N6-methyladenosine methylation-related genes YTHDF2, METTL3, and ZC3H13 predict the prognosis of hepatocellular carcinoma patients
Highlight box
Key findings
• The m6A methylation-related genes could potential be high-risk independent prognostic factors, including YTHDF2, METTL3, and ZC3H13, providing a theoretical basis for the prognosis evaluation of patients with HCC.
What is known and what is new?
• High expression of YTHDF2, METTL3, ZC3H13 was associated with higher follicular helper T cell counts and lower resting memory CD4 T cell counts, which provided our research on immune cells in the tumor microenvironment of HCC.
• High expression of ZC3H13 is associated with poor prognosis of HCC, while high expression in clinical samples means longer survival, which provides a real-world basis for our study.
What is the implication, and what should change now?
• Multivariable prediction models of potential biomarkers associated with HCC progression could further guide the precise treatment.
• The genes of YTHDF2, METTL3, ZC3H13 could be as the novel targets for HCC therapy.
Introduction
Hepatocellular carcinoma (HCC) is one of the most common cancers in Asia and a common cause of cancer-related death in humans (1). It is estimated that in 2018, there were approximately 42,220 new cases of liver and intrahepatic bile duct cancers and 30,200 deaths in America (2). Moreover, the overall 1- and 3-year survival rates of HCC patients are only 36% and 17%, respectively (3). Although significant progress has been made in HCC therapy in recent years, such as surgical resection, systemic therapy, radiofrequency ablation, and liver transplantation, HCC patients’ prognoses remain poor (4). Also, due to individual differences, HCC patients with the same tumor stage or other similarities in clinicopathological characteristics may have different prognoses. Hence, developing new biomarkers to precisely predict the prognosis of HCC patients is vital because HCC is a heterogeneous disease with a dismal prognosis. With the rapid development of gene sequencing technology, multivariable prediction models have been suggested for potential biomarkers associated with HCC progression. However, the study of these multiple biomarker models is limited (5).
N6-methyladenosine (m6A) methylation is the most common RNA modification in human cells (5). Extensive research has shown that m6A methylation regulates cellular processes, including cell self-renewal, differentiation, invasion, and apoptosis, by modulating gene expression (5). Modulators of m6A methylation play a significant and pleiotropic role in the regulation of HCC. But now the studies about prognostic function of m6A genes on HCC were explored only based on bioinformatics analysis (6,7). The accurate role of m6A methylation in the tumorigenesis and progress of HCC is still not well defined neither verified by clinical cases. Messenger RNA (mRNA) and non-coding RNA (ncRNA) are involved in m6A-mediated biological processes in HCC (7). Some studies found that the most common m6A-driven regulatory functions in HCC are mRNA stability and degradation (6,7). Moreover, ncRNAs can be regulated by the m6A modification in various ways, including preprocessing, splicing, decay, and stability; thus, ncRNAs affect and participate in the m6A process of HCC (7). According to existing literature, m6A modification regulators include “writers”, which are methyltransferases that are mainly responsible for transferring the methyl group to the N6 position; “readers”, which are RNA-binding proteins that regulate RNA functions by recognizing specific m6A-modified positions; and “erasers”, which are demethylases that remove the methyl group (8,9). The m6A methylation-related genes, including “writer” genes (RBM15/15B, METTL3, METTL14, WTAP VIRMA, and ZC3H13), “reader” genes (IGF2BP1/2/3, YTHDC1/2, YTHDF1/2/3, HNRNP, and eIF3), and “eraser” genes (ALKBH3, ALKBH5, and FTO), interact to ensure that the dynamic and reversible balance of m6A methylation is cooperatively maintained (10).
Upon consulting the literature, we found some previous m6A methylation research in HCC. For example, METTL3 has been found to be related to the poor prognosis of HCC patients (11). It promotes the proliferation, migration, and colony formation of HCC cells via posttranscriptional silencing of SOCS2, which depends on YTHDF2 (11). In addition, METTL14 is a reader that plays a beneficial role in HCC by regulating m6A-dependent miRNA processing (12). MiR-145 downregulates YTHDF2 by targeting the 3'-untranslated region (3'-UTR). In summary, the upregulation of METTL3 or downregulation of METL14 can predict poor prognosis for HCC patients and lead to HCC progression and metastasis (12). METTL3 inhibits the expression of SOCS2 in HCC via the miR-145/m6A/YTHDF2 axis (11). These studies provide a new dimension for investigating epigenetic changes in liver cancer. To explore the prognostic model of effectively predicted the prognosis of HCC patients based on m6A methylation-related genes, in this study, we conducted extensive analyses based on The Cancer Genome Atlas (TCGA) and International Cancer Genome Consortium (ICGC) databases. We used consensus clustering analysis, least absolute shrinkage and selection operator (LASSO) regression, and Cox regression analyses to develop m6A methylation-related gene signatures. Finally, using comprehensive bioinformatics analyses, we developed a novel prognostic model based on m6A modifications as an independent risk factor predicting HCC prognosis, which we preliminarily verified with clinical cases to provide potential therapeutic targets for HCC. We present the following article in accordance with the TRIPOD reporting checklist (available at https://atm.amegroups.com/article/view/10.21037/atm-22-5964/rc).
