A retrospective diagnostic test study on circulating tumor cells and artificial intelligence imaging in patients with lung adenocarcinoma
Highlight box
Key findings
• FR+CTC and/or AI tumor volume are independent indicators of the invasiveness of LUAD, and the prediction model based on FR+CTC and AI tumor volume could assist in evaluating the invasiveness of LUAD, in further guiding therapy plan and promoting prognosis.
What is known and what is new?
• Either tumor volume or CTC has been proven effective in predicting tumor cell invasion. CTC count and tumor volume have synergistic action on the prognosis prediction of small cell lung cancer (SCLC).
• This manuscript adds a predictive model based on FR+CTC and/or AI tumor volume for diagnosing the invasiveness of LUAD.
What is the implication, and what should change now?
• This nomogram could be a basic diagnostic model for further study to construct a more accurate diagnostic method to help distinguish patients with LUAD and improve the prognosis. The model needs to be further tested in prospective, multicenter, and large-sample studies. Moreover, this model did not include commonly used clinical serum biomarkers, such as CEA, CA125, and ProGRP.
Introduction
Lung cancer is one of the leading causes of cancer deaths worldwide, and non-small cell lung carcinoma (NSCLC) accounts for 85% of all lung cancer (1). As the most common subtype, adenocarcinoma accounts for 40% of NSCLC (2). Adenocarcinoma is classified into preinvasive lesions [atypical adenomatous hyperplasia (AAH), adenocarcinoma in situ (AIS)], minimally invasive adenocarcinoma (MIA), and invasive pulmonary adenocarcinoma (IPA) (3), with each of these subtypes having different treatment and prognosis (4). For stage I lung adenocarcinoma (LUAD), the 5-year disease-free survival is 100% for AIS and MIA, compared with 38–86% for IPA (5,6). The standard surgical treatment for LUAD remains lobectomy, but non-IPA patients also have the option of limited surgical resection (7) Therefore, LUAD subtypes differ in their surgical approaches, follow-up management and prognoses. Clarifying invasive subtypes prior to operation is crucial (8). At present, pathological diagnosis has been regarded as the gold standard for the diagnosis of lung cancer, but its clinical application is limited due to the invasive procedure and it normally takes several days to get results (9). Imaging examinations such as positron emission tomography/computed tomography (PET/CT) shows limited sensitivity in identifying AIS (10), and although perioperative frozen section diagnosis typically has high specificity, it has limited sensitivity and usually overestimates tumor invasiveness (11). Thus, a non-invasive, precise and responsive diagnostic assessment between IPA and non-IPA is greatly needed. Currently, circulating tumor cell (CTC) analyses are primarily based on the expression of epithelial cell adhesion molecule (EpCAM). CellSearch, the only FDA-approved CTC assay system, specifically captures EpCAM-positive CTC in peripheral blood by immunomagnetic bead assay (12). However, tumor cells often undergo epithelial–mesenchymal transition (EMT) during progression, resulting in loss of EpCAM expression, which leads to missed CTC detection and affects the predictive value of CTC detection (13). The folate receptor (FR) is a transmembrane single-chain glycoprotein that is specifically highly expressed in most human tumor cells, such as ovarian, lung, and urological cancers, and rarely expressed in normal organs, so it can be used as an antitumor drug target (14). In the circulatory system, there is normally minimal cellular expression of FR, except for a small amount present on the surface of activated macrophages. Once epithelial cells become cancerous, its expression increases dramatically; 72–83% of lung tumor cells show high surface expression of FR, making it an ideal target for tumor cell screening (15). In a multicenter prospective study, preoperative FR+CTC testing was performed in 484 patients with small nodules ≤2 cm. The results showed that among several clinical indicators and tumor markers, only the maximum tumor diameter (MTD) and FR+CTC were independent predictors of tumor invasiveness, and tumor size combined with CTC could effectively distinguish between AIS and IPA with a sensitivity and specificity of 73–82% and 83–88%, respectively (16). A multiple linear regression analysis revealed that in lung adenocarcinoma patients, particularly in those with distant metastases, CTC numbers may be related to primary tumor volume (β=0.903, P=0.002), and it also indicated that the whole tumor volume was associated with disease-free survival (DFS) (17).
