,5-bisphosphate 3-kinase catalytic subunit alpha isoform (PIK3CA), tumor protein p53 (TP53), AKT serine/threonine kinase 1 (AKT1), epidermal growth factor receptor (EGFR), mitogen-activated protein kinase 1 (MAPK1), histone acetyltransferase p300 (EP300), and vascular endothelial growth factor A (VEGFA), indicating their importance in the network.
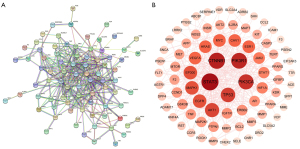
Table 2
Gene | Degree | Closeness | Betweenness |
---|---|---|---|
STAT3 | 31 | 0.558 | 0.177 |
CTNNB1 | 28 | 0.527 | 0.114 |
PIK3R1 | 27 | 0.524 | 0.048 |
PIK3CA | 25 | 0.517 | 0.040 |
TP53 | 23 | 0.510 | 0.113 |
AKT1 | 22 | 0.513 | 0.035 |
EP300 | 20 | 0.524 | 0.093 |
EGFR | 20 | 0.510 | 0.033 |
MAPK1 | 20 | 0.524 | 0.084 |
CAV1 | 17 | 0.484 | 0.100 |
ESR1 | 17 | 0.510 | 0.012 |
MYC | 17 | 0.503 | 0.036 |
HRAS | 17 | 0.470 | 0.034 |
VEGFA | 17 | 0.472 | 0.036 |
JAK2 | 14 | 0.456 | 0.029 |
STAT1 | 13 | 0.484 | 0.010 |
HIF1A | 13 | 0.490 | 0.030 |
ERBB2 | 11 | 0.453 | 0.008 |
AR | 11 | 0.481 | 0.006 |
IGF1R | 11 | 0.470 | 0.005 |
GO functional analysis
A total of 860 GO items were obtained by GO enrichment analysis of 98 common targets using DAVID database and R language, including 673 biological processes (BP), 84 cellular components (CC), and 103 molecular functions (MF). In order to understand the gene function more intuitively, the P value was used as the reference for sorting, and the top 10 items were selected for each part to generate a bubble chart (Figure 6).
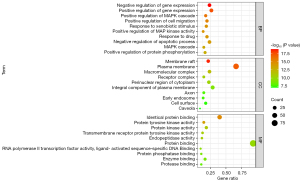
The BP terms mainly involved in the regulation of gene expression, the positive regulation of MAPK cascade, the positive regulation of cell migration, the positive regulation of cell migration, response to xenobiotic stimulus, positive regulation of MAP kinase activity, and response to drugs. The CC terms were mainly enriched in membrane raft, plasma membrane, macromolecular complex, receptor complex, and perinuclear region of cytoplasm. The MF terms mainly involved identical protein binding, protein tyrosine kinase activity, protein kinase activity, transmembrane receptor protein tyrosine kinase activity, and endopeptidase activity.
KEGG pathway enrichment analysis
In order to reveal the potential mechanism of Rhubarb-Coptis in the treatment of DN, we used the DAVID database and R language to conduct KEGG enrichment analysis on 98 targets of drug-diabetic nephropathy, and a total of 151 KEGG pathways were obtained. The top 20 pathways ranked by P value were selected to draw a bar bubble chart of KEGG enrichment (Figure 7). The −log10(P-value) represents the significance of enrichment, and the redder the color, the higher the significance.
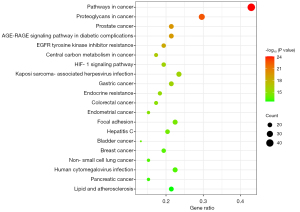
The results showed that the anterior pathways involved in the treatment of DN by Rhubarb-Coptis were mainly involved in tumor signaling pathway and AGE-RAGE signaling pathway, HIF-1 signaling pathway, and endocrine resistance signaling pathway in diabetes and diabetic complications.
