Establishment and validation of a nomogram for predicting immune-related prognostic features in trunk melanoma-specific death
Highlight box
Key findings
• We developed and validated a nomogram for the specific death prognosis of trunk melanoma.
What is known and what is new?
• Trunk melanoma is a common skin cancer with a poor prognosis. The traditional AJCC staging system is used to predict the survival of melanoma of trunk, but is not specific for melanoma of trunk; thus, a more feasible survival predictive model is needed.
• Improved prognostic accuracy and discriminative ability in patients with melanoma of trunk when compared with the AJCC system. This nomogram can be used as a potential tool for the prognostic risk management of trunk melanoma.
What is the implication, and what should change now?
• The nomogram under the competitive risk model has a better performance in predicting CSD.
Introduction
Cancer is currently the first or second most common cause of premature death in most countries worldwide. Due to the aging of the population, the strong impact of population growth changes on the incidence of cancer and its high cost of treatment are a major challenge facing today’s society (1,2). Melanoma, one of the most advanced malignancies with the worst prognosis, is growing at a rate of 3–5% per year, the fastest rate of any malignancy (3). Melanoma has a very high mortality rate and remains by far the most refractory malignancy in the world. Nodular melanoma is most commonly found in the trunk, head, or neck, accounting for 10–15% of all melanomas (4-6). Currently, the 5-year survival rate for trunk melanoma is as high as around 90% in high-income countries, but less than half that in low- and middle-income countries (7,8). Although the incidence of melanoma is relatively low among all cancers, it is displaying an increasing trend of incidence among the young and middle-aged group, which will cause a great burden to the families of patients (9,10).
Currently, the American Joint Committee on Cancer (AJCC) staging system is the main method to evaluate the prognosis of patients with melanoma. However, there are significant differences in the subsequent treatment effects of patients in the same stage, suggesting that the AJCC staging system needs to be improved in predicting prognosis and treatment (11). This method is only suitable for evaluating primary tumor (T), tumor thickness, ulcer status, regional lymph nodes (N), number of metastatic lymph nodes (LNs), satellite lesion, intermediate metastasis status, distant metastasis status (M), tumor location, and lactate dehydrogenase (LDH) level. However, the linearity decreases compared with the previous staging system, and the groups tend to overlap, which does not mean that the prognosis is poor; there is still substantial room for improvement (12,13).
Melanoma has long been considered a malignant tumor with few treatment options. Currently, the main treatment methods remain surgical resection and targeted therapy (14-17). Increased biological understanding and unprecedented innovations in therapies targeting mutated driver genes and immune checkpoints have substantially improved the prognosis of patients. However, the low response rate and inevitable occurrence of resistance to currently available targeted therapies have stymied further advancements in melanoma management. Therefore, it is necessary to understand the mechanisms underlying melanoma pathogenesis more comprehensively, which might lead to more substantial progress in therapeutic approaches and expand clinical options for melanoma therapy.
Nomograms are widely used as prognostic devices in oncology and medicine to generate individual probabilities of clinical events by integrating various prognostic and determining variables (18). Previous studies have developed and validated methods for predicting recurrence and melanoma-specific death in patients with negative sentinel LNs, as well as novel nomograms and risk classification systems for predicting cancer-specific death (CSD) in patients initially diagnosed with metastatic cutaneous melanoma. Factors incorporated into such nomograms have included sex, age, date of diagnosis, date of sentinel lymph node biopsy (SLNB), characteristics of the primary tumor (Breslow thickness, ulceration), recurrence and follow-up details, race, marital status, insurance, AJCC stage T and N, number of metastatic organs, surgical treatment, and chemotherapy (19,20). Recently, many studies have also documented the prognosis of other tumors, such as parotid carcinoma, maxillary sinus carcinoma, and breast cancer in the elderly (21-23). Although some studies have mentioned melanoma, no specific studies have been conducted on trunk melanoma due to its small sample size, single source of sample size, or lack of CSD risk factor assessment. So it is necessary to carry out more studies on trunk melanoma (24-26). In addition, most existing models focus on the outcome of all-cause death. Therefore, the accuracy of these risk models in predicting competitive events, which make up a relatively large portion and are a random factor in the model of all-cause death, may be significantly compromised. Furthermore, a small number of models use traditional survival analysis to explore specific deaths, which requires removing competitive events. This can also cause serious bias when the proportion of competitive events is large.
The Surveillance, Epidemiology, and End Results (SEER) database is a publicly available, population-based resource, because local registries report information for all cancer cases within a specific region and/or defined racial/ethnic population (27). The datasets retrieved from the SEER database are of sufficient size to create a prediction model. The data of the cases used in this study are reliable and the sample size is sufficient. Through data mining technology, we can reduce the characteristics of dimensional heterogeneity, timeliness, scarcity, irregularity, and so on to a certain extent, to better assess the risk of patients and assist clinical decision-making to establish disease prediction models (28).
Within this study, we utilized a representative cohort of patients with trunk melanoma from the SEER database registry to identify independent prognostic factors and to develop and validate a predictive model for trunk melanoma in the form of a nomogram to help clinicians in the prevention and treatment of trunk melanoma, to make specific decisions, and to accurately predict disease outcomes. We present the following article in accordance with the TRIPOD reporting checklist (available at https://atm.amegroups.com/article/view/10.21037/atm-22-6045/rc).
Methods
Data source
The SEER database is representative of the US population, with patient-level data abstracted from 18 geographically diverse populations that represent rural, urban, and regional populations. Trunk melanoma cases between 2010 and 2015 in the SEER public access database and their corresponding details were retrieved with the use of SEER*Stat software. A total of 25,542 samples were included, so there was no need to introduce external validation.