The most important imaging feature to judge the malignant nodule is its diameter. With the increase of the nodule size, the degree of invasion increases (18). Since the pulmonary nodule is not spherical, it is impossible to accurately estimate the size of the nodule according to the lung images. Therefore, a fully automatic measurement based on artificial intelligence could be used to assist in evaluating the volume of the pulmonary nodule. Research shows that the volume of IAC is significantly higher than that of non-invasive adenocarcinoma (18). Recent studies have reported artificial intelligence (AI) quantitative parameters based on pathologic features or CT images of squamous cell carcinoma, adenocarcinoma and small cell lung carcinoma (19,20). Further studies of AI quantitative parameters were based on CT images of pathological subtypes of LUAD with pure ground glass nodules (GGNs) or early LUAD (21-23). Others have reported CT-based deep learning models for invasiveness classification of LUAD (24-26).
The CTC levels in peripheral blood is very low and it is difficult to enrich and detect. The quality of AI deep learning model is related to the learning content input. Both detection methods have limitations. There are also some studies verified the synergy of the CTC count and tumor volume in small cell lung cancer (SCLC), in which show the advantage of the incorporation of the CTC count and the tumor volume for the prediction of prognosis to control for the interference of disease stage (27). However, FR+CTC combined with AI quantitative parameters based on CT images to distinguish pathological subtypes of LUAD has not been reported. We conducted a single-center, retrospective cohort to evaluate the value of FR+CTC combined with AI tumor volume as a predictive model for diagnosing the invasiveness of LUAD. We present the following article in accordance with the TRIPOD reporting checklist (available at https://atm.amegroups.com/article/view/10.21037/atm-22-5668/rc).
Methods
Study design
Observational clinical research was conducted. This retrospective diagnostic test study was done in The First Hospital of Lanzhou University between October 2019 and October 2021. We enrolled a total of 226 patients who were diagnosed with LUAD by biopsy. The pathological classification was based on the International Association for the Study of Lung Cancer (IASLC, 2011 edition), the American Thoracic Society (ATS) classification in 2011, and the European Respiratory Society (ERS) classification in 2011 (28) and the WHO classification of thoracic tumors (5th edition) 2021 (29). The inclusion criteria were: (I) FR+CTC detection before anticancer therapy; (II) AI imaging before anticancer therapy; and (III) definite histopathologic diagnosis. Exclusion criteria were: (I) incomplete key information (FR+CTC value, AI tumor volume or the number of nodule ); (II) ≥2 nodules; and (III) refusal of consent to use medical information for research purpose. Finally, 146 patients were included for the predictive model development. The study was conducted in accordance with the Declaration of Helsinki (as revised in 2013). The study was approved by the Ethics Committee of The First Hospital of Lanzhou University (No. LDYYLL2022-49). Individual consent for this retrospective analysis was waived.
Data collection and outcome definition
Clinicopathologic characteristics, including age, sex, nodule type, maximum density, smoking history, FR+CTC, tumor volume (by AI) and histologic type, were collected retrospectively from medical records. AIS + MIA and IPA patients were assigned as the non-invasion and invasion group, respectively.
Assessment of the pathological subtypes of LUAD
The pathological examination of the specimens was completed in the Department of Pathology of The First Hospital of Lanzhou University. The specimens of pulmonary nodules were fixed in formalin, embedded in paraffin, and stained with special methods. The final pathological diagnosis was determined by two pathologists with the title of attending physician or above. Among them, the invasive subtypes of lung adenocarcinoma include: AAH, mild to moderate atypical epithelial hyperplasia, no interstitial inflammatory reaction and fibrous hyperplasia; AIS showed that the tumor cells grew along the alveolar wall without interstitial, vascular or pleural infiltration; MIA adherent growth, showing isolation and invasion range ≤0.5 cm; IAC is lung adenocarcinoma with the invasion of interstitial, vascular and pleura, and invasion range >0.5 cm.
