Development of personalized classifier based on metastasis and the immune microenvironment to predict the prognosis of osteosarcoma patients
Highlight box
Key findings
• We developed a personalized classifier based on metastasis and immune cells to achieve better prognostic prediction in osteosarcoma.
What is known and what is new?
• Immune microenvironment is crucial to the metastasis and drug treatment response of osteosarcoma. Hence, an integrated metastasis-immune microenvironment signature may benefit clinical classification of osteosarcoma.
• Here, we developed a metastasis- immune microenvironment classifier based on comprehensive analysis of immune cells and metastasis-related genes in osteosarcoma.
What is the implication, and what should change now?
• The classifier might be a beneficial tool to aid in the prognostic evaluation and risk stratification of osteosarcoma patients.
Introduction
Osteosarcoma is a common primary bone malignant tumor that predominantly affects children and adolescents, accounting for about 5% of tumors in children worldwide (1,2). Owing to its high aggressiveness, osteosarcoma has high rates of morbidity and mortality. According to statistics, there are approximately 4.4 cases of osteosarcoma per million children each year (3). A combination of neoadjuvant chemotherapy and surgical resection is the standard treatment for osteosarcoma, and the 5-year survival rates of early-stage osteosarcoma patients have significantly improved to 60–70% after treatment (4,5). However, the prognosis and overall survival (OS) rates of advanced-stage osteosarcoma patients with metastasis remain very low (~20–30%). In addition, the effective rate of osteosarcoma patients who respond to targeted therapy is also very low (6-8). Thus, it is important to clarify the molecular mechanism of osteosarcoma metastasis and identify new biomarkers to accurately predict the survival time and prognostic differences of osteosarcoma patients.
Increasing evidence indicates that the tumor microenvironment (TME) is crucial to the metastasis and drug treatment response of osteosarcoma through various infiltrating immune cells, chemokines, and signaling pathways (9-11). Moreover, the tumor immune microenvironment is also an important factor influencing the survival prognosis of cancer patients (12). Tumor-infiltrating immune cells are mainly composed of T cells, natural killer (NK) cells, macrophages, monocytes, dendritic cells, and B cells. According to several studies, the increased infiltration of macrophages is linked to a better prognosis and prolonged progression-free survival (PFS) time in osteosarcoma (13,14). Moreover, patients with metastasis have an elevated number of tumor-infiltrating T cells compared to those with primary tumors, and the effector of CD8+ T cell infiltration is positively associated with prognosis and survival time in osteosarcoma (15). Several clinical trials and experimental studies have demonstrated the potential of TME-based therapy to improve the clinical survival outcome of osteosarcoma patients (16-18). Therefore, to improve the prognosis and effectiveness of osteosarcoma treatment, there is a pressing need to explore metastasis and the specific immune microenvironment, and also investigate the underlying molecular mechanisms involved.
In this study, we aimed to develop a personalized classifier to enhance the risk stratification and predictive accuracy of osteosarcoma patients by incorporating metastasis and immune cells, not only focus on metastasis or TME. Firstly, the metastasis-related gene signature (MRS) and TME scores of patients were calculated utilizing the genes related to metastasis and immune cells in the TME, respectively. Then, an MRS-TME classifier was developed by combining the MRS and TME scores. Furthermore, the sensitivity and specificity of the classifier were validated in a separate osteosarcoma cohort (GSE21257) and diverse clinical characteristic subgroups. Finally, we determined the association between the MRS-TME classifier, the immune response profile, and the molecular signaling pathways to guide the personalized treatment of osteosarcoma patients. We present the following article in accordance with the TRIPOD reporting checklist (available at https://atm.amegroups.com/article/view/10.21037/atm-22-5856/rc).