Study population
We retrospectively analyzed data from the SEER data repository from 2010 to 2015. The SEER data warehouse (https://seer.cancer.gov/) is publicly accessible. We followed the SEER 18 rules for custom data selection (with an additional search completed and uploaded in November 2019 [1992–2017]. All patients with a primary diagnosis of trunk melanoma were included in the analysis. The exclusion criteria were as follows: nonconformance to the primary site and indeterminate factors [race, marriage, positive regional LNs, and survival months, derived AJCC stage group, RX-Ubiquitin like small molecule modifier (RX SUMO)-surgery other Registry/Digital Information System (Reg/Dis), THOR database Cross-reference data (DX) bone bound SEER met, DX brain bound SEER met, DX liver bound SEER met, DX lung bound SEER met]; some sites were not operated on [RX Summary-Surg Prim Site (1998+), RX Summary-Scope Reg LN Sur (2003+)]; and LNs were not submitted for examination. The study was conducted in accordance with the Declaration of Helsinki (as revised in 2013).
Variable selection
We calculated the ratio of deaths from other causes to the total number of deaths. When the ratio of deaths from other causes was high (e.g., >20%), a competing risk model was constructed. Factors including age, race, sex, marriage, laterality, behavior, stage, T stage, N stage, surgery, LN surgery, surgery other, radiation, chemotherapy, Breslow, pretreatment, LDH, mitotic, ulceration, LN positive, bone, brain, liver, and lung involvement, malignant, time, and status were the independent predictive factors. We randomly divided the cohort into a training cohort and validation cohort according to the ratio of 7:3. In the training cohort, univariate and multivariate competing risk models were used to screen for variables with P>0.1 among univariate variables. The variable of 0.1 was included in the multivariate competing risk model, and P>0,1 was selected as the multivariate factor. A variable of 0.05 constructed a competing risk model for the predicted years plot. Non-metrology and different receiver operating characteristic (ROC) curves were used to evaluate the prediction accuracy of each variable, and calibration curves were used to evaluate the calibration of the predicted years plot.
Statistical analyses
The data after cleaning is randomly sampled, and the data is split according to the ratio of 7:3. The data is randomly divided into the training queue and the verification queue, and the basic information of the training set and the verification set is statistically analyzed. The training set data is used for internal model validation, and the validation set data is used for external model validation. Data were statistically analyzed according to normal distribution. Measurements (age and tumor size) were expressed as means and standard deviations, using t-tests for independent samples, n-test and frequency (%) for categorical variables, chi-square tests for comparison between groups, and the chi-square test or non-parametric U-test was applied for difference analysis. Log-rank test and Kaplan-Meier curve were used to analyze the differences in survival between groups. All statistical methods were analyzed by R software 4.2.0 (The R Foundation for Statistical Computing, Vienna, Austria) and SPSS 13.0 (IBM Corp., Chicago, IL, USA). A P value <0.05 was considered statistically significant.
Results
Patient characteristics
As shown in Figure 1, we initially retrieved 12,7188 patient cases from the SEER database, and after screening, a total of 21,198 patients with trunk melanoma were eligible, and 3814 of them died (17.99%). Among the death cases, deaths from other causes accounted for 66.50%. Therefore, the impact of deaths from other causes cannot be eliminated when considering specific mortality by Cox regression analysis or all-cause death. The median age of the cases at diagnosis was 59.93±15.60 years, 68.16% were male, most patients were White (n=21,004, 99. 08%, P<0.05), and 69.72% were married. Among 21,198 cases of trunk melanoma, laterality was found in 8,726 cases (41.16%) on the left side. Among them, stage I was the longest concurrent tumor stage (n=16,205, 76.45%), followed by stage II (n=2,753, 12.99%) and stage III (n=1,983, 9.35%, P<0.05), and stage IV (n=257, 1.21%). In addition, most of the torso melanoma patients were classified as T1 (66.48%), followed by T2 (16.77%), T3 (9.27%), and T4 (7.48%). Almost 95.84% of patients did not have LN metastasis (N0), and 85.16% of patients did not have ulcers. Nearly half of the patients received surgery (n=11,380, 53.68%), and a minority of patients received chemotherapy (n=321, 1.51%). Detailed population statistics and clinical characteristics are provided in Table 1.