FR+CTC analysis
The GenoSaber Biotech Co. Ltd. (Shanghai, China) CytoploRare® Detection Kit was used to identify FR+CTC as previously described (30). In brief, 3 mL preoperative blood samples were collected into EDTA-containing anticoagulant tubes for each participant. The negative depletion method was used to collect rare FR+CTC. And the red and white blood cells in the sample were removed consecutively by a lysing buffer and immunomagnetic beads composed of anti-CD45 and anti-CD14. An FR-targeting oligonucleotide probe was subsequently used to label the enriched FR+CTC. FR+CTC were then quantified by the proprietary ligand-targeted polymerase chain reaction (PCR) technique (31). A standard curve for FR+CTC qualification was constructed using a set of standard calibrators that contained oligonucleotides [10−14 to 10−9 M, equivalent to 2–2×105 folate receptor units (FU) per 3 mL of peripheral blood]. The standard curve was used to generate the FU/3 mL blood value, which was utilized to represent the FR+CTC level in each sample.
AI tumor volume
Tumor volume was obtained by AI using the AI diagnostic system described previously (32,33), in brief, chest CT data and clinical data were imported into the Intelligent Auxiliary Screening system for the Pulmonary Nodules system (ScrynPro, Dianei Biotechnology Co., Ltd.) in the DICOM format for calculation of the pulmonary GGN image microfeatures, including nodule location, standard nodule volume (mm3), long nodule diameter (cm), short nodule diameter (cm), pure and solid ratio, nodule calcification (%), maximum nodule density [Hounsfield units (HU)], minimum nodule density (HU) and nodule center density (HU), and microvascular cluster (%). We choose standard nodule volume as “AI tumor volume”.
Statistical analysis
The normality test was first applied to the data. Normally distributed data are shown as mean ± standard derivation (SD), and non-normally distributed data are shown as median, interquartile range (IQR). Student’s t-test was used to analyze normally distributed data between groups. Categorical data are presented as counts and percentages and compared using the Chi-square test. Non-normally distributed data were analyzed by the Mann-Whitney U test. The receiver operating characteristic (ROC) curve was used to evaluate the diagnostic value of hematologic biomarkers based on the area under the curve (AUC).
The clinical data were used to construct univariate logistic regression and multivariate logistic regression models. A nomogram was drawn based on the multivariate logistic regression model, and its validity was evaluated by the calibration curve and Hosmer-Lemeshow goodness-of-fit test. Statistical analysis was conducted using R software version 4.1.0 (The Free Software Foundation, Boston, MA, USA). The “pROC” and “ggplot2” package were used to draw the ROC and calibration curves. The “generalhoslem” package was used to conduct the Hosmer-Lemeshow test. A two-sided P<0.05 was considered significant.
Results
Patients’ characteristics
According to the inclusion and exclusion criteria, 44 patients were excluded for missing key information, 12 for having ≥2 nodules, and 24 patients for refusing to consent to the usage of their medical information for research purposes. Of the 146 patients retrospectively enrolled, 41 and 105 had the pathologic classification of AIS + MIA and IPA, respectively. Most were >60 years old (41.1%). There were 96 males, 49 females and 1 without sex information. No significant difference was noted between the proportion of sexes and smoking history in the two groups (P=0.155, P=0.442, respectively). Nodule type, maximum density (HU), tumor volume (by AI), and FR+CTC showed significant differences between the AIS + MIA and IPA groups (P<0.05) (Table 1).
