Establishment and verification of a nomogram and a preliminary study on predicting the clinical response of conventional synthetic disease-modifying antirheumatic drugs (csDMARDs) in rheumatoid arthritis patients
Highlight box
Key findings
• We verified the prognostic value of some inflammatory indicators in RA and establish a model to predict the remission rate after treatment.
What is known and what is new?
• IL-6, Gal-9 and other indicators had a significant impact on the prognosis.
• Patient age, CRP, IL-6, Gal-9, HAQ, and DAS28-ESR were included in the prognostic model to predict the remission rate.
What is the implication, and what should change now?
• We need to establish good prediction models that can accurately predict the remission rate of patients to choose good treatment schemes.
Introduction
Rheumatoid arthritis (RA) is a common autoimmune disease which is characterized by synovial hyperplasia and pannus infiltration, wherein various inflammatory cytokines promote the persistence of synovial inflammation (1). Without effective treatment, some patients are not only prone to joint disability but also other complications, such as cardiovascular, pulmonary interstitial, and blood system diseases, which seriously affect their quality of life (1,2). Although RA is still incurable, most patients can achieve remission or low disease activity (LDA) through effective treatment (3). Effective treatment of RA relies on early diagnosis and swift initiation of treatment. The disease activity score-28 for RA with erythrocyte sedimentation rate (DAS28-ESR) is one of the most widely used indicators to measure disease activity. The clinical remission threshold is defined as DAS28-ESR <2.6. The core treatment principles of RA are shared decision-making and regular review with a target of remission or LDA (within 3–6 months or as long as possible) to ensure optimal outcomes (4-6). However, the concept of treat-to-target (T2T) can only be realized in some patients, and patients who are resistant or intolerant to conventional synthetic disease-modifying antirheumatic drugs (csDMARDs) have to adjust the treatment scheme to a combination of csDMARDs and targeted DMARDs (tDMARDs) (7). The main reason is that the early intervention for RA patients is insufficient, and patients with poor therapeutic effects cannot be screened out in the early stage.
In the past decade, significant progress has been made in targeting cytokines as a treatment for RA. Cytokines are closely related to every stage of RA, and their complex role greatly promotes the development of arthritis. Targeted cytokine therapy has made great progress as a therapeutic regimen for RA, but the role of these inflammatory factors in disease prediction, prognosis, and recurrence remains controversial (8). Interleukin-6 (IL-6) is a very important pro-inflammatory factor in the occurrence and development of RA as it can regulate the inflammatory response, angiogenesis, and bone metabolism (9). Galectin-9 (Gal-9) is a tandem repeat glycoprotein in the galactose lectin family (10). Previous studies have confirmed that tumor necrosis factor-α (TNF-α) can stimulate high expression of Gal-9 in vascular endothelial cells in vitro (11,12), and exogenous Gal-9 can promote RA progression by inducing angiogenesis (13). It has been shown that that plasma Gal-9 is closely related to disease activity, TNF-α, and vascular endothelial growth factor (VEGF) level in RA patients, and both plasma and T cell subsets of Gal-9 can reflect the therapeutic response effect of csDMARDs to a certain extent (14). The serum levels of the above-mentioned cytokines can be accurately measured in active RA disease. Theoretically, they can be used for the diagnosis and prognosis of RA. However, at present, no single index can be used to predict patient prognosis. Therefore, it is necessary to combine biomarkers, clinical symptoms, and objective indicators to objectively measure the prognosis of patients and their response to treatment.
Predictive models have been established in many autoimmune diseases to evaluate the individual probability of clinical remission, such as the duration of remission, and have provided a more accurate individual risk assessment method than the traditional staging system. The use of nomograms to assess the individual probability of clinical events in autoimmune diseases provides a more accurate individual risk assessment method compared with the traditional staging system (15-17). However, most of the established nomograms in RA only predict the disease activity of patients based on their clinical characteristics (18,19). There is no good prediction model that can accurately predict the remission rate of patients to choose a good treatment scheme. The combination of disease activity indicators and inflammatory factor indicators helps to combine subjective symptoms with objective examinations, comprehensively evaluate the current disease status of patients, and make the prediction model more accurate and effective. Currently, only a few cytokines are included in these nomograms to predict disease activity, and there are no reports of predictive models for the therapeutic efficacy of csDMARDs.
In this study, we evaluated the significance of cytokine expression levels closely related to disease activity in RA plasma samples and verified the predictive potential of these inflammatory factors in RA for therapeutic efficacy. More importantly, we further constructed a nomogram based on baseline inflammatory factors levels and disease activity indicators (DAIs), which showed significant discrimination, accuracy, and clinical applicability in predicting prognosis. We present the following article in accordance with the TRIPOD reporting checklist (available at https://atm.amegroups.com/article/view/10.21037/atm-22-5791/rc).