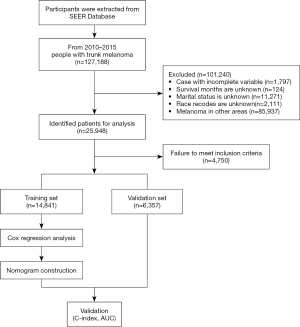
Table 1
Factors | Define | Train (n=14,841) | Test (n=6,357) | All (n=21,198) |
---|---|---|---|---|
Age (years) | 59.89±15.64 | 60.02±15.49 | 59.93±15.60 | |
Marriage | Married | 10,296 (69.38) | 4,484 (70.54) | 14,780 (69.72) |
Divorced | 2,503 (16.87) | 1,005 (15.81) | 3,508 (16.55) | |
Single | 890 (6.00) | 388 (6.10) | 1,278 (6.03) | |
Other | 1,152 (7.76) | 480 (7.55) | 1,632 (7.70) | |
Race | White | 14,700 (99.05) | 6,304 (99.17) | 21,004 (99.08) |
Other | 141 (0.95) | 53 (0.83) | 194 (0.92) | |
Sex | Male | 10,141 (68.33) | 4,308 (67.77) | 14,449 (68.16) |
Female | 4,700 (31.67) | 2,049 (32.23) | 6,749 (31.84) | |
Laterality | Left | 6,034 (40.66) | 2,692 (42.35) | 8,726 (41.16) |
Right | 5,807 (39.13) | 2,374 (37.34) | 8,181 (38.59) | |
Bilateral | 3,000 (20.21) | 1,291 (20.31) | 4,291 (20.24) | |
Behavior | Behav1 | 6,735 (45.38) | 2,840 (44.68) | 9,575 (45.17) |
Behav2 | 5,836 (39.32) | 2,542 (39.99) | 8,378 (39.52) | |
Behav3 | 2,270 (15.30) | 975 (15.34) | 3,245 (15.31) | |
Stage | I | 11,353 (76.50) | 4,852 (76.33) | 16,205 (76.45) |
II | 1,906 (12.84) | 847 (13.32) | 2,753 (12.99) | |
III | 1,386 (9.34) | 597 (9.39) | 1,983 (9.35) | |
IV | 196 (1.32) | 61 (0.96) | 257 (1.21) | |
T | T1 | 9,859 (66.43) | 4,233 (66.59) | 14,092 (66.48) |
T2 | 2,511 (16.92) | 1,044 (16.42) | 3,555 (16.77) | |
T3 | 1,368 (9.22) | 598 (9.41) | 1,966 (9.27) | |
T4 | 1,103 (7.43) | 482 (7.58) | 1,585 (7.48) | |
N | N0 | 14,213 (95.77) | 6,103 (96.00) | 20,316 (95.84) |
N1 | 408 (2.75) | 187 (2.94) | 595 (2.81) | |
N2 | 220 (1.48) | 67 (1.05) | 287 (1.35) | |
Surgery | Surg1 | 7,935 (53.47) | 3,445 (54.19) | 11,380 (53.68) |
Surg2 | 5,621 (37.87) | 2,420 (38.07) | 8,041 (37.93) | |
Surg3 | 217 (1.46) | 88 (1.38) | 305 (1.44) | |
Surg4 | 1,068 (7.20) | 404 (6.36) | 1,472 (6.94) | |
LNSur | None | 6,276 (42.29) | 2,718 (42.76) | 8,994 (42.43) |
1–3 | 5,825 (39.25) | 2,509 (39.47) | 8,334 (39.32) | |
4+ | 339 (2.28) | 130 (2.04) | 469 (2.21) | |
Others | 2,401 (16.18) | 1,000 (15.73) | 3,401 (16.04) | |
SurgOth | None | 9,158 (61.71) | 3,901 (61.37) | 13,059 (61.60) |
Yes | 5,683 (38.29) | 2,456 (38.63) | 8,139 (38.40) | |
Radiation | No | 14,677 (98.89) | 6,267 (98.58) | 20,944 (98.80) |
Yes | 164 (1.11) | 90 (1.42) | 254 (1.20) | |
Chemotherapy | No | 14,605 (98.41) | 6,272 (98.66) | 20,877 (98.49) |
Yes | 236 (1.59) | 85 (1.34) | 321 (1.51) | |
Breslow | 0.7 [0.4, 1.4] | 0.7 [0.4, 1.3] | 0.7 [0.4, 1.4] | |
Pretreatment LDH | Other | 13,288 (89.54) | 5,696 (89.60) | 18,984 (89.56) |
Normal level | 1,112 (7.49) | 444 (6.98) | 1,556 (7.34) | |
Above | 441 (2.97) | 217 (3.41) | 658 (3.10) | |
Ulceration | No | 12,626 (85.08) | 5,427 (85.37) | 18,053 (85.16) |
Yes | 2,215 (14.92) | 930 (14.63) | 3,145 (14.84) | |
Mitotic | 0 [0, 2] | 0 [0, 2] | 0 [0, 2] | |
Positive | No | 2,404 (16.20) | 1,018 (16.01) | 3,422 (16.14) |
Yes | 6,220 (41.91) | 2,653 (41.73) | 8,873 (41.86) | |
Other | 6,217 (41.89) | 2,686 (42.25) | 8,903 (42.00) | |
Bone | No | 14,790 (99.66) | 6,343 (99.78) | 21,133 (99.69) |
Yes | 51 (0.34) | 14 (0.22) | 65 (0.31) | |
Brain | No | 14,791 (99.66) | 6,340 (99.73) | 21,131 (99.68) |
Yes | 50 (0.34) | 17 (0.27) | 67 (0.32) | |
Liver | No | 14,788 (99.64) | 6,344 (99.80) | 21,132 (99.69) |
Yes | 53 (0.36) | 13 (0.20) | 66 (0.31) | |
Lung | No | 14,758 (99.44) | 6,333 (99.62) | 21,091 (99.50) |
Yes | 83 (0.56) | 24 (0.38) | 107 (0.50) | |
Malignant | 1 | 9,378 (63.19) | 4,004 (62.99) | 13,382 (63.13) |
>1 | 5,463 (36.81) | 2,353 (37.01) | 7,816 (36.87) | |
Status | 0 | 12,167 (81.98) | 5,217 (82.07) | 17,384 (82.01) |
1 | 1,204 (8.11) | 505 (7.94) | 1,709 (8.06) | |
2 | 1,470 (9.90) | 635 (9.99) | 2,105 (9.93) | |
Time (years) | 59.34±24.96 | 59.19±24.75 | 59.30±24.90 |
Data are present as n (%), median [range], or mean ± SD. LNSur, LN surgery; LN, lymph node; SurgOth, surgery other; LDH, lactate dehydrogenase; SD, standard deviation.