Table 1
Characteristic | AIS + MIA (N=41) | IPA (N=105) | P value | Overall (N=146) |
---|---|---|---|---|
Age, years | 0.103 | |||
Mean (SD) | 53.7 (11.0) | 57.7 (10.0) | 56.6 (10.4) | |
<60 | 29 (70.7%) | 57 (54.3%) | 86 (58.9%) | |
≥60 | 12 (29.3%) | 48 (45.7%) | 60 (41.1%) | |
Sex | 0.155 | |||
Female | 18 (43.9%) | 31 (29.5%) | 49 (33.6%) | |
Male | 23 (56.1%) | 73 (69.5%) | 96 (65.8%) | |
Missing | 0 (0%) | 1 (1.0%) | 1 (0.7%) | |
Nodule type | 0.035 | |||
Solid | 13 (31.7%) | 40 (38.1%) | 53 (36.3%) | |
mGGO | 9 (22.0%) | 16 (15.2%) | 25 (17.1%) | |
GGO | 9 (22.0%) | 6 (5.7%) | 15 (10.3%) | |
Missing | 10 (24.4%) | 43 (41.0%) | 53 (36.3%) | |
Maximum density (HU) | <0.01 | |||
Mean (SD) | −158 (271) | 18.7 (240) | −40.1 (263) | |
Missing | 10 (24.4%) | 43 (41.0%) | 53 (36.3%) | |
Smoking history | 0.442 | |||
Non-smoker | 35 (85.4%) | 77 (73.3%) | 112 (76.7%) | |
Former smoker | 0 (0%) | 2 (1.9%) | 2 (1.4%) | |
Smoker | 5 (12.2%) | 20 (19.0%) | 25 (17.1%) | |
Missing | 1 (2.4%) | 6 (5.7%) | 7 (4.8%) | |
Tumor volume (mm3) | <0.001 | |||
Median (25–75% IQR) | 274 (81, 619) | 527 (231, 2,457) | 486 (171, 1,336) | |
<118 | 12 (29.3%) | 4 (3.8%) | 16 (11.0%) | |
≥118 | 19 (46.3%) | 58 (55.2%) | 77 (52.7%) | |
Missing | 10 (24.4%) | 43 (41.0%) | 53 (36.3%) | |
FR+CTC (FU/3 mL) | 0.002 | |||
Median (25–75% IQR) | 9.4 (7.5, 13.8) | 12.80 (9.2, 18.0) | 12.1 (8.5, 16.4) | |
<9.75 | 22 (53.7%) | 27 (25.7%) | 49 (33.6%) | |
≥9.75 | 18 (43.9%) | 74 (70.5%) | 92 (63.0%) | |
Missing | 1 (2.4%) | 4 (3.8%) | 5 (3.4%) |
AIS, adenocarcinoma in situ; FR+CTC, folate-receptor-positive circulating tumor cells; FU, folate receptor units; GGO, ground-glass opacity; HU, Hounsfield units; IPA, invasive pulmonary adenocarcinoma; IQR, interquartile range; MIA, minimally invasive adenocarcinoma.
Relationship between FR+CTC expression/AI tumor volume and tumor invasiveness
For the FR+CTC analysis, the median levels in patients with AIS + MIA and IPA were 9.35 [95% confidence interval (CI): 7.50–13.75] and 12.80 FU/3 mL (95% CI: 9.20–18.00). Also, a significant difference was shown between the FR+CTC levels of the AIS + MIA and IPA groups (P=0.0033, Figure 1A). For the AI tumor volume analysis, the median levels in patients with AIS + MIA and IPA were 274 (95% CI: 81.0–618.5) and 527 mm3 (95% CI: 230.5–2,457.0), showing a significant difference between the two groups (P=0.035, Figure 1B).
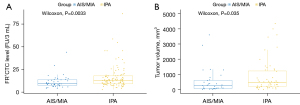
Diagnostic value of FR+CTC or/and AI tumor volume for tumor invasiveness
To assess the value of FR+CTC and AI tumor volume in diagnosing tumor invasiveness, patients with AIS + MIA and IPA were assigned as non-invasion and invasion groups, respectively. The AUCs of FR+CTC and tumor volume in diagnosing tumor invasiveness were 0.659 (95% CI: 0.560–0.759) and 0.698 (95% CI: 0.585–0.810), respectively (Table 2; Figure 2A,2B). A predictive model combining FR+CTC with AI tumor volume showed a sensitivity of 86.89% and specificity of 70.94%, and the AUC was 0.841 (95% CI: 0.754–0.927) for the whole cohort (Table 2, Figure 2C).
Table 2
Characteristic | Cut off | Sensitivity (%) | Specificity (%) | AUC (95% CI) |
---|---|---|---|---|
FR+CTC (FU/3 mL) | 9.75 | 73.27 | 55.00 | 0.659 (0.560–0.759) |
Tumor volume (mm3) | 118 | 93.55 | 38.71 | 0.698 (0.585–0.810) |
Model: FR+CTC + tumor volume | 0.610 | 86.89 | 70.97 | 0.841 (0.754–0.927) |
AUC, area under the curve; FR+CTC, folate-receptor-positive circulating tumor cells; FU, folate receptor units.
