Identification and validation of endocrine resistance-related and immune-related long non-coding RNA (lncRNA) signatures for predicting endocrinotherapy response and prognosis in breast cancer
Highlight box
Key findings
• We thoroughly investigated immune-related lncRNAs affecting endocrine therapeutic response and prognosis in BRCA, constructed a stable prognostic signature and analyzed underlying mechanisms involving TME.
What is known and what is new?
• LncRNAs are intimately involved in tumorigenesis, drug resistance, and immune-related pathways of cancer. However, there has been less exploration of the roles played by lncRNAs and their effects on the TME in endocrine resistant BRCA.
• In this study, our evaluation of ERIR-lncRNAs not only constructs a prognostic signature that predicts response to endocrine therapy, while the analysis of TME contributes to a better understanding of the underlying molecular mechanisms of endocrine resistant BRCA.
What is the implication, and what should change now?
• The ERIR-lncRNA prognostic features may become a new target against endocrine resistance and provide new thoughts for clinical precision treatment.
Introduction
Breast cancer (BRCA) is the most common malignancy in women, and approximately 70–80% of BRCA cases are pathologically estrogen receptor (ER)-positive (1,2). ER, as a transcription factor, is one of the most established therapeutic targets in breast cancer by binding to estrogen to form dimers and moving toward the nucleus, interacting with estrogen response elements (ERE) and activating target genes together with other coactivators. It also binds to growth factor receptor tyrosine kinase and cell survival signaling molecules to make ER more active. Although endocrine therapy has considerably reduced the recurrence and mortality of BRCA, primary and acquired resistance remain a major challenge (3,4). Several mechanisms of endocrine resistance have been reported, such as aberrant regulation of ERα-36 converts breast cancer cells to a growth factor-dependent state, resulting in resistance to tamoxifen (5,6), and activation of bypasses between ER and growth factor receptors such as PI3K/Akt/mTOR or Ras/Raf/MAPK, and some epigenetic modulations such as histone de acetylases (HDACs) have also been suggested as potential mechanisms of endocrine therapy resistance (7-9), In addition, there is an inextricable link between endocrine resistance and the tumor microenvironment (TME) in BRCA (10-12). Recent studies suggest a role for the tumor-infiltrating lymphocytes (TILs) in ER+ tumors associated with poor response to endocrine therapy (13). Endocrine therapy also significantly affects macrophage infiltration and differentiation which induces immunosuppression (14,15). Furthermore, despite the limited infiltration of natural killer (NK) cells in hormone receptor (HR)+ tumors, HR+ BRCA cell lines are more susceptible to interleukin 2 (IL-2)-stimulated NK cell lysis than triple negative breast cancer (TNBC) or HER2+ cell lines, indicating that immunotherapy targeting NK cells may be a potential strategy for the treatment of HR+ tumors (16,17). The tumor immune microenvironment (TIME) is widely involved in tumorigenesis and endocrine resistance of BRCA, the combination of endocrine therapy and immunotherapy may provide long-term survival benefits and promote the development of individualized clinical treatments. Therefore, the role of immune-related factors in endocrine resistance BRCA deserves to be studied for identifying potential targets of early diagnosis and treatment.
Long non-coding RNAs (lncRNA) are highly heterogeneous RNA characterized by their length of more than 200 nucleotides and lack of protein translation capabilities (18). The lncRNA recruits transcription factors and chromatin modification complexes to specific genomic loci and regulates chromatin structure through steps such as histone modifications and DNA methylation. With the development of gene sequencing and bioinformatics technology, numerous lncRNAs associated with endocrine therapy resistance and cancer progression in breast cancer have been explored (19,20), such as, in breast cancer, HOTAIR has been proven to enhance downstream genes of the ER pathway and promote EMT and metastasis; LncRNA-ROR binds to miR205-5p to induce the EMT process, and UCA1 mediates tamoxifen resistance via HIF-1α and Wnt/β-catenin (9,21). Aberrant expression of lncRNA plays a very critical role in the immune-related biological pathways of tumors and contributes to mediating immune and cancer cell interactions; it has been widely recognized to predict the prognosis of several cancer types. including non-small cell lung cancer, renal clear cell carcinoma, and hepatocellular carcinoma (22-24). However, there has been less exploration of the roles played by lncRNAs and their effects on the TME in endocrine resistant BRCA. In this study, we assessed the ERIR-lncRNAs by integrating the Gene Expression Omnibus (GEO) dataset, The Cancer Genome Atlas (TCGA) dataset, ImmPort databases, and InnateDB databases, construct a prognostic signature for predicting endocrine therapeutic response and prognosis of BRCA. The assessment of the TME contributes to a better understanding of the underlying molecular mechanisms of endocrine resistant BRCA. We present the following article in accordance with the TRIPOD reporting checklist (available at https://atm.amegroups.com/article/view/10.21037/atm-22-6158/rc).
Methods
Datasets collection
The dataset GSE144378 from the GEO database (www.ncbi.nlm.nih.gov/geo) was used to screen for ER-lncRNAs. Transcriptome sequencing data (RNA-Seq), clinical information, and somatic mutation data of BRCA were downloaded from the TCGA database (https://portal.gdc.cancer.gov/) for the construction of prognostic signatures. The TCGA-BRCA data were randomly and equally divided into a training set and internal testing set. To validate the signature, 2 independent datasets, GSE9195 and GSE42568, which contain patients who have received endocrine therapy, were selected from the GEO database as external validation. The complete-case analysis approach was used to address the missing data. The study was conducted in accordance with the Declaration of Helsinki (as revised in 2013).
Identification of endocrine resistance and immune-related lncRNA
The differentially expressed lncRNA (DElncRNA) between endocrine resistance and sensitive groups were identified by the “limma” package, with the screening criteria set as |log2[fold change (FC)]| >1 and false discovery rate (FDR) <0.05, and results were visualized with the “pheatmap” package and “ggplot2” package (25). We selected 2 classical immunology databases ImmPort (https://www.immport.org/home) (26) and InnateDB (https://www.innatedbdb.com) (27) to obtain all immune-related genes and obtained the intersection set. Spearman correlation algorithm (R “psych” package, screening condition correlation >0.4, P<0.001) were used to further analyze lncRNA that were significantly correlated with immune-related genes. Based on immune-related genes and ERIR-lncRNAs, a co-expression network was constructed. Gene Ontology (GO) and Kyoto Encyclopedia of Genes and Genomes (KEGG) enrichment analyses were performed using the “ConensusClusterPlus” R package to further investigate the biological pathways that may be involved (adjusted P value <0.05) (28).