Molecular docking
In order to validate the study results of network analysis, the top 5 active components and the top 5 potential targets of Rhubarb-Coptis were selected. The molecular docking was performed by AutoDock software, and the visualization was performed by PyMOL software. Generally, the lower the binding energy of small molecule ligands and protein receptors, the more stable the binding molecules are, indicating the greater possibility of interaction between molecules. The binding energy <−5 kcal/mol indicates that the small molecule binds well to the large molecule, and the smaller the value, the more likely it is to bind spontaneously (21). Through docking simulations, 25 pairs of docking results were yielded (Table 3). The results showed that quercetion, epiberberine, berberine, moupinamide, and eupatin were associated with CTNNB1, PIK3CA, PIK3R1, STAT3, and TP53 combined energies were all <−5 kcal/mol, indicating good molecular docking. The docking results of PIK3CA with quercetin, berberine, epiberberine, and moupinamide with the strongest binding force were selected and displayed (Figure 8). The binding energy of quercetin with PIK3CA was the lowest (−9.2 kcal/mol), the binding energy of quercetin with berberine was −8.9 kcal/mol, and the binding energy of quercetin with epirberine and moupinamide was −8.5 kcal/mol. The above molecular docking results indicate that their combination may have an essential role in treating DN with Rhubarb-Coptis.
Table 3
PDB ID | Key targets | Compounds | Binding energy (kcal/mol) |
---|---|---|---|
4JPS | PIK3CA | Quercetin | −9.2 |
4JPS | PIK3CA | Berberine | −8.9 |
4JPS | PIK3CA | Epiberberine | −8.5 |
4JPS | PIK3CA | Moupinamide | −8.5 |
4JPS | PIK3CA | EUPATIN | −8.0 |
6NJS | STAT3 | Berberine | −7.5 |
1UOL | TP53 | Berberine | −7.4 |
6NJS | STAT3 | Epiberberine | −7.4 |
1UOL | TP53 | Moupinamide | −6.9 |
6NJS | STAT3 | EUPATIN | −6.8 |
1UOL | TP53 | Epiberberine | −6.8 |
1JDH | CTNNB1 | Quercetin | −6.6 |
1JDH | CTNNB1 | Berberine | −6.6 |
1JDH | CTNNB1 | EUPATIN | −6.6 |
1UOL | TP53 | Quercetin | −6.5 |
1JDH | CTNNB1 | Epiberberine | −6.5 |
6NJS | STAT3 | Moupinamide | −6.4 |
1JDH | CTNNB1 | Moupinamide | −6.3 |
1UOL | TP53 | EUPATIN | −6.3 |
6NJS | STAT3 | Quercetin | −6.2 |
5M6U | PIK3R1 | Berberine | −6.1 |
5M6U | PIK3R1 | Epiberberine | −6.1 |
5M6U | PIK3R1 | Moupinamide | −6.1 |
5M6U | PIK3R1 | Quercetin | −5.5 |
5M6U | PIK3R1 | EUPATIN | −5.2 |
PDB, Protein Data Bank.
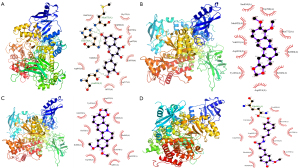
Discussion
DN is considered one of the 4 major fatal chronic diseases in the world (22), which seriously threatens the health and life of diabetic patients. However, because the pathogenesis of DN is still unclear, most patients with DN cannot get achieve a good treatment effect. A growing body of evidence shows that TCM has sufficient advantages in the treatment of diabetes and its complications. However, due to the multi-approach, multi-target, and multi-mechanism characteristics of TCM, its specific mechanism of action is still unclear. Network pharmacology can systematically combine network analysis with pharmacology to illustrate the interactions between diseases, drugs, and targets. For example, in the treatment of COVID-19, aiming at ACE/AngII/AT1R pathway, the network pharmacology method was used to explore effective traditional Chinese medicine and its active compounds, providing reference for further exploring effective traditional Chinese medicine and its mechanism of action in the treatment of COVID-19 (23). Therefore, the use of network pharmacology can combine the holistic view of traditional Chinese medicine and network information, and can more directly clarify the mechanism of Rhubarb-Coptis in treating DN.