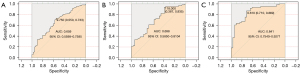
Univariate and multivariate analyses for distinguishing tumor invasiveness
In the univariate analysis, age, sex, and smoking history were not significantly correlated with the IPA group (P>0.05). Nodule type, maximum density, tumor volume, and FR+CTC level showed statistically significant correlation with tumor invasive degree of LUAD (P<0.05). The highest performing characteristic was tumor volume [odds ratio (OR), 9.16; 95% CI: 2.64–31.79; P<0.0001; Table 3]. In addition, the multivariate analysis identified FR+CTC (OR, 5.49; 95% CI: 1.75–17.29; P<0.01), and tumor volume (OR, 9.05; 95% CI: 2.20–37.21; P<0.01) as independent predictors (Table 3).
Table 3
Characteristic | Univariate analysis | Multivariate analysis | |||
---|---|---|---|---|---|
OR (95% CI) | P value | OR (95% CI) | P value | ||
Age (≥60) | 2.04 (0.94–4.42) | 0.07 | – | – | |
Sex (female) | 1.84 (0.87–3.89) | 0.11 | – | – | |
Nodule type | |||||
Solid | – | – | – | – | |
mGGO | 0.58 (0.21,1.62) | 0.3 | 0.47 (0.13–1.69) | 0.25 | |
GGO | 0.22 (0.06–0.72) | 0.01 | 0.41 (0.06–2.89) | 0.37 | |
Maximum density (HU) | 1 (1–1) | <0.0001 | 1.0 (1.00–1.01) | 0.07 | |
Smoking history (non-smoker) | – | – | |||
Smoking history (former smoker) | 2,617,187 (0–Inf) | 0.99 | – | – | |
Smoking history (smoker) | 1.82 (0.63–5.24) | 0.2700 | – | – | |
Tumor volume (≥118 mm3) | 9.16 (2.64–31.79) | <0.0001 | 9.05 (2.20–37.21) | <0.01 | |
FR+CTC level (≥9.75) | 3.35 (1.56–7.18) | <0.0001 | 5.49 (1.75–17.29) | <0.01 |
FR+CTC, folate-receptor-positive circulating tumor cells; GGO, ground-glass opacity; HU, Hounsfield units.
Nomogram development and validation
A predictive model was established with the variables of nodule type, maximum density, FR+CTC and AI tumor volume, and the model is presented as a nomogram (Figure 3) for predicting the invasiveness of LUAD. A calibration curve was used to verify the consistency and predictive accuracy of the nomogram (Figure 4), and it showed that the predicted values estimated by the nomogram were in good agreement with actual observations, with a C-index of 0.840. Also, the Hosmer-Lemeshow test yielded non-significant goodness-of-fit (P=0.09).
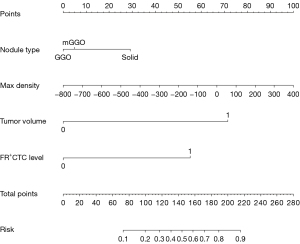
Discussion
Low-dose CT, as the most commonly used tool for lung cancer screening, may lead to a high false-positive rate and high radiation exposure (34). A study reported that lesion borders and lesion margins on CT images routinely used in clinical practice showed poor consistency (35). Currently, the determination of tumor invasiveness depends on biopsy or puncture as the gold standard. Thus, a new model of tumor invasiveness is highly warranted. In this study, we developed a predictive model combining FR+CTC and AI tumor volume.
