Predictive value of the serum anion gap for 28-day in-hospital all-cause mortality in sepsis patients with acute kidney injury: a retrospective analysis of the MIMIC-IV database
Highlight box
Key findings
• Anion gap (AG) levels can predict the death risk of patients with sepsis-associated acute kidney injury (SA-AKI) during hospitalization.
What is known and what is new?
• The case fatality rate of SA-AKI is high.
• The risk of death of SA-AKI patients within 28 days during hospitalization in the ICU was increased when AG ≥14 mmol/L. The relationship between AG level and the risk of death of SA-AKI patients during hospitalization was S-shaped.
What is the implication, and what should change now?
• Clinicians should pay attention to the specific AG detection value, rather than just whether it is in the normal range.
Introduction
Sepsis is an immune reaction disorder syndrome in the body following bacterial invasion of the blood circulation, which causes a systemic inflammatory reaction. It is a common complication in severe trauma, burn, shock, infection, and major surgery (1), and the main symptoms are fever, shortness of breath, and changes in the mental state. Severe sepsis patients often present with circulatory disorders and (or) organ dysfunction (2). Sepsis, severe sepsis, and septic shock are three manifestations of the severity of sepsis. If further developed, these cases can lead to life-threatening organ dysfunction, which is one of the main factors leading to the death of severe sepsis patients (1,3).
The kidney is one of the most vulnerable organs in sepsis patients, which mainly manifests as sepsis-associated acute kidney injury (SA-AKI); that is, creatinine increases to 1.5 times or more than the basic level within 7 days, creatinine increases ≥26.5 within 48 hours µmol/L, or urine volume <0.5 mL/(kg·h) for 6 hours (4). According to relevant data from the United States, Adhikari et al. estimated that there are about 19 million cases of sepsis globally every year (5). At least one of every three sepsis patients has SA-AKI. According to this estimate, there are approximately six million cases of SA-AKI worldwide annually (i.e., one case of SA-AKI per 1,000 people) (6). However, the actual number may be higher. It is estimated that there are about 4.2 million SA-AKI cases in the United States each year and about 98 million SA-AKIs worldwide (7). For patients receiving treatment in the intensive care unit (ICU), 40–50% of patients with acute kidney injury (AKI) have sepsis (7), and about 47.1–51% of sepsis patients have AKI (8,9). The case fatality rate of SA-AKI is also high, about 20.9–56.8% (10). Thus, predicting SA-AKI-related death risk has important clinical significance (11). Previous studies showed that some factors were associated with the death risk in SA-AKI patients, including age, related scores of critical illness, mechanical ventilation (7,8,10,11).
The serum anion gap (AG), as an easy to obtained and important clinical indicator to evaluate the acid-base imbalance in the body, mainly reflects the difference between undetected cations and undetected anions in human plasma. Physiologically active ions are proteins, lactates, acetoacetates, sulfates, and other anions. At present, the pathogenesis of serum AG in sepsis is unclear. On the one hand, it is believed that sepsis can lead to abnormal energy metabolism, accompanied by changes in hemodynamics, and subsequently lead to microcirculation dysfunction under the condition of systemic inflammatory reaction (12,13). Insufficient tissue perfusion in sepsis patients will lead to different degrees of hypoxia, reduce the use of oxygen in tissues, accelerate the rate of glycolysis, and increase the production of Lac in the body. On the other hand, sepsis can result in high decomposition under persistent inflammation and immunosuppression, and the production of proteins such as α-ketoacids increases. In addition, body tissues are destroyed in large amounts due to persistent inflammation, leading to the decomposition of a series of substances. These acidic metabolites can consume the buffer HCO3– in the blood and produce anions such as lactate, acetoacetate, and sulfate, which will lead to increased AG (13,14). However, the specific mechanism still requires further research and exploration. At present, some studies have confirmed that serum AG can predict the prognosis of critically ill patients (15,16).
The Medical Information Mart for Intensive Care (MIMIC) database is one of the most important databases for research into critical care medicine. So far, it has been updated to the fourth edition, MIMIC-IV. Researchers have performed significant deep mining on the database and obtained several important research results. This study investigated the predictive effect of the AG on the in-hospital mortality risk of SA-AKI patients based on data obtained from the MIMIC-IV database. We present the following article in accordance with the TRIPOD reporting checklist (available at https://atm.amegroups.com/article/view/10.21037/atm-22-5916/rc).