Developing and validating the nomogram
A competing risk model was constructed and factors of significance in univariate analysis (P<0.05) were included in the multivariate competing risk model analysis. The results showed that the risk factors of trunk melanoma included age, marriage, race, sex, behavior, stage, T stage, N stage, surgery, LN surgery, surgery other, pretreatment LDH, radiation, mitotic, ulceration, LN positive, bone, brain, liver, Breslow, and lung involvement. Independent predictors, specific deaths at different levels, and deaths from other causes are described in Figure S1.
All variables were further identified by multivariate assessment of the Fine-Gray ratio example distribution risk model. After optimizing the nomogram, we finally included variables in the estimation model and summarized their effect sizes (Table 2). We used these variables to create a competing event nomogram to assess 3- and 5-year CSD probabilities (see Figure S2 for more details). The likelihood of death at different time points for each patient was calculated through this model by adding a score for each integration variable.
Table 2
Characteristics | Define | Univariate analysis | Multivariate analysis | |||
---|---|---|---|---|---|---|
HR (95% CI) | Z (P) | HR (95% CI) | Z (P) | |||
Age | 1.018 (1.014–1.022) | 9.222 (<0.001) | 1.017 (1.012–1.022) | 7.173 (<0.001) | ||
Marriage | Married | Ref | NA | Ref | NA | |
Divorced | 1.601 (1.387–1.848) | 6.436 (<0.001) | 1.241 (1.047–1.47) | 2.495 (0.013) | ||
Single | 2.416 (2.008–2.907) | 9.352 (<0.001) | 1.252 (0.999–1.57) | 1.949 (0.051) | ||
Other | 1.804 (1.497–2.173) | 6.208 (<0.001) | 1.249 (1.021–1.527) | 2.162 (0.031) | ||
Race | White | Ref | NA | Ref | NA | |
Other | 3.655 (2.595–5.149) | 7.416 (<0.001) | 1.748 (1.165–2.622) | 2.697 (0.007) | ||
Sex | Female | Ref | NA | Ref | NA | |
Male | 1.388 (1.219–1.581) | 4.936 (<0.001) | 1.139 (0.982–1.322) | 1.718 (0.086) | ||
Behavior | Behav1 | Ref | NA | Ref | NA | |
Behav2 | 0.665 (0.575–0.769) | −5.499 (<0.001) | 0.968 (0.829–1.132) | −0.405 (0.69) | ||
Behav3 | 0.359 (2.446–3.172) | 15.436 (<0.001) | 1.111 (0.952–1.298) | 1.333 (0.18) | ||
Stage | I | Ref | NA | Ref | NA | |
II | 8.244 (7.021–9.679) | 25.747 (<0.001) | 2.538 (1.881–3.425) | 6.09 (<0.001) | ||
III | 17.756 (15.276–20.639) | 37.473 (<0.001) | 5.821 (4.295–7.888) | 11.358 (<0.001) | ||
IV | 71.670 (55.927–91.843) | 33.760 (<0.001) | 6.949 (4.19–11.524) | 7.512 (<0.001) | ||
T | T1 | Ref | NA | Ref | NA | |
T2 | 4.428 (3.690–5.314) | 15.994 (<0.001) | 1.495 (1.125–1.986) | 2.774 (0.006) | ||
T3 | 11.217 (9.445–13.322) | 27.554 (<0.001) | 1.488 (1.056–2.095) | 2.274 (0.023) | ||
T4 | 23.176 (19.676–27.298) | 37.632 (<0.001) | 1.868 (1.19–2.931) | 2.717 (0.007) | ||
N | N0 | Ref | NA | Ref | NA | |
N1 | 8.186 (6.950–9.642) | 25.179 (<0.001) | 1.386 (1.119–1.718) | 2.990 (0.003) | ||
N2 | 14.959 (12.197–18.345) | 25.981 (<0.001) | 1.533 (1.149–2.044) | 2.906 (0.004) | ||
Laterality | Left | Ref | NA | Ref | NA | |
Right | 1.001 (0.882–1.137) | 0.022 (0.022) | Ref | NA | ||
Bilateral | 1.027 (0.882–1.196) | 0.340 (0.340) | Ref | NA | ||
Surgery | Surg1 | Ref | NA | Ref | NA | |
Surg2 | 1.937 (1.712–2.191) | 10.514 (<0.001) | 1.199 (0.566–2.538) | 0.473 (0.640) | ||
Surg3 | 3.035 (2.091–4.405) | 5.842 (<0.001) | 0.731 (0.35–1.527) | −0.834 (0.400) | ||
Surg4 | 2.482 (2.044–3.013) | 9.185 (<0.001) | 1.497 (1.174–1.908) | 3.259 (0.001) | ||
LNSur | No | Ref | NA | Ref | NA | |
LNSur1–3 | 2.611 (2.256–3.021) | 12.877 (<0.001) | 0.866 (0.383–1.962) | −0.344 (0.730) | ||
LNSur4+ | 6.976 (5.474–8.888) | 15.710 (<0.001) | 0.966 (0.496–1.882) | −0.102 (0.920) | ||
LNSur others | 2.502 (2.098–2.983) | 10.220 (<0.001) | 1.009 (0.55–1.85) | 0.029 (0.980) | ||
SurgOth | No | Ref | NA | Ref | NA | |
Yes | 1.709 (1.525–1.913) | 9.290 (<0.001) | 1.126 (0.652–1.947) | 0.427 (0.670) | ||
Pretreatment LDH | Low | Ref | NA | Ref | NA | |
Normal level | 1.621 (1.354–1.940) | 5.270 (<0.001) | 0.903 (0.733–1.111) | −0.966 (0.330) | ||
Above | 2.657 (2.113–3.340) | 8.362 (<0.001) | 1.133 (0.874–1.468) | 0.945 (0.340) | ||
Radiation | No | Ref | NA | Ref | NA | |
Yes | 16.869 (13.486–21.099) | 25.931 (<0.001) | 1.876 (1.383–2.546) | 4.043 (<0.001) | ||
Chemotherapy | No | Ref | NA | Ref | NA | |
Yes | 9.194 (7.507–11.260) | 21.451 (<0.001) | 1.56 (1.186–2.051) | 3.181 (0.002) | ||
Mitotic | 1.275 (1.261–1.290) | 41.035 (<0.001) | 1.054 (1.033–1.076) | 5.182 (<0.001) | ||
Ulceration | 7.589 (6.778–8.498) | 35.116 (<0.001) | 1.408 (1.211–1.638) | 4.436 (<0.001) | ||
LN positive | No | Ref | NA | Ref | NA | |