Methods
Data download and processing
The potential m6A methylation-related genes, including KIAA1429, METTL14, RBM15, METTL3, WTAP, ZC3H13, HNRNPC, YTHDC1, YTHDC2, YTHDF1, YTHDF2, ALKBH5, and FTO were obtained. The HCC RNA-seq transcriptome and clinical data were obtained from the TCGA database. Patients with incomplete survival time, survival status, or clinicopathological characteristics were excluded. The ICGC cohort was used to validate the prognostic model.
Consensus clustering analysis
The HCC patients were clustered into different subgroups by consensus expression of m6A methylation-related genes with the “Consensus Cluster Plus” R package (13). The accuracy of the clustering results was verified by principal component analysis (PCA). A Kaplan-Meier analysis was used to calculate the overall survival (OS) difference between clusters. The Wilcoxon rank-sum test or the Kruskal-Wallis test was used to analyze the differences in baseline characteristics, such as age, gender, grade, and stage between clusters.
Construction and validation of the prognostic model
We used a univariate Cox regression analysis to identify the prognostic m6A methylation-related genes. We performed a LASSO regression analysis, a machine learning algorithm, to eliminate over-fitting (14). All the m6A methylation-related genes identified as independent prognostic factors of OS were then screened by multivariate Cox regression analysis, and their regression coefficients were calculated.
Risk scores were conducted by multivariable fractional polynomials (MFPs) (14). The calculation was conducted as follows: (where vi is the gene expression and ci is the regression coefficient). Based on the median risk score, HCC patients were divided into high- and low-risk groups. A Kaplan-Meier survival curve was used to compare OS between the two risk subgroups. In addition, the prognostic model’s accuracy was analyzed by a receiver operating characteristic (ROC) curve.
To further ensure the stability of the prognostic model, we calculated the risk score of HCC patients in the ICGC cohort. A Kaplan-Meier survival curve and survival ROC curve were developed to show the predictive ability of the prognostic model in the validation cohort. In the meantime, an independent prognostic analysis was performed to predict whether the prognostic model could be used as an independent factor for HCC prognosis.
Tumor immune microenvironment evaluation
The tumor microenvironment (TME) scores and the fraction of 21 types of immune cells were obtained from CIBERSORT in R software and were used to further explore the tumor immune microenvironment differences among different subgroups of the prognostic model. A P value <0.05 was defined as statistically significant (15).
m6A methylation-related gene validation by other databases
To explore whether the expression of m6A methylation-related genes was different between HCC and other liver cancers, we analyzed several databases, including Oncomine, the Human Protein Atlas (HPA), and the Kaplan-Meier plotter online tool (16). The gene and protein expressions of the m6A methylation-related genes in HCC tissues and liver tissues were obtained from the Oncomine database and the HPA. The prognostic value of m6A methylation-related genes in HCC was verified by the Kaplan-Meier plotter online tool.
Clinical sample validation
To verify the conclusions drawn by the above analysis, we collected pathological specimens from patients from the Department of Pathology, Affiliated Hospital of Qingdao University, who had undergone liver cancer surgery (the samples had previously been analyzed and diagnosed as HCC by the Department of Pathology). Specimens from 2015 to 2020 were analyzed by immunohistochemical staining. The study was approved by the Institutional Review Board of the Affiliated Hospital of Qingdao University Ethics Committee for Clinical Investigation (No. QYFYKYLL471311920), and conducted in accordance with the Declaration of Helsinki (as revised in 2013) and good clinical practice. All patients in our study had undergone liver surgery without comprehensive systemic treatment due to complex and heterogeneous reasons. And all of them signed informed consent of this study.
Statistical analysis
All bioinformatics analysis were performed by Perl language and R software 3.6.1. Univariate and multivariate Cox regression, LASSO regression, ROC curve, and Kaplan-Meier survival analyses were performed by R software and corresponding R packages. For all comparisons, a statistically significant difference was defined as a P value <0.05.