Methods
Patient information
The study involved the medical records of RA patients with moderate and severe disease activity (DAS28-ESR of ≥3.2) who received csDMARDs in the Outpatient Department of Rheumatology, Qilu Hospital, Shandong University (Shandong, China) from June 2014 to June 2020. All cases met the RA standard of the American College of Rheumatology (ACR) in 1987 or 2010 the ACR/European League Against Rheumatism (EULAR) criteria. The inclusion criteria were as follows: (I) age >18 years; (II) no pregnancy preparation or pregnancy planned within 6 months; (III) stable extraarticular manifestations; (IV) DAS28-ESR of ≥3.2. The exclusion criteria were as follows: (I) patients receiving biological agents within 3 months; (II) patients with liver and kidney dysfunction; (III) patients with acute and chronic infection; (IV) patients with a history of malignant tumor; (V) patients with severe hypertension, diabetes, coronary heart disease, or other autoimmune diseases. All patients who participated in this study signed an informed consent form, and this study was approved by the Human Research Ethics Committees of Qilu Hospital (KYLL-2015-269; 2016072). The study was conducted in accordance with the Declaration of Helsinki (as revised in 2013). Sample sizes were calculated by R software (The R Foundation for Statistical Computing, Vienna, Austria). Participants were randomly divided into the development cohort and validation cohort by R software at an approximate ratio of 7:3 to establish and verify the prediction model.
Treatment and follow-up
After collecting a detailed medical history and performing a comprehensive physical examination, the clinical information of all patients was recorded, including age, gender, course of disease, erythrocyte sedimentation rate (ESR), C-reactive protein (CRP), tenderness joint 28 count (T28), swelling joint 28 count (SW28), patient global assessment (PGA), physician global assessment (PHGA), health assessment questionnaire (HAQ), and DAS28-ESR. PGA was scored using a visual analogue scale (VAS). Depending on the type of score used, the PGA can range from 0–10 cm. Higher scores represent a higher level of disease activity or a worse global health. PHGA was scored on a scale of 0 defined as absence of disease activity to 10 defined as maximum disease activity, based on an overall assessment of disease activity in the past weeks. DAS28-ESR was calculated as 0.56 × √(T28) + 0.28 × √(SW28) + 0.7 × ln ESR + 0.014 × VAS-GH. Plasma was collected to detect the levels of TNF-α, VEGF, Gal-9, and IL-6. The patients were treated with 1 or more combinations of methotrexate (MTX), leflunomide (LEF), hydroxychloroquine (HCQ), iguratimod (IGU) and tacrolimus (TAC). Some patients were also treated with glucocorticoid (GC) and non-steroidal anti-inflammatory drugs (NSAIDs). At each visit, the physician made adjustments to the medication regimen according to the disease activity and medicine response and tolerance to ensure the stability of the patient’s treatment strategy. The follow-up was planned at 0, 3, and 6 months within the first 6 months, and then every 3 months. The maximum follow-up time was 36 months. Many patients were not followed up at the planned time point, but we accurately recorded the appointed time and disease activity evaluation of each follow-up visit. If clinically necessary, we arranged additional follow-ups. The first remission time was defined as the time from the beginning of treatment to the first observation of DAS28-ESR of <2.6. There were 4 forms of observed end events: (I) DAS28-ESR of <2.6 had been reached at the time of analysis; (II) traditional DMARDs drugs were continued when DAS28-ESR of <2.6 had not been reached at the time of analysis; (III) at the last observation, patients who not reached a DAS28-ESR of <2.6 were changed to biologic DMARDS (bDMARDs) or tDMARDs; (IV) the patient was lost after the last observation.
Statistical methods
The software SPSS 25.0 (IBM Corp., Armonk, NY, USA) and R version 4.0.2 (The R Foundation, Boston, MA, USA) were used for statistical analysis, and the frequency and proportion of classified variables were described. Continuous variables were described using median [interquartile range (IQR)] or mean [standard deviation (SD)]. Student’s t-test was used to test continuous measurements between study groups, and either the chi-square test or Fisher’s exact test was used for classified measurements. The best critical value of plasma inflammatory factor level was calculated according to the Cutoff Finder package in R, and the patients were divided into subgroups. The first remission time between subgroups was compared by Kaplan-Meier analysis of the log rank test. Least absolute shrinkage and selection operator (LASSO) regression was used to screen the 14 variables at baseline. Univariate Cox regression analysis and multivariate Cox regression analysis were performed for the screened variables, and the prediction model was established to draw the nomogram. The prediction model was evaluated by 3 aspects: discrimination, calibration, and clinical net income; c-index was calculated, and the time-dependent receiver operating characteristic (ROC) curve was used to evaluate the discrimination ability of the model. A calibration curve was used for the inspection and evaluation of calibration capability. Decision curve analysis (DCA) was used to evaluate clinical effectiveness. A P value of <0.05 was considered statistically significant.