Construction and validation of ERIR-lncRNA prognostic signature
ERIR-lncRNAs with significant prognostic predictive value were identified by univariate Cox analysis using the “survival” R package, and lncRNAs with a P value <0.05 were eligible for further analysis. To avoid overfitting, we further performed the least absolute shrinkage and selection operator (LASSO) Cox regression (10,000 iterations) via the “glmnet” R package (29,30). The lncRNAs with independent prognostic predictive value were eventually included in the construction of the signature with the following formula (31): Risk score=∑(Exp(Xi)×Coef(Xi)). The expr (lncRNA) indicated the expression of lncRNA, Coef (lncRNA) was the regression coefficients of lncRNA. Patients were divided into a high-risk group and a low-risk group based on the median risk score. Principal component analysis (PCA) was performed using the “prcomp“ function of the “STATS“ R package to examine whether signatures could better distinguish risk status, the “Survminer” and “timeROC” R package were used to evaluate the predictive capability of the risk signatures (32). In addition, we performed univariate and multifactorial Cox regression to evaluate whether the ERIR-lncRNA signature could be an independent prognostic predictor for endocrine resistant BRCA. External validation of the ITIR-lncRNA signature was performed in 2 independent datasets (GSE9195, GSE42568) from the GEO database, to assess the performance of the signature in determining clinical outcomes.
Nomogram construction and verification
We aimed to develop a quantitative approach to predict the individual probability of a clinical event in endocrine resistant BRCA patients. Based on the risk score signature, we established a nomogram containing other independent prognostic factors, to predict the 1-, 3-, and 5-year overall survival (OS). The calibration plot which was calculated by the “calibrate” function was used to verify the ability of the nomogram for predicting the prognoses (33).
Immune infiltration analysis
To reveal the relationship between risk signature and tumor-infiltrating immune cells, we executed 7 algorithms, including Tumor Immune Estimation Resource (TIMER), Cell-type Identification by Estimating Relative Subsets of RNA Transcripts (CIBERSORT), CIBERSORT-ABS, quanTIseq, Microenvironment Cell Populations (MCP)-counter, XCELL, and Estimate the Proportion of Immune and Cancer cells (EPIC) (34), to calculate the immune infiltration values among the samples in the endocrine treated BRCA, using bubble plots to show the correlation coefficients between scores and immune cells. Subsequently, single sample gene set enrichment analysis (ssGSEA) from the “GSVA” R package was performed to quantitatively assess the infiltration abundance between the high-risk group and the low-risk group, comparing the relative proportions of tumor-infiltrating immune cells and the expression of immune checkpoint genes (ICG) between the two risk subgroups (35). The Estimation of Stromal and Immune cells in Malignant Tumor tissues (ESTIMATE) algorithm was used to calculate the immune score, stromal score, and estimate score according to the proportion of immune cells and stromal cells. Violin plots were plotted to demonstrate the differences in scores between the two groups (36). Enrichment analysis of the 3 ERIR-lncRNA that included in the signature were performed separately, to further analysis of possible regulatory mechanisms.
Correlation between tumor mutational burden and ERIR-lncRNA signature
Based on the somatic mutation data and clinical information of post-endocrine treated BRCA, Wwe calculated the tumor mutational burden (TMB) for each patient and compared the TMB between the high-risk and low-risk groups. Somatic mutation data in the 2 subgroups were visualized using the R package “maftools”. In parallel, we explored the impact of ERIR-lncRNA signature combined with TMB scores on patient survival probability.
Cell culture and siRNA transfection
Human breast cancer cell line MCF7 were obtained from the American Type Culture Collection (ATCC, Manassas, VA, USA), and cultured in Dulbecco’s modified Eagle’s medium (DMEM, 4.5 g/L glucose) containing 5% fetal bovine serum (FBS), 10 µg/mL insulin, 100 U/mL penicillin, and 100 µg/mL streptomycin. Stable tamoxifen-resistant breast cancer cell line MCF7-TAMR1 were purchased from EMD Millipore (Millipore, Burlington, MA, USA), and were maintained in phenol red-free DMEM/F12 supplemented with 1% fetal bovine serum, 6 ng/mL insulin, and 1 µM-4 hydroxytamoxifen (MCE, Monmouth Junction, NJ, USA). All cells were stored at 37 ℃ in 95% humid air and 5% CO2.
SChLPA1 siRNA and negative control siRNA were purchased from Sigma-Aldrich. The sequence was 5'-CCAAUGAUGAGGAGCGGGA-3'. The cells were incubated with either SChLPA1 siRNA or negative control siRNA using Lipofectamine 2000 Transfection Reagent (Invitrogen, Carlsbad, CA, USA). Cells were incubated at room temperature for 15 min and further analysis was performed after 48 h of cell culture.
Real- time quantitative PCR
Total RNA was extracted with TRIzol (Invitrogen, Carlsbad, CA, USA), Equal amounts of RNA were reversely transcribed to cDNA with the SuperScript Reverse Transcriptase Kit (Thermo Fisher Scientific, Waltham, MA, USA). Total cDNA was then amplified and analyzed by SYBR Green PCR Master Mix (Thermo Fisher Scientific) in a Fast Real-time PCR 7500 System (Applied Biosystems, Foster City, CA, USA). The standard 2−ΔΔCT method was applied in this study for calculation of relative expression difference in each group sample. The primer sequences were:
SChLPA1-F: 5'-GAATGGAATGACTGGGGAAGAAGTGC-3' and SChLPA1-R: 5'-TTCTTCAGGGAGGTGGTATCTGCATC-3'.
Cell Counting Kit‑8 (CCK‑8) assay
For CCK-8 assay, cells were transfected with SChLPA1 siRNA or negative control siRNA were seeded in 96 well plates at a density of 1×104 cells/well and incubated with 5 µmol/L tamoxifen for 0, 24, 48, and 72 h, cells were incubated in medium with 10% CCK-8 reagent for 2 h. Then the absorbance of each well was measured at the wavelength of 450 nm with a microplate reader (EnSpire 2300; PerkinElmer, Inc., USA).
Transwell assay
Transwell chamber was used to assess cell migration. A total of 1.5×105 cells were seeded onto the upper chamber, while 600 µL DMEM medium containing 15% fetal bovine serum were added into the lower chambers. After 18 h of incubation, the migratory cells on the lower surface were washed and stained by 0.1% crystal violet.
Statistical analyses
All statistical analyses were performed on R 4.2.0 (R Foundation for Statistical Computing, Vienna, Austria). For quantitative data, the Students t-test was used to estimate the statistical significance of normally distributed variables, and non-normally distributed variables were analyzed by Wilcoxon rank-sum test. When more than two groups were compared, Kruskal-Wallis test and one-way analysis of variance (ANOVA) were used as non-parametric and parametric analysis methods. Two-sided Fisher exact tests were used to analyze contingency tables; Spearman method was applied for correlation analysis between two continuous variables. A P value <0.05 was considered statistically significant in this study.
Results
Identification of ERIR- lncRNA
The flowchart of the study is shown in Figure 1. We identified 781 DElncRNA between the endocrine resistance and sensitivity groups from the GSE114378 dataset, including 403 up-regulated and 378 down-regulated lncRNA. The expression profiles of DElncRNA were visualized in the form of volcano map and heatmap (Figure 2A,2B). We obtained 1,793 and 2,188 immune-related genes from 2 classical immunology databases, ImmPort and InnateDB, respectively, and took the intersecting genes to finally obtain 449 genes (Figure 2C). Spearman correlation analysis was used to further identify 12 lncRNA significantly associated with immune genes among ER-lncRNAs, including WNT5A-AS1, MIR205HG, LINC00871, GATA2-AS1, ZNF436-AS1, SChLPA1, LINC01341, DNAJC9-AS1, VIPR1-AS1, AC108676.1, LINC01579, and RBM26-AS1. We constructed a Sankey plot and co-expression networks to demonstrate the correlation of ERIR-lncRNA with immune-related genes (Figure 2D,2E).