Methods
Data collection and procession
The Therapeutically Applicable Research to Generate Effective Treatments Osteosarcoma (TARGET-OS https://ocg.cancer.gov/programs/target/projects/osteosarcoma) project provided the gene expression (RNA-Sequencing) data and concordant clinical information of 84 osteosarcoma patients. Additionally, the Gene Expression Omnibus (GEO https://www.ncbi.nlm.nih.gov/geo/) database was employed to download the gene expression profile (microarray data) of 53 osteosarcoma patients in the GSE21257 dataset (platform GPL10295). When available, the following clinical information of these two cohorts were gathered: patient identification (ID), gender, recurrence status, age, recurrence-free survival (RFS) time, vital status, metastasis status, survival follow-up time, and tumor grade (available online: https://cdn.amegroups.cn/static/public/atm-22-5856-1.csv, https://cdn.amegroups.cn/static/public/atm-22-5856-2.csv). We excluded osteosarcoma patients with incomplete data and included those with a follow-up time greater than 1 month to ensure the rationality of the clinical data. In this study, the TARGET-OS cohort was used to develop the MRS-TME classifier for osteosarcoma patients, and the GSE21257 cohort was used as the validation cohort. For the subsequent analysis, the entire microarray expression and the RNA-sequencing data were normalized. The study was conducted in accordance with the Declaration of Helsinki (as revised in 2013).
Analysis of metastasis-related genes and immune cells
Based on gene expression in the count format, the Bioconductor package edgeR was employed to detect the metastasis-related differentially expressed genes (DEGs) in osteosarcoma patients (19). The differential expression threshold was defined using a cutoff of |log2 (fold change)| >0 and P<0.05. The transcript per million (TPM) and single sample gene set enrichment analysis (ssGSEA) algorithms were employed to evaluate the relative abundance of immune cells in the TME. This algorithm was based on the gene signature, and the gene signature of 28 immune cells was congregated from the Tumor and Immune System Interaction Database (TISIDB) (20). The ssGSEA algorithm can distinguish different immune cell types, mainly including CD8+ T cells subgroups, CD4+ T cells subgroups, dendritic cells, B cells, macrophages, NK cells, neutrophils, fibroblasts, monocytes, and other T cells. The immune checkpoints and immune cells were analyzed to examine the relationship between the tumor immune microenvironment and the MRS in patients with osteosarcoma.
Establishment of the MRS, TME score, and MRS-TME classifier
Through univariate Cox regression analysis, the prognosis-related genes were identified to construct the MRS. Based on these genes, we constructed the MRS for osteosarcoma patients using Least Absolute Shrinkage and Selection Operator (LASSO) Cox regression analysis with the glmnet package. Also, the risk score of the MRS was calculated using the expression level of the candidate genes and corresponding LASSO coefficients: MRS = ∑i Coefficient (mRNAi) × Expression (mRNAi). Furthermore, the prognostic immune cells were identified by Kaplan-Meier (K-M) survival analysis by the survival R package. A novel TME score was calculated from the immune cells selected by the LASSO model and the risk groups were established using the appropriate thresholds. The normalized score for the MRS and TME was employed to facilitate further analysis.
The MRS-TME classifier was then developed by integrating the MRS and TME scores. The following subgroups were established by further classifying the patients based on the mean value of the MRS and TME scores in every cohort: MRSlow/TMEhigh, intermediate mixed (MRSlow/TMElow and MRShigh/TMEhigh), and MRShigh/TMElow. K-M survival and receiver operating characteristic (ROC) analyses were performed in the diverse osteosarcoma cohorts as well as in the clinical subgroups, and the predictive accuracy of the MRS-TME classifier was assessed and validated.
Functional enrichment analysis
Gene Ontology (GO) and Kyoto Encyclopedia of Genes and Genomes (KEGG) pathway enrichment analysis were carried out using the Bioconductor package clusterProfiler (21). The enrichment results were considered significant when the P value was <0.05. In addition, gene set variation analysis (GSVA) analysis of osteosarcoma patients across different groups was performed using the Bioconductor package GSVA (22). All reference gene sets were gathered from the Molecular Signatures Database (MSigDB, version 7.5) (23,24).
Statistical analysis
R software (Version 4.1.2) was employed to conduct the statistical analysis. The “survminer” and “survival” R packages were used to analyze the survival curves, and differences were assessed using the log-rank test and the K-M method. A Student’s t-test analysis of variance was performed to examine the differences between groups in terms of variables with a normal distribution. P<0.05 was considered statistically significant.
Results
Identification of metastasis-related genes in osteosarcoma
In this study, we first determined the impact of metastasis on the prognosis of osteosarcoma patients. Two groups contained all the osteosarcoma patients in the TARGET-OS cohort according to whether they had metastasis, and osteosarcoma patients with metastasis had a poorer OS and RFS than those without metastasis (Figure 1A,1B). The results were also validated in the GSE21257 cohort and similarly found that metastasis was markedly associated with a worse OS (Figure 1C). Furthermore, a total of 894 genes were identified as significant DEGs [|log2 (FC)| >1 and P<0.05] from the TARGET-OS cohort, including 506 genes up-regulated and 388 genes down-regulated in metastasized osteosarcoma relative to non-metastasized tissues (Figure 1D, available online: https://cdn.amegroups.cn/static/public/atm-22-5856-3.xlsx).