Firstly, we reported that the FR+CTC levels of IPA group were significantly higher than those in the non-IPA group. In a prospective study, FR+CTC detection in patients with different pathologic types of LUAD, the sensitivity in patients with AIS, MIA, invasive glands, and IA variants were 60%, 73.2%, 73.9%, and 75%, respectively (36). Zhou et al. reported that FR+CTCin patients with AIS + MIA versus IPA, the sensitivity was 77.3–84.1% (16). In our study, the sensitivity and specificity of FR+CTC for differentiating the IPA and non-IPA groups were 73.27% and 55.00%, respectively, which is in line with the previous studies (16,36). Ding et al. constructed two diagnostic models by deep learning based on CT scans to distinguishing the degree of lung nodule invasiveness, and the AUC values of the diagnosis were 0.88 and 0.86, respectively (24). Recently, a study reported that in terms of artificial intelligence parameters, from AAH/AIS to MIA, and IAC, there was a gradual increase in two-dimensional mean diameter, three-dimensional mean diameter, mean CT value, maximum CT value, and volume of GGNs. Two models that distinguished the pathologic subtypes of LUAD have been developed using AI, and the AUCs of the predictive model for identifying AAH/AIS and MIA and of the model for identifying MIA and IAC were 0.779 and 0.918, respectively (22). When the diagnostic value of the AI tumor volume was accessed, the AUC of tumor volume based on AI in diagnosing tumor invasiveness was 0.698, which was slightly lower than previously reported findings (22,24). Based on the FR+CTC level, tumor invasiveness predictive models have been established. Zhou et al. created a predictive model for the diagnosis of tumor invasiveness based on FR+CTC combined with MTD, with an AUC value of 0.830 (0.707–0.953), sensitivity of 63.6% and specificity of 73.7% (16). Our predictive model combining FR+CTC and AI tumor volume showed a sensitivity of 86.89% and a specificity of 70.94%, and the AUC was 0.841, which was significantly greater than the AUC of the single characteristics, similar to the results for previous models (16). Primary tumor volume might be related to CTC level in patients with lung cancer, because tumor volume better reflects cancer burden (17); however, we did not explore the relationship between CTC level and AI tumor volume. FR+CTC AI tumor volume, maximum density, and nodule type were included in our predictive model. The nomogram yielded a C-index of 0.840, suggesting potential clinical application value.
This study has several limitations. First, it was a single-center retrospective study with a small sample size, and the model needs to be further tested in prospective, multicenter, and large sample studies. Second, commonly used clinical serum biomarkers, such as CEA, CA125, and ProGRP, were not included in this model, and only tumor volume from among the AI parameters was included in the predictive model. So, this model has a lot of room for improvement.
Conclusions
We found that FR+CTC and AI tumor volume were independent indicators of the invasiveness of LUAD, and our predictive nomogram, which included nodule type, maximum density, FR+CTC and AI tumor volume, can be used for preoperative screening of NSCLC patients and to assist radiologists and thoracic surgeons make their clinical decisions.
Acknowledgments
Funding: The study was supported by the Science-Technology Foundation for Young Scientists of Gansu Province (Nos. 18JR3RA305, 21JR1RA107); and the Natural Science Foundation of Gansu Province (Nos. 21JR1RA118, 21JR1RA092).
Footnote
Reporting Checklist: The authors have completed the TRIPOD reporting checklist. Available at https://atm.amegroups.com/article/view/10.21037/atm-22-5668/rc
Data Sharing Statement: Available at https://atm.amegroups.com/article/view/10.21037/atm-22-5668/dss
Conflicts of Interest: All authors have completed the ICMJE uniform disclosure form (available at https://atm.amegroups.com/article/view/10.21037/atm-22-5668/coif). The authors have no conflicts of interest to declare.
Ethical Statement: The authors are accountable for all aspects of the work in ensuring that questions related to the accuracy or integrity of any part of the work are appropriately investigated and resolved. The study was conducted in accordance with the Declaration of Helsinki (as revised in 2013). The study was approved by the Ethics Committee of The First Hospital of Lanzhou University (No. LDYYLL2022-49). Individual consent for this retrospective analysis was waived.
Open Access Statement: This is an Open Access article distributed in accordance with the Creative Commons Attribution-NonCommercial-NoDerivs 4.0 International License (CC BY-NC-ND 4.0), which permits the non-commercial replication and distribution of the article with the strict proviso that no changes or edits are made and the original work is properly cited (including links to both the formal publication through the relevant DOI and the license). See: https://creativecommons.org/licenses/by-nc-nd/4.0/.
References
- Basumallik, N. and M. Agarwal, Small Cell Lung Cancer, in StatPearls. Treasure Island (FL), 2022.
