Analysis of C-X-C motif chemokine receptors in breast cancer: potential value in immunotherapy and prognostic prediction
Highlight box
Key findings
• CXCR6 expression is closely related to CD8+ T cell infiltration in BRCA.
• Patients with high CXCR6 expression and high PD1 expression have better survival in BRCA.
• The CXCRs were divided into 2 molecular subgroups according to CXCR expression patterns and we constructed a CXCR score to predict overall survival.
What is known and what is new?
• We have known that the expression patterns of CXCR family members in BC patients. CXCR6 expression is closely related to CD8+ T cell infiltration in melanoma.
• For the first time, the CXCR family was divided into two subgroups based on CXCR expression patterns in BRCA and explored the differences in prognosis, immune infiltration, functional enrichment, hallmark and immune response between the two subgroups. The CXCR subgroups were established to pave the way for individualized immunotherapy for BRCA.
What is the implication, and what should change now?
• We provide a novel idea for the prognosis and immunotherapy of BRCA, which will guide clinical individualized treatment.
Introduction
Breast cancer (BRCA) has become the most frequently diagnosed cancer worldwide, with an estimated 2.3 million new cases in 2020, accounting for 11.7% of all new cancers (1). Its annual upward trend poses a serious threat to women’s health. As the space upon which tumor progression and metastasis depend, the tumor microenvironment (TME) of BRCA consists not only of BRCA cells but also a complex network of vascular endothelial cells, fibroblasts, immune cells, extracellular matrix, and a range of soluble and other growth factors (2). Among them, the complexity and diversity of tumor-infiltrating immune cells are closely related to the prognosis and clinical outcome of tumor patients (3). Based on the degree of T-cell infiltration, the TME can be categorized into “cold” tumors and “hot” tumors (4). “Cold” tumors are characterized by the absence or rejection of T cells, whereas “hot” tumors are characterized by T cell infiltration and immune activity (5). However, due to low lymphocyte infiltration, most BRCAs have a “cold” immunologic nature (6,7). Therefore, T-cell infiltration and activation in TME are critical to predicting and guiding immunotherapeutic responses.
Three stages constitute the tumor immune editing process, namely, elimination, homeostasis, and escape, among which the immune escape mechanism is critical in suppressing the antitumor immune response (8). Specifically, by releasing signals to prevent dendritic cells (DCs) from presenting tumor antigens or directly inhibiting the conversion of naive T cells into mature T cells, tumor cells can evade the function of immune recognition and killing (9). This mechanism can inhibit the effective anti-tumor immune response of the body. Therefore, tumor immune escape plays a crucial role in tumorigenesis and progression and has been identified as one of the hallmarks of cancer (10). Immune checkpoints are protein molecules that regulate the body’s immune function, and their overexpression has a negative regulatory effect on the immune system. When immune cells are continuously activated, immune checkpoints are overexpressed and mediate immunosuppression, preventing cytotoxic T lymphocytes (CTLs) from functioning and ultimately promoting the immune escape of tumor cells (11). However, immune checkpoint inhibitors (ICIs) can target critical factors leading to immune escape, eliminate signals that inhibit T-cell activation, accurately identify tumor antigens to establish an effective anti-tumor T-cell response, and destroy tumor cells (12,13). In addition, many ICIs, including cytotoxic T-lymphocyte antigen-4 (CTLA-4) and programmed cell death protein 1 (PD-1)/programmed cell death protein-ligand 1 (PD-L1), are immunotherapeutic tools that have emerged in recent years (14). Although ICIs have primarily been shown to prolong the survival time of patients with “hot” tumors, the overall effectiveness and proportion of beneficiaries of ICI therapy for “cold” tumor BRCA, particularly the more aggressive triple-negative BRCA, is quite slight (15). Therefore, to help patients access accurate and effective treatment options, further research and identification of biomarkers that can predict the efficacy of ICI in BRCA is necessary so that patients with a drug response can obtain the best treatment option as soon as feasible.
C-X-C motif chemokine receptors (CXCRs) are G protein-coupled receptors (GPCRs) consisting of 7 mutually parallel, tightly arranged transmembrane-spanning segments that have important implications for cancer progression and prognosis (16). They are expressed not only on inflammatory cells such as macrophages and neutrophils, but also on endothelial cells and some tumor-derived epithelial cells (17,18). When CXCRs bind to their homologous ligands, the receptor conformation is altered, triggering a signaling pathway mediated by G proteins or β-arrestins (19). In the TME of BRCA, CXCRs and their ligands can not only directly promote tumor cell growth or inhibit apoptosis, but can also indirectly maintain the immunosuppressive microenvironment by affecting angiogenesis and recruiting immune cells (20-22). A previous study has shown that targeting CXCRs can provide a unique strategy to promote infiltration of CD8+ T cells, thereby facilitating the conversion of “cold” tumors into “hot” tumors and inhibiting tumor growth in concert with anti-PD-1 therapy (23). Thus, CXCRs are emerging as new targets for immunotherapy. New therapeutic options in BRCA immunotherapy can be generated through comprehensive studies of chemokine receptors.