Results
Clinical characteristics of patients
A total of 223 patients provided baseline blood samples and had at least 1 follow-up, of which 156 patients were assigned to the development cohort, and 67 patients were assigned to the validation cohort (Figure 1). There were no significant differences in baseline demographics, clinical characteristics, and inflammatory factor levels between the 2 cohorts (Table 1).
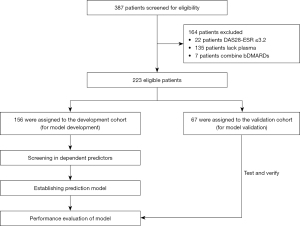
Table 1
Characteristic | Development cohort (n=156) | Validation cohort (n=67) | P value |
---|---|---|---|
Age, mean ± SD, years | 48.22±11.44 | 47.27±10.98 | 0.60 |
Sex(male/female) | 20/136 | 11/56 | – |
Disease duration, median (IQR), months | 12 (6–36) | 12 (3–36) | 0.77 |
ACPA+, rate (%) | 75.20 | 78.20 | 0.66 |
ESR, mean ± SD, mm/h | 48.45±29.71 | 52.11±32.31 | 0.46 |
CRP, mean ± SD, mg/L | 14.69±20.38 | 17.25±18.38 | 0.42 |
TNF-α, median (IQR), pg/mL | 3.36 (2.99–5.66) | 3.30 (2.94–4.80) | 0.49 |
VEGF, median (IQR), pg/mL | 91.15 (41.53–317.58) | 80.66 (40.08–208.66) | 0.72 |
IL-6, median (IQR), pg/mL | 4.71 (2.54–14.79) | 10.35 (2.85–16.67) | 0.31 |
Gal-9, median (IQR), pg/mL | 5,240.00 (3,180.00–8,640.00) | 5,000 (3,240.00–9,440.00) | 0.40 |
T28, mean ± SD | 8.33±4.17 | 9.15±5.6 | 0.27 |
SW28, mean ± SD | 5.42±4.02 | 6.00±5.63 | 0.43 |
PGA, mean ± SD | 2.72±0.94 | 2.91±1.13 | 0.24 |
PHGA, mean ± SD | 2.92±0.79 | 3.05±0.89 | 0.32 |
HAQ, mean ± SD | 0.43±0.37 | 0.43±0.27 | 0.98 |
DAS28-ESR, mean ± SD | 4.72±0.83 | 4.85±0.9 | 0.24 |
DAS28-CRP, mean ± SD | 3.87±0.78 | 4.06±0.95 | 0.32 |
GC combination rate (%) | 41.09 | 32.73 | 0.29 |
MTX combination rate (%) | 60.47 | 70.91 | 0.18 |
HCQ combination rate (%) | 70.54 | 81.82 | 0.11 |
T-614 combination rate (%) | 60.50 | 61.80 | 0.86 |
FK506 combination rate (%) | 39.50 | 38.20 | 0.86 |
RA, rheumatoid arthritis; SD, standard deviation; IQR, interquartile range; ACPA, anticitrullinated protein antibody; ESR, erythrocyte sedimentation rate; CRP, C-reactive protein; TNF-α, tumor necrosis factor-α; VEGF, vascular endothelial growth factor; IL-6, interleukin-6; Gal-9, galectin-9; T28, tenderness joint 28 count; SW28, swelling joint 28 count; PGA, patient global assessment; PHGA, physician global assessment; HAQ, health assessment questionnaire; DAS28-ESR, disease activity score-28 for rheumatoid arthritis with erythrocyte sedimentation rate; DAS28-CRP, disease activity score in 28 joints using C-reactive protein; GC, glucocorticoid; MTX, methotrexate; HCQ, hydroxychloroquine; T-614, iguratimod; FK506, tacrolimus.
Prognostic value of inflammatory factors
In the development cohort, the median first remission time was 8 months [95% confidence interval (CI): 4.01–11.98 months], and the remission rates in 3, 6, and 12 months were 38.76%, 58.91%, and 81.40%, respectively.
