The potential of glutamine metabolism-related long non-coding RNAs (lncRNAs) as prognostic biomarkers in multiple myeloma patients
Highlight box
Key finding
• Classifier of multiple myeloma and lncRNA risk model based on glutamine metabolism maybe useful for accurately predicting prognosis for multiple myeloma.
What is known and what is new?
• Glutamine metabolism has become a hot topic to improve cancer treatment.
• Gln-associated lncRNAs signature was useful for predicting prognosis for MM.
What is the implication, and what should change now?
• Classifier of multiple myeloma and lncRNA risk model based on glutamine metabolism may be used for clinical application in multiple myeloma to help clinicians develop personalized treatment.
Introduction
Multiple myeloma (MM) is a rare hematological malignancy, which accounts for about 10% of blood cancer (1). The typical characteristics of MM are the infiltration of abnormal clonal plasma cells in bone marrow and the excessive production of monoclonal protein that can lead to multiple clinical symptoms including hypercalcemia, renal insufficiency, anemia, and bone lesions (1). MM occurs more frequently in the elderly (aged 60 to 70 years) compared with the young (2). Males have a higher incidence rate than females, with age-standardized rates of 2.2% and 1.5%, respectively, according to 2020 global cancer statistics (3). With the development of autologous stem cell transplantation (ASCT) and chemotherapy, the median overall survival (OS) of MM patients has reached 6 years (4).
However, before or after ASCT administration, a subgroup of exhausted/senescent cluster of differentiation (CD)8(+) T cells has been observed to cause T cell exhaustion and immune escape by expressing elevated immune checkpoints such as programmed cell death protein 1 (PD-1), cytotoxic T-lymphocyte-associated protein 4 (CTLA-4), 2B4, and CD160 (5,6). In this case, anti-immune checkpoint therapy is encouraged to rescue the anti-tumor response (7). Various preclinical and clinical studies of immune checkpoint inhibition on MM patients have revealed favorable outcomes. Pembrolizumab is a PD-1 inhibitor approved by the American Food and Drug Administration (FDA), and 7 of 23 (31%) MM patients receiving pembrolizumab after ASCT show a complete response in (8). In high-risk or recurrent MM patients after ASCT, ipilimumab in combination with nivolumab increases the progression-free survival (PFS) with 18 months follow-up (9). Reprogramming of glutamine (Gln) metabolism in tumors modulates immune escape by regulating tumor PD1 ligand (PD-L1) expression. Similarly, the reprogramming of glutamine metabolism in immune cells also affects their immune function (10). However, it is still a challenging task to increase the accuracy and response rate of immunotherapy in MM patients.
Gln is an important amino acid, especially under catabolic stressed conditions. Previous research has illustrated that deprivation of Gln results in rapid necrosis and dying of cancer cells (11). Glu metabolism is an alternative source in the tricarboxylic acid (TCA) cycle in cancer cells, which can support fatty acid synthesis through reductive carboxylation (12). Lines of evidence have demonstrated that reductive carboxylation can promote lipid synthesis and regulate the expression levels of reactive oxygen species (ROS) benefiting cancer cell growth (13-15). The activated Gln metabolism is associated with cancer progression and Gln metabolism has become a therapeutic target in anti-cancer therapy (16). It has also been reported that human myeloma cell lines (HMCLs) are highly sensitive to Gln depletion and MM cells are also addictive to Gln (17). There are studied reported that MM cells are highly reliant on glutamine metabolism (18,19). It was found that continued cell survival in the absence of glutamine maintained the expression of myeloid leukemia factor 1, but importantly induced the expression of pro-apoptotic BIM expression (20). However, cancer metabolic reprogramming makes the requirement of Gln heterogenous. Some lncRNAs have been shown to play an important role in the progression of MM and can be used as an indicator of patient prognosis. Upregulation of MALAT1 was significantly associated with poor prognosis of MM, including overall survival (OS) and progression-free survival (PFS) (21). Upregulation of 3 pseudogene 1 (PDIA3P) (22), H19 (23), colon-associated transcript 1 (CCAT1) (24), and colorectal neoplasia differential expression (CRNDE) were closely associated with MM survival outcome.
To further understand the role of Gln and explore the heterogeneity of Gln metabolism in MM patients, this study focused on Gln metabolism-related long non-coding RNAs (lncRNAs). Various lncRNAs have been identified in Gln metabolism, such as XLOC_006390 (25), TUG1 (26), and GIRGL (27). In this study, we identified Gln phenotype-based molecular subtypes through consensus clustering, and assessed the association of Gln metabolism with prognosis, immune microenvironment, and biological pathways. Moreover, we established a Gln-associated risk model for distinguishing high-risk MM patients. We present the following article in accordance with the TRIPOD reporting checklist (available at https://atm.amegroups.com/article/view/10.21037/atm-22-6190/rc).