Current biomarkers play a significant role in the clinical management of BRCA (24). However, some classical prognostic markers of breast cancer do not apply to most patients, and their applications are limited, so there is still a need to find new biomarkers for prognosis prediction and treatment of patients (25,26). Combining multiple prognostic markers to establish breast cancer prognostic models has dramatically increased the population of biomarker applications. However, the detection accuracy remains unsatisfactory. For example, the area under curve (AUC) of the BRCA prognostic model established by Zhou et al. was 0.732 (27). Combining multiple prognostic markers to develop breast cancer prognostic, advances in RNA-based technologies has improved the understanding of the complexity and diversity of the tumor immune microenvironment and its impact on therapy. RNAs or RNA regulators associated with the tumor immune microenvironment may be promising targets for anticancer immunotherapy (28). Thus, our CXCR family genes-based prognostic model not only improved the prognostic accuracy of the model but also provided potential drug therapy targets for breast cancer by screening independent prognostic markers. We present the following article in accordance with the TRIPOD reporting checklist (available at https://atm.amegroups.com/article/view/10.21037/atm-22-6056/rc).
Methods
Data acquisition
The workflow diagram of this study was shown in Figure S1. A total of 1,095 BRCA patients with transcriptome data, mutation data, and clinical information were downloaded from The Cancer Genome Atlas (TCGA) data portal (http://cancergenome.nih.gov/). The detailed information on the samples is presented in Table S1. The RNA-sequencing (RNA-seq) data of 1,113 cancer samples and 113 paraneoplastic samples were used for differential analysis. The RNA-seq data of 1,095 patients with primary location were used for subgroup analysis; 1,080 patients with complete follow-up information were used for constructing and validating the prognostic model. Gene expression data were log2-transformed for subsequent analysis. The study was conducted in accordance with the Declaration of Helsinki (as revised in 2013).
Immune infiltration analysis
The Estimation of STromal and Immune cells in MAlignant Tumor tissues using Expression data (ESTIMATE) algorithm was used to assess the proportion of stromal cells, immune cells, and tumor purity (29) associated with CXCR family members in BRCA TME. The quanTIseq, Cell-type Identification by Estimating Relative Subsets of RNA Transcripts (CIBERSORT), and Estimating the Proportion of Immune and Cancer cells (EPIC) algorithms were used to infer the infiltration levels of immune cells associated with CXCR family members in the TME (30,31). In addition, TIP Tracking Tumor Immunophenotype (TIP; http://biocc.hrbmu.edu.cn/TIP/), as a service to address tumor immunophenotype analysis, was used to download the activation levels of the 7-step antitumor immune cycle (32).
Subgroup classification and functional enrichment analysis
Via ConsensusClusterPlus, a consensus clustering algorithm (33) was used to classify BRCA patients into two subgroups based on gene expression of the CXCR family members. Each time, 80% of the samples were taken, and k-means clustering was repeated 500 times. Finally, the optimal number of clusters was determined based on the cumulative distribution function (CDF), and stable clustering results were selected. Differentially expressed genes (DEGs) within different subgroups were interpreted by the R package DEseq (34). Genes with |Log2FoldChange| >1 and adjusted P value <0.05 were considered DEGs. We then used the R package clusterProfiler to perform Gene Ontology (GO)/Kyoto Encyclopedia of Genes and Genomes (KEGG) pathways and gene set enrichment analysis (GSEA) functional enrichment analysis to analyze the functional characteristics of DEGs (35,36). Single sample GSEA (ssGSEA) was used to measure cancer hallmark activity in tumor samples, and the difference in hallmark activity between the two subgroups was analyzed using the Wilcoxon test.
Construction and validation of the prognostic signature
We equally randomly divided 1,080 patients with complete follow-up information from the TCGA cohort into the training and test sets according to a 1:1 ratio, and performed survival analysis, consistency analysis, standard curve, and decision curve. Least absolute shrinkage and selection operator (LASSO) Cox regression analysis was used to construct CXCR family genes based on prognostic signature in the training set using the glmnet R package. The applicability of this signature was validated in the validation test set. In addition, risk scores were derived from regression coefficients (risk score = sum of Cox coefficients of gene Gi X expression value of gene Gi). We defined the risk score as CXCR score using the following equation:
Patients were divided into high- and low-risk groups based on the median risk score. Kaplan-Meier survival curves were depicted within the two risk groups, and the log-rank test was used to determine survival differences. Time-dependent receiver operating characteristic (ROC) curves obtained with the pROC package were used to assess the predictive accuracy of the signatures. The slope of the ROC curve at any point is equal to the ratio of the two density functions describing the distribution of independent variables in the poor and good prognosis populations, i.e., the likelihood ratio. A monotonically increasing likelihood ratio corresponds to a concave ROC curve, and the AUC summarizes the entire position of the ROC curve rather than depending on a specific working point. The AUC is a validated composite measure of sensitivity and specificity, and models with an AUC greater than 0.7 are generally considered to have some prognostic power. Pooled analysis of clinical variables was then performed with the “rms” package to display nomograms. Calibration curves were used to assess the predictive validity of the nomograms. Decision curve analysis (DCA) was also performed to measure the clinical benefit of the nomogram. As a result of using the nomogram as a screening tool, the net benefit of determining whether an intervention should be performed on a genuinely high-risk patient was determined.