The clinical sample size was calculated using PASS software version 15.0 to obtain a 90% confidence level and 90% study power. In the randomized cohorts, we enrolled 54 evaluable patients, 27 of whom were positive for METTL3, YTHDF2, and ZC3H13 staining and compared their samples with the adjacent normal tissues, and 27 patients negative for METTL3, YTHDF2, and ZC3H13. All patients who survived approximately 6 months or longer would provide 90% power to detect a doubling of the median progression-free survival (PFS) of more than 4.0 months at a two-sided α significance level of 0.05.
The Kaplan-Meier method was used to analyze the survival analysis of PFS and OS, and the differences between groups was evaluated by the log-rank test. The hazard ratios (HRs) were calculated by Cox proportional hazards model with 95% as their corresponding confidence intervals (CIs). For all evaluations, the P value of 0.05 was used to indicate statistical significance. The SPSS 20 software (IBM Corp., Armonk, NY, USA) and Graph Pad Prism 9 (Graph Pad Software, Inc., San Diego, CA, USA) were used to analyze all the results. All experiments were replicated three times. P<0.05 was considered a statistically significant difference.
Results
Clinical data of HCC patients
A total of 273 HCC patients were included in the TCGA cohort, comprising 186 males and 87 females from 2001 to 2020. There were 260 patients in the ICGC cohort, comprising 192 males and 68 females from 2001 to 2020 (Table 1) (17).
Table 1
Clinical characteristics | TCGA cohorts (n=273) | ICGC cohorts (n=260) |
---|---|---|
Total cases | ||
Survival status, n (%) | ||
Alive | 186 (68.1) | 214 (82.3) |
Dead | 87 (31.9) | 46 (17.7) |
Age (years), n (%) | ||
<65 | 174 (63.7) | 91 (35.0) |
≥65 | 99 (36.3) | 169 (65.0) |
Gender, n (%) | ||
Male | 186 (38.1) | 192 (73.8) |
Female | 87 (31.9) | 68 (26.2) |
Tumor stage, n (%) | ||
I + II | 196 (71.8) | 157 (60.4) |
III + IV | 77 (28.2) | 103 (39.6) |
Metastasis stage, n (%) | ||
M0 | 269 (98.5) | – |
M1 | 4 (1.5) | – |
HCC, hepatocellular carcinoma; TCGA, The Cancer Genome Atlas; ICGC, International Cancer Genome Consortium.
Differentially expressed m6A methylation-related genes
We visualized the differential expression of m6A methylation-related genes between tumor tissues and normal tissues with a heatmap. The expression of RBM15, YTHDC1, KIAA1429, YTHDC2, ALKBH5, HNRNPC, METTL3, YTHDF2, FTO, WTAP, and YTHDF1 was significantly higher in tumor samples than in normal tissues (Figure 1A). Additionally, the expression pattern of the differentially expressed m6A methylation-related genes was visualized by volcano plots and box plots (Figure 1B). Among all the interactions of m6A methylation-related genes, Pearson correlation analysis showed that METTL3 was most associated with HNRNPC (r=0.72) (Figure 1C).
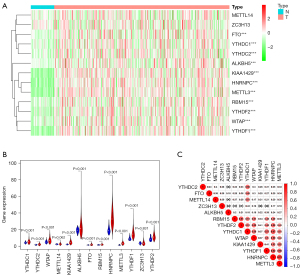
Consensus clustering of m6A methylation-related genes
To gain much more insight into the molecular heterogeneity of HCC and explore whether m6A methylation-related genes presented discernable patterns in HCC, we performed an unsupervised consensus analysis of all samples. The result of k=2 seemed to be more accurate, stratifying the samples into two subgroups with less correlation between them (Figure 2A-2C). Then, we performed a PCA to show the effect of the stratification on the transcriptional profile between cluster 1 and cluster 2 (Figure 2D). The 5-year OS of cluster 1 was significantly longer than that of cluster 2 (P<0.001) (Figure 2E). Then, the associations between the clusters and clinicopathological features were evaluated. Significant differences were found in features between cluster 1 and cluster 2, including grade (P<0.05), sex (P<0.005), and survival (P<0.05) (Figure 2F).
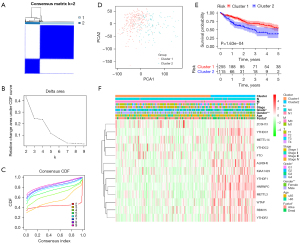
Construction and validation of the prognostic model
To explore the prognostic value of m6A methylation-related genes in HCC, we first performed a univariate Cox regression analysis to identify OS-associated genes (Figure 3A). Then, we performed LASSO regression and multivariate Cox regression analyses to establish an optimal multigene prognostic model for OS, which resulted in the identification of three genes: YTHDF2, METTL3, and ZC3H13 (Table 2, Figure 3B,3C). Our model for predicting prognosis based on these three m6A methylation-related genes used the following formula: risk score = (YTHDF2 expression × 0.094698) + (METTL3 expression × 0.195866) + (ZC3H13 expression × −0.16639).