In the present study, quercetin, berberine, eupatin, epiberberine, and moupinamide were defined as the crucial compounds of Rhubarb-Coptis in treating DN. It is reported that quercetin can fight inflammation, reduce oxidative stress injury of the kidneys, improve renal morphology, reduce renal pathological injury, and delay the progression of DN (24,25). Berberine can accelerate the secretion of antioxidant molecule Nrf2 and promote the synthesis of superoxide dismutase (SOD) by upregulating the KEAP1-NRF2/ARE signaling pathway, so as to reduce the levels of oxidative stress, inflammation, and fibrosis in the kidneys of DN rats (26). Epimberine can reduce the expression of TGF-β1 and Smad2, alleviate diabetes-related metabolic disorders, improve renal function, and alleviate renal tissue fibrosis (27). Eupatin has a good anti-inflammatory effect (28). Moupinamide is closely related to α-glucosidase inhibitory activity and free radical scavenging activity (29). These major compounds of Rhubarb-Coptis have the functions of regulating glucose and lipid metabolism, antioxidant, anti-inflammatory, and anti-fibrosis, which comprise the pharmacological basis of Rhubarb-Coptis in the treatment of DN.
Through the PPI network analysis, STAT3, CTNNB1, PIK3R1, PIK3CA, TP53, AKT1, EGFR, MAPK1, EP300, and VEGFA were the key targets of Rhubarb-Coptis in treating DN. These targets are mainly related to inflammation, oxidative stress, blood lipids, and vascular permeability, which are in line with the pathogenesis of DN to a certain extent. Study has shown that (30) STAT3 plays an important role in the occurrence and development of DN, and is widely involved in cell proliferation, differentiation, inflammation, and other biological effects. Under chronic hyperglycemia, the expression of STAT3 is increased, which promotes inflammation and apoptosis, leading to DN. The rapid accumulation of CTNNB1 in renal tubular epithelial cells promotes the formation of renal mesenchymal fibroblasts and benign proliferation of other renal mesenchymal epithelial cells, aggravating renal mesenchymal fibrosis (31). PIK3R1 and PIK3CA are members of the phosphatidylinositol-3-kinase (PI3K) protein family, including PI3K regulatory subunit and catalytic subunit, respectively. Studies have shown that the activation of PI3K pathway can promote the proliferation of mesangial cells, mediate podocyte injury, and accelerate the progression of DN (32,33). TP53 is a tumor suppressor that induces TGF-β1 signaling, and renal cells produce fibrotic extracellular matrix proteins, leading to glomerulosclerosis and tubulointerstitial fibrosis (34).
The GO functional enrichment analysis of 98 common targets related to Rhubarb-Coptis active ingredients in the treatment of DN was conducted. As shown in Figure 6, the top 10 most meaningful BP terms were mainly related to oxidative stress, apoptosis, and gene regulation. Studies have shown that the occurrence and development of DN are closely related to oxidative stress, apoptosis, and gene regulation (35,36). The MF terms were mainly included identical protein binding, protein kinase activity, endopeptidase activity, transcription factor binding, and protein kinase binding, and the main targets involved include STAT3, PIK3R1, VEGFA, TP53, and MAPK1, among others, which are mainly involved in inflammation, oxidative stress, and apoptosis. Both play an important role in the development of DN. In addition, CC terms were mainly enriched in membrane rafts, plasma membrane, macromolecular complex, receptor complex, and the perinuclear region of cytoplasm; the key targets STAT3, PIK3R1, CTNNB1, and VEGFA are included in these terms. In conclusion, the pathological mechanisms of DN are complex and diverse. Rhubarb-Coptis can treat DN by regulating various molecular functions and cellular components.
For the purpose of exploring the potential mechanism of Rhubarb-Coptis in the treatment of DN, we conducted KEGG enrichment analysis on 98 potential targets of Rhubarb-Coptis in DN. As shown in Figure 7, we found that the main pathways of Rhubarb-Coptis in the treatment of DN may be the AGE-RAGE signaling pathway and HIF-1 signaling pathway, which mainly involve 2 aspects: (I) accumulation of advanced glycation end-products: The metabolism of AGEs is accelerated in high glucose environment, and its accumulation in the kidney is the main cause of progressive renal damage and the key factor leading to DN. The toxic effects of AGEs, on the one hand, can directly destroy renal tissues and cells, and on the other hand, they can specifically bind to RAGE and activate a series of downstream signaling pathways, which can cause inflammation, oxidative stress, and apoptosis leading to renal injury. Studies have shown that the AGE-RAGE signaling pathway can activate NADPH oxidase to cause a large amount of reactive oxygen species (ROS) production, induce persistent oxidative stress in vascular tissues (37), and further activate the JAK/STAT, AKT, mTOR, MAPK, and other signaling pathways to phosphorylate and activate NF-κB. This causes the expression and release of a large number of adhesion factors, transforming growth factors, proinflammatory cytokines, increase vascular permeability, such as MCP-1, TGF-β1, VEGF, and TNF-α, increases proteinuria, and promotes renal fibrosis, leading to the occurrence and development of DN (38,39). (II) Dysregulation of oxygen metabolism: studies have shown that chronic hypoxia is an important pathogenic mechanism and potential initiating factor of the occurrence and development of DN (40). The stability of HIF-1α plays a key role in the activity of HIF-1, it can participate in renal inflammation and fibrosis by regulating different targets (41). Other studies have shown that HIF-1α mediates renal hypoxic metabolic response by regulating the expression of VEGF, inhibits HIF-1α and VEGF, and increases the expression of podocyte related proteins, which can alleviate podocyte injury and slow down the process of glomerular fibrosis in diabetic patients (42). HIF-1α may play a protective role in diabetic renal tubular injury by regulating mitochondrial dynamics through up-regulating HO-1 (43).