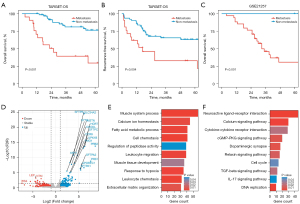
In addition, GO analysis revealed that these genes were primarily involved in the muscle system process, calcium ion homeostasis, cell chemotaxis, leukocyte migration, and the response to hypoxia (Figure 1E). KEGG enrichment analysis revealed that these genes primarily engaged in the cytokine-cytokine receptor interaction and calcium signaling pathways (Figure 1F). Finally, there were 2,336 up-regulated and 2,009 down-regulated DEGs in GSE21257 (available online: https://cdn.amegroups.cn/static/public/atm-22-5856-4.xlsx). Of these, 173 common DEGs in both TARGET-OS and GSE21257 cohorts were defined as metastasis-related genes (MRGs) (available online: https://cdn.amegroups.cn/static/public/atm-22-5856-5.xlsx).
Development of a metastasis-related signature in osteosarcoma
Based on the TARGET-OS cohort, univariate Cox analysis was first employed to detect the OS-related genes. The results showed that patients’ OS was substantially correlated with 16 genes, including six protective and 10 risk genes (P<0.05, Figure 2A). Next, LASSO Cox regression analysis was performed to develop a prognostic MRS for osteosarcoma patients (Figure 2B). Ten MRGs were selected, namely, MYC proto-oncogene (MYC), F-box, and WD repeat domain containing 12 (FBXW12), farnesyl diphosphate synthase (FDPS), phosphoglycerate mutase family member 4 (PGAM4), poly(A) polymerase beta (PAPOLB), C-X-C motif chemokine ligand 13 (CXCL13), GLI pathogenesis-related 1 (GLIPR1), S100 calcium binding protein A8 (S100A8), coagulation factor XIII A chain (F13A1), and phospholipase C beta 1 (PLCB1). The LASSO Cox coefficients of the 10 MRGs are shown in Figure 2C.
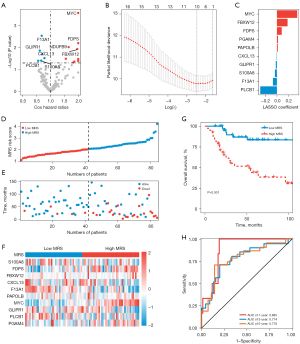
After classifying the osteosarcoma patients into two groups, we observed that patients with high MRS had greater risk scores and mortality compared to those with low MRS (Figure 2D,2E). Moreover, patients in the high-MRS group had higher expression levels of MYC, FBXW12, FDPS, and PGAM4 (Figure 2F). Also, the prognosis of patients with high MRS was remarkably worse than that of patients with low MRS (P<0.001; Figure 2G). As for the 1-, 3-, and 5-year OS rates, our results also suggested that the area under the curves (AUC) of the MRS were 0.885, 0.774, and 0.778, respectively (Figure 2H).
Correlation between the tumor immune microenvironment and the MRS
The relative number of immune cells in the osteosarcoma patients was evaluated using the ssGSEA algorithm to illustrate the tumor immune microenvironment of these patients. The immune cells varied remarkably between the low- and high-MRS groups (Figure 3A). In particular, we discovered that the osteosarcoma patients with high MRS had a lower relative content of 15 immune cells [activated (Act) B cells, Act CD8 cells, CD56bright cells, immature (Imm) B cells, macrophages, mast cells, myeloid derived suppressor cells (MDSCs), monocytes, neutrophils, natural killer (NK) cells, central memory CD4 T (Tcm CD4) cells, Tcm CD8 cells, effector memory CD8 T (Tem CD8) cells, type 1 T helper (Th1) cells, and regulatory T cells (Tregs)] than the osteosarcoma patients with low-MRS. As shown in Figure 3B, the MRS score exhibited a significantly negative correlation to the expression of the M2 macrophage markers (CD163, CD209), CD4+ T cells marker CD4, CD8+ T cells marker CD8A, Treg marker FOXP3, M0 macrophage marker CD68, and cytotoxic markers (GZMB, PRF1). Additionally, S100A8, GLIPR1, F13A1, and CXCL13 were significantly positively related to these immune cell markers. Also, the expression of MYC was significantly positively linked to these immune cell markers. Furthermore, the correlation between the immune checkpoints and MRS was also explored, and we discovered that the MRS score was negatively linked to the expressions of CD274, CTLA4, and PDCD1 (Figure 3C,3D).