Methods
Data acquisition and processing of MM samples
The gene expression files and survival information of MM samples were obtained from the Gene Expression Omnibus (GEO) database, including the GSE4581 (https://www.ncbi.nlm.nih.gov/geo/query/acc.cgi?acc=GSE4581) and GSE57317 (https://www.ncbi.nlm.nih.gov/geo/query/acc.cgi?acc=GSE57317) datasets. The gene transfer format (GTF) file and sequencing data of human reference genome (release 41 [GRCh38.p13]) were downloaded from GENCODE (https://www.gencodegenes.org/human/). Seqmap software (28) was used to map the sequence of probes to the human reference genome (mismatch =0). The probes matching to multiple gene symbols were removed. The averaged expression level was selected when multiple probes matched to one gene symbol. Then, the expression profiles of lncRNA and messenger RNA (mRNA) were extracted. For clinical data, samples without survival time and survival status were excluded. After processing, there were 413 and 55 samples in the GSE4581 and GSE57317 datasets respectively. The study was conducted in accordance with the Declaration of Helsinki (as revised in 2013).
Screening of Gln-associated lncRNAs
Gln metabolism pathway (GOBP GLUTAMINE FAMILY AMINO ACID METABOLIC PROCESS) was downloaded from Molecular Signature Database (MSigDB) (29). Single-sample gene set enrichment analysis (ssGSEA) was performed through GSVA R package (30) to calculate the enrichment score of Gln metabolism pathway. Spearman correlation analysis was performed through Hmisc R package to evaluate the relation between Gln metabolism score and lncRNA expression. The Gln-associated lncRNAs were screened under P<0.05 and |R| >0.25.
Molecular subtyping of MM samples
We used ConsensusClusterPlus R package (31) to cluster MM samples in the GSE4581 dataset based on the expression data of Gln-associated lncRNAs. The “hc” algorithm Spearman distance was used. Some 500 bootstraps were conducted with each bootstrap having 80% samples. Cluster number k was selected from 2 to 10. Cumulative distribution function (CDF) and consensus matrix were used to confirm the optimal cluster number. The final cluster number was the number of molecular subtypes.
Establishment of a risk model related to Gln phenotype
Firstly, limma R package (32) was employed to identify differentially expressed lncRNAs (DElncRNAs) in different molecular subtypes [|log2 (fold change (FC)| >log2(1.5) and false discovery rate (FDR) <0.05]. Univariate Cox regression analysis was conducted to screen DElncRNAs significantly associated with MM prognosis (P<0.05). Then, least absolute shrinkage and selection operator (LASSO) regression (33) and stepwise Akaike information criterion (stepAIC) (34) were used to decrease the number of DElncRNAs and construct a risk model. Multivariate Cox regression analysis was performed to calculate the coefficients of lncRNAs in the model. Finally, the risk model was constructed as follows: risk score = Σβi×Expi, where β represented coefficients, Exp represented the expression levels of lncRNAs and i represented lncRNAs.
Validation of the risk model
GSE4581 was used as the training dataset and GSE57317 was used as the validation dataset. Risk score was calculated for each sample and was converted to z-score. Z-score =0 was set as a cut-off to divide sample into high-risk (z-score >0) and low-risk (z-score <0) groups. Kaplan-Meier survival analysis was used to delineate survival curve of 2 risk groups. Receiver operating characteristic (ROC) curve analysis (35) was implemented to assess the performance of the risk model in predicting the prognosis of MM.
Functional and immune analysis
Hallmark pathways (h.all.v7.5.1.symbols.gmt) and Kyoto Encyclopedia of Genes and Genomes (KEGG) pathways (c2.cp.kegg.v7.5.1.symbols.gmt) were downloaded from MSigDB. ClusterProfiler R package (36) was utilized to annotate the enriched hallmark pathways in different molecular subtypes (adjusted P<0.05). SsGSEA was conducted to calculate the enrichment score of KEGG pathways. Spearman correlation analysis was performed to screen KEGG pathways significantly associated with risk score under |R| >0.2 and P<0.05.
The Estimation of Stromal and Immune cells in Malignant Tumor tissues using Expression data (ESTIMATE) algorithm (37) was used to evaluate immune infiltration and stromal infiltration of different subtypes. SsGSEA was performed to estimate the proportion of 28 immune-related cells (38). Immune checkpoint genes were downloaded from a previous study (39). Spearman correlation analysis was used to assess the association between risk score and immune cells.
Statistical analysis
The analysis in this study was supported by the Sangerbox platform (http://vip.sangerbox.com/) (40). Wilcoxon test was used to examine the difference between two groups. Kruskal-Wallis test was used to compare the difference among 3 groups. Log-rank test was conducted in Cox regression analysis and survival analysis. Benjamini and Hochberg (BH) method was used to correct P values. A P value <0.05 was considered significant.