To further explore the potential molecular mechanism of Rhubarb-Coptis in treatment of DN, we conducted molecular docking studies of the first 5 key targets closely related to DN. The results showed that STAT3, CTNNB1, PIK3R1, PIK3CA, and TP53 had good ability to bind relevant active compounds in Rhubarb-Coptis.
In the field of traditional Chinese medicine in the treatment of DN, in addition to rhubarb and coptis, there are many traditional Chinese medicines that have significant effects in the treatment of DN. For example, the isoflavone compound puerarin extracted from kudzu root can significantly improve the disorder of lipid metabolism and insulin resistance in diabetic rats, and inhibit podocyte autophagy to reduce the progress of DN damage (44). Tanshinone, the active ingredient in Salvia miltiorrhiza, can change TGF in kidney-β/Smad and NF-κB pathway expression to inhibit inflammation and renal fibrosis (45). Astragaloside A can inhibit endoplasmic reticulum stress and mitochondrial mediated apoptosis (46). Sophora Qi Huang can reduce podocyte apoptosis by regulating endoplasmic reticulum stress protein, maintaining mitochondrial calcium homeostasis (47). Therefore, traditional Chinese medicine has significant advantages in treating DN, which can be treated from multiple angles, channels and targets.
There were still some limitations to this study, which need to be continuously improved. For example, we only discussed the mechanism of Rhubarb-Coptis in the treatment of DN from the perspective of network pharmacology. However, in view of the current information technology and data accuracy, the real-time effects need to be improved, it is necessary to conduct further mechanism experiments to clarify the synergistic effects of multi-components, multi-targets, and multi-pathways involved in the treatment process of DN by Rhubarb-Coptis.
In the future drug research and development, we can use network pharmacology to study the active components and mechanism of action of drugs to treat diseases, and to screen the active components, action targets and pathways of known drugs, so as to find new indications and related mechanisms of action of drugs. However, there are still some problems to be solved in the application of network pharmacology in studying the treatment of DN with traditional Chinese medicine; the data of each database platform is different, which makes the prediction result biased. Therefore, in the application of network pharmacology to the study of the mechanism of action of traditional Chinese medicine in treating DN, we should take into account its informatization, networking and the application of the holistic view, further explore the TCM syndromes of DN, take syndrome differentiation as the program, integrate the basic experimental data and TCM clinical syndromes, and build a network model of disease syndrome integration.
Conclusions
The present study analyzed the mechanisms of Rhubarb-Coptis in the treatment DN by constructing Rhubarb-Coptis-target-DN-related pathway network using network pharmacology. We found that quercetin, berberine, eupatin, epiberberine, and moupinamide can be defined as 5 crucial compounds. STAT3, CTNNB1, PIK3R1, PIK3CA, and TP53 may be the 5 most important therapeutic targets. The AGE-RAGE and HIF-1 are 2 key signaling pathways of Rhubarb-Coptis for the treatment of DN. The underlying mechanisms are mainly related to oxidative stress, inflammatory response, and apoptosis. The results of this study provide a reference for further exploring the mechanism of Rhubarb-Coptis in the treatment of DN.
Acknowledgments
Funding: This work was supported by Jilin Provincial Natural Science Foundation, based on miR-200a, discusses the mechanism of detoxification, unblocking collaterals and expelling wind therapy for diabetic nephropathy (No. YDZJ202201ZYTS192).