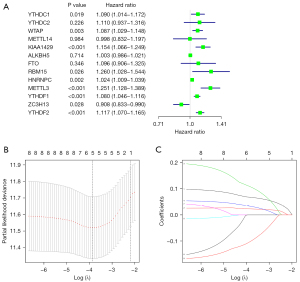
Table 2
Gene | Coef | HR | HR.95L | HR.95H | P value |
---|---|---|---|---|---|
YTHDF2 | 0.094697968 | 1.099326773 | 1.050486621 | 1.150437645 | 4.42E-05 |
METTL3 | 0.195865822 | 1.216363685 | 1.081123362 | 1.368521544 | 0.00112586 |
ZC3H13 | 0.16638959 | 0.846716298 | 0.765465428 | 0.9365916 | 0.001226368 |
m6A, N6-methyladenosine; coef, coefficient; HR, hazard ratio; HR.95L, hazard ratio with low 95% confidence index; HR.95H, hazard ratio with high 95% confidence index.
HCC patients were divided into low- and high-risk groups based on the median value of the risk score. The low-risk group had a higher survival rate than the high-risk group (HR, 1.547; 95% CI, 1.320–1.812; P<0.001) according to the Kaplan-Meier survival curves (Figure 4A). In addition, we assessed the accuracy of the OS-related prognostic model by constructing a ROC curve, and the AUC of the risk score was significantly higher than that of other clinicopathological parameters (Figure 4B). Finally, we ranked the HCC patients by risk score to analyze the survival distribution. As the risk score increased, the mortality rate of HCC patients also increased (Figure 4C,4D).
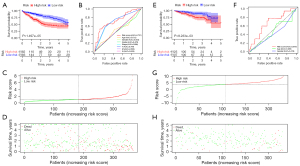
We also calculated the risk score of the ICGC cohort patients (Liver Cancer-RIKEN, Japan) to validate the formula externally. Similar to the TCGA cohort, the Kaplan-Meier survival curves and the ROC curve showed that the low-risk group had a higher survival rate than the high-risk group, and the risk score had a good ability to predict the prognosis of HCC patients (P<0.001) (Figure 4E,4F). Meanwhile, as the risk score increased, the mortality rate of HCC patients also increased (Figure 4G,4H). Therefore, these results revealed that the prognostic signature for OS described here could effectively identify high-risk HCC patients with relatively worse OS.
The relationships between the prognostic model and immune function
According to the univariate and multivariate Cox regression analysis results (P<0.001), our model can be used as a prognostic factor for HCC (Figure 5A,5B). Thus, we evaluated the association of the prognostic model and tumor-infiltrating immune cells in the HCC immune microenvironment using the CIBERSORT algorithm. The CIBERSORT results showed that the populations of 22 types of immune cells differed between the two subgroups (Figure 6A,6B). Moreover, there were more follicular helper T cells in the high-risk group (P=0.016), while there were fewer resting memory CD4 T cells in the low-risk group (P=0.014) (Figure 6C).
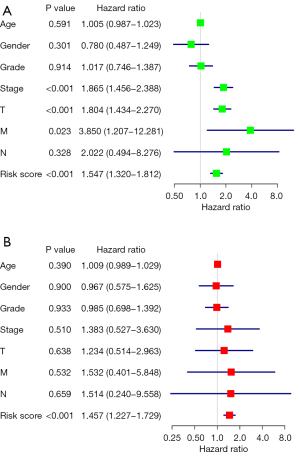
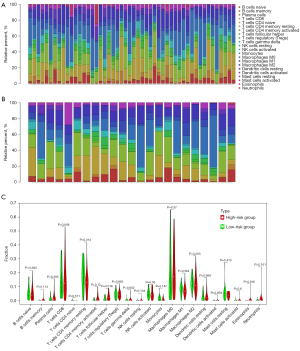
Expression of m6A methylation-related genes in samples from other databases
We analyzed the gene expression levels of YTHDF2, METTL3, and ZC3H13 in samples from different databases, including the Oncomine and HPA databases and the Kaplan-Meier plotter online tool. The expression levels of YTHDF2 and METTL3 in HCC were higher than those in other liver cancers in two Wurmbach Liver studies (222430_s_at, P=0.002; 209265_s_at, P=1.37e-05) (Figure 7A,7B). The expression level of ZC3H13 showed no prognostic value in the Oncomine dataset. Moreover, we verified the histological levels of YTHDF2, METTL3, and ZC3H13 expressions in samples from the HPA database. The results showed that the expression of YTHDF2 and ZC3H13 were upregulated in HCC tissues compared to normal tissues (Figure 7C,7D). The histological level of METTL3 was not detected in samples from the HPA database. The prognostic value of YTHDF2, METTL3, and ZC3H13 was evaluated with the Kaplan-Meier plotter online tool. We found that a high expression of YTHDF2 (HR, 1.52; 95% CI, 1.07–2.15; log-rank P=0.017) and METTL3 (HR, 1.77; 95% CI, 1.21–2.60; predicted poor survival, while a high expression of ZC3H13 (HR, 0.43; 95% CI, 0.26–0.69; log-rank P=0.00033) had a higher survival (Figure 7E-7G). These results show that YTHDF2, METTL3, and ZC3H13 are highly expressed in HCC tissues and closely related to OS in HCC patients.