For the measured inflammatory indexes, the best cutoff value was calculated by using the biometric tool cutoff finder, and the patients were divided into 2 subgroups. We found that the first remission time for patients with IL-6 of ≤9.03 pg/mL (6 months, 95% CI: 1.46–10.54 months) was shorter than that for patients with IL-6 of >9.03 pg/mL (15 months, 95% CI: 3.53–26.47 months) (log rank, P=0.03). The first remission time for patients with Gal-9 of ≤4,490 pg/mL (4 months, 95% CI: 2.56–5.44 months) was shorter than that for patients with Gal-9 of >4,490 pg/mL (15 months, 95% CI: 11.27–18.73 months) (log rank, P=0.000). We also found that TNF-α, VEGF, and other indexes alone had no significant predictive ability for the first remission time (Figure 2).
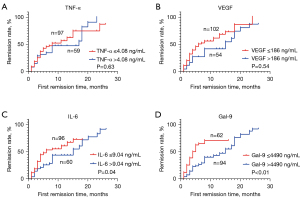
Establishment of prognosis model based on inflammatory factors and disease activity
There were 14 clinical features and inflammatory indicators in the development cohort. LASSO regression analysis was used to select the best 6 potential predictors, which were age, CRP, IL-6, Gal-9, HAQ, and DAS28-ESR (Figure 3).
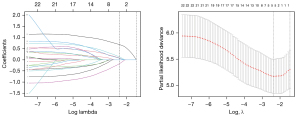
Univariate Cox regression analysis was run for these 6 predictors, and it found that Gal-9, IL-6, HAQ, and DAS28-ESR were significantly correlated with the first remission time. When multiple logistic regression analysis was performed, Gal-9, IL-6, and DAS28-ESR were still significantly correlated with the first remission time (Table 2). Then, we used the above-indicated 6 predictors to construct a nomogram for predicting the first remission time. It showed that IL-6, Gal-9, and other indicators had a significant impact on the prognosis (Figure 4). The C-index of the development cohort was 0.73 (95% CI: 0.56–0.90). The C-index of the validation cohort was 0.70 (95% CI: 0.53–0.88). The time-dependent ROC curve analysis showed that the nomogram based on inflammatory factors and disease activity had significant discrimination in predicting remission. The higher the nomogram score, the shorter the first remission time. According to the nomogram, the areas under the curve (AUCs) of the development cohort at 3, 6, and 12 months were 0.76, 0.82, and 0.73, respectively (Figure 5). The calibration map showed the best consistency between nomogram prediction and actual observation (Figure 6). We used DCA to analyze the clinical usefulness. The results showed that the nomogram demonstrated the net benefit of nomogram-assisted decisions at a wide range of threshold probability (Figure 7).
Table 2
Variable | Univariate | Multivariate | |||||
---|---|---|---|---|---|---|---|
HR | 95% CI | P value | HR | 95% CI | P value | ||
Age, years | |||||||
≤45 vs. >45 | 0.69 | 0.43–1.12 | 0.13 | 0.75 | 0.46–1.21 | 0.24 | |
CRP | |||||||
≤10 vs. >10 | 0.87 | 0.53–1.42 | 0.57 | 1.56 | 0.87–2.82 | 0.14 | |
IL-6 | |||||||
≤9.04 vs. >9.04 | 0.58 | 0.34–0.97 | 0.04 | 0.54 | 0.31–0.95 | 0.03 | |
Gal-9 | <0.01 | 0.03 | |||||
≤4,490 vs. >4,490 and ≤8,980 | 0.37 | 0.18–0.73 | <0.01 | 0.43 | 0.21–0.89 | 0.02 | |
≤4,490 vs. >8,980 | 0.44 | 0.26–0.77 | <0.01 | 0.51 | 0.29–0.90 | 0.02 | |
HAQ | |||||||
≤0.5 vs. >0.5 | 1.71 | 1.01–2.9 | 0.05 | 0.65 | 0.36–1.17 | 0.15 | |
DAS28-ESR | |||||||
≤5.3 vs. >5.3 | 0.56 | 0.34–0.93 | 0.02 | 0.50 | 0.28–0.87 | 0.01 |
HR, hazard ratio; CI, confidence interval; CRP, C-reactive protein; IL-6, interleukin-6; Gal-9, galectin-9; HAQ, health assessment questionnaire; DAS28-ESR, disease activity score-28 for rheumatoid arthritis with erythrocyte sedimentation rate.