Yes | 2.025 (1.710–2.398) | 8.180 (<0.001) | 0.779 (0.583–1.042) | −1.682 (0.093) | ||
Other | 0.574 (0.470–0.702) | −5.408 (<0.001) | 0.89 (0.478–1.658) | −0.368 (0.710) | ||
Bone | No | Ref | NA | Ref | NA | |
Yes | 38.806 (24.615–61.179) | 15.752 (<0.001) | 1.811 (0.935–3.505) | 1.762 (0.078) | ||
Brain | No | Ref | NA | Ref | NA | |
Yes | 39.498 (25.846–60.359) | 41.035 (<0.001) | 4.466 (2.456–8.12) | 4.905 (<0.001) | ||
Liver | No | Ref | NA | Ref | NA | |
Yes | 29.086 (18.138–46.644) | 13.987 (<0.001) | 0.995 (0.501–1.974) | −0.015 (0.99) | ||
Breslow | 1.419 (1.398–1.441) | 44.799 (<0.001) | 1.046 (0.993–1.103) | 1.687 (0.092) | ||
Lung | No | Ref | NA | Ref | NA | |
Yes | 24.708 (17.581–34.724) | 18.472 (<0.001) | 1.176 (0.694–1.995) | 0.603 (0.55) | ||
Malignant | 1 | Ref | NA | Ref | NA | |
2+ | 1.038 (0.924–1.166) | 0.632 (0.530) | – | – |
HR, hazard ratio; CI, confidence interval; NA, not available; LNSur, LN surgery; LN, lymph node; SurgOth, surgery other; LDH, lactate dehydrogenase.
The C-index obtained through internal verification of the training set was 0.899 [standard error (SE): 0.001], and the areas under the nomogram curve were 0.928 [95% confidence interval (CI): 0.99–0.945], 0.907 (95% CI: 0.895–0.918) and 0.891 (95% CI: 0.879–0.902). The C-index obtained through external verification of the validation set was 0.890 (SE: 0.002), and the areas under the nomogram ROC curve were 0.927 (95% CI: 0.899–0.955), 0.916 (95% CI: 0.901–0.930) and 0.905 (95% CI: 0.899–0.921), respectively. The calibration curve was highly consistent with the nomogram ideal curve, the calibration degree was ideal, and the discrimination degree of the area under the ROC curve (AUC) was high (Figures 2,3).
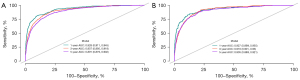
Discussion
Despite the low incidence of trunk melanoma, its growth rate is faster, and the fatality rate is higher in patients with late stage. Although studies with substantial sample sizes of melanoma patients have been conducted, and nomograms have been constructed, their clinical application has been limited by lack of information and external data validation. This study on trunk melanoma involved competitive risk analysis and a nomogram was developed and verified for its specificity in predicting CSD (29).
Within this study, for the first time, we used SEER data to create a prognostic line chart of patients with trunk melanoma, and to include more accurate indicators. The larger sample size in the SEER database reduced the error in this study to some extent. The established nomogram can ensure that the selected variables can be directly related to the prognosis of melanoma, which has good clinical application value.
Whereas previous studies of melanoma based on the SEER database have focused on incidence and survival outcomes (30-32), we focused in this study on creating a predictive nomogram with strong clinical application. Both the training set and the validation set showed the ideal calibration degree. The model we constructed can be applied to all trunk melanoma patients, is suitable for clinical application in medical institutions at all levels, and can, to a certain extent, make up for the inadequacy of AJCC staging method and provide an accurate prognostic assessment tool. In addition, our nomogram can facilitate clinician and patient interactions. In addition, local datasets can be used for external verification of the modified nomogram according to the situation of different medical institutions, to optimize adaption to the local situation.
Other studies have evaluated the predictive nomogram for other types of melanoma. Xu et al. included a 300-column study in their research on head and neck melanoma and obtained a C-index value >0.7. Xiao et al. obtained a C-index is 0.817 for the prognosis of non-metastatic abdominal melanoma. However, Zeng et al. obtained a C-index of 0.778 in the study of uveal melanoma (25,33,34).
In order to include a larger amount of samples, we choose the SEER database for its numerous data on tumor, which can provide a wide platform for further research. Additional, prediction models have strict requirements for the information on samples, and the SEER database has relatively complete data to better reflect the authenticity of nomogram and its clinical application value. We obtained 26 indicators, including 24 specific factors, and finally used 8 indicators to construct the nomogram with a C-index of 0.89. In general, a C-index >0.7 is considered to have very good prediction accuracy, so our model can be used as a very desirable prospective prediction tool for melanoma.