Results
Identification of Gln-associated molecular subtypes in MM
We used ssGSEA to calculate the enrichment score of Gln-related pathway “GOBP_GLUTAMINE_FAMILY_AMINO_ACID_METABOLIC_PROCESS” for MM samples in GSE4581 dataset. To screen Gln-associated lncRNAs, we calculated the correlation between lncRNAs and the ssGSEA score of Gln-related pathway (termed Gln score in the following). A total of 50 lncRNAs significantly associated with Gln score were screened (P<0.05, |R| >0.25, Table S1). Then, we performed unsupervised consensus clustering to classify MM samples based on the expression data of 50 Gln-associated lncRNAs. According to the CDF curves and the area under CDF curves of different cluster number (k), k=3 was determined to divide samples into 3 clusters (Figure 1A-1C). Kaplan-Meier survival analysis showed a significant difference of OS in 3 clusters/subtypes (P=0.0024), where clust3 had the worst prognosis than other 2 subtypes (Figure 1D). The Gln score of clust3 was significantly higher than that of clust1 and clust2, indicating that Gln score may be a risk factor (Figure 1E). Previous research had identified 7 subgroups of MM (CCND (CD)1, CD2, MMSET (MS), MAF/MAFB (MF), hyperdiploid (HY), proliferation (PR), and low bone disease (LB)) based on mRNA expression profiles in CD138-enriched plasma cells using unsupervised hierarchic clustering (41). Among them, PR and MS had a worse prognosis compared with other MM subgroups. We compared our subtypes with previous subgroups and the result was visualized by Sankey diagram (Figure 1F). The MM samples with dead status were mostly in clust3 (Figure 1F,1G), and PR and MS also had higher proportions of dead samples compared with other subgroups. Our results of subtyping showed a consistent with previous research to some extent, which supported that the reliability of our subtyping based on Gln-associated lncRNAs. In another aspect, the subtyping also suggested that Gln-associated lncRNAs played an important role in MM progression.
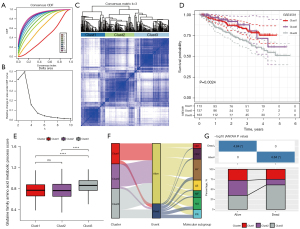
Biological pathways and immune characteristics of three subtypes
To understand the mechanism of Gln-associated lncRNAs in MM, we compared the enrichment of biological pathways in 3 subtypes by using gene set enrichment analysis (GSEA). In clust3 versus no_clust3, we observed that some oncogenic pathways and cell cycle-related pathways such as angiogenesis, epithelial-mesenchymal transition (EMT), G2M checkpoint, and E2F targets were relatively activated in clust3, whereas immune-related pathways such as interferon (IFN)-gamma response and IFN-alpha response were relatively suppressed in clust3 (Figure 2A). In clust2 versus no_clust2 and clust1 versus no_clust1, the above pathways were not significantly enriched, suggesting that Gln-associated lncRNAs may be involved in the regulation of these pathways.
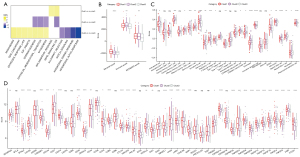
Tumor immune microenvironment is highly associated with tumor prognosis, which can be modulated by various factors such as hypoxia and metabolism. The association of Gln metabolism with tumor microenvironment has been revealed in the previous study (42). We assessed the immune infiltration in 3 subtypes, and found that clust3 had a lower ESTIMATE score than other 2 subtypes (Figure 2B). Enrichment analysis of 28 immune cells revealed a significant difference on the enrichment score of 20 immune cells in 3 subtypes (Figure 2C). In addition, we evaluated the expression levels of immune checkpoints, and most of immune checkpoints were differentially expressed in 3 subtypes (Figure 2D). Furthermore, we calculated the enrichment score of 15 immune-related pathways as shown in a heatmap (Figure S1). Three subtypes showed an evidently different expression pattern of these pathways. The different levels of immunomodulators and different enrichment of immune cells led to different immune response in three subtypes. Also, the above results also revealed an association between Gln metabolism and tumor immune microenvironment.
Establishment of a risk model related to Gln phenotype
As we found a significant difference of biological pathways and immune microenvironment in 3 subtypes, we then tried to construct a risk model based on the DElncRNAs among 3 subtypes. Firstly, we used differential analysis to identify DElncRNAs in clust1 versus no_clust1, clust2 versus no_clust2, and clust3 versus no_clust3. As a result, 154 DElncRNAs with 138 upregulated and 16 downregulated were identified in clust1 versus no_clust1 (Figure 3A). A total of 355 DElncRNAs with 15 upregulated and 340 downregulated were identified in clust2 versus no_clust2 (Figure 3B). A total of 17 DElncRNAs with 11 upregulated and 6 downregulated were identified in clust3 versus no_clust3 (Figure 3C). For a total of 421 DElncRNAs, we used univariate Cox regression to screen the DEGs associated with MM OS. A total of 27 DElncRNAs were screened with 21 risk lncRNAs and 6 protective lncRNAs under P<0.05 (Figure 3D). Subsequently, we used LASSO regression to decrease the number of prognostic genes. When lambda =0.01442, the model reached the optimal and 22 lncRNAs remained (Figure 3E,3F). Furthermore, stepAIC was conducted to further optimize the model by reducing AIC value. Finally, a total of 11 prognostic lncRNAs remained. The risk model was determined as follows: Risk Score = −0.624 × LINC01653 + 0.192 × TMPO-AS1 + 0.443 × THOC7-AS1 − 0.192 × AC116366.2 − 0.395 × AC090983.1 + 0.254 × ERVH-1 + 0.196 × MRGPRF-AS1 + 0.212 × AFDN-DT −0.191 × TARID −0.247 × C1QTNF1-AS1 + 0.288 × AL033530.1.