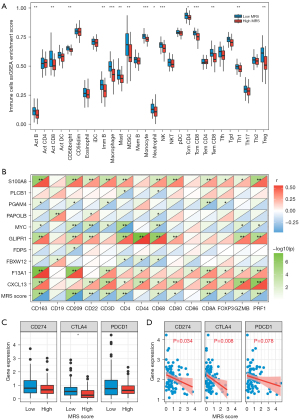
Construction of the MRS-TME classifier improved prognostic assessment
The prognostic value of immune cells was further determined. As shown in Figure 4A, the results of multivariate Cox regression analysis showed that CD56bright, Tcm.CD8, and Tem.CD8 were considerably linked to patient survival (P<0.05). We subsequently used LASSO Cox analysis to develop a TME score based on the prognostic TME signatures. TME score = (−4.127 × Tcm CD8) + (−5.359 × CD56bright) + (−2.011 × NKT). Our findings also indicated that patients with high TME had a remarkably better prognosis than those with low TME (P<0.001; Figure 4B).
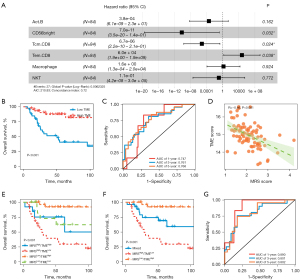
As for the 1-, 3-, and 5-year OS rates, the ROC analysis demonstrated that the AUCs of the TME were 0.747, 0.761, and 0.768, respectively (Figure 4C). In addition, the TARGET-OS cohort-related MRS score and TME score exhibited a statistically significant negative correlation (Figure 4D). As a result, we developed the MRS-TME classifier by integrating the MRS and TME scores, which was used to divide the patients into four subgroups: MRSlow/TMEhigh, MRSlow/TMElow, MRShigh/TMEhigh, and MRShigh/TMElow.
A statistically distinct patient prognosis was revealed by the MRS-TME classifier (Figure 4E). After comparing patients from the other three subgroups, it was found that those in the MRSlow/TMEhigh subgroup had the best prognosis. The prognosis of patients in the MRSlow/TMElow and MRShigh/TMEhigh subgroups was less divergent. Consequently, we created a mixed subgroup by merging these two subgroups (Figure 4F). The MRS-TME classifier exhibited a higher AUC than the MRS or TME (Figure 4G). In summary, these findings revealed that our MRS-TME classifier enhanced the accuracy of prognostic prediction for osteosarcoma patients.
Validation of the MRS-TME classifier in other clinical cohorts and different subgroups
Furthermore, we validated the prognostic value of the MRS-TME classifier in a diverse osteosarcoma cohort GSE21257 and different subgroups. Similar outcomes were also observed in the GSE21257 cohort, and our results revealed a poorer survival prognosis in patients with high-MRS or low-TME (Figure 5A,5B). There was also a statistically substantial negative correlation between the MRS and TME scores in the GSE21257 cohort (P<0.01; Figure 5C). Importantly, the MRS-TME classifier depicted a statistically distinct prognosis in the GSE21257 cohort and compared to the patients from the other three subgroups, patients in the MRSlow/TMEhigh subgroup had the best prognosis (P=0.001; Figure 5D). For the 1-, 3-, and 5-year OS rates, the ROC analysis depicted that the AUCs of the MRS-TME classifier were 0.781, 0.719, and 0.645, respectively (Figure 5E).
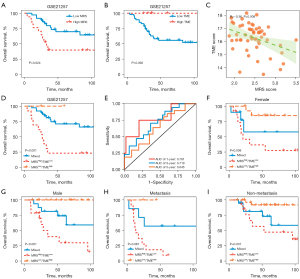
Furthermore, a clinical subgroup analysis was carried out to investigate the prognostic value of the MRS-TME classifier in patients suffering from osteosarcoma with diverse clinical features, such as gender and metastasis, and similar outcomes were observed (Figure 5F-5I). We also discovered that osteosarcoma patients with MRSlow/TMEhigh had a longer survival prognosis than other patients. Our results also demonstrated the prognostic value of the MRS-TME classifier in different osteosarcoma cohorts as well as in the clinical subgroups.