- Zappa C, Mousa SA. Non-small cell lung cancer: current treatment and future advances. Transl Lung Cancer Res 2016;5:288-300. [Crossref] [PubMed]
- Travis WD, Brambilla E, Nicholson AG, et al. The 2015 World Health Organization Classification of Lung Tumors: Impact of Genetic, Clinical and Radiologic Advances Since the 2004 Classification. J Thorac Oncol 2015;10:1243-60. [Crossref] [PubMed]
- MacMahon H, Naidich DP, Goo JM, et al. Guidelines for Management of Incidental Pulmonary Nodules Detected on CT Images: From the Fleischner Society 2017. Radiology 2017;284:228-43. [Crossref] [PubMed]
- Hattori A, Hirayama S, Matsunaga T, et al. Distinct Clinicopathologic Characteristics and Prognosis Based on the Presence of Ground Glass Opacity Component in Clinical Stage IA Lung Adenocarcinoma. J Thorac Oncol 2019;14:265-75. [Crossref] [PubMed]
- Pedersen JH, Saghir Z, Wille MM, et al. Ground-Glass Opacity Lung Nodules in the Era of Lung Cancer CT Screening: Radiology, Pathology, and Clinical Management. Oncology (Williston Park) 2016;30:266-74. [PubMed]
- Fan L, Fang M, Li Z, et al. Radiomics signature: a biomarker for the preoperative discrimination of lung invasive adenocarcinoma manifesting as a ground-glass nodule. Eur Radiol 2019;29:889-97. [Crossref] [PubMed]
- Zhang P, Li T, Tao X, et al. HRCT features between lepidic-predominant type and other pathological subtypes in early-stage invasive pulmonary adenocarcinoma appearing as a ground-glass nodule. BMC Cancer 2021;21:1124. [Crossref] [PubMed]
- Ning J, Ge T, Jiang M, et al. Early diagnosis of lung cancer: which is the optimal choice? Aging (Albany NY) 2021;13:6214-27. [Crossref] [PubMed]
- Hattori A, Suzuki K, Matsunaga T, et al. Tumour standardized uptake value on positron emission tomography is a novel predictor of adenocarcinoma in situ for c-Stage IA lung cancer patients with a part-solid nodule on thin-section computed tomography scan. Interact Cardiovasc Thorac Surg 2014;18:329-34. [Crossref] [PubMed]
- He P, Yao G, Guan Y, et al. Diagnosis of lung adenocarcinoma in situ and minimally invasive adenocarcinoma from intraoperative frozen sections: an analysis of 136 cases. J Clin Pathol 2016;69:1076-80. [Crossref] [PubMed]
- Cristofanilli M, Budd GT, Ellis MJ, et al. Circulating tumor cells, disease progression, and survival in metastatic breast cancer. N Engl J Med 2004;351:781-91. [Crossref] [PubMed]
- Gorges TM, Tinhofer I, Drosch M, et al. Circulating tumour cells escape from EpCAM-based detection due to epithelial-to-mesenchymal transition. BMC Cancer 2012;12:178. [Crossref] [PubMed]
- Ledermann JA, Canevari S, Thigpen T. Targeting the folate receptor: diagnostic and therapeutic approaches to personalize cancer treatments. Ann Oncol 2015;26:2034-43. [Crossref] [PubMed]
- Nunez MI, Behrens C, Woods DM, et al. High expression of folate receptor alpha in lung cancer correlates with adenocarcinoma histology and EGFR [corrected] mutation. J Thorac Oncol 2012;7:833-40. [Crossref] [PubMed]
- Zhou Q, Geng Q, Wang L, et al. Value of folate receptor-positive circulating tumour cells in the clinical management of indeterminate lung nodules: A non-invasive biomarker for predicting malignancy and tumour invasiveness. EBioMedicine 2019;41:236-43. [Crossref] [PubMed]
- Kang BJ, Ra SW, Lee K, et al. Circulating Tumor Cell Number Is Associated with Primary Tumor Volume in Patients with Lung Adenocarcinoma. Tuberc Respir Dis (Seoul) 2020;83:61-70. [Crossref] [PubMed]
- Su Z, Mao W, Li B, et al. Clinical Study of Artificial Intelligence-assisted Diagnosis System in Predicting the Invasive Subtypes of Early-stage Lung Adenocarcinoma Appearing as Pulmonary Nodules. Zhongguo Fei Ai Za Zhi 2022;25:245-52. [PubMed]