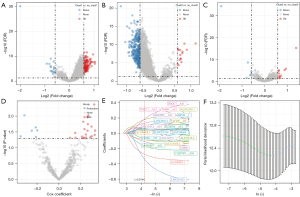
Validation of the 11-lncRNA risk model
GSE4581 was used as the training dataset and GSE57317 was used the validation dataset to assess the performance of the 11-lncRNA risk model. We calculated the risk score for each MM sample and converted risk score to z-score. The samples were divided into high-risk (z-score >0) and low-risk (z-score <0) groups according to the cut-off of z-score =0 (Figure 4A). Dead samples were more accumulated in the high-risk group compared with the low-risk group. The expression of 11 lncRNAs showed different enrichment in 2 risk groups, where TMPO-AS1, THOC7-AS1, MRGPRF-AS1, ERVH-1, AL033530.1, and AFDN-DT were relatively more highly expressed in the high-risk group. The results of ROC curve analysis presented that the risk model had a higher area under the ROC curve (AUC) score of 1-, 3-, and 5-year in the GSE4581 dataset, with 0.74, 0.71, and 0.79 respectively (Figure 4B). Two risk groups also displayed significantly differential OS in the GSE4581 dataset (Figure 4C). In the validation dataset, similar results were observed (Figure 4D,4E), suggesting that the 11-lncRNA risk model was effective to predict the prognosis of MM.
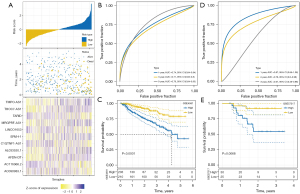
Next, we evaluated the performance of the risk model in the 7 MM subgroups. Survival analysis showed that MS and PR had shorter OS than other subgroups, which was consistent with the previous research (41) (Figure 5A). The Sankey diagram displayed that high-risk samples were more accumulated in MS and PR subgroups (Figure 5B). Comparison of risk score in the 7 subgroups revealed that PR subgroup had the highest risk score, followed by the MS subgroup (Figure 5C), indicating that the risk model was reliable and effective.
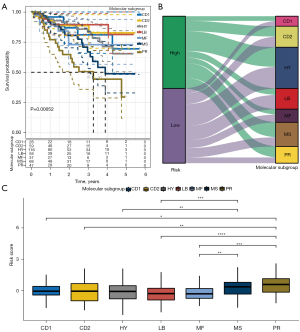
Pathway and immune analyses of two risk groups
We calculated the enrichment score of KEGG pathways for each MM sample in GSE4581 dataset. Spearman correlation analysis was performed to evaluate the relationship of risk score with KEGG pathways. A total of 7 pathways were screened to be significantly related to risk score (|R| >0.2 and P<0.05), where cell cycle was positively correlated with risk score and the Notch signaling pathway was negatively correlated with risk score (Figure 6A,6B). In the relationship of risk score with immune cell infiltration, we observed that some immune cells such as activated CD4 T cells, activated CD8 T cells, and memory B cells were differentially enriched in 2 risk groups (Figure 6C). Correlation analysis also showed a significant correlation of risk score with some immune cells including activated CD4 T cells, activated CD8 T cells, central memory CD4 T cells, memory B cells, type 1 T helper cells, type 2 T helper cells, CD56dim natural killer (NK) cells, eosinophils, macrophages, and NK cells (Figure 6D).
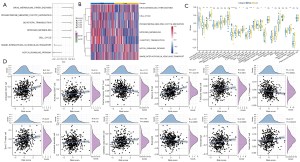
The prognostic value of 11 lncRNAs
The 11-lncRNA risk model was verified to be effective to predict MM prognosis in 2 independent datasets. We evaluated the relation of each lncRNA with OS by univariate Cox regression analysis. The result showed that 6 lncRNAs (TMPO-AS1, THOC7-AS1, MRGPRF-AS1, ERVH-1, AL033530.1, and AFDN-DT) were risk factors [hazard ratio (HR) >1] and 5 lncRNAs (LINC01653, AC090983.1, AC116366.2, TARID, and C1QTNF1-AS1) were protective factors (HR <1, Figure 7A). We examined their performance in classifying high-risk and low-risk groups. Except for TARID, the other 10 lncRNAs were effective to divide MM samples into 2 risk groups with distinct OS (Figure 7B).