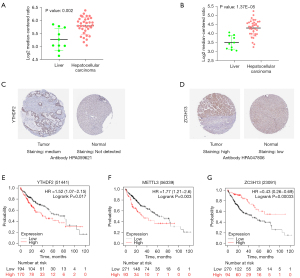
Clinical sample validation
To verify the conclusions drawn by the above analysis, we collected pathological specimens from 70 patients from the Department of Pathology, Affiliated Hospital of Qingdao University who had undergone liver cancer surgery (the samples had been previously analyzed and diagnosed as HCC by the Department of Pathology), and the specimens were analyzed using immunohistochemical staining. The patients were aged 18–65, with an average age of 47. Among them, 24 were female, and 46 were male. None had hepatitis B. Among the 70 HCC specimens that underwent immunohistochemical staining, the cancer tissues were all positive for METTL3, YTHDF2, and ZC3H13 compared with the adjacent tissues, as shown in Figure 8A-8C. Among them, in the METTL3-related immunohistochemical staining, 39 specimens (about 56%) were strongly stained, and 31 (about 44%) were weakly stained. In the YTHDF2-related immunohistochemical staining, there were 42 specimens that were strongly stained (about 60%) and 28 (about 40%) with weak staining. In the ZC3H13-related immunohistochemical staining, 43 specimens (about 61%) had strong staining, and 27 (about 39%) had weak staining. By collecting the clinical data of the patients, we conducted a Kaplan-Meier survival analysis. The results showed that patients with low METTL3 expression had a longer survival time than patients with high METTL3 expression, and patients with low YTHDF2 expression had a longer survival time than patients with high YTHDF2 expression. At the same time, patients with high ZC3H13 expression lived longer than those with low ZC3H13 expression. This result is consistent with the above bioinformatics analysis conclusion, as shown in Figure 8D-8F. Further prospective study to verify the correlations between the expressions of YTHDF2, METTL3, and ZC3H13 and the clinicopathological characteristics of HCC has been designed and added in the registered clinical trial (clinical trial No. NCT05292885).
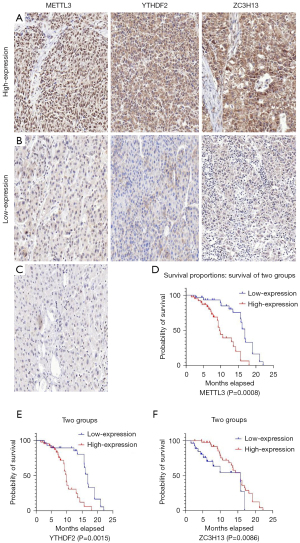
Discussion
The HCC carcinogenesis involves an intricate regulatory network. Compared to using a single clinicopathological parameter or gene, combining diverse biomarkers and establishing a prognostic model is a more effective way to predict tumor prognosis. It is now well-established that m6A methylation plays a significant role in various types of cancer. m6A methylation is ubiquitous in the occurrence and development of cancer. A prognostic model based on selected m6A methylation-related genes may be more accurate and effective than a single clinicopathological parameter.
Herein, we analyzed the relationship between m6A methylation-related genes and the prognosis of HCC patients. We obtained the expression patterns of m6A methylation-related genes in samples from the TCGA database. Moreover, we identified three m6A methylation-related genes (YTHDF2, METTL3, ZC3H13) by multivariate Cox regression analysis and used them to construct the prognostic model. We verified the prognostic model via the ICGC cohort, thus indicating that the model could be used as an independent prognostic biomarker for HCC patients.