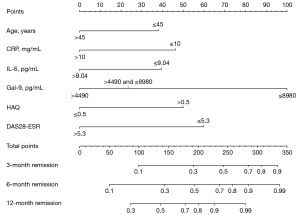
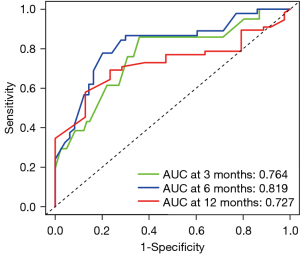
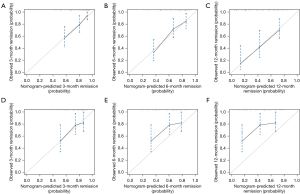
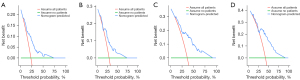
Application of the prognosis model
According to the risk score calculation constructed by the nomogram, the risk score of each participant was calculated in the development cohort. The Cutoff Finder calculated the best critical value of the risk score (192.5 points) and divided the whole group of patients into high-risk and low-risk risk groups. In the development cohort, the first remission time of the low-risk group was 4 months (95% CI: 3.12–4.89 months), and the first remission time in the high-risk group was 17.0 months (95% CI: 13.45–20.54 months) (P=0.000). In the validation cohort, the first remission time in the low-risk group was 6 months (95% CI: 3.00–9.00 months), and the first remission time in the high-risk group was 18.0 months (95% CI: 12.91–23.10 months) (P=0.000) (Figure 8).
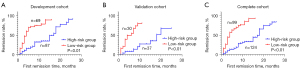
Discussion
The goal of RA treatment is to rapidly achieve and maintain remission. Accurate measurement of the first remission time in the early stage of treatment is of great significance for the selection of a treatment plan and management of chronic diseases (20). The T2T recommendations are a treatment method adjusted to set time intervals to achieve clear clinically relevant goals (21). The selection of targets for standard treatment is based on medical evidence. Large randomized clinical trials have shown that the T2T treatment strategy produces better results compared with standard treatment. However, the implementation of T2T in autoimmune diseases is much more complicated, and the goal related to reaching the standard is not a single parameter but requires the combined scores of multiple clinical and laboratory changes (22). Compared with standard treatment, T2T has a better response to treatment, higher remission rate, and less imaging detectable damage in RA patients (23,24). The patients in this study had high compliance and good overall efficacy. All patients in our cohort studies had moderate-to-severe disease activity, and there were many poor prognostic factors. However, no matter which index, the DAS28-ESR, DAS28-CRP, simplified disease activity index (SDAI), or clinical disease activity index (CDAI), is used to describe disease activity; most patients can achieve remission within 12 months after treatment with csDMARDs and remain stable in the long-term follow-up. Our research provides better practical significance for T2T implementation. However, there are always some patients who cannot achieve remission in the long-term treatment follow-up, which requires us to screen these patients at an early stage and select a stronger treatment scheme.
The pathological feature of RA is the infiltration of immune and inflammatory cells into the joint synovium, which prolifically secrete inflammatory factors (25,26). Different inflammatory factors can coexist in the inflammatory microenvironment of RA and reach a certain level in the serum. These inflammatory factors play a synergistic, antagonistic, or non-interference role in the occurrence, development, and response to RA treatment (27). No matter the role of these inflammatory factors, they may be related to disease activity and have certain prognostic value. In patients with RA, high levels of IL-6 can be detected in synovial fluid, suggesting that IL-6 is a crucial factor in RA. The cytokine IL-6 induces nuclear factors κ molecules, such as B receptor activator ligand (RANKL), prostaglandins, and matrix metalloproteinases, which mediate signs and symptoms of RA, such as pain and swelling (28). Previous studies have shown that the level of IL-6 is usually regarded as a marker for most infectious and inflammatory diseases (29,30). Especially after the advent of IL-6 antagonists, the correlation between the level of IL-6 and the prognosis of patients using IL-6 antagonists have been continuously reported (31,32). This evidence shows that IL-6 has a strong predictive effect on the prognosis.
The cytokine Gal-9 plays an important role in cell adhesion, aggregation, and cell death and is closely related to the occurrence and development of RA (33). According to existing reports, the functions of Gal-9 are complex and even contradictory. Knockout of Gal-9 by small interfering RNA (siRNA) can induce apoptosis of synovial fibroblasts in RA. Our own research has also shown that when C57BL/6 mice were injected with Gal-9 directly into the knee, the inflammation and monocyte migration were significantly higher than those in the negative control (34). This suggests that endogenous and exogenous forms of Gal-9 play opposite roles in regulating the occurrence and development of RA, and the role of Gal-9 in RA needs to be investigated in further research. No matter the role Gal-9 plays in RA, our previous studies have confirmed that Gal-9 can distinguish RA patients from normal controls and it has shown the potential to predict treatment results (13). In addition, there is a correlation between Gal-9, adverse clinical outcomes, and therapeutic potential of some autoimmune diseases (35,36), including systemic lupus erythematosus and dermatomyositis. In this study, we detected the expression of IL-6 and Gal-9. Our results showed that IL-6 and Gal-9 were closely related to the remission rate of patients, showed good discrimination ability, and were tested as factors predicting the time of the first remission.