Differences in the signaling pathways and immune response profiler among the different MRS-TME subgroups
Given the significant prognostic variations based on the MRS-TME classifier, a cancer-related signaling pathways analysis was conducted to explore the underlying molecular mechanism (Figure 6A). The results showed that patients in the different subgroups exhibited diverse patterns in terms of the immune response, metabolism-related signaling pathways, and tumor proliferation. Interestingly, it was found that patients in the high-MRS score subgroups exhibited a much greater enrichment of bile acid metabolism, xenobiotic metabolism, KRAS signaling, and unfolded protein response-related gene expression compared with patients with a low MRS score. Furthermore, patients in the high-TME score subgroups exhibited a significantly higher enrichment of complement, inflammatory response, p53 signaling, and IL6/JAK/STAT3 signaling-related gene expressions compared to low-TME score tumors. These results suggested that patients with high TME scores experience less tumor growth and a stronger antitumor immune response. The immune response-related genes across the diverse subgroups were then further examined from two aspects: inhibitory immune markers and activation immune markers. It was noted that the MRSlow/TMEhigh subgroup generally had a higher expression of the inhibitory immune marker and activation immune markers compared with the MRShigh/TMElow subgroups (Figure 6B,6C).
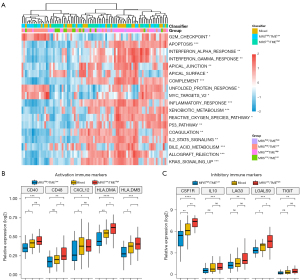
Discussion
Metastasis, a characteristic of cancer, is the primary cause of death in patients with osteosarcoma. Several studies have demonstrated that MRSs could predict the clinical prognosis in these patients (25,26). In the present study, the prognostic value of metastasis in osteosarcoma was determined, and our results also demonstrated that metastasis was significantly associated with a poorer patient prognosis, as compared to non-metastasis patients. To clarify the molecular mechanism underlying osteosarcoma metastasis, we discovered the DEGs between metastasis and non-metastasis patients in the TARGET-OS and GSE21257 cohorts. Functional enrichment analysis revealed that these DEGs were primarily involved in the muscle system process, calcium ion homeostasis, cell chemotaxis, leukocyte migration, and the response to hypoxia. These results suggested that the TME is a key factor in the progression and metastasis of osteosarcoma. Therefore, it is crucial to scrutinize the interactive effects of metastasis and the TME on the clinical prognosis and treatment outcomes of osteosarcoma patients.
Additionally, we looked at ways to predict the clinical prognosis of patients with osteosarcoma. As osteosarcoma is a highly heterogeneous tumor, Tumor Node Metastasis (TNM) staging systems cannot accurately predict the survival prognosis of patients. It has been revealed that biomarkers increase the accuracy of clinical prognosis prediction at the molecular level (16,17,27). The MRS was developed in the present study to predict the clinical prognosis of osteosarcoma patients based on 10 genes (MYC, FBXW12, FDPS, PGAM4, PAPOLB, CXCL13, GLIPR1, S100A8, F13A1, and PLCB1). Patients with high MRS exhibited a considerably poorer prognosis than those with low MRS. Subsequently, we created a TME score based on the prognosis-related immune cells. Our findings also indicated that patients with high TME had a remarkably better prognosis than those with low TME. Thus, we developed the MRS-TME classifier, which divided patients into four categories by combining the MRS score with the TME score: MRSlow/TMEhigh, MRSlow/TMElow, MRShigh/TMEhigh, and MRShigh/TMElow. The four risk groups showed substantial variations in survival. Compared with patients from the other three subgroups, it was discovered that those in the MRSlow/TMEhigh subgroup had the best prognosis. The MRS-TME classifier was also validated in an independent cohort, GSE21257, as well as different clinical subgroups, which included gender and metastasis subgroups. Our findings exhibited that the MRS-TME classifier enhanced the prognostic assessment and risk stratification of osteosarcoma patients.