Previous studies indicated m6A genes could regulate the PD-L1 expression and immune infiltration in multiple solid tumors (18-20). But in HCC the relationship between m6A regulation and tumor immune microenvironment remains ambiguous. A study of 18 m6A regulators (21) indicated HCC patients with higher m6A scores associated with higher PD-L1 expression. However, the patient with low m6A scores might be benefit from immune checkpoint inhibitor therapy according to clinical outcomes. May be the m6A regulation group of the study mentioned above both associated the PD-L1 expression and TME cell infiltration (21). Although the results was still debated, it can be confirmed that m6A regulation is a potential biomarker for prognostic prediction of immunotherapy for HCC. And the accurate prognostic model should further explored. Herein, the CIBERSORT results showed higher counts of follicular helper T cells in the high-risk group, while the resting memory CD4 T cell counts were higher in the low-risk group. Follicular helper T cells, which are key regulators in the tumor immune microenvironment, are a specialized type of T cell that helps B cells and drives the response of germinal centers (22-24). Recent evidence suggests that follicular helper T cells participate in antitumor immune responses (25). However, only a few studies have reported the relationship between resting CD4 memory T cells and cancer immunotherapy. According to these results high-risk patients identified by our prognostic model may be sensitive to immunotherapy. And our results indicated a novel insight to the relationship of the m6A regulation and the HCC immunotherapy.
YTHDF2, the first functionally verified m6A reader, promotes the degradation of m6A-modified mRNAs in humans (26). A previous study has shown that YTHDF2 destabilizes m6A-containing RNA via direct recruitment of the CCR4-NOT deadenylase complex. And the CCR4-NOT deadenylase complex is recruited to m6A-containing RNAs through a direct interaction with the N-terminal region of YTHDF2 (27). YTHDF2 has a vast impact on mRNA degradation in different tissues and cell types in vertebrates (28). In addition, YTHDF2 may act as a tumor suppressor to repress cell proliferation and growth by destabilizing EGFR mRNA in HCC cells (29). Our results further revealed that YTHDF2 might play an important role in liver cancer development and could be used as a therapeutic target for HCC patients. METTL3, a RNA methyltransferase, has been implicated in mRNA decay, biogenesis, and translation control through m6A modification (30). Some studies have shown that METTL3-mediated m6A modification is involved in regulating numerous genes in various types of cancer (30-33). Moreover, one study reported that crosstalk between METTL3 and miR-186 regulates hepatoblastoma progression through the Wnt/β-catenin signaling pathway (34). Another study showed that METTL3 promotes the progression of HCC via m6A-mediated upregulation of microRNA-873-5p (35). Our observations suggest that METTL3 abnormalities contribute to an increased risk of developing HCC. ZC3H13, a canonical CCCH zinc finger protein, plays an important role in modulating RNA m6A methylation in the nucleus (36,37). One report has stated that ZC3H13 suppresses colorectal cancer proliferation and invasion by inactivating Ras-ERK signaling (36). However, there are few studies about the mechanism of ZC3H13 in cancer. Our findings suggest that ZC3H13 is a promising diagnostic marker in HCC. The HCC RNA-seq transcriptome and clinical data in our study were all obtained from the TCGA database. All the conclusion should be verified and other biomarkers should be further studied by clinical trials. According to our study, the YTHDF2, METTL3, and ZC3H13 potential predict the prognosis of HCC patients independently. And the interactions of the three genes should be further explored.
In addition, we assessed the expression of the three m6A methylation-related genes in samples from other databases. YTHDF2, METTL3, and ZC3H13 were highly expressed in HCC tissues and closely related to OS in HCC patients. Based on existing reports, the mechanism of YTHDF2, METTL3, and ZC3H13 in HCC remains unclear. Our study firstly used multiple databases to establish a prognostic model related to YTHDF2, METTL3, and ZC3H13 expression for the prognosis predicting of HCC, which maybe provide novel treatment strategy for HCC. However, our research still has certain limitations. Firstly, it is a retrospective study, there may be biases concerning the choice of variables. Second, the mechanisms of action of the m6A methylation-related genes in HCC need to be validated by in vivo and in vitro experiments to confirm our results. Third, all the samples were nonrepresentative, and samples from clinical trials should be further studied. Finally, our sample size was limited with few events per predictor. And missing data may have also potentially influenced the objectivity of the study.
Conclusions
In conclusion, our research systematically demonstrated that the m6A methylation-related genes YTHDF2, METTL3, and ZC3H13 were significantly associated with the tumor microenvironment and may have further potential to effectively predict the prognosis of HCC patients.
Acknowledgments
The authors appreciate the academic support from the AME Hepatocellular Carcinoma Collaborative Group.
Funding: This study was supported, in part, by Shandong Medical and Health Science and Technology Development Program (No. 202102040497).
Footnote
Reporting Checklist: The authors have completed the TRIPOD reporting checklist. Available at https://atm.amegroups.com/article/view/10.21037/atm-22-5964/rc
Data Sharing Statement: Available at https://atm.amegroups.com/article/view/10.21037/atm-22-5964/dss
Conflicts of Interest: All authors have completed the ICMJE uniform disclosure form (available at https://atm.amegroups.com/article/view/10.21037/atm-22-5964/coif). The authors have no conflicts of interest to declare.