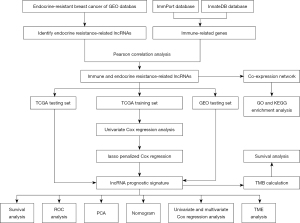
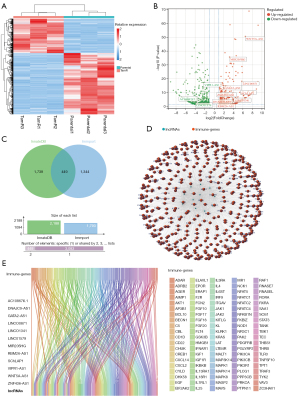
Construction of ERIR-lncRNA prognostic signature
Based on 12 ERIR-lncRNA, we constructed a prognostic signature to predict the prognosis and endocrine therapeutic response of ER+ BRCA. Univariate Cox regression and Lasso regression analysis were used to screen the ERIR-lncRNA that were associated with prognosis, finally 3 ERIR-lncRNA including LINC00871, WNT5A-AS1, and SChLPA1 were retained according to the optimum λ value (Figure 3A-3D). Figure 3E demonstrates the correlation between 3 hub lncRNAs and immune genes. Based on the coefficients of each lncRNA, the formula was calculated as follows: RiskScore = SChLPA1(exp) × 0.303337969303109 + WNT5A-AS1(exp) × −0.393865581304723 + LINC00871(exp) × 0.2595503464363. The median risk score was calculated to divide the entire cohort into low- and high-risk groups. To test whether ERIR-lncRNA signature could better distinguish risk status, PCA was performed and revealed that patients could be well separated (Figure 3F). The 3 lncRNAs enrolled in the signature predicted poor prognosis of breast cancer respectively (Figure 3G). The Kaplan-Meier curve demonstrated a notable shorter OS time and lower survival probability in the high-risk population (P<0.001, Figure 4A), predictive accuracy of the ERIR-lncRNA signature was assessed by the time-dependent receiver operating characteristic (ROC) curve, and showed that the area under the curve (AUC) values at 1, 3, and 5 years were 0.710, 0.649, and 0.672, respectively (Figure 4B). Compared with other clinical characteristics, the risk models also showed better predictive capability (Figure 4C). The risk score and survival status revealed that mortality was significantly associated with risk score (P<0.001, Figure 4D,4E). In endocrine resistant patients, risk scores were significantly higher than in non-resistant patients (P=0.01, Figure 4F). We performed univariate and multivariate Cox regression and the results showed that risk signature was an independent predictor of prognosis [hazard ratio (HR): 1.597, 95% confidence interval (CI): 1.185–2.154, P=0.002, Figure 4G,4H]. Validation was performed in the TCGA internal testing set, all results were highly consistent with the training cohort (Figure 5).
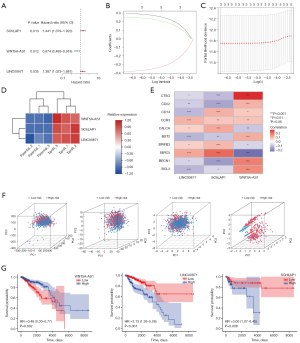
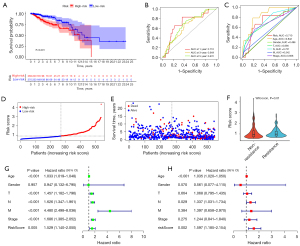
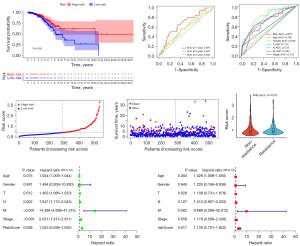
External validation of ERIR-lncRNA prognosis signature
We used 2 independent datasets, GSE9195 and GSE42568, from the GEO database to validate the reliability of the ERIR-lncRNA prognostic signature and classify patients into high-risk and low-risk groups based on the same score cutoff criteria as the training set. In GSE9195, patients with high-risk score had a significantly lower survival probability (P=0.009, Figure 6A), and the timeROC curve presented with the AUC values of the 1-, 3-, and 5-year ROC curve were 0.737, 0.594, and 0.514 (Figure 6B). The correlation between mortality and risk score was also revealed by risk score distribution and survival status (P<0.001, Figure 6C,6D). Endocrine resistant patients had higher risk scores than non-resistant patients (P=0.02, Figure 6E). Consistent results were also obtained in GSE42568, the low-risk group presented with longer OS time than the high-risk group (P=0.014, Figure 6F), the AUC values of the 1-, 2-, and 3-year timeROC curve were 0.777, 0.691, and 0.602, respectively (Figure 6G). The risk score distribution and survival status also showed that the high-risk group was associated with higher mortality (Figure 6H,6I) and was highly prevalent in endocrine resistant patients (Figure 6J). All of these findings indicated the accuracy of ERIR-lncRNA signature for prognostic prediction.
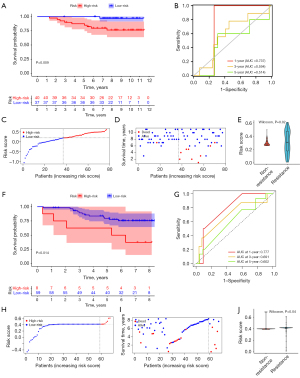
Construction and verification of nomogram
As shown in Figure 7, we adopted 7 independent OS prognostic features, including ERIR-lncRNA prognostic signature, T-stage, N-stage, M-stage, gender, age, and American Joint Committee on Cancer (AJCC) stage, to quantitatively estimate 1-, 3-, and 5-year survival probabilities for endocrine-treated BRCA (Figure 7A,7C). In the nomogram scoring system, each variable was assigned a point and the sum of the points is calculated as the total score, with the total score corresponding to the 1-, 3-, and 5-year predicted probability of survival. The accuracy and sensitivity of the nomogram was confirmed by the calibration plot, which showed that the prediction results were generally consistent with reality (Figure 7B,7D).