Methods
Study population
This is a retrospective study involving data obtained from the MIMIC-IV (v0.4) database. This database collected the clinical information of more than 60,000 patients hospitalized in the ICU of Beth Israel Dikang Medical Center from 2008 to 2019.
The subjects of this study were sepsis patients who were admitted in the ICU. The inclusion criteria were as follows: (I) adult patients (age ≥18); (II) first stay in ICU (for those who had stayed in the ICU several times, only the first stay information was recorded); (III) first-time diagnosis of multiple organ dysfunction syndrome (MODS); and (IV) stay time in the ICU ≥24 hours. Patients were excluded based on the following criteria: (I) lack of key information, such as the severe illness score; and (II) discharged automatically. The study was conducted in accordance with the Declaration of Helsinki (as revised in 2013).
Data extraction
We used the Structured Query Language (SQL) to acquire the following clinical information from the MIMIC-IV database: demographic variables, laboratory test results including blood routine, liver function, renal function, blood lipid, blood sugar, serum electrolytes (potassium, sodium, calcium); AG; blood HCO3–; sequential organ failure assessment (SOFA); length of stay in the ICU; death in the ICU; complications; the utility of mechanical ventilation, vasoactive drugs, and renal replacement therapy (RRT) during the ICU hospitalization; and major adverse events such as ventilator-associated pneumonia (VAP), urinary tract infection (UTI), diabetes ketoacidosis (DKA), and acute myocardial infarction (AMI). All laboratory test parameters only included the data generated within the first 24 hours after the patient entered the ICU (i.e., baseline value) and the extreme value [i.e., maximum value (max) and minimum value (min)] during hospitalization in the ICU.
Outcome
The main purpose of this study was to analyze the impact of AG on the short-term prognosis of SA-AKI patients after admission to the ICU. Therefore, the primary end point of observation was the mortality rate within 28 days after admission to the ICU.
Statistical analysis
SPSS software (version 23.0, IBM, Chicago, USA) was used for statistical analysis. Continuous variables were expressed as the mean [standard deviation (SD)], and comparisons between the two groups were conducted using an independent sample t-test. Categorical variables were expressed as the quantity (percentage), and comparisons between the two groups were conducted using the χ2 test. Univariate and multivariate logistic regression analyses were performed to analyze the risk factors related to the death of SA-AKI patients within 28 days during hospitalization in the ICU. A two-sided P<0.05 was considered statistically significant.
Results
General information
According to the inclusion and exclusion criteria, we included 3,684 SA-AKI patients from the MIMIC-IV database in the final analysis (Table 1). According to the AG level, they were divided into low and high AG groups (Table 2). The AG of patients in the low AG group was <18 mmol/L, and that of patients in the high AG group was ≥18 mmol/L. There were 3,305 cases in the low AG group, including 1,967 women (59.5%), and 379 cases in the high AG group, of which 217 were female (57.3%). The Simplified Acute Physiology Score (SAPS) [19.0 (17.0, 22.0) vs. 21.0 (18.0, 24.0), P<0.001] and SOFA [5.0 (4.0, 7.0) vs. 6.0 (4.0, 8.0), P<0.001] scores of patients with low AG were lower than those in patients with high AG. Patients in the low AG group received mechanical ventilation (88.0% vs. 82.1%, P=0.001) significantly more frequently than those in the high AG group, while their rates of dobutamine (1.1% vs. 3.2%, P=0.003) and norepinephrine (22.8% vs. 30.6%, P=0.001) use were markedly lower than those in the high AG group. These results suggest that the condition of patients in the low AG group was not as serious as that in the high AG group (Tables 1,2). A total of 497 patients (13.5%) died during hospitalization, including 376 cases (11.4%) in the low AG group and 121 cases (31.9%) in the high AG group. The mortality rate in the low AG group was considerably lower than that in the high AG group (P<0.001, Table 2).