Additionally, a strong correlation between the MRS scores and the TNM stages was discovered, with advanced-stage osteosarcoma patients having higher MRS scores compared to those with early-stage osteosarcoma. These results suggested that genes related to metastasis might be involved in the onset and progression of osteosarcoma. Myc is a famous cancer oncogene, and its amplification indicates a poor survival prognosis (28). It plays a wide range of roles in cancer, including the promotion of growth, cell cycle progression, metabolism, and survival (29). A previous study has supported Myc as a novel prognostic biomarker and therapeutic target in the treatment of osteosarcoma (30). Through its interactions with several cellular receptors, the biological role of FDPS in numerous malignancies has been documented (31,32). However, no research has yet been performed to determine the biological role of FDPS in osteosarcoma. Furthermore, CXCL13 and its chemokine receptor 5 (CXCR5) are crucial chemotactic factors that are essential for understanding cancer cell biology (33). In osteosarcoma, Liu et al. reported that the CXCL13/CXCR5 interaction assists vascular cell adhesion molecule (VCAM)-1-dependent migration (34). Additionally, S100A8 is a Ca2+ binding protein belonging to the S100 family that is related to epithelial-mesenchymal transition and cancer metastasis and plays a significant role in the progression of osteosarcoma (35).
The impact of MRS on the tumor immune microenvironment was also investigated in this study. The relative number of immune cells was examined by employing the ssGSEA algorithm. Our findings revealed that low-GRS osteosarcoma patients had a greater percentage of immunity signatures compared to high-MRS patients. CD8+ T cells are considered the main driver of anti-tumor immunity as they mediate tumor immunity by identifying tumor antigens and directly killing tumor cells via the secretion of perforin and granzyme B (36). The cytotoxicity of CD8+ T cells can be improved by cytokines such as interleukin-2 (IL-2), IL-12, and interferon (IFN) γ, which can be activated by naive CD8+ T cells (37). The increase of cytotoxic CD8+ T cells in the TME is related to the anti-tumor effect and prognostic improvement of cancers. In this study, compared to patients with high-GRS osteosarcoma, patients with low-MRS osteosarcoma exhibited higher proportions of CD8+ T cells, which are essential for enhancing antitumor immunity. NK cells exert many functions that can limit the growth and spread of cancer cells. Under the stimulation of proinflammatory chemokines of the TME, circulating NK cells can be recruited to the site of the tumor to kill tumor cells (38). In addition, the activation of antigen-presenting cells, including macrophages, B cells, and dendritic cells, has been demonstrated to be influenced by CD4+ T cells (39). These findings suggest that patients with low GRS have more immunoactivity cells, which promote anti-tumor immune responses and contribute to better clinical survival.
This study had some limitations that should be noted. Firstly, the prognostic model was validated using the GSE21257 cohort; however, more cohorts and higher sample sizes are required to enhance the reliability of our findings. Secondly, our results may be biased due to the retrospective nature of the study. Therefore, prospective trials must be planned and carried out in the future. Thirdly, a series of bioinformatics approaches were employed to develop the prognostic model. However, further functional studies are required to investigate its role in vivo as well as its underlying molecular mechanisms. Thus, future research is required to address these drawbacks.
Conclusions
The present study developed and validated a personalized classifier based on metastasis and TME to assist in the prediction of survival prognosis in osteosarcoma patients, which might assist in guiding treatment decision-making.
Acknowledgments
Funding: None.
Footnote
Reporting Checklist: The authors have completed the TRIPOD reporting checklist. Available at https://atm.amegroups.com/article/view/10.21037/atm-22-5856/rc
Conflicts of Interest: All authors have completed the ICMJE uniform disclosure form (available at https://atm.amegroups.com/article/view/10.21037/atm-22-5856/coif). The authors have no conflicts of interest to declare.
Ethical Statement: The authors are accountable for all aspects of the work in ensuring that questions related to the accuracy or integrity of any part of the work are appropriately investigated and resolved. The study was conducted in accordance with the Declaration of Helsinki (as revised in 2013).
Open Access Statement: This is an Open Access article distributed in accordance with the Creative Commons Attribution-NonCommercial-NoDerivs 4.0 International License (CC BY-NC-ND 4.0), which permits the non-commercial replication and distribution of the article with the strict proviso that no changes or edits are made and the original work is properly cited (including links to both the formal publication through the relevant DOI and the license). See: https://creativecommons.org/licenses/by-nc-nd/4.0/.