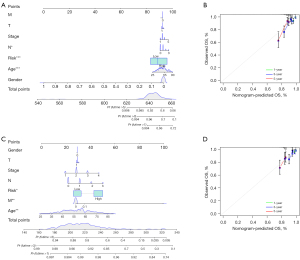
Analysis of immunogenomic landscape and potential biological processes
To further investigate the biological behavior of ERIR-lncRNA in endocrine resistant BRCA, we performed GO enrichment analysis and found that the cellular molecular composition and biological functions were mainly involved in T cell signaling and T follicular helper (Tfh) cell differentiation, such as “alpha-beta T cell receptor complex”, “negative regulation of T-helper 1 type immune response”, “positive regulation of T-helper 2 cell cytokine production”, and “MHC class II protein complex”, which also coincided with the results of KEGG signaling pathway enrichment (Figure 8A,8B). We next investigated the immune microenvironmental characteristics that may regulate tumorigenesis in the high-risk group; the 22 immune cell-related infiltrating score were calculated by 7 algorithms, including XCELL, TIMER, and quanTIseq, etc., and the correlation between risk scores and immune cell is shown in Figure 8C. With the increase of risk score, immune cells like CD8+ T cells, dendritic cells, macrophages, and neutrophils were decreased in endocrine-treated BRCA patients, whereas the risk score demonstrated a significantly positive correlation with Th1, Th2, and B cells. The ESTIMATE algorithm based on ssGSEA estimated the TME components of each sample and found that the stromal and ESTIMATE scores were higher in the high-risk group (P<0.001), with no significant differences in immune scores (Figure 8D). Analysis of immune cell subpopulations between the low and high-risk groups showed statistically differences in Tfh, neutrophils, and mast cells (Figure 8E), and the immune checkpoints with significant differences included NRP1, CD276, CD86, TNFSF4, CD80, VTCN1, HAVCR2, LAIR1, TNFSF15, and PDCD1LG2 (P<0.05, Figure 8F).
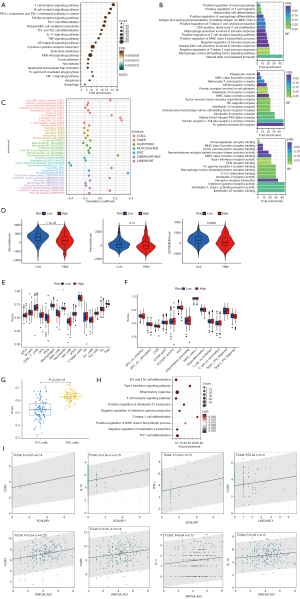
Moreover, in the high-risk group, a shift in the Th1/Th2 balance toward Th2 was found (Figure 8G), which was in accordance with the results annotated in Figure 8B for “negative regulation of T-helper 1 type immune response” and “positive regulation of T-helper 2 cell cytokine production”, that is well-known as a tumor-promoting phenomenon (37). Subsequently, the ERIR-lncRNA included in the prognostic signature were functionally annotated separately, and notably, a lncRNA named SChLPA1 was mainly involved in “Th1 and Th2 cell differentiation”, “T cell receptor signaling pathway”, and “T-helper 1 cell differentiation” (Figure 8H), and was positively associated with the Th1-specific markers of interferon-γ (IFN-γ) and tumor necrosis factor-α (TNF-α) and the Th2-specific markers of CCR3 and IL-10. The other 2 lncRNA correlations were also found with CCR3, CCR4, interleukin 4 (IL-4), and IL-10 (Figure 8I).
Correlation between TMB and ERIR-lncRNA signature
Theoretically, the higher accumulation of somatic mutations, the more neoantigens that can be recognized by T cells, in turn, patients get more benefit from immunotherapy. Considering that ERIR-lncRNA is highly enriched in chromatin remodeling and DNA repair, we investigated the TMB between the high- and low-risk groups to analyze the correlation between TMB and the ETIR-lncRNA signature. Based on the single nucleotide variant (SNV) data obtained from TCGA-BRCA, TMB values were calculated for each sample. In terms of somatic mutation, the high-risk group presented a higher mutational rate (52.6%) than the low-risk group (37.28%) (Figure 9A,9B), patients in the high-risk group showed a significantly higher TMB (Figure 9C). According to the Kaplan-Meier curve, combination of TMB and the ETIR-lncRNA signature helped to predict OS (Figure 9D); the signature might be an important predictive factor to indicate the immunotherapy response.
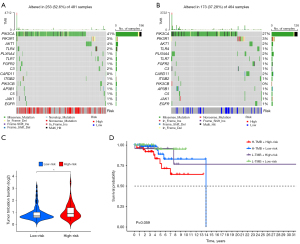
SChLPA1 is highly expressed in endocrine resistant BRCA cells and promotes cell growth and migration
Since the biological properties and functions of LINC0087 and WNT5A-AS1 have not been explored, there is a lack of relevant literature and research base in tumors. In contrast, SChLAP1 has been shown to be associated with poor prognosis in certain cancers.We first examined the expression levels of SChLPA1 in MCF-7 and MCF-7/R cell lines and found that SChLPA1 was significantly elevated in endocrine resistant breast cancer cells when compared to sensitive cells (Figure 10A).We then constructed a stable SChLPA1 knockdown MCF-7/R cell line (Figure 10B) and performed CCK8 assays, which showed that the silencing of SChLPA1 significantly inhibited cell growth (Figure 10C), and the transwell assay showed that migration of SChLPA1-silenced endocrine resistant cells was significantly reduced in comparison to control cells (Figure 10D).
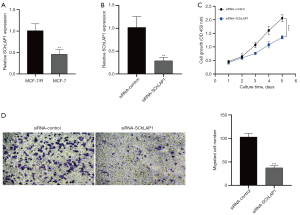
Discussion
Owing to the strong dependency of breast tumorigenesis on the estrogen-ER axis, estrogen suppression and ER antagonists have remained the mainstay of ER+ BRCA treatment for several decades, the high heterogeneity of BRCA makes endocrine therapy ineffective. Although a complex TME may support malignancy development and immune escape, at the same time, the interaction between tumors and infiltrating immune cells has important implications for the development of new therapeutic approaches. To date, the immune microenvironmental features of ER+ tumors have not been well investigated (12,38-40), but recent studies have suggested a non-negligible role of TME in endocrine resistant BRCA. Several components of TME, including hypoxia (41), cancer-associated fibroblasts (42), ECM (11), immune cells, and inflammatory cytokines such as IL-1β, transforming growth factor-β (TGF-β), and TNF-α (10,43) have been shown to be associated with endocrine resistance. Our team has also revealed some of the endocrine resistance mechanisms involving the immune microenvironment in previous studies such as that high SGLT1 expression mediates enhanced glucose uptake and lactic acid secretion, promoting M2-like tumor-associated macrophage (TAM) polarization and feedback activation of EGFR/PI3K/Akt/SGLT1 signaling in tumor cells to enhance tamoxifen resistance (44). Another article also proposed that TAMs increased the expression of cyclooxygenase-2 (COX-2)/prostaglandin E2 (PGE2), which promoted tamoxifen resistance (45). However, there is no definitive clinical evidence to confirm the predictive value of immune features for endocrine resistant BRCA; looking for biomarkers with long-term benefits to guide treatment is necessary. The lncRNA are widely expressed in human cells and closely related to immune-related pathways of tumor progression (46-48), have the possibility to be potential prognostic markers and therapeutic targets. Combined with the advancement of sequencing technology, it provides an excellent platform to explore the key molecules of endocrine resistance. Our team previously found that FOXO3A-induced LINC00926 suppressed breast tumor growth and metastasis through inhibition of PGK1-mediated Warburg effect (49). Another study identified lncRNA ST7-AS1 as a potential new biomarker and associated with immune infiltration in BRCA (50).