Table 1
Variables | Total (n=3,684) |
---|---|
Age (years), mean ± SD | 65.4±16.6 |
Gender, n (%) | |
Female | 2,184 (59.3) |
Male | 1,500 (40.7) |
BMI (kg/m2), mean ± SD | 27.4±5.3 |
Death in-hospital, n (%) | 497 (13.5) |
First care unit, n (%) | |
CCU | 1,151 (31.2) |
NICU | 1,939 (52.6) |
MICU/SICU | 170 (4.6) |
TSICU | 424 (11.5) |
Ethnicity, n (%) | |
Asian | 119 (3.2) |
Black | 269 (7.3) |
Hispanic | 102 (2.8) |
White | 2,439 (66.2) |
Other | 755 (20.5) |
Heart rate (beat/min), mean ± SD | 89.4±16.0 |
SBP (mmHg), mean ± SD | 119.0±15.6 |
Respiratory rate (times/min), mean ± SD | 21.0±4.4 |
Temperature (℃), Mean ± SD | 37.0±0.6 |
SpO2 (%), mean ± SD | 96.0±2.8 |
ICU days, median (IQR) | 4.0 (2.3, 7.9) |
Hospital days, median (IQR) | 10.1 (6.5, 16.9) |
RRT, n (%) | 56 (1.5) |
Ventilation, n (%) | 3,220 (87.4) |
Dobutamine, n (%) | 48 (1.3) |
dopamine, n (%) | 121 (3.3) |
Epinephrine, n (%) | 210 (5.7) |
Norepinephrine, n (%) | 871 (23.6) |
SOFA, ICU first day, median (IQR) | 5.0 (3.0, 7.0) |
APSIII, ICU first day, median (IQR) | 49.0 (36.0, 67.0) |
Comorbidities, n (%) | |
Myocardial infarction | 656 (17.8) |
Congestive heart failure | 1,100 (29.9) |
Cerebrovascular disease | 612 (16.6) |
Chronic pulmonary disease | 989 (26.8) |
Mild liver disease | 483 (13.1) |
Severe liver disease | 221 (6.0) |
Renal disease | 625 (17.0) |
Malignant cancer | 471 (12.8) |
Laboratory parameters | |
Anion gap (mmol/L), mean ± SD | 13.6±3.2 |
Bicarbonate (mmHg), median (IQR) | 23.0 (21.0, 26.0) |
BUN (mmol/L), median (IQR) | 18.0 (12.0, 29.0) |
Calcium (mmol/L), median (IQR) | 8.2 (7.8, 8.6) |
Chloride (mmol/L), median (IQR) | 106.0 (102.0, 109.0) |
Creatinine (μmol/L), median (IQR) | 0.9 (0.7, 1.3) |
Glucose (mmol/L), median (IQR) | 126.0 (107.0, 153.2) |
Sodium (mmol/L), median (IQR) | 139.0 (136.0, 142.0) |
Potassium (mmol/L), median (IQR) | 4.1 (3.8, 4.4) |
WBC (109/L), median (IQR) | 11.4 (8.5, 15.0) |
INR, median (IQR) | 1.3 (1.2, 1.4) |
PT (s), median (IQR) | 14.2 (13.3, 15.3) |
PTT (s), median (IQR) | 31.0 (28.6, 35.0) |
Hematocrit, median (IQR) | 30.8 (27.4, 34.7) |
Hemoglobin (g/L), median (IQR) | 10.3 (9.1, 11.6) |
Platelet (109/L), median (IQR) | 172.0 (123.0, 237.0) |
RBC (1012/L), median (IQR) | 3.4 (3.0, 3.9) |
RDW, median (IQR) | 14.6 (13.5, 16.0) |
Magnesium (mmol/L), median (IQR) | 2.1 (1.9, 2.3) |
Phosphate (mmol/L), median (IQR) | 3.2 (2.7, 3.8) |
BMI, body mass index; SD, standard deviation; CCU, critical care unit; NICU, neurologic intensive care unit; MICU, medical intensive care unit; SICU, surgical intensive care unit; TSICU, transplantation surgical intensive care unit; SBP, systolic blood pressure; SpO2, pulse saturation of oxygen; ICU, intensive care unit; IQR, interquartile range; RRT, renal replacement therapy; SOFA, sequential organ failure assessment; APSIII, acute physiological score; BUN, blood urea nitrogen; WBC, white blood cell; INR, international normalization ratio; PT, prothrombin time; PTT, partial thrombin time; RBC, red blood cell; RDW, red blood cell distribution width.