Currently, the prediction of RA prognosis mainly includes the evaluation of disease activity, autoantibodies, and genetics. However, these indicators are mainly based on the stratification of RA patients, and there is no appropriate tool to predict the remission time and rate. We used LASSO regression to select and model the variables of 14 clinical features and inflammatory indexes in the way of punishment. Being different from other statistical modeling methods, the LASSO process uses shrinkage attributes, and the selected variables are more stable (37). A nomogram is a statistical model developed to maximize prediction accuracy. The tool shows advantages in individualized prognosis prediction of various autoimmune diseases (38). However, most nomograms established for RA patients thus far mainly focus on the prediction of recurrence or sustained remission by clinical characteristics. In this study, we established a nomogram based on combined inflammatory indicators and disease activity to provide a personalized prediction for patients treated with csDMARDs. In addition to inflammatory indicators, the nomogram also included 2 other independent indicators for evaluating disease activity, namely HAQ and DAS28-ESR, to predict the remission rate. Similar additions have been reported by other teams (39). The nomogram showed strong discrimination and considerable stability in the first remission time of patients. It is a simple, useful, and reliable prognostic tool.
This study had several limitations. First, unlike in randomized controlled trials, selection bias is inevitable in this real-world analysis. Although the baseline disease activity and therapeutic drugs of the development and the validation cohorts are matched, the possibility that other potential confounding factors may affect the outcome measurement cannot be ruled out. Importantly, many patients did not strictly follow the visit schedule in the study. Second, this was a single-center study with a relatively small sample size and a lack of external validation. Further research will require a larger sample cohort and multicenter validation. Additionally, these inflammatory factors or nomograms cannot predict sustained remission, nor can they make recommendations for the selection of csDMARDs. More studies are needed to clarify the value of inflammatory factor levels in the choice of treatment options for RA.
Conclusions
In conclusion, we studied the expression of inflammatory factors in the plasma of RA patients. The prognostic value of serum inflammatory factors has been shown not only in predicting the remission rate of csDMARDs treatment, but has also been applied to the personalized prediction of remission time to provide better clinical decision-making. These data support the valuable potential of IL-6 and Gal-9 levels in autoimmune diseases and show that evaluating the level and balance of inflammatory factor levels can provide useful information for accurate prognosis prediction of drug treatment of csDMARDs.
Baseline IL-6 and Gal-9 levels can predict the first remission time after treatment of csDMARDs. Biomarkers need to be combined with patients’ clinical symptoms and objective indicators to measure patients’ response to treatment.
In this study, we verified the prognostic value of cytokines such as Gal-9, IL-6 and some indicators that can reflect the disease activity, and we built a predictive nomogram in the RA patients. The nomogram had a strong predictive ability for the remission rate of patients in 3, 6 and 12 months, which is helpful to facilitate the identification of high-risk patients early and intervene with them as soon as possible.
Acknowledgments
Our gratitude goes first to the Chinese Rheumatism Data center (CRDC) with Chinese Rheumatology Information platform (CRIP) developed by Professor Xiaofeng Zeng, Department of Rheumatology, Peking Union Medical College Hospital, Chinese Academy of Medical Sciences & Peking Union Medical College, Key laboratory of Rheumatology and Clinical Immunology, for establishing a nationwide RA register system for real word study and follow-up.
Funding: This study was supported by ECCM Program of Clinical Research Center of Shandong University (2021SDUCRCB010) and Shandong Provincial Natural Science Foundation (ZR2022MH177).
Footnote
Reporting Checklist: The authors have completed the TRIPOD reporting checklist. Available at https://atm.amegroups.com/article/view/10.21037/atm-22-5791/rc
Data Sharing Statement: Available at https://atm.amegroups.com/article/view/10.21037/atm-22-5791/dss
Conflicts of Interest: All authors have completed the ICMJE uniform disclosure form (available at https://atm.amegroups.com/article/view/10.21037/atm-22-5791/coif). The authors have no conflicts of interest to declare.
Ethical Statement: The authors are accountable for all aspects of the work, including ensuring that any questions related to the accuracy or integrity of any part of the work have been appropriately investigated and resolved. All patients who participated in this study signed an informed consent form, and this study was approved by the Human Research Ethics Committees of Qilu Hospital (KYLL-2015-269; 2016072). The study was conducted in accordance with the Declaration of Helsinki (as revised in 2013).
Open Access Statement: This is an Open Access article distributed in accordance with the Creative Commons Attribution-NonCommercial-NoDerivs 4.0 International License (CC BY-NC-ND 4.0), which permits the non-commercial replication and distribution of the article with the strict proviso that no changes or edits are made and the original work is properly cited (including links to both the formal publication through the relevant DOI and the license). See: https://creativecommons.org/licenses/by-nc-nd/4.0/.