In this study, we screened ERIR-lncRNA based on transcript data, clinical information, and immune-related genes obtained from public databases, and functional annotation was performed by co-expression network and enrichment analysis. An ERIR-lncRNA prognostic signature including LINC00871, WNT5A-AS1, and SChLPA1 was constructed by univariate Cox regression and Lasso-Cox regression. Based on the median risk score, patients were divided into high- and low-risk groups. According to PCA, survival curves, ROC curves, univariate and multivariate Cox regression, it was concluded that the risk signature was an accurate and independent predictor. Subsequently, we constructed a nomogram which combined with clinical elements to predict survival more intuitively. To further explore the biological function of ERIR-lncRNA and seek potential mechanisms, we performed functional annotation and found that it is mainly enriched in T cell receptor signaling pathway and Th1 and Th2 cell differentiation. Th1 and Th2 not only showed a positive correlation with the risk score, but there was also a significant difference in Tfh infiltration between the high- and low-risk groups. Among them, SChLPA1 is involved in the Th1 and Th2 cell differentiation, negative regulation of IFN-γ production, and positive regulation of IL-10 production. In endocrine-treated BRCA patients, SChLPA1 was positively associated with the markers and secreted cytokines of T helper cells, such as IFN-γ and TNF-α of Th1, and CCR3 and IL-10 of Th2. The other lncRNA were also found to be differentially linked with Tfh, such as LINC00871 with CCR3, and WNT5A-AS1 with CCR5, CCR4, IL-4, and IL-10. Notably, the expression of Th2 was higher than Th1 in the high-risk group, this shift of Th1/Th2 balance toward th2 is known as a pro-tumor immune phenomenon (37) and corresponds to the results of the enrichment analysis described above.
The research progress of three lncRNAs in oncology is discussed separately. Among them, LINC0087 and WNT5A-AS1 are still functionally unknown and no tumor-related research has been reported. As for SChLPA1, which was initially found to be highly expressed in prostate cancer and could promote cancer progression by antagonizing the oncogenic function of the SWI/SNF complex, the SChLPA1 expression levels could independently predict poor patient outcomes (51). Subsequently, SChLPA1 induced poor prognostic in breast cancer, lung cancer and human glioblastoma were revealed individually. The active mechanism include, SChLPA1 promotes immune evasion of CD8+ T cells by NSCLC cells through regulation of PD-1/PD-L1; regulating malignant tumor behavior of breast cancer cells via miR-524-5p/HMGA2 axis; and activating NF-κB signaling pathway by forming a complex with HNRNPL to regulate the stability of ACTN4 in human glioblastoma (52-54). In this study, we confirmed that SChLPA1 was highly expressed in endocrine resistant breast cancer cells and promoted the growth and migration of tumor cells, combined with the enrichment analysis of SChLPA1, which was mainly involved in “Endocrine resistance”, “Breast cancer”, “cellular response to drug”, “PD-L1 expression and PD-1 checkpoint pathway in cancer”, etc. It is suggested that SChLPA1 induces poor prognosis in endocrine resistant breast cancer, but the exact mechanism still needs to be explored in subsequent experiments.
BRCA is considered to be an immunologically quiescent tumor, which greatly inhibits the efficacy of immunotherapy. However a growing body of evidence supports the immune infiltration of the TME as a determinant in predicting the prognosis of BRCA (55). Tumor growth and progression are associated with structural changes of immune cells in the TME, which support tumor development while modulating the function of neighboring immune effector cells, thereby impairing endogenous immune surveillance. Interaction of tumor cells with a large number of mesenchymal and intratumoral immune infiltrating cells may enhance or diminish immunotherapeutic or targeted treatment responses (56). As mentioned, the hub lncRNA incorporated into the risk signature is closely related to T helper cells and appears to induce an immunosuppressive, tumor-promoting microenvironmental phenotype (37,57). It is well-known that Thf are essential regulators in tumor immunity, Th1 cells have strong anti-tumor properties by increasing the activities of NK cells and CD8+ cells, inducing STAT1 and STAT4 activation by secreted IFN-γ, which positively feeds back to promote Th1 differentiation and inhibit Th2 (58,59). In contrast, Th2 promotes tumor progression over time, and secreted IL-4 positively regulates Th2 differentiation while suppressing Th1 (60,61). Considering the special significance of Tfh differentiation and Th1/Th2 balance, it is commonly considered as an indicator of the BRCA patient's immune status. Functional analysis of ERIR-lncRNA showed that the nuances of CD4+ Th cell regulation and the balance between anti-tumor and pro-tumor subtypes may be critical in exploring the relationship between endocrine resistant BRCA and TME. It has been proposed that the interaction between ECM and Thf subtypes creates a balanced TME that can affect the prognosis and therapeutic response of BRCA, the IFN-γ secreted by Th1 cells may be responsible for ECM remodeling and regulated by Th2 (11,62). The expression levels of type I IFNs correlate negatively with clinical outcome but positively with tumor grade in patients with ER-positive BRCA (63). Emerging evidence points to a critical role of IFN signaling, IFNs upregulate survival factors such as G1P3, which promote tumor cell survival and lead to poor prognosis in ER+ BRCA (64), which also induces an IFN-related DNA damage-resistant signature to generate treatment tolerance (65). In our risk signature, SChLPA1 is enriched in the type I IFN signaling pathway and can positively regulate IFN-β and IFN-α production. SChLPA1 was first identified as an independent predictor of metastasis and specific death in prostate cancer, and recently it has been reported that SChLPA1 is also found to be highly expressed in BRCA, which can be used as a potential biomarker for diagnosis, SChLPA1 regulates the poor prognosis of ER+ BRCA and may be associated with Thf balance based on IFN-related pathways. In addition, Thf regulate cancer-associated fibroblast (CAF)-induced collagen synthesis through inflammatory factors, and in turn, CAF secrete cytokines and chemokines to regulate Thf’s activation, this process not only affects T cell differentiation, but also influences the spatial distribution of T cells to regulate antitumor immunity (42,66,67). Other researchers have also found that the serum sST2 is significantly associated with poor prognosis in ER+ BRCA, whereas ST2 is widely expressed in Th2 cells and binding to the ligand expressed by CD4+ T cells to trigger their differentiation to the Th2 phenotype (68,69). A study further proposed that in BRCA, saikosaponin A (SSa) and its derivatives increase the T cell penetration of the TME and promote the Th1/Th2 balance towards Th1 through the activation of the IL-12/STAT4 pathway (70). There may be benefit from combining endocrine therapy with SSa. From the foregoing we can see that Thf has important implications in BRCA recurrence and drug resistance, the underlying mechanism of ERIR-lncRNA prognosis signature remains to be further investigated.