Table 2
Characteristics | AG <18, mmol/L (n=3,305) | AG ≥18, mmol/L (n=379) | P value | Test |
---|---|---|---|---|
LOS ICU day, median [Q1, Q3] | 4.0 [2.3, 7.9] | 4.4 [2.8, 7.4] | 0.046 | Kruskal-Wallis |
LOS hospital day, median [Q1, Q3] | 10.0 [6.5, 16.8] | 10.3 [6.6, 17.9] | 0.700 | Kruskal-Wallis |
Female, n (%) | 1,967 (59.5) | 217 (57.3) | 0.428 | Chi-squared |
Age (years), median [Q1, Q3] | 67.0 [55.0, 78.0] | 69.0 [56.5, 81.0] | 0.002 | Kruskal-Wallis |
BMI (kg/m2), median [Q1, Q3] | 26.8 [25.4, 28.8] | 26.8 [24.3, 26.8] | 0.004 | Kruskal-Wallis |
Death during hospitalization, n (%) | 376 (11.4) | 121 (31.9) | <0.001 | Chi-squared |
Mean anion gap (mmol/L), median [Q1, Q3] | 13.0 [11.0, 15.0] | 19.0 [18.0, 20.0] | <0.001 | Kruskal-Wallis |
AKI stage, n (%) | 0.631 | Chi-squared | ||
1 | 2787 (84.3) | 313 (82.6) | ||
2 | 463 (14.0) | 58 (15.3) | ||
3 | 55 (1.7) | 8 (2.1) | ||
APSIII ICU first day, median [Q1, Q3] | 48.0 [36.0, 66.0] | 57.0 [45.0, 73.5] | <0.001 | Kruskal-Wallis |
SAPS ICU first day, median [Q1, Q3] | 19.0 [17.0, 22.0] | 21.0 [18.0, 24.0] | <0.001 | Kruskal-Wallis |
SOFA sepsis first day, median [Q1, Q3] | 5.0 [4.0, 7.0] | 6.0 [4.0, 8.0] | <0.001 | Kruskal-Wallis |
CCI, median [Q1, Q3] | 5.0 [4.0, 7.0] | 6.0 [4.0, 9.0] | <0.001 | Kruskal-Wallis |
Myocardial infarct, n (%) | 575 (17.4) | 81 (21.4) | 0.065 | Chi-squared |
Congestive heart failure, n (%) | 945 (28.6) | 155 (40.9) | <0.001 | Chi-squared |
Peripheral vascular disease, n (%) | 385 (11.6) | 39 (10.3) | 0.484 | Chi-squared |
Cerebrovascular disease, n (%) | 546 (16.5) | 66 (17.4) | 0.711 | Chi-squared |
Dementia, n (%) | 136 (4.1) | 16 (4.2) | 0.970 | Chi-squared |
Chronic pulmonary disease, n (%) | 891 (27.0) | 98 (25.9) | 0.691 | Chi-squared |
Rheumatic disease, n (%) | 111 (3.4) | 11 (2.9) | 0.750 | Chi-squared |
Peptic ulcer disease, n (%) | 80 (2.4) | 22 (5.8) | <0.001 | Chi-squared |
Mild liver disease, n (%) | 409 (12.4) | 74 (19.5) | <0.001 | Chi-squared |
Paraplegia, mean (SD) | 0.1 (0.2) | 0.1 (0.3) | 0.539 | T-test |
Renal disease, n (%) | 502 (15.2) | 123 (32.5) | <0.001 | Chi-squared |
Malignant cancer, n (%) | 415 (12.6) | 56 (14.8) | 0.253 | Chi-squared |
Severe liver disease, n (%) | 185 (5.6) | 36 (9.5) | 0.004 | Chi-squared |
Metastatic solid tumor, n (%) | 209 (6.3) | 30 (7.9) | 0.279 | Chi-squared |
AIDS, n (%) | 35 (1.1) | 0 | 0.045 | Fisher’s exact |
RRT, n (%) | 56 (1.7) | 0 | 0.020 | Chi-squared |
Ventilation, n (%) | 2909 (88.0) | 311 (82.1) | 0.001 | Chi-squared |
Dobutamine, n (%) | 36 (1.1) | 12 (3.2) | 0.003 | Fisher’s exact |
Dopamine, n (%) | 105 (3.2) | 16 (4.2) | 0.353 | Chi-squared |
Epinephrine, n (%) | 197 (6.0) | 13 (3.4) | 0.058 | Chi-squared |
Norepinephrine, n (%) | 755 (22.8) | 116 (30.6) | 0.001 | Chi-squared |
Mean heart rate (beat/min), median [Q1, Q3] | 88.0 [78.0, 100.0] | 87.0 [77.0, 99.0] | 0.220 | Kruskal-Wallis |
Mean SBP (mmHg), median [Q1, Q3] | 117.0 [108.0, 128.0] | 117.0 [109.0, 128.0] | 0.953 | Kruskal-Wallis |