Statistical analysis
The above statistics and analyses were performed using R software 4.1.3 (The R Foundation for Statistical Computing, Vienna, Austria), and all figures were stitched by Adobe Illustrator (Adobe Systems, Munich, Germany) software. The Wilcoxon rank sum test was used for the comparative analysis of differences in box plots. Binary variables were compared using the Pearson χ2 test. Logistic regression results P<0.05 were considered to be statistically significant (P<0.05). The Spearman correlation coefficient was used for correlation analysis. A chi-square test (using Fisher’s exact test, if necessary) was used to compare the clinical factors of the two subgroups. Clinical characteristics affecting clustering were assessed using multivariate logistic regression analysis, and survival curves were plotted using Kaplan-Meier estimation methods. Cox proportional hazards regression analysis was used to determine factors associated with survival. All hypothesis tests were 2-sided, P values for multiple testing were corrected for false discovery rate (FDR), and adjusted P values below 0.05 were considered statistically significant.
Results
CXCRs regulate the TME of BRCA
The expression patterns of the 6 CXCRs were measured in BRCA and normal samples. Compared to normal samples, CXCR3/4/5 mRNA expression levels were upregulated 1.5-fold (P value =8.3e-12), 1.2-fold (P value =1.2e-20), and 3.3-fold (P value =3.0e-09) in BRCA samples. CXCR1 and CXCR2 mRNA expression levels were downregulated 0.7-fold (P value =3.4e-07) and 0.4-fold (P value =1.4e-42). (Figure 1A). We then analyzed the correlation of CXCRs with the immune score, stromal score, and tumor purity (Figure 1B). CXCRs exhibited higher immune/stromal scores and lower tumor purity, indicating that CXCRs are significantly associated with TME and are immune-related genes in TME. We then used CIBERSORT, EPIC, and quanTIseq to infer further correlation of CXCRs with immune infiltrating cells (Figure 1C, Figure S2) and the association with the TIP immune cycle in TME (Figure 1D). We discovered that CXCR1 and CXCR2 were significantly associated with M2 macrophages, whereas CXCR3 was directly associated with CD8+ T cells and Tregs. CXCR5 was significantly associated with B cells, CD8+ T cells, and Tregs. CXCR6 was significantly associated with CD8+ T cells, macrophages, and Tregs. Meanwhile, CXCR family genes were consistently associated with most immune circulating cells, such as CD4+ T cells, CD8+ T cells, DCs, macrophages, and natural killer (NK) cells. In particular, the association of CXCR6 with CD8+ T cells was remarkable, and we further confirmed the positive association of CXCR6 with CD8+ T cells by several immune infiltration algorithms (Figure 1E).
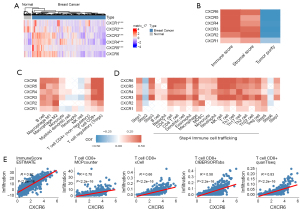
CXCRs and immunotherapy
We then analyzed the impact of each CXCR on the prognosis of BRCA patients (Figure 2A-2F). High expression of CXCR3, CXCR5, and CXCR6 was associated with a favorable prognosis. According to a complementary study of 6 CXCR family genes and immunotherapy targets (Figure 2G-2L, Figure S3), patients with higher expression of PD-1 and CXCR6 had an increased probability of survival (Figure 2L). In contrast, there was no difference in patient survival when these genes were combined with PD-L1 (Figure S3), suggesting that CXCR6 further differentiated the efficacy of patients treated with PD-1 inhibitors.
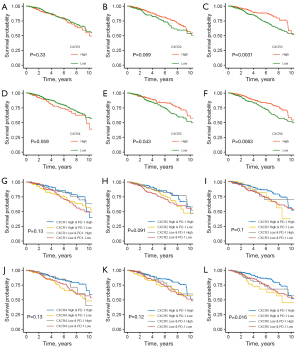
The characteristics of CXCR subgroups in BRCA
We first analyzed the correlation within CXCRs (Figure 3A). CXCR6 and CXCR3 showed a significant positive correlation, and CXCR2, CXCR4, and CXCR5 were also positively correlated. Then, we used a consistent clustering method to classify BRCA patients into two subgroups based on CXCRs. A total of 594 patients were classified into subgroup A, and 501 patients were classified into subgroup B (Figure 3B, Figure S4). Subsequently, we demonstrated that patients in subgroup B had a better prognosis than those in subgroup A (Figure 3C). Further investigation revealed a significant upregulation of CXCR3 and CXCR6 in subgroup B and a significant upregulation of CXCR1/2/4/5 in subgroup A (Figure 3D). It was observed that patients in subgroup B were strongly associated with the C2 [interferon gamma (IFN-γ) dominant] immune subtype. Meanwhile, CXCR family subgroups were associated with pathologic stage, TCGA subtype, and immune subtype. (Figure 3D and Table S2). In addition, different subgroups exhibited different immune microenvironment characteristics (Figure 3E,3F). For instance, subgroup B had higher levels of lymphocyte infiltration, IFN-γ response, M1 macrophages, and CD8+ T cells, which strongly activated the immune microenvironment, whereas subgroup A had higher levels of M2 macrophages. In addition, the results showed that the expression level of immune checkpoints was significantly higher in subgroup B than in subgroup A (Figure 3G).