References
- Radner H, Smolen JS, Aletaha D. Comorbidity affects all domains of physical function and quality of life in patients with rheumatoid arthritis. Rheumatology (Oxford) 2011;50:381-8. [Crossref] [PubMed]
- Crowson CS, Rollefstad S, Ikdahl E, et al. Impact of risk factors associated with cardiovascular outcomes in patients with rheumatoid arthritis. Ann Rheum Dis 2018;77:48-54. [Crossref] [PubMed]
- Aletaha D, Nell VP, Stamm T, et al. Acute phase reactants add little to composite disease activity indices for rheumatoid arthritis: validation of a clinical activity score. Arthritis Res Ther 2005;7:R796-806. [Crossref] [PubMed]
- Singh JA, Furst DE, Bharat A, et al. 2012 update of the 2008 American College of Rheumatology recommendations for the use of disease-modifying antirheumatic drugs and biologic agents in the treatment of rheumatoid arthritis. Arthritis Care Res (Hoboken) 2012;64:625-39. [Crossref] [PubMed]
- Paccou J, Wendling D. Current treatment of psoriatic arthritis: update based on a systematic literature review to establish French Society for Rheumatology (SFR) recommendations for managing spondyloarthritis. Joint Bone Spine 2015;82:80-5. [Crossref] [PubMed]
- Smolen JS, Breedveld FC, Burmester GR, et al. Treating rheumatoid arthritis to target: 2014 update of the recommendations of an international task force. Ann Rheum Dis 2016;75:3-15. [Crossref] [PubMed]
- Gazitt T, Oren S, Reitblat T, et al. Treat-to-target concept implementation for evaluating rheumatoid arthritis patients in daily practice. Eur J Rheumatol 2019;6:136-41. [Crossref] [PubMed]
- Noack M, Miossec P. Selected cytokine pathways in rheumatoid arthritis. Semin Immunopathol 2017;39:365-83. [Crossref] [PubMed]
- Feldmann M, Maini RN. Lasker Clinical Medical Research Award. TNF defined as a therapeutic target for rheumatoid arthritis and other autoimmune diseases. Nat Med 2003;9:1245-50. [Crossref] [PubMed]
- Wiersma VR, de Bruyn M, Helfrich W, et al. Therapeutic potential of Galectin-9 in human disease. Med Res Rev 2013;33:E102-26. [Crossref] [PubMed]
- Shu Q, Amin MA, Ruth JH, et al. Suppression of endothelial cell activity by inhibition of TNFα. Arthritis Res Ther 2012;14:R88. [Crossref] [PubMed]
- Steelman AJ, Smith R 3rd, Welsh CJ, et al. Galectin-9 protein is up-regulated in astrocytes by tumor necrosis factor and promotes encephalitogenic T-cell apoptosis. J Biol Chem 2013;288:23776-87. [Crossref] [PubMed]
- Sun J, Sui Y, Wang Y, et al. Galectin-9 expression correlates with therapeutic effect in rheumatoid arthritis. Sci Rep 2021;11:5562. [Crossref] [PubMed]
- Mahtani KR, Miller A, Rivero-Arias O, et al. Autoimmune markers for the diagnosis of rheumatoid arthritis in primary care: primary care diagnostic technology update. Br J Gen Pract 2013;63:553-4. [Crossref] [PubMed]
- Sun F, Wang H, Zhang D, et al. One-year renal outcome in lupus nephritis patients with acute kidney injury: a nomogram model. Rheumatology (Oxford) 2022;61:2886-93. [Crossref] [PubMed]
- Xu D, Zhu L, Cai R, et al. A multi-predictor model to predict risk of scleroderma renal crisis in systemic sclerosis: a multicentre, retrospective, cohort study. Clin Exp Rheumatol 2021;39:721-6. [Crossref] [PubMed]
- Yin R, Wang G, Yang X, et al. Identification of prognostic factors and construction of a nomogram for patients with relapse/refractory adult-onset Still's disease. Clin Rheumatol 2021;40:3951-60. [Crossref] [PubMed]
- Hu XL, Gu Y, Wu DL, et al. A nomogram to predict recurrence of RA patients in clinical remission within one year. Eur Rev Med Pharmacol Sci 2020;24:9797-806. [Crossref] [PubMed]
- Alivernini S, Tolusso B, Gessi M, et al. Inclusion of Synovial Tissue-Derived Characteristics in a Nomogram for the Prediction of Treatment Response in Treatment-Naive Rheumatoid Arthritis Patients. Arthritis Rheumatol 2021;73:1601-13. [Crossref] [PubMed]