In addition, we further explored the correlation between ERIR-lncRNA risk score and TMB. It is currently believed that TMB can predict tumor immunotherapy efficacy, and the tumor-specific neoantigens generated by somatic mutations are presented to T cells by major histocompatibility complex (MHC), which stimulate the immune system to recognize and attack cancer cells. Theoretically, the higher the accumulated somatic mutations, the more neoantigens that can be recognized by T cells, and patients can gain more benefit from immunotherapy (71,72). Compared to immunogenic tumors, breast cancer had intermediate TMB values, and triple-negative breast cancer (TNBC) had a higher TMB than ER(+) or HER2(+) cancer (73). High TMB is associated with prolonged survival in the treatment of ICIs and also has good predictive power for clinical outcomes in breast cancer patients treated with neoadjuvant chemotherapy, targeted therapy, or standard chemotherapy. The use of TMB as a prognostic biomarker helps to evaluate a greater number of breast cancer patients who may benefit from ICIs, ICIs combined with chemotherapy or targeted therapy (74). We found that the ERIR-lncRNA signature was significantly associated with TMB, with patients in high-risk group having higher TMB than the low-risk group, revealing a potential link between endocrine-resistant BRCA and immunotherapy. Combination therapy may be beneficial in improving patient survival, reiterating the importance of the immune microenvironment in endocrine resistant patients. Our study still needs to be further validated in prospective studies, complementing the basic experiments to further reveal the potential mechanisms by which ERIR-lncRNA affects the prognosis of BRCA.
At the current stage, the clinical translation of lncRNA-based therapies has been hampered. The main issues are related to specificity, deliverability and tolerability (75). The reasons for poor specificity include off-target effects due to excessive similar sequences or uptake by non-target cells, in addition some lncRNAs are degraded once they enter the circulation, which is usually associated with higher RNAse activity in the blood of cancer patients. Delivery difficulties are mainly due to the instability of lncRNA structure and the lack of suitable delivery vectors. The tolerance problem is the recognition of the RNA structure by natural immune cells resulting in adverse immune effects. Taken together, lncRNA therapy is still facing many challenges and requires multidisciplinary cooperation.
Conclusions
In summary, we identified an effective and stable prognostic signature based on ERIR-lncRNA in BRCA, and analyzed the potential functional mechanisms that may be associated with tumor immune infiltration. As an independent biomarker and predictor of therapeutic response, the ERIR-lncRNA prognostic signature may become a new target against endocrine resistance and provide fresh thoughts for clinical treatment.
Acknowledgments
Funding: This work was supported by the National Natural Science Foundation of China (Nos. 81730074, 81672599, and 82002781); China Postdoctoral Science Foundation (No. 2018M641858); Hei Long Jiang Postdoctoral Foundation (No. LBH-Z18115); Knowledge Innovation Program of Harbin Medical University (No. 31041180112); and The National Science Foundation of Heilongjiang Province of China for Returnees (No. LC2017037).
Footnote
Reporting Checklist: The authors have completed the TRIPOD reporting checklist. Available at https://atm.amegroups.com/article/view/10.21037/atm-22-6158/rc
Conflicts of Interest: All authors have completed the ICMJE uniform disclosure form (available at https://atm.amegroups.com/article/view/10.21037/atm-22-6158/coif). The authors have no conflicts of interest to declare.
Ethical Statement:
Open Access Statement: This is an Open Access article distributed in accordance with the Creative Commons Attribution-NonCommercial-NoDerivs 4.0 International License (CC BY-NC-ND 4.0), which permits the non-commercial replication and distribution of the article with the strict proviso that no changes or edits are made and the original work is properly cited (including links to both the formal publication through the relevant DOI and the license). See: https://creativecommons.org/licenses/by-nc-nd/4.0/.
References
- Haque R, Ahmed SA, Inzhakova G, et al. Impact of breast cancer subtypes and treatment on survival: an analysis spanning two decades. Cancer Epidemiol Biomarkers Prev 2012;21:1848-55. [Crossref] [PubMed]
- DeSantis CE, Ma J, Gaudet MM, et al. Breast cancer statistics, 2019. CA Cancer J Clin 2019;69:438-51. [Crossref] [PubMed]
- Szostakowska M, Trębińska-Stryjewska A, Grzybowska EA, et al. Resistance to endocrine therapy in breast cancer: molecular mechanisms and future goals. Breast Cancer Res Treat 2019;173:489-97. [Crossref] [PubMed]
- Bray F, Ferlay J, Soerjomataram I, et al. Global cancer statistics 2018: GLOBOCAN estimates of incidence and mortality worldwide for 36 cancers in 185 countries. CA Cancer J Clin 2018;68:394-424. [Crossref] [PubMed]
- Angus L, Smid M, Wilting SM, et al. The genomic landscape of metastatic breast cancer highlights changes in mutation and signature frequencies. Nat Genet 2019;51:1450-8. [Crossref] [PubMed]
- Bertucci F, Ng CKY, Patsouris A, et al. Genomic characterization of metastatic breast cancers. Nature 2019;569:560-4. [Crossref] [PubMed]
- Hinohara K, Wu HJ. KDM5 Histone Demethylase Activity Links Cellular Transcriptomic Heterogeneity to Therapeutic Resistance. Cancer Cell 2019;35:330-2. [Crossref] [PubMed]
- Patten DK, Corleone G, Győrffy B, et al. Enhancer mapping uncovers phenotypic heterogeneity and evolution in patients with luminal breast cancer. Nat Med 2018;24:1469-80. [Crossref] [PubMed]
- Nagini S. Breast Cancer: Current Molecular Therapeutic Targets and New Players. Anticancer Agents Med Chem 2017;17:152-63. [Crossref] [PubMed]
- Stender JD, Nwachukwu JC, Kastrati I, et al. Structural and Molecular Mechanisms of Cytokine-Mediated Endocrine Resistance in Human Breast Cancer Cells. Mol Cell 2017;65:1122-1135.e5. [Crossref] [PubMed]
- Jallow F, O'Leary KA, Rugowski DE, et al. Dynamic interactions between the extracellular matrix and estrogen activity in progression of ER+ breast cancer. Oncogene 2019;38:6913-25. [Crossref] [PubMed]
- Loi S, Sirtaine N, Piette F, et al. Prognostic and predictive value of tumor-infiltrating lymphocytes in a phase III randomized adjuvant breast cancer trial in node-positive breast cancer comparing the addition of docetaxel to doxorubicin with doxorubicin-based chemotherapy: BIG 02-98. J Clin Oncol 2013;31:860-7. [Crossref] [PubMed]
- Heindl A, Sestak I, Naidoo K, et al. Relevance of Spatial Heterogeneity of Immune Infiltration for Predicting Risk of Recurrence After Endocrine Therapy of ER+ Breast Cancer. J Natl Cancer Inst 2018; [Crossref]
- Bense RD, Sotiriou C, Piccart-Gebhart MJ, et al. Relevance of Tumor-Infiltrating Immune Cell Composition and Functionality for Disease Outcome in Breast Cancer. J Natl Cancer Inst 2016;109:djw192. [Crossref] [PubMed]
- Mehta AK, Kadel S, Townsend MG, et al. Macrophage Biology and Mechanisms of Immune Suppression in Breast Cancer. Front Immunol 2021;12:643771. [Crossref] [PubMed]
- Frazao A, Messaoudene M, Nunez N, et al. CD16(+)NKG2A(high) Natural Killer Cells Infiltrate Breast Cancer-Draining Lymph Nodes. Cancer Immunol Res 2019;7:208-18. [Crossref] [PubMed]
- Kajitani K, Tanaka Y, Arihiro K, et al. Mechanistic analysis of the antitumor efficacy of human natural killer cells against breast cancer cells. Breast Cancer Res Treat 2012;134:139-55. [Crossref] [PubMed]
- Li Y, Li L, Wang Z, et al. LncMAP: Pan-cancer atlas of long noncoding RNA-mediated transcriptional network perturbations. Nucleic Acids Res 2018;46:1113-23. [Crossref] [PubMed]
- Eptaminitaki GC, Wolff N, Stellas D, et al. Long Non-Coding RNAs (lncRNAs) in Response and Resistance to Cancer Immunosurveillance and Immunotherapy. Cells 2021;10:3313. [Crossref] [PubMed]
- Bao S, Zhao H, Yuan J, et al. Computational identification of mutator-derived lncRNA signatures of genome instability for improving the clinical outcome of cancers: a case study in breast cancer. Brief Bioinform 2020;21:1742-55. [Crossref] [PubMed]
- Chen WT, Wu HT, Shen JX, et al. Long non-coding RNAs engender drug resistance to different subtypes of treatments of breast cancers and may provide a "next generation" therapy option. Discov Med 2020;29:27-39.