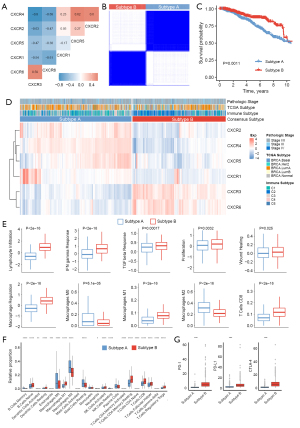
Functional enrichment and hallmark analysis of CXCR subgroups
Next, we analyzed the differences between the 2 subgroups. A total of 595 genes were upregulated in subgroup B and 170 genes were upregulated in subgroup A (Figure 4A and https://cdn.amegroups.cn/static/public/10.21037atm-22-6056-1.xlsx). The GSEA analysis revealed that the NF-kappa B signaling pathway, T cell receptor signaling pathway, and cell adhesion molecules were significantly enriched in subgroup B, whereas the ascorbic acid and aldehyde metabolism signaling pathways were significantly enriched in subgroup A (Figure 4B). The GO functional enrichment analysis revealed that various immune activation pathways, such as T cell activation, leukocyte cell-cell adhesion, and leukocyte-mediated immunity, were significantly enriched in subgroup B (Figure 4C). Some metabolic pathways, such as amino acid transport regulation, catecholamine secretion, and signaling release, were significantly enriched in subgroup A (Figure 4D). In addition, CXCR subgroups were detected, involving many hallmarks (Figure 4E). For example, subgroup B had higher hallmark activities such as IFN-γ response, KRAS signaling, and apoptosis. Conversely, subgroup A had higher hallmark activities such as oxidative phosphorylation, protein secretion, and glycolysis.
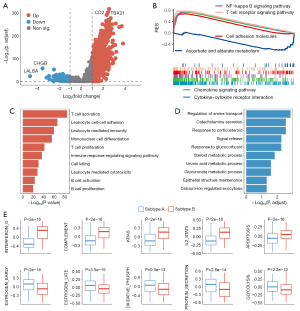
Establishment of the prognostic signature based on CXCRs
Patients with BRCA were randomly divided into a training set (50%) and a validation set (50%). We constructed the signature in training set by LASSO regression analysis (Figure 5A). Risk score = 0.455 × CXCR1 expression + 0.251 × CXCR4 expression + 0.837 × CXCR5 expression + (−0.243) × CXCR6 expression (Figure 5B). Patients were divided into low- and high-risk groups according to the median risk score (Figure 5C). Patients in the high-risk group had a lower survival rate (Figure 5D). Univariate and multivariate Cox analysis identified risk score as an independent prognostic factor (Table S3). In addition, we assessed the model’s predictive capacity using time-dependent ROC curves, the ROC curves showed the prognostic ability of the training set for OS (3-year AUC =0.89, 5-year AUC =0.79, and 8-year AUC =0.75), which showed good survival time prediction (Figure 5E). The corresponding analysis was repeated in the validation group, the ROC curves showed the prognostic ability of the validation sets for OS (3-year AUC =0.86, 5-year AUC =0.76, and 8-year AUC =0.70) (Figure 5F-5H).
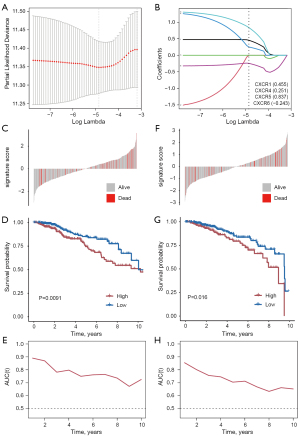
CXCR score as a prognostic marker
To further explore the value of the CXCR score in the clinical prognosis of BRCA, we integrated the CXCR score and clinical factors in the training set to construct a nomogram (Figure 6A). Calibration curves were used to assess the predictive accuracy of 3-, 5-, and 8-year survival rates. It is important to note that the predicted risk was essentially similar to the actual risk, which validated the reliability of our bar graph (Figure 6B). We performed DCA on the training set to assess the clinical benefit of the nomogram (Figure 6C). Regarding 3-year survival, the curves showed that the nomogram showed more clinical benefit than all interventions, no interventions, and focused competing intervention strategies based on various clinical intervention indicators. We also observed that the nomogram had excellent predictive power in the test set (Figure 6D,6E).