- Aletaha D, Smolen JS. Diagnosis and Management of Rheumatoid Arthritis: A Review. JAMA 2018;320:1360-72. [Crossref] [PubMed]
- Atar D, Birkeland KI, Uhlig T. 'Treat to target': moving targets from hypertension, hyperlipidaemia and diabetes to rheumatoid arthritis. Ann Rheum Dis 2010;69:629-30. [Crossref] [PubMed]
- Smolen JS. Treat to Target in Rheumatology: A Historical Account on Occasion of the 10th Anniversary. Rheum Dis Clin North Am 2019;45:477-85. [Crossref] [PubMed]
- Smolen JS, Landewé R, Bijlsma J, et al. EULAR recommendations for the management of rheumatoid arthritis with synthetic and biological disease-modifying antirheumatic drugs: 2016 update. Ann Rheum Dis 2017;76:960-77. [Crossref] [PubMed]
- Stoffer MA, Schoels MM, Smolen JS, et al. Evidence for treating rheumatoid arthritis to target: results of a systematic literature search update. Ann Rheum Dis 2016;75:16-22. [Crossref] [PubMed]
- Wehr P, Purvis H, Law SC, et al. Dendritic cells, T cells and their interaction in rheumatoid arthritis. Clin Exp Immunol 2019;196:12-27. [Crossref] [PubMed]
- Nygaard G, Firestein GS. Restoring synovial homeostasis in rheumatoid arthritis by targeting fibroblast-like synoviocytes. Nat Rev Rheumatol 2020;16:316-33. [Crossref] [PubMed]
- Buch MH, Eyre S, McGonagle D. Persistent inflammatory and non-inflammatory mechanisms in refractory rheumatoid arthritis. Nat Rev Rheumatol 2021;17:17-33. [Crossref] [PubMed]
- Brown KD, Claudio E, Siebenlist U. The roles of the classical and alternative nuclear factor-kappaB pathways: potential implications for autoimmunity and rheumatoid arthritis. Arthritis Res Ther 2008;10:212. [Crossref] [PubMed]
- Santos LL, Morand EF. The role of macrophage migration inhibitory factor in the inflammatory immune response and rheumatoid arthritis. Wien Med Wochenschr 2006;156:11-8. [Crossref] [PubMed]
- Zarkesh M, Sedaghat F, Heidarzadeh A, et al. Diagnostic value of IL-6, CRP, WBC, and absolute neutrophil count to predict serious bacterial infection in febrile infants. Acta Med Iran 2015;53:408-11.
- Sakyi SA, Buckman TA, Antwi-Berko D, et al. Intracytoplasmic Expression of IL-6 and IL-17A in Circulating CD4+ T Cells Are Strongly Associated with and Predict Disease Activity in Rheumatoid Arthritis: A Case-Control Study in Ghana. Int J Rheumatol 2020;2020:2808413. [Crossref] [PubMed]
- Anderson AE, Pratt AG, Sedhom MA, et al. IL-6-driven STAT signalling in circulating CD4+ lymphocytes is a marker for early anticitrullinated peptide antibody-negative rheumatoid arthritis. Ann Rheum Dis 2016;75:466-73. [Crossref] [PubMed]
- Gieseke F, Kruchen A, Tzaribachev N, et al. Proinflammatory stimuli induce galectin-9 in human mesenchymal stromal cells to suppress T-cell proliferation. Eur J Immunol 2013;43:2741-9. [Crossref] [PubMed]
- Wang Y, Song L, Sun J, et al. Expression of Galectin-9 and correlation with disease activity and vascular endothelial growth factor in rheumatoid arthritis. Clin Exp Rheumatol 2020;38:654-61.
- Kokkonen H, Söderström I, Rocklöv J, et al. Up-regulation of cytokines and chemokines predates the onset of rheumatoid arthritis. Arthritis Rheum 2010;62:383-91. [Crossref] [PubMed]
- Moar P, Tandon R. Galectin-9 as a biomarker of disease severity. Cell Immunol 2021;361:104287. [Crossref] [PubMed]
- Fernández-Delgado M, Sirsat MS, Cernadas E, et al. An extensive experimental survey of regression methods. Neural Netw 2019;111:11-34. [Crossref] [PubMed]
- Song X, Fan Y, Jia Y, et al. A novel aGAPSS-based nomogram for the prediction of ischemic stroke in patients with antiphospholipid syndrome. Front Immunol 2022;13:930087. [Crossref] [PubMed]
- Doumen M, De Cock D, Pazmino S, et al. Treatment response and several patient-reported outcomes are early determinants of future self-efficacy in rheumatoid arthritis. Arthritis Res Ther 2021;23:269. [Crossref] [PubMed]
(English Language Editor: J. Jones)