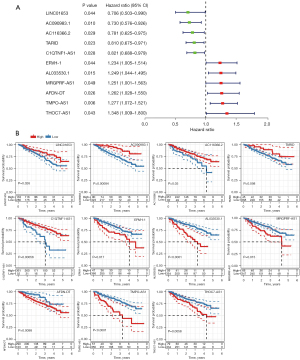
Discussion
Gln is the second commonly used source for energy after glucose in cancer cells. Gln can degrade to glutamate and ammonia (NH4+) under glutaminase (43). HMCLs produce excessive NH4+ and the upregulated level of NH4+ is also detected in CD138+ cells of MM patients (17). Inhibition of Gln transporter ASCT2 can effectively decrease Gln uptake and suppress HCML growth through a series downstream effects including changes in mTORC1 kinase activity, MYC transcription, protein synthesis, cell proliferation, and autophagy (43,44). LncRNAs are abundant in eukaryotes and have various functions according to different cellular localization. For instance, nuclear lncRNAs influence histone modification or transcriptional regulation (45). A study has demonstrated that lncRNAs regulate glucose, Gln, and lipid metabolism in cancer (46). However, the role of lncRNAs in Gln metabolism in MM patients is under-reported.
In the present study, we identified 50 lncRNAs that were significantly associated with Gln metabolism. Based on the expression profiles of the 50 lncRNAs, we identified 3 molecular subtypes (clust1, clust2, and clust3) through consensus clustering. Clust3 had the worst OS and the highest enrichment of Gln metabolism, indicating that the 50 lncRNAs may be involved in Gln metabolism and thus affect the prognosis in MM patients. Previous research also identified 7 subgroups of MM patients (CD1, CD2, HY, LB, MF, MS, and PR) according to the mRNA expression profiles of CD138-enriched plasma cells (41). In the comparison of our subtypes with previous subgroups, clust3 was mostly distributed in MS and PR subgroups, in line with the previous result that MS and PR had poor prognosis. The molecular subtyping based on Gln-associated lncRNAs suggested that the lncRNAs played an essential role in regulating Gln metabolism and thus affected MM prognosis,
We further assessed the link of Gln-associated lncRNAs with potential pathways and immune microenvironment. Some tumor-related pathways were significantly activated in clust3 compared with other subtypes, including angiogenesis, EMT, G2M checkpoint, and E2F targets. Angiogenesis is a typical hallmark of tumors, which enables tumor cells and epithelial cells to survive the hypoxic environment. It has been demonstrated that angiogenesis can be promoted under the addiction of epithelial cells to Gln (47). G2M checkpoint and E2F targets are cell cycle-related pathways that are frequently dysregulated in tumor, and G2M has been identified as a potential prognostic biomarker for breast cancer (48). EMT promotes cell proliferation and migration, which is a biomarker of poor prognosis in both solid tumor and hematological malignancy (49). It has been reported that hypoxia promotes the formation of bone marrow foci in MM cells through EMT process (49,50).
Immune-related pathways including IFN-alpha response and IFN-gamma response were relatively inhibited in clust3, indicating a suppressed immune response of clust3. Immune analysis also revealed that clust3 had lower immune infiltration than other subtypes. In addition, many immune cells and most of immune checkpoints were differentially enriched in 3 subtypes, which indicated that 3 subtypes had different immune microenvironments. Angiogenesis and EMT are highly associated with orchestration of the immune microenvironment (51,52), which may contribute to the different immune response in the 3 subtypes. Moreover, a study has shown that tumor cells competitively prey on glutamine in TME, resulting in limited availability of glutamine to tumor-infiltrating T lymphocytes, leading to immune escape (53). It has been proposed that the “glutamine steal” hypothesis that selectively blocking Gln metabolism in tumor cells can eliminate the metabolic competition for Gln in TME while releasing Gln for use by immune cells, thus enhancing the anti-tumor immune response (10).
Furthermore, we screened the DElncRNAs among 3 subtypes, and established a risk model based on the DElncRNAs. A total of 11 lncRNAs were included in the risk model, including TMPO-AS1, THOC7-AS1, MRGPRF-AS1, ERVH-1, AL033530.1, AFDN-DT, LINC01653, AC090983.1, AC116366.2, TARID, and C1QTNF1-AS1. The 11-lncRNA risk model was effective and robust to divide MM patients into high-risk and low-risk groups in 2 independent datasets. The high-risk group had significantly worse prognosis than the low-risk group. Notably, MS and PR subgroups showed higher risk score than the other subgroups, which was accordant with previous observations. TMPO-AS1 was reported to be overexpressed in various tumors such as prostate cancer and triple-negative breast cancer (54,55). TARID was revealed to promote demethylation and activation of TCF21, a tumor suppressor (56). Other lncRNAs have been scarcely reported in cancer, but they may be potential regulators involved in MM.
The relationship of risk score with biological pathways and immune cell infiltration was also evaluated in MM patients. Cell cycle and nitrogen metabolism were positively correlated with risk score, which was consistent with the results in subtype analysis. In addition, multiple immune cells were significantly correlated with risk score, including activated CD4 T cells, activated CD8 T cells, central memory CD4 T cells, memory B cells, type 1 T helper cells, type 2 T helper cells, CD56dim NK cells, eosinophils, macrophages, and NK cells. The close relationship of risk score with immune cells suggested that the 11 lncRNAs possibly played a regulatory role in the immune microenvironment. However, the experimental analysis in more clinical MM patients is needed to verify the risk model.