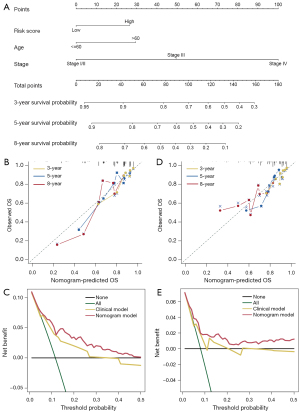
Discussion
As one of the 4 significant subfamilies of chemokine receptors, CXCRs play an essential role in the TME by regulating tumor leukocyte trafficking and influencing the proliferation, migration, and progression of various tumor cells (37-39). Nevertheless, the underlying mechanisms of CXCRs in the BRCA microenvironment remain to be investigated in depth. Initially, using the TCGA database and multiple public datasets, our investigations revealed a strong association between CXCR family members and the BRCA microenvironment. Subsequently, we identified a link between the expression of CXCR family members and various immune cells in the TME, and intriguingly, we found a relationship between CXCR6 and CD8+ T cells. According to previous studies, CD8+ T cells are one of the most effective immune defense cells in tumor tissues, and the high abundance of CD8+ T cells with killing function is a favorable prognostic indicator for BRCA (40,41). Also, increased infiltration of CD8+ T cells is critical for the therapeutic response to ICIs. Therefore, the correlation between CXCR6 and CD8+ T cells may provide new evidence for ICI therapy. To determine whether CXCRs expression affects the efficacy of ICIs, we analyzed the prognosis of PD-1 or PD-L1 co-expression with CXCR family members. Surprisingly, patients with high CXCR6 expression and high PD-1 expression had better survival, suggesting that CXCR6 could be considered as a biomarker to differentiate anti-PD-1 efficacy further. CXCR6 was previously reported to be the strongest overall survival (OS) predictor of all chemokine receptor genes in melanoma, recruiting CTLs and assisting their localization within the TME to receive necessary survival signals that trigger immune tumor control (42). High CXCR6 expression in the TME mediated increased tissue damage and high lethality of CD8+ T cells, mediating more effective antitumor activity and enhanced response to anti-PD-1 therapy (43). Thus, maintaining CXCR6 expression plays a crucial role in tumor immunotherapy.
In the following experiments, to detect complete classification criteria to differentiate the prognosis of BRCA patients according to the expression of different CXCR family genes, we divided BRCAs into two subgroups by consistent cluster analysis, namely subgroup A with high expression of CXCR1, CXCR2, CXCR4, and CXCR5 and subgroup B with increased expression of CXCR3 and CXCR6. In addition, the survival rate of patients in subgroup B was significantly better than that in subgroup A. CXCR3 is a chemokine receptor possessing 3 ligands, chemokine (C‐X‐C motif) ligand 9 (CXCL9), chemokine (C‐X‐C motif) ligand 10 (CXCL10), and chemokine (C‐X‐C motif) ligand 11 (CXCL11), which is mainly expressed on CD4+ T cells and CD8+ T cells and plays an essential role in regulating antitumor immune responses and immunotherapy (44). In our study, CXCR3 is considered an integral component of subtype B. It can regulate favorable prognosis for the proliferation and activation of immune cells, especially T cells, in the immune microenvironment, which enhances the response to ICI and is closely associated with the response to IFN-γ. It has been shown that CXCR3 promotes the proliferation of CD8+ T cells in malignant tumors in the presence of PD-1 suppression, affecting the efficacy of anti-PD-1 therapies (45). After the knockdown of CXCR3 in tumor-bearing mice, anti-PD-1 treatment failed to produce tumor-suppressive effects (46). Thus, increasing the intratumor activity of the CXCR3 chemokine system could promote enhanced clinical efficacy of ICIs. It has also been reported that in the context of IFN-γ, CXCR3 ligands could serve as novel biomarkers of anti-PD-1/PD-L1 therapeutic sensitivity, not only recruiting and triggering effector CD8+ T cells but also inhibiting tumor angiogenesis (47). Thus, IFN-γ is essential for the upregulation of CXCR3 ligand expression in ICI-treated tumor tissues and is required for PD-1/PD-L1 inhibition to exert anti-tumor and anti-angiogenic effects in vivo. This is consistent with our study that subgroup B had higher level of IFN-γ response and is crucial for ICI treatment.
Our study also identified poor prognostic indicators for subgroup A-related genes significantly associated with glycolysis. As an essential member of subgroup A, CXCR4 plays a crucial role in promoting tumor cell proliferation and mediating the suppressive effects of immune cells. According to recent literature, CXCR4 plays a significant role in metabolic reprogramming (48). Specifically, the binding of CXCR4 to its ligand triggers the recruitment of β-arrestin molecules, which then initiates β-arrestin-dependent signaling through the activation of extracellular signal-regulated kinases 1 and 2 (ERK1/2). Meanwhile, phosphorylated ERK1/2 drives pyruvate kinase M2 (PKM2) to upregulate glycolytic genes, triggering the pentose phosphate pathway and promoting glucose metabolism to a greater extent reshaping the metabolic reprogramming of cancer cells (49). Furthermore, by comparing the characteristics of 22 infiltrating immune cells in subgroups A and B, we found that the poor prognostic factors in subgroup A were closely associated with M2 polarization. Tumor-associated macrophages (TAMs) are considered the most abundant immune-associated cells in the TME, showing high plasticity and heterogeneity, exhibiting M1-like tumor suppressor phenotype and M2-like tumor-promoting phenotype through different activation pathways during maturation and differentiation (50). In the multiple myeloma (MM) microenvironment, signaling molecules involved in the JAK/STAT pathway and the Wnt signaling pathway mediate M2 polarization by increasing CXCL12/CXCR4 expression in monocytes. In contrast, inhibition of JAK1/2 not only reduced the expression of genes involved in the JAK/STAT pathway, but also inhibited the accumulation of CXCR4 ligand in TME to block M2 polarization (51). In pancreatic ductal adenocarcinoma (PDAC), CXCR4 and PD-1 can interact directly, and the application of CXCR4-selective antagonists increases the expression of PD-1 on the cell surface. Based on this case, the application of PD-1 inhibitors will prevent the migration of PDAC cells (52). Thus, combined PD-1 and CXCR4 would provide further clinically beneficial therapeutic options for tumor patients.