Ethical Statement: The authors are accountable for all aspects of the work in ensuring that questions related to the accuracy or integrity of any part of the work are appropriately investigated and resolved. The study was approved the Institutional Review Board of the Affiliated Hospital of Qingdao University Ethics Committee for Clinical Investigation (No. QYFYKYLL471311920) and conducted in accordance with the Declaration of Helsinki (as revised in 2013) and good clinical practice. Informed consent was taken from all the patients.
Open Access Statement: This is an Open Access article distributed in accordance with the Creative Commons Attribution-NonCommercial-NoDerivs 4.0 International License (CC BY-NC-ND 4.0), which permits the non-commercial replication and distribution of the article with the strict proviso that no changes or edits are made and the original work is properly cited (including links to both the formal publication through the relevant DOI and the license). See: https://creativecommons.org/licenses/by-nc-nd/4.0/.
References
- Nakano S, Eso Y, Okada H, et al. Recent Advances in Immunotherapy for Hepatocellular Carcinoma. Cancers (Basel) 2020;12:775. [Crossref] [PubMed]
- Bray F, Ferlay J, Soerjomataram I, et al. Global cancer statistics 2018: GLOBOCAN estimates of incidence and mortality worldwide for 36 cancers in 185 countries. CA Cancer J Clin 2018;68:394-424. [Crossref] [PubMed]
- El-Serag HB. Hepatocellular carcinoma: recent trends in the United States. Gastroenterology 2004;127:S27-34. [Crossref] [PubMed]
- Zheng Z, Liang W, Wang D, et al. Adjuvant chemotherapy for patients with primary hepatocellular carcinoma: a meta-analysis. Int J Cancer 2015;136:E751-9. [Crossref] [PubMed]
- Huo J, Wu L, Zang Y. Development and validation of a CTNNB1-associated metabolic prognostic model for hepatocellular carcinoma. J Cell Mol Med 2021;25:1151-65. [Crossref] [PubMed]
- Huang H, Bai Y, Lu X, et al. N6-methyladenosine associated prognostic model in hepatocellular carcinoma. Ann Transl Med 2020;8:633. [Crossref] [PubMed]
- Lu J, Qian J, Yin S, et al. Mechanisms of RNA N(6)-Methyladenosine in Hepatocellular Carcinoma: From the Perspectives of Etiology. Front Oncol 2020;10:1105. [Crossref] [PubMed]
- Liu N, Dai Q, Zheng G, et al. N(6)-methyladenosine-dependent RNA structural switches regulate RNA-protein interactions. Nature 2015;518:560-4. [Crossref] [PubMed]
- Meyer KD, Jaffrey SR. Rethinking m(6)A Readers, Writers, and Erasers. Annu Rev Cell Dev Biol 2017;33:319-42. [Crossref] [PubMed]
- Wang T, Kong S, Tao M, et al. The potential role of RNA N6-methyladenosine in Cancer progression. Mol Cancer 2020;19:88. [Crossref] [PubMed]
- Chen M, Wei L, Law CT, et al. RNA N6-methyladenosine methyltransferase-like 3 promotes liver cancer progression through YTHDF2-dependent posttranscriptional silencing of SOCS2. Hepatology 2018;67:2254-70. [Crossref] [PubMed]
- Ma JZ, Yang F, Zhou CC, et al. METTL14 suppresses the metastatic potential of HCC by modulating m6 A-dependent primary miRNA processing. Hepatology 2017;65:529-43. [Crossref] [PubMed]
- Wilkerson MD, Hayes DN. ConsensusClusterPlus: a class discovery tool with confidence assessments and item tracking. Bioinformatics 2010;26:1572-3. [Crossref] [PubMed]
- Sauerbrei W, Royston P, Binder H. Selection of important variables and determination of functional form for continuous predictors in multivariable model building. Stat Med 2007;26:5512-28. [Crossref] [PubMed]
- Chen B, Khodadoust MS, Liu CL, et al. Profiling Tumor Infiltrating Immune Cells with CIBERSORT. Methods Mol Biol 2018;1711:243-59. [Crossref] [PubMed]
- Wang L, Zhou N, Qu J, et al. Identification of an RNA binding protein-related gene signature in hepatocellular carcinoma patients. Mol Med 2020;26:125. [Crossref] [PubMed]
- Rhodes DR, Yu J, Shanker K, et al. ONCOMINE: a cancer microarray database and integrated data-mining platform. Neoplasia 2004;6:1-6. [Crossref] [PubMed]