The C-index of some published models for predicting melanoma ranges from 0.70 to 0.82, which is not sufficient for clinical application. The low C-index is mainly because the competing event is a random event, which will reduce the accuracy of all-cause death. In the opinions of Zaorsky et al. and Yang et al., Due to the large deviation of independent influencing factors, Cox regression would seriously overestimate the accuracy, which was confirmed in our study. The impact of deaths from other causes cannot be eliminated when considering specific mortality by Cox regression analysis or all-cause death. So, we recommend the use of competing risk models in this study, especially in large sample sizes (35,36).
Melanoma is one of the most immunogenic cancers and, as such, is most likely to respond favorably to immunotherapy. However, like many cancers, melanomas acquire a variety of inhibitory mechanisms that often work in concert to evade detection and destruction by innate and adaptive immunity. Intensive investigation of the cellular and molecular events associated with melanoma formation, ultimately leading to immune suppression, has led to the discovery of new therapeutic targets and synergistic combinations of immunotherapy, targeted therapy, and chemotherapy (37). Melanoma is a complex disease with multiple factors involved in body activities and resulting in chaos in the functional balance of each system. Melanoma reprograms its metabolic pathways to enable it to survive, grow, and proliferate. Using metabolomics to study the metabolism of different melanomas has become an urgent task (38).
There are 2 main immunotherapy options for melanoma, 3 if oncolytic viruses (T-VEC; Imlygic) are included, although they are rarely used clinically. The 2 most important classes of drugs are anti-programmed cell death 1 (PD-1) drugs (nivolumab or pembrolizumab) and anti-cytotoxic T lymphocyte-associated antigen-4 (anti-CTLA-4) drugs (ipilimumab) (39,40). Evidence has shown that the long-term survival rate with anti-PD-1 drugs alone is 40–45%. The combination of anti-CLTA-4 agents has been shown to increase the 5-year survival rate by an additional 7–10% (41,42). Therefore, for patients with metastatic trunk melanoma, we recommend the use of anti-PD-1 drugs alone or combined with anti-CTLA-4 drugs in clinical treatment, either from the perspective of efficacy or safety.
Immunotherapy for melanoma starts with the T-cell CTLA-4 molecule. CTLA-4 is an inhibitory immune checkpoint expressed on the surface of T cells and used to downregulate immune responses. Ipilimumab (Yervoy) activates the immune system by targeting CTLA-4, thereby enhancing the activation and proliferation of T cells and stimulating antitumor immune responses. Nivolumab (Opdivo) is a PD-1 antibody that binds to the PD-1 receptor on T cells, thereby preventing PD-1 from binding to PD-L1 and activating the body’s immune system to attack tumors (43). Although the above two drugs show better therapeutic properties, for melanoma, it is important that patients receive immunotherapy on the first line. As first-line agents alone, the PD-1 inhibitors nivolumab and pembrolizumab have been shown to improve 5-year survival. The combination of nivolumab and ipilimumab, a CTLA-4 inhibitor, may also improve remission in melanoma patients and may be the treatment of choice in this field (44). The advent of immunotherapy has revolutionized the treatment of patients with melanoma, regardless of biomarker status. Therefore, we suggest that future research on melanoma treatment should consider the direction of immune metabolism, and carry out clinical validation of single and combination therapies.
Studies have shown that 5-year survival rates in melanoma patients eligible for clinical trials can approach 50% with immunotherapy, which is certainly a way to greatly improve the mortality rate specific to trunk melanoma (45-47). Not all patients will respond to the drug, with its effect depending on whether the patient’s T cells can respond. Even for some patients with T-cell immune response, their T cells may not function in the tumor microenvironment for some reason. Therefore, whether or not patients use drugs and what kind of drugs they use are included in the basic information of SEER database, which will bring a more convincing platform to the subsequent related research.
Our study was the first to build a competitive risk model to predict the prognosis of trunk melanoma-specific death. Our research still has some limitations: as a result of our data sources in the SEER database, the small amount of basic information meant that among the large population obtained, we only had a handful of the information that could be used for modeling and part of the cancer characteristics lacked some clinical parameters, some cases were lacking certain background information (48). At the same time, we conducted internal verification and did not introduce external data for verification; we recommend the introduction of external data as verification in the competing risk model analysis in the future.
Conclusions
The competing risks nomogram presented here can be used to assess CSD in patients with trunk melanoma and can be used to develop antitumor immune-related prognostic rehabilitation programs and follow-up strategies that are favorable for survival. And our predictive model is appropriate for all individuals with trunk melanoma and could be broadly utilized at all levels of medical centers. The comprehensiveness of this nomogram could compensate the inefficiencies of the AJCC staging tool, and allow a precise assessment of the prognosis of individuals with trunk melanoma. However, our risk evaluation model needs to be further verified by external data, and we will continue to pay attention to it in follow-up research.
Acknowledgments
We wish to thank the prompt help given by Miss. Zilan Li, McGill University in the revision of the article and for advising on the immunization section of the discussion.
Funding: None.
Footnote
Reporting Checklist: The authors have completed the TRIPOD reporting checklist. Available at https://atm.amegroups.com/article/view/10.21037/atm-22-6045/rc
Conflicts of Interest: All authors have completed the ICMJE uniform disclosure form (available at https://atm.amegroups.com/article/view/10.21037/atm-22-6045/coif). The authors have no conflicts of interest to declare.
Ethical Statement: The authors are accountable for all aspects of the work in ensuring that questions related to the accuracy or integrity of any part of the work are appropriately investigated and resolved. The study was conducted in accordance with the Declaration of Helsinki (as revised in 2013).