Finally, to predict the prognostic risk of CXCR family genes expression in BRCA, we comprehensively and systematically constructed a risk scoring system for CXCR family members in BRCA by LASSO Cox regression model, assessed the survival differences between high- and low-risk groups, applied ROC to assess the predictive power of the model. The risk scoring system was found to assess individual survival with strong predictive power. To further evaluate the impact of the CXCR score on the clinical prognosis of BRCA, a nomogram model calibration curve was constructed using the integration of CXCR score and clinical characteristics to assess the predictive accuracy of 3-, 5-, and 8-year survival. Ultimately, the predicted risks were used to analyze further the impact of the CXCR score on the clinical prognosis of BRCA. The integration of CXCR score and clinical characteristics was used to generate nomogram model calibration curves to test the predictive accuracy of 3-, 5-, and 8-year survival rates. Ultimately, the predicted risk was highly similar to the actual risk, thus further demonstrating the accuracy of the CXCR score and providing additional clinical benefit to BRCA patients.
In summary, the results of our study explored genetic and transcriptional levels of CXCRs in BRCA and suggested that CXCRs play a crucial role in remodeling the BRCA microenvironment. These results strengthened our understanding of the tumor immune microenvironment and may provide a basis for CXCRs to predict prognosis and develop better individualized immunotherapies for BRCA. A limitation of our research is the lack of experimental verification, and we will proceed to conduct a series of experiments to verify our results.
Conclusions
CXCR genes were associated with immune infiltration and survival in BRCA patients, and our CXCR-based prognostic model could better predict the prognosis of patients with BRCA and provide potential immunotherapy targets for clinical purposes.
Acknowledgments
Funding: This work was supported by the National Natural Science Foundation of China (Nos. 81730074, 81672599, and 82002781); China Postdoctoral Science Foundation (No. 2018M641858); Hei Long Jiang Postdoctoral Foundation (No. LBH-Z18115); Knowledge Innovation Program of Harbin Medical University (No. 31041180112); and The National Science Foundation of Heilongjiang Province of China for Returnees (No. LC2017037).
Footnote
Reporting Checklist: The authors have completed the TRIPOD reporting checklist. Available at https://atm.amegroups.com/article/view/10.21037/atm-22-6056/rc
Conflicts of Interest: All authors have completed the ICMJE uniform disclosure form (available at https://atm.amegroups.com/article/view/10.21037/atm-22-6056/coif). The authors have no conflicts of interest to declare.
Ethical Statement: The authors are accountable for all aspects of the work in ensuring that questions related to the accuracy or integrity of any part of the work are appropriately investigated and resolved. The study was conducted in accordance with the Declaration of Helsinki (as revised in 2013).
Open Access Statement: This is an Open Access article distributed in accordance with the Creative Commons Attribution-NonCommercial-NoDerivs 4.0 International License (CC BY-NC-ND 4.0), which permits the non-commercial replication and distribution of the article with the strict proviso that no changes or edits are made and the original work is properly cited (including links to both the formal publication through the relevant DOI and the license). See: https://creativecommons.org/licenses/by-nc-nd/4.0/.