- Yi L, Wu G, Guo L, et al. Comprehensive Analysis of the PD-L1 and Immune Infiltrates of m6A RNA Methylation Regulators in Head and Neck Squamous Cell Carcinoma. Mol Ther Nucleic Acids 2020;21:299-314. [Crossref] [PubMed]
- Zhang B, Wu Q, Li B, et al. m(6)A regulator-mediated methylation modifcation patterns and tumor microenvironment infltration characterization in gastric cancer. Mol Cancer. 2020;19:53. [Crossref] [PubMed]
- Chong W, Shang L, Liu J, et al. m6A regulator-based methylation modification patterns characterized by distinct tumor microenvironment immune profiles in colon cancer. Theranostics 2021;11:2201-17. [Crossref] [PubMed]
- Yin T, Zhao L, Yao S. Comprehensive characterization of m6A methylation and its impact on prognosis, genome instability, and tumor microenvironment in hepatocellular carcinoma. BMC Med Genomics 2022;15:53. [Crossref] [PubMed]
- Hetta HF, Elkady A, Yahia R, et al. T follicular helper and T follicular regulatory cells in colorectal cancer: A complex interplay. J Immunol Methods 2020;480:112753. [Crossref] [PubMed]
- Guisier F, Barros-Filho MC, Rock LD, et al. Janus or Hydra: The Many Faces of T Helper Cells in the Human Tumour Microenvironment. Adv Exp Med Biol 2020;1224:35-51. [Crossref] [PubMed]
- Panneton V, Chang J, Witalis M, et al. Inducible T-cell co-stimulator: Signaling mechanisms in T follicular helper cells and beyond. Immunol Rev 2019;291:91-103. [Crossref] [PubMed]
- Qian G, Wu M, Zhao Y, et al. Thyroid cancer metastasis is associated with an overabundance of defective follicular helper T cells. APMIS 2020;128:487-96. [Crossref] [PubMed]
- Wang X, Lu Z, Gomez A, et al. N6-methyladenosine-dependent regulation of messenger RNA stability. Nature 2014;505:117-20. [Crossref] [PubMed]
- Du H, Zhao Y, He J, et al. YTHDF2 destabilizes m(6)A-containing RNA through direct recruitment of the CCR4-NOT deadenylase complex. Nat Commun 2016;7:12626. [Crossref] [PubMed]
- Fei Q, Zou Z, Roundtree IA, et al. YTHDF2 promotes mitotic entry and is regulated by cell cycle mediators. PLoS Biol 2020;18:e3000664. [Crossref] [PubMed]
- Zhong L, Liao D, Zhang M, et al. YTHDF2 suppresses cell proliferation and growth via destabilizing the EGFR mRNA in hepatocellular carcinoma. Cancer Lett 2019;442:252-61. [Crossref] [PubMed]
- Lin S, Choe J, Du P, et al. The m(6)A Methyltransferase METTL3 Promotes Translation in Human Cancer Cells. Mol Cell 2016;62:335-45. [Crossref] [PubMed]
- Cai X, Wang X, Cao C, et al. HBXIP-elevated methyltransferase METTL3 promotes the progression of breast cancer via inhibiting tumor suppressor let-7g. Cancer Lett 2018;415:11-9. [Crossref] [PubMed]
- Lin A, Zhou M, Hua RX, et al. METTL3 polymorphisms and Wilms tumor susceptibility in Chinese children: A five-center case-control study. J Gene Med 2020;22:e3255. [Crossref] [PubMed]
- Liu ZF, Yang J, Wei SP, et al. Upregulated METTL3 in nasopharyngeal carcinoma enhances the motility of cancer cells. Kaohsiung J Med Sci 2020;36:895-903. [Crossref] [PubMed]
- Cui X, Wang Z, Li J, et al. Cross talk between RNA N6-methyladenosine methyltransferase-like 3 and miR-186 regulates hepatoblastoma progression through Wnt/β-catenin signalling pathway. Cell Prolif 2020;53:e12768. [Crossref] [PubMed]
- Zhao M, Jia M, Xiang Y, et al. METTL3 promotes the progression of hepatocellular carcinoma through m6A-mediated upregulation of microRNA-873-5p. Am J Physiol Gastrointest Liver Physiol 2020;319:G636.
- Zhu D, Zhou J, Zhao J, et al. ZC3H13 suppresses colorectal cancer proliferation and invasion via inactivating Ras-ERK signaling. J Cell Physiol 2019;234:8899-907. [Crossref] [PubMed]
- Wen J, Lv R, Ma H, et al. Zc3h13 Regulates Nuclear RNA m(6)A Methylation and Mouse Embryonic Stem Cell Self-Renewal. Mol Cell 2018;69:1028-38.e6. [Crossref] [PubMed]
(English Language Editor: D. Fitzgerald)