- Dehkharghanian T, Rahnamayan S, Riasatian A, et al. Selection, Visualization, and Interpretation of Deep Features in Lung Adenocarcinoma and Squamous Cell Carcinoma. Am J Pathol 2021;191:2172-83. [Crossref] [PubMed]
- Aydın N, Çelik Ö, Aslan AF, et al. Detection of Lung Cancer on Computed Tomography Using Artificial Intelligence Applications Developed by Deep Learning Methods and the Contribution of Deep Learning to the Classification of Lung Carcinoma. Curr Med Imaging 2021;17:1137-41. [Crossref] [PubMed]
- Tao XM, Fang R, Wu CC, et al. Prediction of Pathological Subtypes of Lung Adenocarcinoma with Pure Ground Glass Nodules by Deep Learning Model. Zhongguo Yi Xue Ke Xue Yuan Xue Bao 2020;42:477-84. [PubMed]
- Fang W, Zhang G, Yu Y, et al. Identification of pathological subtypes of early lung adenocarcinoma based on artificial intelligence parameters and CT signs. Biosci Rep 2022;42:BSR20212416. [Crossref] [PubMed]
- Wang C, Shao J, Lv J, et al. Deep learning for predicting subtype classification and survival of lung adenocarcinoma on computed tomography. Transl Oncol 2021;14:101141. [Crossref] [PubMed]
- Ding H, Xia W, Zhang L, et al. CT-Based Deep Learning Model for Invasiveness Classification and Micropapillary Pattern Prediction Within Lung Adenocarcinoma. Front Oncol 2020;10:1186. [Crossref] [PubMed]
- Xia X, Gong J, Hao W, et al. Comparison and Fusion of Deep Learning and Radiomics Features of Ground-Glass Nodules to Predict the Invasiveness Risk of Stage-I Lung Adenocarcinomas in CT Scan. Front Oncol 2020;10:418. [Crossref] [PubMed]
- Zhao W, Yang J, Sun Y, et al. 3D Deep Learning from CT Scans Predicts Tumor Invasiveness of Subcentimeter Pulmonary Adenocarcinomas. Cancer Res 2018;78:6881-9. [Crossref] [PubMed]
- Fu L, Zhu Y, Jing W, et al. Incorporation of circulating tumor cells and whole-body metabolic tumor volume of (18)F-FDG PET/CT improves prediction of outcome in IIIB stage small-cell lung cancer. Chin J Cancer Res 2018;30:596-604. [Crossref] [PubMed]
- Travis WD, Brambilla E, Noguchi M, et al. International association for the study of lung cancer/american thoracic society/european respiratory society international multidisciplinary classification of lung adenocarcinoma. J Thorac Oncol 2011;6:244-85. [Crossref] [PubMed]
- Board, W.C.o.T.E. WHO classification of tumours. Thoracic Tumours (M). 5th ed. Lyon: IARC Press, 2021.
- Wang L, Wu C, Qiao L, et al. Clinical Significance of Folate Receptor-positive Circulating Tumor Cells Detected by Ligand-targeted Polymerase Chain Reaction in Lung Cancer. J Cancer 2017;8:104-10. [Crossref] [PubMed]
- He W, Kularatne SA, Kalli KR, et al. Quantitation of circulating tumor cells in blood samples from ovarian and prostate cancer patients using tumor-specific fluorescent ligands. Int J Cancer 2008;123:1968-73. [Crossref] [PubMed]
- Gebremariam A, Assefa M, Addissie A, et al. Delayed initiation of adjuvant chemotherapy among women with breast cancer in Addis Ababa, Ethiopia. Breast Cancer Res Treat 2021;187:877-82. [Crossref] [PubMed]
- Deng J, Zhao M, Li Q, et al. Implementation of artificial intelligence in the histological assessment of pulmonary subsolid nodules. Transl Lung Cancer Res 2021;10:4574-86. [Crossref] [PubMed]
- Ashraf SF, Yin K, Meng CX, et al. Predicting benign, preinvasive, and invasive lung nodules on computed tomography scans using machine learning. J Thorac Cardiovasc Surg 2022;163:1496-505.e10. [Crossref] [PubMed]
- Lee SM, Park CM, Goo JM, et al. Invasive pulmonary adenocarcinomas versus preinvasive lesions appearing as ground-glass nodules: differentiation by using CT features. Radiology 2013;268:265-73. [Crossref] [PubMed]
- Ding C, Zhou X, Xu C, et al. Circulating tumor cell levels and carcinoembryonic antigen: An improved diagnostic method for lung adenocarcinoma. Thorac Cancer 2018;9:1413-20. [Crossref] [PubMed]