Open Access Statement: This is an Open Access article distributed in accordance with the Creative Commons Attribution-NonCommercial-NoDerivs 4.0 International License (CC BY-NC-ND 4.0), which permits the non-commercial replication and distribution of the article with the strict proviso that no changes or edits are made and the original work is properly cited (including links to both the formal publication through the relevant DOI and the license). See: https://creativecommons.org/licenses/by-nc-nd/4.0/.
References
- Soerjomataram I, Bray F. Planning for tomorrow: global cancer incidence and the role of prevention 2020-2070. Nat Rev Clin Oncol 2021;18:663-72. [Crossref] [PubMed]
- Mullard A. Addressing cancer's grand challenges. Nat Rev Drug Discov 2020;19:825-6. [Crossref] [PubMed]
- Schadendorf D, van Akkooi ACJ, Berking C, et al. Melanoma. Lancet 2018;392:971-84. [Crossref] [PubMed]
- Siegel RL, Miller KD, Fuchs HE, et al. Cancer statistics, 2022. CA Cancer J Clin 2022;72:7-33. [Crossref] [PubMed]
- Sung H, Ferlay J, Siegel RL, et al. Global Cancer Statistics 2020: GLOBOCAN Estimates of Incidence and Mortality Worldwide for 36 Cancers in 185 Countries. CA Cancer J Clin 2021;71:209-49. [Crossref] [PubMed]
- Chen ML, de Vere Hunt IJ, John EM, et al. Differences in Thickness-Specific Incidence and Factors Associated With Cutaneous Melanoma in the US From 2010 to 2018. JAMA Oncol 2022;8:755-9. [Crossref] [PubMed]
- Demer AM, Hanson JL, Maher IA, et al. Association of Mohs Micrographic Surgery vs Wide Local Excision With Overall Survival Outcomes for Patients With Melanoma of the Trunk and Extremities. JAMA Dermatol 2021;157:84-9. [Crossref] [PubMed]
- Allais BS, Beatson M, Wang H, et al. Five-year survival in patients with nodular and superficial spreading melanomas in the US population. J Am Acad Dermatol 2021;84:1015-22. [Crossref] [PubMed]
- Aggarwal P, Knabel P, Fleischer AB Jr. United States burden of melanoma and non-melanoma skin cancer from 1990 to 2019. J Am Acad Dermatol 2021;85:388-95. [Crossref] [PubMed]
- Sung H, Siegel RL, Rosenberg PS, et al. Emerging cancer trends among young adults in the USA: analysis of a population-based cancer registry. Lancet Public Health 2019;4:e137-47. [Crossref] [PubMed]
- Gershenwald JE, Scolyer RA, Hess KR, et al. Melanoma staging: Evidence-based changes in the American Joint Committee on Cancer eighth edition cancer staging manual. CA Cancer J Clin 2017;67:472-92.
- Barreiro-Capurro A, Andrés-Lencina JJ, Podlipnik S, et al. Differences in cutaneous melanoma survival between the 7th and 8th edition of the American Joint Committee on Cancer (AJCC). A multicentric population-based study. Eur J Cancer 2021;145:29-37.
- Elmore JG, Elder DE, Barnhill RL, et al. Concordance and Reproducibility of Melanoma Staging According to the 7th vs 8th Edition of the AJCC Cancer Staging Manual. JAMA Netw Open 2018;1:e180083.
- Murphy GF, Wilson BJ, Girouard SD, et al. Stem cells and targeted approaches to melanoma cure. Mol Aspects Med 2014;39:33-49. [Crossref] [PubMed]
- Li X, Zhao Z, Zhang M, et al. Research progress of microneedles in the treatment of melanoma. J Control Release 2022;348:631-47. [Crossref] [PubMed]
- Pretto F, Neri D. Pharmacotherapy of metastatic melanoma: emerging trends and opportunities for a cure. Pharmacol Ther 2013;139:405-11. [Crossref] [PubMed]
- Guo W, Wang H, Li C. Signal pathways of melanoma and targeted therapy. Signal Transduct Target Ther 2021;6:424. [Crossref] [PubMed]
- Balachandran VP, Gonen M, Smith JJ, et al. Nomograms in oncology: more than meets the eye. Lancet Oncol 2015;16:e173-80. [Crossref] [PubMed]
- Verver D, van Klaveren D, Franke V, et al. Development and validation of a nomogram to predict recurrence and melanoma-specific mortality in patients with negative sentinel lymph nodes. Br J Surg 2019;106:217-25. [Crossref] [PubMed]
- Li W, Xiao Y, Xu X, et al. A Novel Nomogram and Risk Classification System Predicting the Cancer-Specific Mortality of Patients with Initially Diagnosed Metastatic Cutaneous Melanoma. Ann Surg Oncol 2021;28:3490-500. [Crossref] [PubMed]
- Li X, Hu M, Gu W, et al. Nomogram Predicting Cancer-Specific Death in Parotid Carcinoma: a Competing Risk Analysis. Front Oncol 2021;11:698870. [Crossref] [PubMed]
- Hu M, Li X, Gu W, et al. A Competing Risk Nomogram for Predicting Cancer-Specific Death of Patients With Maxillary Sinus Carcinoma. Front Oncol 2021;11:698955. [Crossref] [PubMed]
- Peng Y, Hu T, Cheng L, et al. Evaluating and Balancing the Risk of Breast Cancer-Specific Death and Other Cause-Specific Death in Elderly Breast Cancer Patients. Front Oncol 2021;11:578880. [Crossref] [PubMed]
- Badakhshi H, Wang ZM, Li RJ, et al. Survival and Prognostic Nomogram for Primary Gastrointestinal Melanoma (PGIM): A Population-based Study. Anticancer Res 2021;41:967-74. [Crossref] [PubMed]
- Xu QQ, Li QJ, Chen L, et al. A nomogram for predicting survival of head and neck mucosal melanoma. Cancer Cell Int 2021;21:224. [Crossref] [PubMed]
- Zhou H, Zou X, Li H, et al. Construction and validation of a prognostic nomogram for primary vulvar melanoma: a SEER population-based study. Jpn J Clin Oncol 2020;50:1386-94. [Crossref] [PubMed]
- Doll KM, Rademaker A, Sosa JA. Practical Guide to Surgical Data Sets: Surveillance, Epidemiology, and End Results (SEER) Database. JAMA Surg 2018;153:588-9. [Crossref] [PubMed]
- Wu WT, Li YJ, Feng AZ, et al. Data mining in clinical big data: the frequently used databases, steps, and methodological models. Mil Med Res 2021;8:44. [Crossref] [PubMed]
- Global Burden of Disease 2019 Cancer Collaboration, Kocarnik JM, Compton K, et al. Cancer Incidence, Mortality, Years of Life Lost, Years Lived With Disability, and Disability-Adjusted Life Years for 29 Cancer Groups From 2010 to 2019: A Systematic Analysis for the Global Burden of Disease Study 2019. JAMA Oncol 2022;8:420-44.