References
- Eaton BR, Schwarz R, Vatner R, et al. Osteosarcoma. Pediatr Blood Cancer 2021;68:e28352. [Crossref] [PubMed]
- Lee JA, Lim J, Jin HY, et al. Osteosarcoma in Adolescents and Young Adults. Cells 2021;10:2684. [Crossref] [PubMed]
- Mirabello L, Troisi RJ, Savage SA. Osteosarcoma incidence and survival rates from 1973 to 2004: data from the Surveillance, Epidemiology, and End Results Program. Cancer 2009;115:1531-43. [Crossref] [PubMed]
- Anderson ME. Update on Survival in Osteosarcoma. Orthop Clin North Am 2016;47:283-92. [Crossref] [PubMed]
- Belayneh R, Fourman MS, Bhogal S, et al. Update on Osteosarcoma. Curr Oncol Rep 2021;23:71. [Crossref] [PubMed]
- Zhao J, Dean DC, Hornicek FJ, et al. Emerging next-generation sequencing-based discoveries for targeted osteosarcoma therapy. Cancer Lett 2020;474:158-67. [Crossref] [PubMed]
- Chen C, Xie L, Ren T, et al. Immunotherapy for osteosarcoma: Fundamental mechanism, rationale, and recent breakthroughs. Cancer Lett 2021;500:1-10. [Crossref] [PubMed]
- Heymann D. Metastatic osteosarcoma challenged by regorafenib. Lancet Oncol 2019;20:12-4. [Crossref] [PubMed]
- Shi D, Mu S, Pu F, et al. Integrative analysis of immune-related multi-omics profiles identifies distinct prognosis and tumor microenvironment patterns in osteosarcoma. Mol Oncol 2022;16:2174-94. [Crossref] [PubMed]
- Gill J, Gorlick R. Advancing therapy for osteosarcoma. Nat Rev Clin Oncol 2021;18:609-24. [Crossref] [PubMed]
- Marchais A, Marques da Costa ME, Job B, et al. Immune Infiltrate and Tumor Microenvironment Transcriptional Programs Stratify Pediatric Osteosarcoma into Prognostic Groups at Diagnosis. Cancer Res 2022;82:974-85. [Crossref] [PubMed]
- Cascini C, Chiodoni C. The Immune Landscape of Osteosarcoma: Implications for Prognosis and Treatment Response. Cells 2021;10:1668. [Crossref] [PubMed]
- Buddingh EP, Kuijjer ML, Duim RA, et al. Tumor-infiltrating macrophages are associated with metastasis suppression in high-grade osteosarcoma: a rationale for treatment with macrophage activating agents. Clin Cancer Res 2011;17:2110-9. [Crossref] [PubMed]
- Wang Z, Wu H, Chen Y, et al. The Heterogeneity of Infiltrating Macrophages in Metastatic Osteosarcoma and Its Correlation with Immunotherapy. J Oncol 2021;2021:4836292. [Crossref] [PubMed]
- Sundara YT, Kostine M, Cleven AH, et al. Increased PD-L1 and T-cell infiltration in the presence of HLA class I expression in metastatic high-grade osteosarcoma: a rationale for T-cell-based immunotherapy. Cancer Immunol Immunother 2017;66:119-28. [Crossref] [PubMed]
- Feng X, Zhao Z, Zhao Y, et al. Development of Personalized Signature Based on the Immune Landscape to Predict the Prognosis of Osteosarcoma and the Response to Immunotherapy and Targeted Therapy. Front Mol Biosci 2021;8:783915. [Crossref] [PubMed]
- Wang X, Wang L, Xu W, et al. Classification of Osteosarcoma Based on Immunogenomic Profiling. Front Cell Dev Biol 2021;9:696878. [Crossref] [PubMed]
- Zhu T, Han J, Yang L, et al. Immune Microenvironment in Osteosarcoma: Components, Therapeutic Strategies and Clinical Applications. Front Immunol 2022;13:907550. [Crossref] [PubMed]
- Robinson MD, McCarthy DJ, Smyth GK. edgeR: a Bioconductor package for differential expression analysis of digital gene expression data. Bioinformatics 2010;26:139-40. [Crossref] [PubMed]
- Ru B, Wong CN, Tong Y, et al. TISIDB: an integrated repository portal for tumor-immune system interactions. Bioinformatics 2019;35:4200-2. [Crossref] [PubMed]