Mean respiratory rate (times/min), median [Q1, Q3] | 20.0 [18.0, 23.0] | 20.0 [18.0, 23.0] | 0.780 | Kruskal-Wallis |
Mean temperature (℃), median [Q1, Q3] | 36.9 [36.7, 37.3] | 36.9 [36.7, 37.3] | 0.212 | Kruskal-Wallis |
Mean SpO2, (%) median [Q1, Q3] | 96.0 [95.0, 98.0] | 96.0 [95.0, 98.0] | 0.747 | Kruskal-Wallis |
Urine output sum (mL), median [Q1, Q3] | 2,200.0 [1,541.0, 3,055.0] | 2,095.0 [1,445.0, 2,990.0] | 0.188 | Kruskal-Wallis |
Mean bicarbonate (mmHg), median [Q1, Q3] | 24.0 [21.0, 26.0] | 20.0 [18.0, 23.0] | <0.001 | Kruskal-Wallis |
Mean BUN (mmol/L), median [Q1, Q3] | 17.0 [12.0, 27.0] | 34.0 [20.0, 57.0] | <0.001 | Kruskal-Wallis |
Mean calcium (mmol/L), median [Q1, Q3] | 8.2 [7.8, 8.5] | 8.3 [7.8, 8.9] | <0.001 | Kruskal-Wallis |
Mean chloride (mmol/L), median [Q1, Q3] | 106.0 [102.0, 109.0] | 103.0 [98.0, 107.0] | <0.001 | Kruskal-Wallis |
Mean creatinine (μmol/L), median [Q1, Q3] | 0.9 [0.7, 1.2] | 1.4 [1.0, 2.2] | <0.001 | Kruskal-Wallis |
Mean glucose (mmol/L), median [Q1, Q3] | 125.0 [106.0, 150.0] | 145.0 [115.0, 191.5] | <0.001 | Kruskal-Wallis |
Mean sodium (mmol/L), median [Q1, Q3] | 139.0 [136.0, 142.0] | 139.0 [135.0, 142.0] | 0.047 | Kruskal-Wallis |
Mean potassium (mmol/L), median [Q1, Q3] | 4.1 [3.8, 4.4] | 4.1 [3.7, 4.5] | 0.077 | Kruskal-Wallis |
Mean WBC (109/L), median [Q1, Q3] | 11.4 [8.4, 14.7] | 12.6 [8.9, 17.6] | <0.001 | Kruskal-Wallis |
Mean INR, median [Q1, Q3] | 1.3 [1.2, 1.4] | 1.3 [1.2, 1.7] | <0.001 | Kruskal-Wallis |
Mean PT (s), median [Q1, Q3] | 14.2 [13.2, 15.1] | 14.3 [13.4, 18.4] | <0.001 | Kruskal-Wallis |
Mean PTT (s), median [Q1, Q3] | 31.0 [28.6, 34.4] | 31.0 [29.2, 42.3] | <0.001 | Kruskal-Wallis |
Mean hematocrit, median [Q1, Q3] | 30.8 [27.5, 34.6] | 31.0 [26.4, 36.2] | 0.692 | Kruskal-Wallis |
Mean hemoglobin (g/L), median [Q1, Q3] | 10.3 [9.1, 11.5] | 10.3 [8.5, 12.0] | 0.730 | Kruskal-Wallis |
Mean platelet (109/L), median [Q1, Q3] | 172.0 [124.0, 235.0] | 177.0 [114.0, 257.5] | 0.390 | Kruskal-Wallis |
Mean RBC (1012/L), median [Q1, Q3] | 3.4 [3.0, 3.8] | 3.4 [2.9, 4.1] | 0.454 | Kruskal-Wallis |
Mean RDW, median [Q1, Q3] | 14.5 [13.5, 15.8] | 15.0 [14.1, 17.1] | <0.001 | Kruskal-Wallis |
Mean magnesium (mmol/L), median [Q1, Q3] | 2.1 [1.9, 2.3] | 2.1 [1.9, 2.3] | 0.013 | Kruskal-Wallis |
Mean phosphate (mmol/L), median [Q1, Q3] | 3.2 [2.7, 3.7] | 3.7 [3.1, 4.7] | <0.001 | Kruskal-Wallis |
AG, anion gap; LOS, length of stay; ICU, intensive care unit; BMI, body mass index; AKI, acute kidney injury; APSIII, acute physiological score; SAPS, simplified acute physiology score; SOFA, sequential organ failure assessment; CCI, Charlson comorbidity index; SD, standard deviation; AIDS, acquired immune deficiency syndrome; RRT, renal replacement therapy; SBP, systolic blood pressure; SpO2, pulse saturation of oxygen; BUN, blood urea nitrogen; WBC, white blood cell; INR, international normalization ratio; PT, prothrombin time; PTT, partial thrombin time; RBC, red blood cell; RDW, red blood cell distribution width.