Our study has three main features. First, a comprehensive analysis of multiple omics data (metabolomics, genomics, transcriptomics) was performed. Secondly, we proposed Gln metabolism combined with lncRNA to construct a prognostic model, which is currently the first study conducted in MM. Finally, compared with a single prognostic gene, the polygenic prognostic model is better able to feedback the overall symptoms of the disease. However, The limitations of this study cannot be ignored. Firstly, all data were downloaded from public databases, and the sample size and clinical information were limited. Second, although a risk score system consisting of 11 lncRNAs has been created, the regulatory network and biological effects between these genes remain to be explored.
Conclusions
In conclusion, this study utilized the lncRNA expression profiles of MM patients to identify molecular subtypes and construct a risk model related to Gln phenotype. We explored the link of Gln metabolism with lncRNAs, biological pathways, and immune microenvironment. The 11-lncRNA risk model had a prognostic value to predict OS for MM patients. In addition, the 11 lncRNAs may serve as potential targets for studying further mechanism of Gln metabolism in MM patients.
Acknowledgments
Funding: None.
Footnote
Reporting Checklist: The authors have completed the TRIPOD reporting checklist. Available at https://atm.amegroups.com/article/view/10.21037/atm-22-6190/rc
Conflicts of Interest: All authors have completed the ICMJE uniform disclosure form (available at https://atm.amegroups.com/article/view/10.21037/atm-22-6190/coif). The authors have no conflicts of interest to declare.
Ethical Statement: The authors are accountable for all aspects of the work in ensuring that questions related to the accuracy or integrity of any part of the work are appropriately investigated and resolved. The study was conducted in accordance with the Declaration of Helsinki (as revised in 2013).
Open Access Statement: This is an Open Access article distributed in accordance with the Creative Commons Attribution-NonCommercial-NoDerivs 4.0 International License (CC BY-NC-ND 4.0), which permits the non-commercial replication and distribution of the article with the strict proviso that no changes or edits are made and the original work is properly cited (including links to both the formal publication through the relevant DOI and the license). See: https://creativecommons.org/licenses/by-nc-nd/4.0/.
References
- Kumar SK, Rajkumar V, Kyle RA, et al. Multiple myeloma. Nat Rev Dis Primers 2017;3:17046. [Crossref] [PubMed]
- Cowan AJ, Green DJ, Kwok M, et al. Diagnosis and Management of Multiple Myeloma: A Review. JAMA 2022;327:464-77. [Crossref] [PubMed]
- Sung H, Ferlay J, Siegel RL, et al. Global Cancer Statistics 2020: GLOBOCAN Estimates of Incidence and Mortality Worldwide for 36 Cancers in 185 Countries. CA Cancer J Clin 2021;71:209-49. [Crossref] [PubMed]
- Kumar SK, Dispenzieri A, Lacy MQ, et al. Continued improvement in survival in multiple myeloma: changes in early mortality and outcomes in older patients. Leukemia 2014;28:1122-8. [Crossref] [PubMed]
- Chung DJ, Pronschinske KB, Shyer JA, et al. T-cell Exhaustion in Multiple Myeloma Relapse after Autotransplant: Optimal Timing of Immunotherapy. Cancer Immunol Res 2016;4:61-71. [Crossref] [PubMed]
- Zelle-Rieser C, Thangavadivel S, Biedermann R, et al. T cells in multiple myeloma display features of exhaustion and senescence at the tumor site. J Hematol Oncol 2016;9:116. [Crossref] [PubMed]
- Suen H, Brown R, Yang S, et al. Multiple myeloma causes clonal T-cell immunosenescence: identification of potential novel targets for promoting tumour immunity and implications for checkpoint blockade. Leukemia 2016;30:1716-24. [Crossref] [PubMed]
- D'Souza A, Hari P, Pasquini M, et al. A Phase 2 Study of Pembrolizumab during Lymphodepletion after Autologous Hematopoietic Cell Transplantation for Multiple Myeloma. Biol Blood Marrow Transplant 2019;25:1492-7. [Crossref] [PubMed]
- Jing W, Gershan JA, Weber J, et al. Combined immune checkpoint protein blockade and low dose whole body irradiation as immunotherapy for myeloma. J Immunother Cancer 2015;3:2. [Crossref] [PubMed]