- Sun J, Zhang Z, Bao S, et al. Identification of tumor immune infiltration-associated lncRNAs for improving prognosis and immunotherapy response of patients with non-small cell lung cancer. J Immunother Cancer 2020;8:e000110. [Crossref] [PubMed]
- Khadirnaikar S, Kumar P, Pandi SN, et al. Immune associated LncRNAs identify novel prognostic subtypes of renal clear cell carcinoma. Mol Carcinog 2019;58:544-53. [Crossref] [PubMed]
- Hong W, Liang L, Gu Y, et al. Immune-Related lncRNA to Construct Novel Signature and Predict the Immune Landscape of Human Hepatocellular Carcinoma. Mol Ther Nucleic Acids 2020;22:937-47. [Crossref] [PubMed]
- Li M, Liang M, Lan T, et al. Four Immune-Related Long Non-coding RNAs for Prognosis Prediction in Patients With Hepatocellular Carcinoma. Front Mol Biosci 2020;7:566491. [Crossref] [PubMed]
- Zalocusky KA, Kan MJ, Hu Z, et al. The 10,000 Immunomes Project: Building a Resource for Human Immunology. Cell Rep 2018;25:513-522.e3. [Crossref] [PubMed]
- Breuer K, Foroushani AK, Laird MR, et al. InnateDB: systems biology of innate immunity and beyond--recent updates and continuing curation. Nucleic Acids Res 2013;41:D1228-33. [Crossref] [PubMed]
- Yu G, Wang LG, Han Y, et al. clusterProfiler: an R package for comparing biological themes among gene clusters. OMICS 2012;16:284-7. [Crossref] [PubMed]
- Gupta S, Lee REC, Faeder JR. Parallel Tempering with Lasso for model reduction in systems biology. PLoS Comput Biol 2020;16:e1007669. [Crossref] [PubMed]
- Song C, Guo Z, Yu D, et al. A Prognostic Nomogram Combining Immune-Related Gene Signature and Clinical Factors Predicts Survival in Patients With Lung Adenocarcinoma. Front Oncol 2020;10:1300. [Crossref] [PubMed]
- Meng T, Huang R, Zeng Z, et al. Identification of Prognostic and Metastatic Alternative Splicing Signatures in Kidney Renal Clear Cell Carcinoma. Front Bioeng Biotechnol 2019;7:270. [Crossref] [PubMed]
- Du X, Zhang Y. Integrated Analysis of Immunity- and Ferroptosis-Related Biomarker Signatures to Improve the Prognosis Prediction of Hepatocellular Carcinoma. Front Genet 2020;11:614888. [Crossref] [PubMed]
- Iasonos A, Schrag D, Raj GV, et al. How to build and interpret a nomogram for cancer prognosis. J Clin Oncol 2008;26:1364-70. [Crossref] [PubMed]
- Wang Z, Wang Y, Peng M, et al. UBASH3B Is a Novel Prognostic Biomarker and Correlated With Immune Infiltrates in Prostate Cancer. Front Oncol 2019;9:1517. [Crossref] [PubMed]
- Newman AM, Steen CB, Liu CL, et al. Determining cell type abundance and expression from bulk tissues with digital cytometry. Nat Biotechnol 2019;37:773-82. [Crossref] [PubMed]
- Yoshihara K, Shahmoradgoli M, Martínez E, et al. Inferring tumour purity and stromal and immune cell admixture from expression data. Nat Commun 2013;4:2612. [Crossref] [PubMed]
- Basu A, Ramamoorthi G, Albert G, et al. Differentiation and Regulation of T(H) Cells: A Balancing Act for Cancer Immunotherapy. Front Immunol 2021;12:669474. [Crossref] [PubMed]
- Schmid P, Rugo HS, Adams S, et al. Atezolizumab plus nab-paclitaxel as first-line treatment for unresectable, locally advanced or metastatic triple-negative breast cancer (IMpassion130): updated efficacy results from a randomised, double-blind, placebo-controlled, phase 3 trial. Lancet Oncol 2020;21:44-59. [Crossref] [PubMed]
- Schmid P, Cortes J, Pusztai L, et al. Pembrolizumab for Early Triple-Negative Breast Cancer. N Engl J Med 2020;382:810-21. [Crossref] [PubMed]
- Schalper KA, Velcheti V, Carvajal D, et al. In situ tumor PD-L1 mRNA expression is associated with increased TILs and better outcome in breast carcinomas. Clin Cancer Res 2014;20:2773-82. [Crossref] [PubMed]
- Wang J, Wu H, Zhao Q, et al. Aggregation-Induced Emission Photosensitizer Synergizes Photodynamic Therapy and the Inhibition of the NF-κB Signaling Pathway to Overcome Hypoxia in Breast Cancer. ACS Appl Mater Interfaces 2022;14:29613-25. [Crossref] [PubMed]
- Brechbuhl HM, Finlay-Schultz J, Yamamoto TM, et al. Fibroblast Subtypes Regulate Responsiveness of Luminal Breast Cancer to Estrogen. Clin Cancer Res 2017;23:1710-21. [Crossref] [PubMed]
- Joffroy CM, Buck MB, Stope MB, et al. Antiestrogens induce transforming growth factor beta-mediated immunosuppression in breast cancer. Cancer Res 2010;70:1314-22. [Crossref] [PubMed]
- Niu X, Ma J, Li J, et al. Sodium/glucose cotransporter 1-dependent metabolic alterations induce tamoxifen resistance in breast cancer by promoting macrophage M2 polarization. Cell Death Dis 2021;12:509. [Crossref] [PubMed]
- Qin Q, Ji H, Li D, et al. Tumor-associated macrophages increase COX-2 expression promoting endocrine resistance in breast cancer via the PI3K/Akt/mTOR pathway. Neoplasma 2021;68:938-46. [Crossref] [PubMed]
- Turner M, Galloway A, Vigorito E. Noncoding RNA and its associated proteins as regulatory elements of the immune system. Nat Immunol 2014;15:484-91. [Crossref] [PubMed]
- Chen YG, Satpathy AT, Chang HY. Gene regulation in the immune system by long noncoding RNAs. Nat Immunol 2017;18:962-72. [Crossref] [PubMed]
- Atianand MK, Caffrey DR, Fitzgerald KA. Immunobiology of Long Noncoding RNAs. Annu Rev Immunol 2017;35:177-98. [Crossref] [PubMed]