- Yu G, Wang LG, Han Y, et al. clusterProfiler: an R package for comparing biological themes among gene clusters. OMICS 2012;16:284-7. [Crossref] [PubMed]
- Hänzelmann S, Castelo R, Guinney J. GSVA: gene set variation analysis for microarray and RNA-seq data. BMC Bioinformatics 2013;14:7. [Crossref] [PubMed]
- Liberzon A, Birger C, Thorvaldsdóttir H, et al. The Molecular Signatures Database (MSigDB) hallmark gene set collection. Cell Syst 2015;1:417-25. [Crossref] [PubMed]
- Liberzon A, Subramanian A, Pinchback R, et al. Molecular signatures database (MSigDB) 3.0. Bioinformatics 2011;27:1739-40. [Crossref] [PubMed]
- Zheng D, Xia K, Yu L, et al. A Novel Six Metastasis-Related Prognostic Gene Signature for Patients With Osteosarcoma. Front Cell Dev Biol 2021;9:699212. [Crossref] [PubMed]
- Ding FP, Tian JY, Wu J, et al. Identification of key genes as predictive biomarkers for osteosarcoma metastasis using translational bioinformatics. Cancer Cell Int 2021;21:640. [Crossref] [PubMed]
- Song YJ, Xu Y, Deng C, et al. Gene Expression Classifier Reveals Prognostic Osteosarcoma Microenvironment Molecular Subtypes. Front Immunol 2021;12:623762. [Crossref] [PubMed]
- Chen H, Liu H, Qing G. Targeting oncogenic Myc as a strategy for cancer treatment. Signal Transduct Target Ther 2018;3:5. [Crossref] [PubMed]
- Dhanasekaran R, Deutzmann A, Mahauad-Fernandez WD, et al. The MYC oncogene - the grand orchestrator of cancer growth and immune evasion. Nat Rev Clin Oncol 2022;19:23-36. [Crossref] [PubMed]
- Feng W, Dean DC, Hornicek FJ, et al. Myc is a prognostic biomarker and potential therapeutic target in osteosarcoma. Ther Adv Med Oncol 2020;12:1758835920922055. [Crossref] [PubMed]
- Seshacharyulu P, Halder S, Nimmakayala R, et al. Disruption of FDPS/Rac1 axis radiosensitizes pancreatic ductal adenocarcinoma by attenuating DNA damage response and immunosuppressive signalling. EBioMedicine 2022;75:103772. [Crossref] [PubMed]
- Seshacharyulu P, Rachagani S, Muniyan S, et al. FDPS cooperates with PTEN loss to promote prostate cancer progression through modulation of small GTPases/AKT axis. Oncogene 2019;38:5265-80. [Crossref] [PubMed]
- Kazanietz MG, Durando M, Cooke M. CXCL13 and Its Receptor CXCR5 in Cancer: Inflammation, Immune Response, and Beyond. Front Endocrinol (Lausanne) 2019;10:471. [Crossref] [PubMed]
- Liu JF, Lee CW, Lin CY, et al. CXCL13/CXCR5 Interaction Facilitates VCAM-1-Dependent Migration in Human Osteosarcoma. Int J Mol Sci 2020;21:6095. [Crossref] [PubMed]
- Li S, Zhang J, Qian S, et al. S100A8 promotes epithelial-mesenchymal transition and metastasis under TGF-β/USF2 axis in colorectal cancer. Cancer Commun (Lond) 2021;41:154-70. [Crossref] [PubMed]
- Huang Y, Jia A, Wang Y, et al. CD8(+) T cell exhaustion in anti-tumour immunity: The new insights for cancer immunotherapy. Immunology 2023;168:30-48. [Crossref] [PubMed]
- Bhat P, Leggatt G, Waterhouse N, et al. Interferon-γ derived from cytotoxic lymphocytes directly enhances their motility and cytotoxicity. Cell Death Dis 2017;8:e2836. [Crossref] [PubMed]
- Bassani B, Baci D, Gallazzi M, et al. Natural Killer Cells as Key Players of Tumor Progression and Angiogenesis: Old and Novel Tools to Divert Their Pro-Tumor Activities into Potent Anti-Tumor Effects. Cancers (Basel) 2019;11:461. [Crossref] [PubMed]
- Borst J, Ahrends T, Bąbała N, et al. CD4+ T cell help in cancer immunology and immunotherapy. Nat Rev Immunol 2018;18:635-47. [Crossref] [PubMed]