Footnote
Reporting Checklist: The authors have completed the STREGA reporting checklist. Available at https://atm.amegroups.com/article/view/10.21037/atm-22-5550/rc
Conflicts of Interest: All authors have completed the ICMJE uniform disclosure form (available at https://atm.amegroups.com/article/view/10.21037/atm-22-5550/coif). The authors have no conflicts of interest to declare.
Ethical Statement: The authors are accountable for all aspects of the work in ensuring that questions related to the accuracy or integrity of any part of the work are appropriately investigated and resolved. The study was conducted in accordance with the Declaration of Helsinki (as revised in 2013).
Open Access Statement: This is an Open Access article distributed in accordance with the Creative Commons Attribution-NonCommercial-NoDerivs 4.0 International License (CC BY-NC-ND 4.0), which permits the non-commercial replication and distribution of the article with the strict proviso that no changes or edits are made and the original work is properly cited (including links to both the formal publication through the relevant DOI and the license). See: https://creativecommons.org/licenses/by-nc-nd/4.0/.
References
- Yao L, Liang X, Qiao Y, et al. Mitochondrial dysfunction in diabetic tubulopathy. Metabolism 2022;131:155195. [Crossref] [PubMed]
- Thomas B. The Global Burden of Diabetic Kidney Disease: Time Trends and Gender Gaps. Curr Diab Rep 2019;19:18. [Crossref] [PubMed]
- Koye DN, Magliano DJ, Nelson RG, et al. The Global Epidemiology of Diabetes and Kidney Disease. Adv Chronic Kidney Dis 2018;25:121-32. [Crossref] [PubMed]
- Wang H, Zhang R, Wu X, et al. The Wnt Signaling Pathway in Diabetic Nephropathy. Front Cell Dev Biol 2021;9:701547. [Crossref] [PubMed]
- Vartak T, Godson C, Brennan E. Therapeutic potential of pro-resolving mediators in diabetic kidney disease. Adv Drug Deliv Rev 2021;178:113965. [Crossref] [PubMed]
- Wang J, Wang L, Lou GH, et al. Coptidis Rhizoma: a comprehensive review of its traditional uses, botany, phytochemistry, pharmacology and toxicology. Pharm Biol 2019;57:193-225. [Crossref] [PubMed]
- Wang S, Huang XF, Zhang P, et al. Chronic rhein treatment improves recognition memory in high-fat diet-induced obese male mice. J Nutr Biochem 2016;36:42-50. [Crossref] [PubMed]
- Xu S, Lv Y, Zhao J, et al. The Inhibitory Effect of Rhein on Proliferation of High Glucose-induced Mesangial Cell Through Cell Cycle Regulation and Induction of Cell Apoptosis. Pharmacogn Mag 2016;12:S257-63. [Crossref] [PubMed]
- Tu Y, Gu L, Chen D, et al. Rhein Inhibits Autophagy in Rat Renal Tubular Cells by Regulation of AMPK/mTOR Signaling. Sci Rep 2017;7:43790. [Crossref] [PubMed]
- Chen W, Chang B, Zhang Y, et al. Rhein promotes the expression of SIRT1 in kidney tissues of type 2 diabetic rat. Xi Bao Yu Fen Zi Mian Yi Xue Za Zhi 2015;31:615-9. [PubMed]
- Xu J, Wang F, Guo J, et al. Pharmacological Mechanisms Underlying the Neuroprotective Effects of Alpinia oxyphylla Miq. on Alzheimer's Disease. Int J Mol Sci 2020;21:2071. [Crossref] [PubMed]
- Ru J, Li P, Wang J, et al. TCMSP: a database of systems pharmacology for drug discovery from herbal medicines. J Cheminform 2014;6:13. [Crossref] [PubMed]
- UniProt Consortium. Reorganizing the protein space at the Universal Protein Resource (UniProt). Nucleic Acids Res 2012;40:D71-5. [Crossref] [PubMed]
- Amberger JS, Hamosh A. Searching Online Mendelian Inheritance in Man (OMIM): A Knowledgebase of Human Genes and Genetic Phenotypes. Curr Protoc Bioinformatics 2017;58:1.2.1-1.2.12.
- Stelzer G, Rosen N, Plaschkes I, et al. The GeneCards Suite: From Gene Data Mining to Disease Genome Sequence Analyses. Curr Protoc Bioinformatics 2016;54:1.30.1-1.30.33.