Univariate analysis of the risk factors for death in SA-AKI patients during hospitalization
The univariate analysis results showed that multiple factors were related to the death of SA-AKI patients during hospitalization. The increase of AG elevated the risk of death of SA-AKI patients during hospitalization, as did the combination of underlying diseases (including chronic heart failure, cerebrovascular disease, liver disease, renal disease, and cancer). Furthermore, the use of adrenaline, increased creatinine levels, and the increase of international normalized ration (INR), increased red blood cell distribution width, and increased phosphorus levels also increased the risk of death in these patients. However, higher body mass index (BMI) and elevated bicarbonate levels may reduce the risk of death of SA-AKI patients during hospitalization. Some factors, including age, urea nitrogen, and prothrombin time (PT), were statistically related to the risk of death, but the clinical significance of their odds ratio (OR) values was very small (Table 3). After adjusting for some risk factors, increased AG still increased the death risk of SA-AKI patients during hospitalization (Table 4).
Table 3
Variables | In-hospital death OR (95% CI) | P |
---|---|---|
Age (years) | 1.0 (1.0, 1.0) | <0.001 |
Gender | ||
Female | 1 | |
Male | 1.1 (0.9, 1.4) | 0.181 |
BMI (kg/m2) | 0.9 (0.9, 1.0) | <0.001 |
Anion gap (mmol/L) | 1.2 (1.2, 1.3) | <0.001 |
Myocardial infarct | 1.1 (0.9, 1.4) | 0.413 |
Congestive heart failure | 1.2 (1.0, 1.5) | 0.039 |
Cerebrovascular disease | 1.5 (1.2, 1.8) | 0.002 |
Chronic pulmonary disease | 1.2 (1.0, 1.5) | 0.072 |
Mild liver disease | 1.7 (1.3, 2.2) | <0.001 |
Severe liver disease | 2.0 (1.5, 2.8) | <0.001 |
Renal disease | 1.4 (1.1, 1.7) | 0.008 |
Malignant cancer | 2.6 (2.1, 3.3) | <0.001 |
RRT | 0.9 (0.4, 2.0) | 0.827 |
Ventilation | 0.8 (0.6, 1.1) | 0.222 |
Dobutamine | 1.5 (0.7, 3.1) | 0.286 |
Dopamine | 0.6 (0.3, 1.1) | 0.091 |
Epinephrine | 0.7 (0.5, 1.2) | 0.189 |
Norepinephrine | 1.3 (1.0, 1.6) | 0.020 |
Heart rate (beat/min) | 1.0 (1.0, 1.0) | 0.679 |
SpO2 (%) | 1.0 (1.0, 1.1) | 0.141 |
SBP (mmHg) | 1.0 (1.0, 1.0) | 0.833 |
Respiratory rate (times/min) | 1.0 (1.0, 1.0) | 0.845 |
Temperature (℃) | 0.9 (0.8, 1.1) | 0.26 |
Bicarbonate (mmHg) | 0.9 (0.9, 0.9) | <0.001 |
BUN (mmol/L) | 1.0 (1.0, 1.0) | <0.001 |
Calcium (mmol/L) | 1.0 (0.9, 1.2) | 0.574 |
Chloride (mmol/L) | 1.0 (1.0, 1.0) | 0.06 |
Creatinine (μmol/L) | 1.4 (1.3, 1.6) | <0.001 |
Glucose (mmol/L) | 1.0 (1.0, 1.0) | <0.001 |
Sodium (mmol/L) | 1.0 (1.0, 1.0) | 0.819 |
Potassium (mmol/L) | 1.0 (0.8, 1.2) | 1 |
WBC (109/L) | 1.0 (1.0, 1.0) | 0.193 |
INR | 1.2 (1.1, 1.4) | <0.001 |
PT (s) | 1.0 (1.0, 1.0) | <0.001 |
PTT (s) | 1.0 (1.0, 1.0) | 0.078 |
Hematocrit | 1.0 (1.0, 1.0) | 0.457 |
Hemoglobin (g/L) | 1.0 (1.0, 1.1) | 0.757 |
Platelet (109/L) | 1.0 (1.0, 1.0) | 0.872 |
RBC (1012/L) | 1.0 (0.9, 1.2) | 0.535 |
RDW | 1.1 (1.0, 1.1) | <0.001 |
Magnesium (mmol/L) | 1.1 (0.8, 1.3) | 0.612 |
Phosphate (mmol/L) | 1.2 (1.1, 1.3) | <0.001 |
OR, odds ratio; CI, confidence interval; BMI, body mass index; RRT, renal replacement therapy; SpO2, pulse saturation of oxygen; SBP, systolic blood pressure; BUN, blood urea nitrogen; WBC, white blood cell; INR, international normalization ratio; PT, prothrombin time; PTT, partial thrombin time; RBC, red blood cell; RDW, red blood cell distribution width.