References
- Sung H, Ferlay J, Siegel RL, et al. Global Cancer Statistics 2020: GLOBOCAN Estimates of Incidence and Mortality Worldwide for 36 Cancers in 185 Countries. CA Cancer J Clin 2021;71:209-49. [Crossref] [PubMed]
- Binnewies M, Roberts EW, Kersten K, et al. Understanding the tumor immune microenvironment (TIME) for effective therapy. Nat Med 2018;24:541-50. [Crossref] [PubMed]
- Giraldo NA, Becht E, Remark R, et al. The immune contexture of primary and metastatic human tumours. Curr Opin Immunol 2014;27:8-15. [Crossref] [PubMed]
- Zhang J, Huang D, Saw PE, et al. Turning cold tumors hot: from molecular mechanisms to clinical applications. Trends Immunol 2022;43:523-45. [Crossref] [PubMed]
- Jerby-Arnon L, Shah P, Cuoco MS, et al. A Cancer Cell Program Promotes T Cell Exclusion and Resistance to Checkpoint Blockade. Cell 2018;175:984-997.e24. [Crossref] [PubMed]
- Pellegrino B, Tommasi C, Cursio OE, et al. A review of immune checkpoint blockade in breast cancer. Semin Oncol 2021;48:208-25. [Crossref] [PubMed]
- Tokumaru Y, Joyce D, Takabe K. Current status and limitations of immunotherapy for breast cancer. Surgery 2020;167:628-30. [Crossref] [PubMed]
- Mittal D, Gubin MM, Schreiber RD, et al. New insights into cancer immunoediting and its three component phases--elimination, equilibrium and escape. Curr Opin Immunol 2014;27:16-25. [Crossref] [PubMed]
- Shahverdi M, Masoumi J, Ghorbaninezhad F, et al. The modulatory role of dendritic cell-T cell cross-talk in breast cancer: Challenges and prospects. Adv Med Sci 2022;67:353-63. [Crossref] [PubMed]
- Hanahan D, Weinberg RA. Hallmarks of cancer: the next generation. Cell 2011;144:646-74. [Crossref] [PubMed]
- Gaynor N, Crown J, Collins DM. Immune checkpoint inhibitors: Key trials and an emerging role in breast cancer. Semin Cancer Biol 2022;79:44-57. [Crossref] [PubMed]
- Wang DR, Wu XL, Sun YL. Therapeutic targets and biomarkers of tumor immunotherapy: response versus non-response. Signal Transduct Target Ther 2022;7:331. [Crossref] [PubMed]
- Wei SC, Duffy CR, Allison JP. Fundamental Mechanisms of Immune Checkpoint Blockade Therapy. Cancer Discov 2018;8:1069-86. [Crossref] [PubMed]
- Andrews LP, Yano H, Vignali DAA. Inhibitory receptors and ligands beyond PD-1, PD-L1 and CTLA-4: breakthroughs or backups. Nat Immunol 2019;20:1425-34. [Crossref] [PubMed]
- Carlino F, Diana A, Piccolo A, et al. Immune-Based Therapy in Triple-Negative Breast Cancer: From Molecular Biology to Clinical Practice. Cancers (Basel) 2022.
- Mollica Poeta V, Massara M, Capucetti A, et al. Chemokines and Chemokine Receptors: New Targets for Cancer Immunotherapy. Front Immunol 2019;10:379. [Crossref] [PubMed]
- Zlotnik A, Yoshie O, Nomiyama H. The chemokine and chemokine receptor superfamilies and their molecular evolution. Genome Biol 2006;7:243. [Crossref] [PubMed]
- Do HTT, Lee CH, Cho J. Chemokines and their Receptors: Multifaceted Roles in Cancer Progression and Potential Value as Cancer Prognostic Markers. Cancers (Basel) 2020;12:287. [Crossref] [PubMed]
- Stone MJ, Hayward JA, Huang C, et al. Mechanisms of Regulation of the Chemokine-Receptor Network. Int J Mol Sci 2017;18:342. [Crossref] [PubMed]
- Liu H, Yang Z, Lu W, et al. Chemokines and chemokine receptors: A new strategy for breast cancer therapy. Cancer Med 2020;9:3786-99. [Crossref] [PubMed]
- Xu H, Lin F, Wang Z, et al. CXCR2 promotes breast cancer metastasis and chemoresistance via suppression of AKT1 and activation of COX2. Cancer Lett 2018;412:69-80. [Crossref] [PubMed]
- Wu J, Li L, Liu J, et al. CC chemokine receptor 7 promotes triple-negative breast cancer growth and metastasis. Acta Biochim Biophys Sin (Shanghai) 2018;50:835-42. [Crossref] [PubMed]
- Bockorny B, Semenisty V, Macarulla T, et al. BL-8040, a CXCR4 antagonist, in combination with pembrolizumab and chemotherapy for pancreatic cancer: the COMBAT trial. Nat Med 2020;26:878-85. [Crossref] [PubMed]
- Thammineni KL, Thakur GK, Kaur N, et al. Significance of MMP-9 and VEGF-C expression in North Indian women with breast cancer diagnosis. Mol Cell Biochem 2019;457:93-103. [Crossref] [PubMed]
- Wu QW, Yang QM, Huang YF, et al. Expression and clinical significance of matrix metalloproteinase-9 in lymphatic invasiveness and metastasis of breast cancer. PLoS One 2014;9:e97804. [Crossref] [PubMed]
- Eikesdal HP, Yndestad S, Elzawahry A, et al. Olaparib monotherapy as primary treatment in unselected triple negative breast cancer. Ann Oncol 2021;32:240-9. [Crossref] [PubMed]
- Zhou W, Pang Y, Yao Y, et al. Development of a Ten-lncRNA Signature Prognostic Model for Breast Cancer Survival: A Study with the TCGA Database. Anal Cell Pathol (Amst) 2020;2020:6827057. [Crossref] [PubMed]