- Li YH, Yu CY, Li XX, et al. Therapeutic target database update 2018: enriched resource for facilitating bench-to-clinic research of targeted therapeutics. Nucleic Acids Res 2018;46:D1121-7. [Crossref] [PubMed]
- Szklarczyk D, Gable AL, Lyon D, et al. STRING v11: protein-protein association networks with increased coverage, supporting functional discovery in genome-wide experimental datasets. Nucleic Acids Res 2019;47:D607-13. [Crossref] [PubMed]
- Zhang L, Han L, Wang X, et al. Exploring the mechanisms underlying the therapeutic effect of Salvia miltiorrhiza in diabetic nephropathy using network pharmacology and molecular docking. Biosci Rep 2021;41:BSR20203520. [Crossref] [PubMed]
- Sherman BT, Hao M, Qiu J, et al. DAVID: a web server for functional enrichment analysis and functional annotation of gene lists (2021 update). Nucleic Acids Res 2022; Epub ahead of print. [Crossref] [PubMed]
- Huang da W. Sherman BT, Lempicki RA. Systematic and integrative analysis of large gene lists using DAVID bioinformatics resources. Nat Protoc 2009;4:44-57. [Crossref] [PubMed]
- Li C, Du X, Liu Y, et al. A Systems Pharmacology Approach for Identifying the Multiple Mechanisms of Action for the Rougui-Fuzi Herb Pair in the Treatment of Cardiocerebral Vascular Diseases. Evid Based Complement Alternat Med 2020;2020:5196302. [Crossref] [PubMed]
- Wang J, Zhang Q, Li S, et al. Low molecular weight fucoidan alleviates diabetic nephropathy by binding fibronectin and inhibiting ECM-receptor interaction in human renal mesangial cells. Int J Biol Macromol 2020;150:304-14. [Crossref] [PubMed]
- Li S, Zhang B. Traditional Chinese medicine network pharmacology: theory, methodology and application Chinese Journal of Natural Medicines 2013;11:110-20. [Crossref] [PubMed]
- Tong F, Liu S, Yan B, et al. Quercetin nanoparticle complex attenuated diabetic nephropathy via regulating the expression level of ICAM-1 on endothelium. Int J Nanomedicine 2017;12:7799-813. [Crossref] [PubMed]
- Hickson LJ, Langhi Prata LGP, Bobart SA, et al. Senolytics decrease senescent cells in humans: Preliminary report from a clinical trial of Dasatinib plus Quercetin in individuals with diabetic kidney disease. EBioMedicine 2019;47:446-56. [Crossref] [PubMed]
- Cheng D, Gao L, Su S, et al. Moringa Isothiocyanate Activates Nrf2: Potential Role in Diabetic Nephropathy. AAPS J 2019;21:31. [Crossref] [PubMed]
- Xiao Y, Deng J, Li C, et al. Epiberberine ameliorated diabetic nephropathy by inactivating the angiotensinogen (Agt) to repress TGFβ/Smad2 pathway. Phytomedicine 2021;83:153488. [Crossref] [PubMed]
- Chou CH, Hsu KC, Lin TE, et al. Anti-Inflammatory and Tau Phosphorylation-Inhibitory Effects of Eupatin. Molecules 2020;25:5652. [Crossref] [PubMed]
- Silva EL, Almeida-Lafetá RC, Borges RM, et al. Dual high-resolution inhibition profiling and HPLC-HRMS-SPE-NMR analysis for identification of α-glucosidase and radical scavenging inhibitors in Solanum americanum Mill. Fitoterapia 2017;118:42-8. [Crossref] [PubMed]
- Yu J, Wu H, Liu ZY, et al. Advanced glycation end products induce the apoptosis of and inflammation in mouse podocytes through CXCL9-mediated JAK2/STAT3 pathway activation. Int J Mol Med 2017;40:1185-93. [Crossref] [PubMed]
- Che X, Deng X, Xie K, et al. Long noncoding RNA MEG3 suppresses podocyte injury in diabetic nephropathy by inactivating Wnt/β-catenin signaling. PeerJ 2019;7:e8016. [Crossref] [PubMed]