- Chu Z, Huo N, Zhu X, et al. FOXO3A-induced LINC00926 suppresses breast tumor growth and metastasis through inhibition of PGK1-mediated Warburg effect. Mol Ther 2021;29:2737-53. [Crossref] [PubMed]
- Zhang Z, Zhang H, Li D, et al. LncRNA ST7-AS1 is a Potential Novel Biomarker and Correlated With Immune Infiltrates for Breast Cancer. Front Mol Biosci 2021;8:604261. [Crossref] [PubMed]
- Raab JR, Smith KN, Spear CC, et al. SWI/SNF remains localized to chromatin in the presence of SCHLAP1. Nat Genet 2019;51:26-9. [Crossref] [PubMed]
- Du Z, Niu S, Wang J, et al. SChLAP1 contributes to non-small cell lung cancer cell progression and immune evasion through regulating the AUF1/PD-L1 axis. Autoimmunity 2021;54:225-33. [Crossref] [PubMed]
- Bai X, Zhang S, Qiao J, et al. Long non-coding RNA SChLAP1 regulates the proliferation of triple negative breast cancer cells via the miR-524-5p/HMGA2 axis. Mol Med Rep 2021;23:446. [Crossref] [PubMed]
- Ji J, Xu R, Ding K, et al. Long Noncoding RNA SChLAP1 Forms a Growth-Promoting Complex with HNRNPL in Human Glioblastoma through Stabilization of ACTN4 and Activation of NF-κB Signaling. Clin Cancer Res 2019;25:6868-81. [Crossref] [PubMed]
- Baxevanis CN, Fortis SP, Perez SA. The balance between breast cancer and the immune system: Challenges for prognosis and clinical benefit from immunotherapies. Semin Cancer Biol 2021;72:76-89. [Crossref] [PubMed]
- Sautès-Fridman C, Petitprez F, Calderaro J, et al. Tertiary lymphoid structures in the era of cancer immunotherapy. Nat Rev Cancer 2019;19:307-25. [Crossref] [PubMed]
- Yang MW, Tao LY, Jiang YS, et al. Perineural Invasion Reprograms the Immune Microenvironment through Cholinergic Signaling in Pancreatic Ductal Adenocarcinoma. Cancer Res 2020;80:1991-2003. [Crossref] [PubMed]
- Saravia J, Chapman NM, Chi H. Helper T cell differentiation. Cell Mol Immunol 2019;16:634-43. [Crossref] [PubMed]
- Zhu J. T Helper Cell Differentiation, Heterogeneity, and Plasticity. Cold Spring Harb Perspect Biol 2018;10:a030338. [Crossref] [PubMed]
- Zhu J. T helper 2 (Th2) cell differentiation, type 2 innate lymphoid cell (ILC2) development and regulation of interleukin-4 (IL-4) and IL-13 production. Cytokine 2015;75:14-24. [Crossref] [PubMed]
- Acuner-Ozbabacan ES, Engin BH, Guven-Maiorov E, et al. The structural network of Interleukin-10 and its implications in inflammation and cancer. BMC Genomics 2014;15:S2. [Crossref] [PubMed]
- Zhou Y, Tian Q, Gao H, et al. Immunity and Extracellular Matrix Characteristics of Breast Cancer Subtypes Based on Identification by T Helper Cells Profiling. Front Immunol 2022;13:859581. [Crossref] [PubMed]
- Ka NL, Lim GY, Kim SS, et al. Type I IFN stimulates IFI16-mediated aromatase expression in adipocytes that promotes E(2)-dependent growth of ER-positive breast cancer. Cell Mol Life Sci 2022;79:306. [Crossref] [PubMed]
- Cheriyath V, Kuhns MA, Jacobs BS, et al. G1P3, an interferon- and estrogen-induced survival protein contributes to hyperplasia, tamoxifen resistance and poor outcomes in breast cancer. Oncogene 2012;31:2222-36. [Crossref] [PubMed]
- Weichselbaum RR, Ishwaran H, Yoon T, et al. An interferon-related gene signature for DNA damage resistance is a predictive marker for chemotherapy and radiation for breast cancer. Proc Natl Acad Sci U S A 2008;105:18490-5. [Crossref] [PubMed]
- Soon PS, Kim E, Pon CK, et al. Breast cancer-associated fibroblasts induce epithelial-to-mesenchymal transition in breast cancer cells. Endocr Relat Cancer 2013;20:1-12. [Crossref] [PubMed]
- Houthuijzen JM, Jonkers J. Cancer-associated fibroblasts as key regulators of the breast cancer tumor microenvironment. Cancer Metastasis Rev 2018;37:577-97. [Crossref] [PubMed]
- Lu DP, Zhou XY, Yao LT, et al. Serum soluble ST2 is associated with ER-positive breast cancer. BMC Cancer 2014;14:198. [Crossref] [PubMed]
- Choi MR, Sosman JA, Zhang B. The Janus Face of IL-33 Signaling in Tumor Development and Immune Escape. Cancers (Basel) 2021;13:3281. [Crossref] [PubMed]
- Zhao X, Liu J, Ge S, et al. Saikosaponin A Inhibits Breast Cancer by Regulating Th1/Th2 Balance. Front Pharmacol 2019;10:624. [Crossref] [PubMed]
- Chan TA, Yarchoan M, Jaffee E, et al. Development of tumor mutation burden as an immunotherapy biomarker: utility for the oncology clinic. Ann Oncol 2019;30:44-56. [Crossref] [PubMed]
- Anagnostou V, Bardelli A, Chan TA, et al. The status of tumor mutational burden and immunotherapy. Nat Cancer 2022;3:652-6. [Crossref] [PubMed]
- Barroso-Sousa R, Jain E, Cohen O, et al. Prevalence and mutational determinants of high tumor mutation burden in breast cancer. Ann Oncol 2020;31:387-94. [Crossref] [PubMed]
- Park SE, Park K, Lee E, et al. Clinical implication of tumor mutational burden in patients with HER2-positive refractory metastatic breast cancer. Oncoimmunology 2018;7:e1466768. [Crossref] [PubMed]
- Winkle M, El-Daly SM, Fabbri M, et al. Noncoding RNA therapeutics - challenges and potential solutions. Nat Rev Drug Discov 2021;20:629-51. [Crossref] [PubMed]
(English Language Editor J. Jones)