- Ma G, Zhang Z, Li P, et al. Reprogramming of glutamine metabolism and its impact on immune response in the tumor microenvironment. Cell Commun Signal 2022;20:114. [Crossref] [PubMed]
- Yuneva M, Zamboni N, Oefner P, et al. Deficiency in glutamine but not glucose induces MYC-dependent apoptosis in human cells. J Cell Biol 2007;178:93-105. [Crossref] [PubMed]
- Ward PS, Patel J, Wise DR, et al. The common feature of leukemia-associated IDH1 and IDH2 mutations is a neomorphic enzyme activity converting alpha-ketoglutarate to 2-hydroxyglutarate. Cancer Cell 2010;17:225-34. [Crossref] [PubMed]
- Sun RC, Denko NC. Hypoxic regulation of glutamine metabolism through HIF1 and SIAH2 supports lipid synthesis that is necessary for tumor growth. Cell Metab 2014;19:285-92. [Crossref] [PubMed]
- Dasgupta S, Putluri N, Long W, et al. Coactivator SRC-2-dependent metabolic reprogramming mediates prostate cancer survival and metastasis. J Clin Invest 2015;125:1174-88. [Crossref] [PubMed]
- Jiang L, Shestov AA, Swain P, et al. Reductive carboxylation supports redox homeostasis during anchorage-independent growth. Nature 2016;532:255-8. [Crossref] [PubMed]
- Altman BJ, Stine ZE, Dang CV. From Krebs to clinic: glutamine metabolism to cancer therapy. Nat Rev Cancer 2016;16:619-34. [Crossref] [PubMed]
- Bolzoni M, Chiu M, Accardi F, et al. Dependence on glutamine uptake and glutamine addiction characterize myeloma cells: a new attractive target. Blood 2016;128:667-79. [Crossref] [PubMed]
- Roberts RS, Hsu HW, Lin KD, et al. Amino acid metabolism of myeloma cells in culture. J Cell Sci 1976;21:609-15. [Crossref] [PubMed]
- Dalva-Aydemir S, Bajpai R, Martinez M, et al. Targeting the metabolic plasticity of multiple myeloma with FDA-approved ritonavir and metformin. Clin Cancer Res 2015;21:1161-71. [Crossref] [PubMed]
- Bajpai R, Matulis SM, Wei C, et al. Targeting glutamine metabolism in multiple myeloma enhances BIM binding to BCL-2 eliciting synthetic lethality to venetoclax. Oncogene 2016;35:3955-64. [Crossref] [PubMed]
- Handa H, Kuroda Y, Kimura K, et al. Long non-coding RNA MALAT1 is an inducible stress response gene associated with extramedullary spread and poor prognosis of multiple myeloma. Br J Haematol 2017;179:449-60. [Crossref] [PubMed]
- Yang X, Ye H, He M, et al. LncRNA PDIA3P interacts with c-Myc to regulate cell proliferation via induction of pentose phosphate pathway in multiple myeloma. Biochem Biophys Res Commun 2018;498:207-13. [Crossref] [PubMed]
- Sun Y, Pan J, Zhang N, et al. Knockdown of long non-coding RNA H19 inhibits multiple myeloma cell growth via NF-κB pathway. Sci Rep 2017;7:18079. [Crossref] [PubMed]
- Meng YB, He X, Huang YF, et al. Long Noncoding RNA CRNDE Promotes Multiple Myeloma Cell Growth by Suppressing miR-451. Oncol Res 2017;25:1207-14. [Crossref] [PubMed]
- He J, Li F, Zhou Y, et al. LncRNA XLOC_006390 promotes pancreatic carcinogenesis and glutamate metabolism by stabilizing c-Myc. Cancer Lett 2020;469:419-28. [Crossref] [PubMed]
- Zeng B, Ye H, Chen J, et al. LncRNA TUG1 sponges miR-145 to promote cancer progression and regulate glutamine metabolism via Sirt3/GDH axis. Oncotarget 2017;8:113650-61. [Crossref] [PubMed]
- Wang R, Cao L, Thorne RF, et al. LncRNA GIRGL drives CAPRIN1-mediated phase separation to suppress glutaminase-1 translation under glutamine deprivation. Sci Adv 2021;7:eabe5708. [Crossref] [PubMed]
- Jiang H, Wong WH. SeqMap: mapping massive amount of oligonucleotides to the genome. Bioinformatics 2008;24:2395-6. [Crossref] [PubMed]
- Liberzon A, Birger C, Thorvaldsdóttir H, et al. The Molecular Signatures Database (MSigDB) hallmark gene set collection. Cell Syst 2015;1:417-25. [Crossref] [PubMed]
- Hänzelmann S, Castelo R, Guinney J. GSVA: gene set variation analysis for microarray and RNA-seq data. BMC Bioinformatics 2013;14:7. [Crossref] [PubMed]
- Wilkerson MD, Hayes DN. ConsensusClusterPlus: a class discovery tool with confidence assessments and item tracking. Bioinformatics 2010;26:1572-3. [Crossref] [PubMed]
- Ritchie ME, Phipson B, Wu D, et al. limma powers differential expression analyses for RNA-sequencing and microarray studies. Nucleic Acids Res 2015;43:e47. [Crossref] [PubMed]
- Friedman J, Hastie T, Tibshirani R. Regularization Paths for Generalized Linear Models via Coordinate Descent. J Stat Softw 2010;33:1-22.