- Qian X, He L, Hao M, et al. YAP mediates the interaction between the Hippo and PI3K/Akt pathways in mesangial cell proliferation in diabetic nephropathy. Acta Diabetol 2021;58:47-62. [Crossref] [PubMed]
- Huang G, Zou B, Lv J, et al. Notoginsenoside R1 attenuates glucose-induced podocyte injury via the inhibition of apoptosis and the activation of autophagy through the PI3K/Akt/mTOR signaling pathway. Int J Mol Med 2017;39:559-68. [Crossref] [PubMed]
- Higgins CE, Tang J, Mian BM, et al. TGF-β1-p53 cooperativity regulates a profibrotic genomic program in the kidney: molecular mechanisms and clinical implications. FASEB J 2019;33:10596-606. [Crossref] [PubMed]
- Sifuentes-Franco S, Padilla-Tejeda DE, Carrillo-Ibarra S, et al. Oxidative Stress, Apoptosis, and Mitochondrial Function in Diabetic Nephropathy. Int J Endocrinol 2018;2018:1875870. [Crossref] [PubMed]
- Zhang Q, Hu Y, Hu JE, et al. Sp1-mediated upregulation of Prdx6 expression prevents podocyte injury in diabetic nephropathy via mitigation of oxidative stress and ferroptosis. Life Sci 2021;278:119529. [Crossref] [PubMed]
- Wang Z, Zhang J, Chen L, et al. Glycine Suppresses AGE/RAGE Signaling Pathway and Subsequent Oxidative Stress by Restoring Glo1 Function in the Aorta of Diabetic Rats and in HUVECs. Oxid Med Cell Longev 2019;2019:4628962. [Crossref] [PubMed]
- Bhattacharjee N, Barma S, Konwar N, et al. Mechanistic insight of diabetic nephropathy and its pharmacotherapeutic targets: An update. Eur J Pharmacol 2016;791:8-24. [Crossref] [PubMed]
- Ahmad A, Biersack B, Li Y, et al. Targeted regulation of PI3K/Akt/mTOR/NF-κB signaling by indole compounds and their derivatives: mechanistic details and biological implications for cancer therapy. Anticancer Agents Med Chem 2013;13:1002-13. [Crossref] [PubMed]
- Sun H, Yuan Y, Sun Z. Update on Mechanisms of Renal Tubule Injury Caused by Advanced Glycation End Products. Biomed Res Int 2016;2016:5475120. [Crossref] [PubMed]
- Bessho R, Takiyama Y, Takiyama T, et al. Hypoxia-inducible factor-1α is the therapeutic target of the SGLT2 inhibitor for diabetic nephropathy. Sci Rep 2019;9:14754. [Crossref] [PubMed]
- Shukla R, Pandey N, Banerjee S, et al. Effect of extract of Pueraria tuberosa on expression of hypoxia inducible factor-1α and vascular endothelial growth factor in kidney of diabetic rats. Biomed Pharmacother 2017;93:276-85. [Crossref] [PubMed]
- Jiang N, Zhao H, Han Y, et al. HIF-1α ameliorates tubular injury in diabetic nephropathy via HO-1-mediated control of mitochondrial dynamics. Cell Prolif 2020;53:e12909. [Crossref] [PubMed]
- Xu W, Tang M, Wang J, et al. Anti-inflammatory activities of puerarin in high-fat diet-fed rats with streptozotocin-induced gestational diabetes mellitus. Mol Biol Rep 2020;47:7537-46. [Crossref] [PubMed]
- Lin X, Zha Y, Zeng XZ, et al. Role of the Wnt/β-catenin signaling pathway in inducing apoptosis and renal fibrosis in 5/6-nephrectomized rats. Mol Med Rep 2017;15:3575-82. [Crossref] [PubMed]
- Guo H, Cao A, Chu S, et al. Astragaloside IV Attenuates Podocyte Apoptosis Mediated by Endoplasmic Reticulum Stress through Upregulating Sarco/Endoplasmic Reticulum Ca2+-ATPase 2 Expression in Diabetic Nephropathy. Front Pharmacol 2016;7:500. [Crossref] [PubMed]
- Li TX, Mao JH, Huang L, et al. Beneficial effects of Huaiqihuang on hyperglycemia-induced MPC5 podocyte dysfunction through the suppression of mitochondrial dysfunction and endoplasmic reticulum stress. Mol Med Rep 2017;16:1465-71. [Crossref] [PubMed]
(English Language Editor: J. Jones)