- Pandey PR, Young KH, Kumar D, et al. RNA-mediated immunotherapy regulating tumor immune microenvironment: next wave of cancer therapeutics. Mol Cancer 2022;21:58. [Crossref] [PubMed]
- Yoshihara K, Shahmoradgoli M, Martínez E, et al. Inferring tumour purity and stromal and immune cell admixture from expression data. Nat Commun 2013;4:2612. [Crossref] [PubMed]
- Newman AM, Liu CL, Green MR, et al. Robust enumeration of cell subsets from tissue expression profiles. Nat Methods 2015;12:453-7. [Crossref] [PubMed]
- Finotello F, Mayer C, Plattner C, et al. Molecular and pharmacological modulators of the tumor immune contexture revealed by deconvolution of RNA-seq data. Genome Med 2019;11:34. [Crossref] [PubMed]
- Xu L, Deng C, Pang B, et al. TIP: A Web Server for Resolving Tumor Immunophenotype Profiling. Cancer Res 2018;78:6575-80. [Crossref] [PubMed]
- Wilkerson MD, Hayes DN. ConsensusClusterPlus: a class discovery tool with confidence assessments and item tracking. Bioinformatics 2010;26:1572-3. [Crossref] [PubMed]
- Anders S, Huber W. Differential expression analysis for sequence count data. Genome Biol 2010;11:R106. [Crossref] [PubMed]
- Huang da W. Sherman BT, Lempicki RA. Bioinformatics enrichment tools: paths toward the comprehensive functional analysis of large gene lists. Nucleic Acids Res 2009;37:1-13. [Crossref] [PubMed]
- Subramanian A, Tamayo P, Mootha VK, et al. Gene set enrichment analysis: a knowledge-based approach for interpreting genome-wide expression profiles. Proc Natl Acad Sci U S A 2005;102:15545-50. [Crossref] [PubMed]
- Xun Y, Yang H, Li J, et al. CXC Chemokine Receptors in the Tumor Microenvironment and an Update of Antagonist Development. Rev Physiol Biochem Pharmacol 2020;178:1-40. [Crossref] [PubMed]
- Peng W, Ye Y, Rabinovich BA, et al. Transduction of tumor-specific T cells with CXCR2 chemokine receptor improves migration to tumor and antitumor immune responses. Clin Cancer Res 2010;16:5458-68. [Crossref] [PubMed]
- Perissinotto E, Cavalloni G, Leone F, et al. Involvement of chemokine receptor 4/stromal cell-derived factor 1 system during osteosarcoma tumor progression. Clin Cancer Res 2005;11:490-7.
- Nelson MA, Ngamcherdtrakul W, Luoh SW, et al. Prognostic and therapeutic role of tumor-infiltrating lymphocyte subtypes in breast cancer. Cancer Metastasis Rev 2021;40:519-36. [Crossref] [PubMed]
- Baxevanis CN, Sofopoulos M, Fortis SP, et al. The role of immune infiltrates as prognostic biomarkers in patients with breast cancer. Cancer Immunol Immunother 2019;68:1671-80. [Crossref] [PubMed]
- Di Pilato M, Kfuri-Rubens R, Pruessmann JN, et al. CXCR6 positions cytotoxic T cells to receive critical survival signals in the tumor microenvironment. Cell 2021;184:4512-4530.e22. [Crossref] [PubMed]
- Wang B, Wang Y, Sun X, et al. CXCR6 is required for antitumor efficacy of intratumoral CD8+ T cell. J Immunother Cancer 2021;9:e003100. [Crossref] [PubMed]
- Karin N. CXCR3 Ligands in Cancer and Autoimmunity, Chemoattraction of Effector T Cells, and Beyond. Front Immunol 2020;11:976. [Crossref] [PubMed]
- Chow MT, Ozga AJ, Servis RL, et al. Intratumoral Activity of the CXCR3 Chemokine System Is Required for the Efficacy of Anti-PD-1 Therapy. Immunity 2019;50:1498-1512.e5. [Crossref] [PubMed]
- Chheda ZS, Sharma RK, Jala VR, et al. Chemoattractant Receptors BLT1 and CXCR3 Regulate Antitumor Immunity by Facilitating CD8+ T Cell Migration into Tumors. J Immunol 2016;197:2016-26. [Crossref] [PubMed]
- Mitsuhashi A, Kondoh K, Horikawa K, et al. Programmed death (PD)-1/PD-ligand 1 blockade mediates antiangiogenic effects by tumor-derived CXCL10/11 as a potential predictive biomarker. Cancer Sci 2021;112:4853-66. [Crossref] [PubMed]
- He Y, Wang X, Lu W, et al. PGK1 contributes to tumorigenesis and sorafenib resistance of renal clear cell carcinoma via activating CXCR4/ERK signaling pathway and accelerating glycolysis. Cell Death Dis 2022;13:118. [Crossref] [PubMed]
- Luker KE, Luker GD. The CXCL12/CXCR4/ACKR3 Signaling Axis Regulates PKM2 and Glycolysis. Cells 2022;11:1775. [Crossref] [PubMed]
- Boutilier AJ, Elsawa SF. Macrophage Polarization States in the Tumor Microenvironment. Int J Mol Sci 2021;22:6995. [Crossref] [PubMed]
- Chen H, Li M, Sanchez E, et al. JAK1/2 pathway inhibition suppresses M2 polarization and overcomes resistance of myeloma to lenalidomide by reducing TRIB1, MUC1, CD44, CXCL12, and CXCR4 expression. Br J Haematol 2020;188:283-94. [Crossref] [PubMed]
- Harper MM, Lin M, Cavnar MJ, et al. Interaction of immune checkpoint PD-1 and chemokine receptor 4 (CXCR4) promotes a malignant phenotype in pancreatic cancer cells. PLoS One 2022;17:e0270832. [Crossref] [PubMed]
(English Language Editor: J. Jones)