- Zhang Z. Variable selection with stepwise and best subset approaches. Ann Transl Med 2016;4:136. [Crossref] [PubMed]
- Blanche P, Dartigues JF, Jacqmin-Gadda H. Estimating and comparing time-dependent areas under receiver operating characteristic curves for censored event times with competing risks. Stat Med 2013;32:5381-97. [Crossref] [PubMed]
- Yu G, Wang LG, Han Y, et al. clusterProfiler: an R package for comparing biological themes among gene clusters. OMICS 2012;16:284-7. [Crossref] [PubMed]
- Yoshihara K, Shahmoradgoli M, Martínez E, et al. Inferring tumour purity and stromal and immune cell admixture from expression data. Nat Commun 2013;4:2612. [Crossref] [PubMed]
- Charoentong P, Finotello F, Angelova M, et al. Pan-cancer Immunogenomic Analyses Reveal Genotype-Immunophenotype Relationships and Predictors of Response to Checkpoint Blockade. Cell Rep 2017;18:248-62. [Crossref] [PubMed]
- Liu Y, He M, Wang D, et al. HisgAtlas 1.0: a human immunosuppression gene database. Database (Oxford) 2017;2017:bax094. [Crossref] [PubMed]
- Shen W, Song Z, Xiao Z, et al. Sangerbox: A comprehensive, interaction‐friendly clinical bioinformatics analysis platform. iMeta 2022;3:e36.
- Zhan F, Huang Y, Colla S, et al. The molecular classification of multiple myeloma. Blood 2006;108:2020-8. [Crossref] [PubMed]
- Zhu L, Zhu X, Wu Y. Effects of Glucose Metabolism, Lipid Metabolism, and Glutamine Metabolism on Tumor Microenvironment and Clinical Implications. Biomolecules 2022;12:580. [Crossref] [PubMed]
- El Arfani C, De Veirman K, Maes K, et al. Metabolic Features of Multiple Myeloma. Int J Mol Sci 2018;19:1200. [Crossref] [PubMed]
- Giuliani N, Chiu M, Bolzoni M, et al. The potential of inhibiting glutamine uptake as a therapeutic target for multiple myeloma. Expert Opin Ther Targets 2017;21:231-4. [Crossref] [PubMed]
- Long Y, Wang X, Youmans DT, et al. How do lncRNAs regulate transcription? Sci Adv 2017;3:eaao2110. [Crossref] [PubMed]
- Lin W, Zhou Q, Wang CQ, et al. LncRNAs regulate metabolism in cancer. Int J Biol Sci 2020;16:1194-206. [Crossref] [PubMed]
- Polet F, Feron O. Endothelial cell metabolism and tumour angiogenesis: glucose and glutamine as essential fuels and lactate as the driving force. J Intern Med 2013;273:156-65. [Crossref] [PubMed]
- Oshi M, Takahashi H, Tokumaru Y, et al. G2M Cell Cycle Pathway Score as a Prognostic Biomarker of Metastasis in Estrogen Receptor (ER)-Positive Breast Cancer. Int J Mol Sci 2020;21:2921. [Crossref] [PubMed]
- Greaves D, Calle Y. Epithelial Mesenchymal Transition (EMT) and Associated Invasive Adhesions in Solid and Haematological Tumours. Cells 2022;11:649. [Crossref] [PubMed]
- Azab AK, Hu J, Quang P, et al. Hypoxia promotes dissemination of multiple myeloma through acquisition of epithelial to mesenchymal transition-like features. Blood 2012;119:5782-94. [Crossref] [PubMed]
- Albini A, Bruno A, Noonan DM, et al. Contribution to Tumor Angiogenesis From Innate Immune Cells Within the Tumor Microenvironment: Implications for Immunotherapy. Front Immunol 2018;9:527. [Crossref] [PubMed]
- Aggarwal V, Montoya CA, Donnenberg VS, et al. Interplay between tumor microenvironment and partial EMT as the driver of tumor progression. iScience 2021;24:102113. [Crossref] [PubMed]
- Edwards DN, Ngwa VM, Raybuck AL, et al. Selective glutamine metabolism inhibition in tumor cells improves antitumor T lymphocyte activity in triple-negative breast cancer. J Clin Invest 2021;131:140100. [Crossref] [PubMed]
- Huang W, Su X, Yan W, et al. Overexpression of AR-regulated lncRNA TMPO-AS1 correlates with tumor progression and poor prognosis in prostate cancer. Prostate 2018;78:1248-61. [Crossref] [PubMed]
- Mitobe Y, Ikeda K, Sato W, et al. Proliferation-associated long noncoding RNA, TMPO-AS1, is a potential therapeutic target for triple-negative breast cancer. Cancer Sci 2020;111:2440-50. [Crossref] [PubMed]
- Arab K, Park YJ, Lindroth AM, et al. Long noncoding RNA TARID directs demethylation and activation of the tumor suppressor TCF21 via GADD45A. Mol Cell 2014;55:604-14. [Crossref] [PubMed]
(English Language Editor: J. Jones)