- Zheng Y, Cong C, Su C, et al. Epidemiology and survival outcomes of primary gastrointestinal melanoma: a SEER-based population study. Int J Clin Oncol 2020;25:1951-9. [Crossref] [PubMed]
- Weinstock MA, Lott JP, Wang Q, et al. Skin biopsy utilization and melanoma incidence among Medicare beneficiaries. Br J Dermatol 2017;176:949-54. [Crossref] [PubMed]
- Khan F, Strohl A, Allen PD, et al. Desmoplastic Melanoma of the Head and Neck: Incidence and Survival, 1992-2013. Otolaryngol Head Neck Surg 2017;157:648-56. [Crossref] [PubMed]
- Xiao Y, Peng S, Hu Y, et al. Development and validation of prognostic nomogram in patients with nonmetastatic malignant melanoma: a SEER population-based study. Cancer Med 2020;9:8562-70. [Crossref] [PubMed]
- Zeng Q, Yao Y, Zhao M. Development and validation of a nomogram to predict cancer-specific survival of uveal melanoma. BMC Ophthalmol 2021;21:230. [Crossref] [PubMed]
- Zaorsky NG, Wang X, Garrett SM, et al. Pan-cancer analysis of prognostic metastatic phenotypes. Int J Cancer 2022;150:132-41. [Crossref] [PubMed]
- Yang Y, Li Y, Qi R, et al. Development and Validation of a Combined Glycolysis and Immune Prognostic Model for Melanoma. Front Immunol 2021;12:711145. [Crossref] [PubMed]
- Pardoll DM. The blockade of immune checkpoints in cancer immunotherapy. Nat Rev Cancer 2012;12:252-64. [Crossref] [PubMed]
- Ruocco MR, Avagliano A, Granato G, et al. Metabolic flexibility in melanoma: A potential therapeutic target. Semin Cancer Biol 2019;59:187-207. [Crossref] [PubMed]
- Tumeh PC, Harview CL, Yearley JH, et al. PD-1 blockade induces responses by inhibiting adaptive immune resistance. Nature 2014;515:568-71. [Crossref] [PubMed]
- Snyder A, Makarov V, Merghoub T, et al. Genetic basis for clinical response to CTLA-4 blockade in melanoma. N Engl J Med 2014;371:2189-99. [Crossref] [PubMed]
- Andrews MC, Duong CPM, Gopalakrishnan V, et al. Gut microbiota signatures are associated with toxicity to combined CTLA-4 and PD-1 blockade. Nat Med 2021;27:1432-41. [Crossref] [PubMed]
- Boutros C, Tarhini A, Routier E, et al. Safety profiles of anti-CTLA-4 and anti-PD-1 antibodies alone and in combination. Nat Rev Clin Oncol 2016;13:473-86. [Crossref] [PubMed]
- D'Angelo SP, Larkin J, Sosman JA, et al. Efficacy and Safety of Nivolumab Alone or in Combination With Ipilimumab in Patients With Mucosal Melanoma: A Pooled Analysis. J Clin Oncol 2017;35:226-35. [Crossref] [PubMed]
- Larkin J, Chiarion-Sileni V, Gonzalez R, et al. Five-Year Survival with Combined Nivolumab and Ipilimumab in Advanced Melanoma. N Engl J Med 2019;381:1535-46. [Crossref] [PubMed]
- Luke JJ, Flaherty KT, Ribas A, et al. Targeted agents and immunotherapies: optimizing outcomes in melanoma. Nat Rev Clin Oncol 2017;14:463-82. [Crossref] [PubMed]
- McDermott D, Lebbé C, Hodi FS, et al. Durable benefit and the potential for long-term survival with immunotherapy in advanced melanoma. Cancer Treat Rev 2014;40:1056-64. [Crossref] [PubMed]
- Huang AC, Zappasodi R. A decade of checkpoint blockade immunotherapy in melanoma: understanding the molecular basis for immune sensitivity and resistance. Nat Immunol 2022;23:660-70. [Crossref] [PubMed]
- Cullison CR, Zheng DX, Levoska MA, et al. Inconsistencies in Cutaneous Melanoma Staging Within SEER Registries. JAMA Dermatol 2021;157:727-9. [Crossref] [PubMed]
(English Language Editor: J. Jones)