Table 4
Anion gap | N | Crude | Adjusted | |||
---|---|---|---|---|---|---|
OR (95% CI) | P | OR (95% CI) | P | |||
≥18 mmol/L | 379 (10.3%) | 1.4 (1.3, 1.5) | <0.001 | 1.4 (1.3, 1.6) | <0.001 |
An 18 mmol/L threshold for anion gap existed for in-hospital death. Adjusted: age, gender, BMI, congestive heart failure, cerebrovascular disease, chronic pulmonary disease, mild liver disease, severe liver disease, renal disease, malignant cancer, dopamine, norepinephrine. OR, odds ratio; CI, confidence interval; BMI, body mass index.
Multivariate analysis of the risk factors for the death of SA-AKI patients during hospitalization
Multivariate analysis of the further grouping of AG levels showed that the risk of death during hospitalization was still increased among patients with an AG <18 mmol/L or an AG of 14–17 (OR =2.2, 95% CI: 1.6–2.9). After adjusting for age and gender, the OR was 2.0 (95% CI: 1.5–2.7), and after adjusting for more factors, the OR was 1.8 (95% CI: 1.3–2.6). No difference was observed in patients with an AG ≥18 mmol/L after further grouping (Table 5). The fitting analysis of AG and death risk showed that the level of AG had an S-shaped relationship with the death risk of SA-AKI patients during hospitalization. When the AG ≥14 mmol/L, the death risk of SA-AKI patients during hospitalization increased rapidly (Figure 1).
Table 5
Variable | Crude Model | Adjusted Model 1 | Adjusted Model 2 | |||||
---|---|---|---|---|---|---|---|---|
OR (95% CI) | P | OR (95% CI) | P | OR (95% CI) | P | |||
Anion gap <18, mmol/L | 1.2 (1.1, 1.2) | <0.001 | 1.2 (1.1, 1.2) | <0.001 | 1.1 (1.1, 1.2) | <0.001 | ||
6–11 | 1.0 | 1.0 | 1.0 | |||||
12–13 | 1.2 (0.8, 1.6) | 0.359 | 1.1 (0.8, 1.6) | 0.417 | 1.3 (0.9, 1.8) | 0.171 | ||
14–17 | 2.2 (1.6, 2.9) | <0.001 | 2.0 (1.5, 2.7) | <0.001 | 1.8 (1.3, 2.6) | <0.001 | ||
P for trend | <0.001 | <0.001 | <0.001 | |||||
Anion gap ≥18, mmol/L | 1.4 (1.3, 1.6) | <0.001 | 1.4 (1.3, 1.6) | <0.001 | 1.5 (1.3, 1.7) | <0.001 | ||
18 | 1.0 | 1.0 | 1.0 | |||||
19–30 | 1.6 (1.0, 2.5) | 0.052 | 1.6 (1.0, 2.5) | 0.052 | 1.6 (0.9, 2.7) | 0.116 | ||
P for trend | 0.052 | 0.052 | 0.116 |
Crude Model: no adjustment. Adjusted Model 1: age, gender. Adjusted Model 2: age, gender, BMI, congestive heart failure, cerebrovascular disease, chronic pulmonary disease, mild liver disease, severe liver disease, renal disease, malignant cancer, dopamine, norepinephrine, SBP, bicarbonate, bun, creatinine, glucose, PT, PTT, RDW, phosphate, SOFA, APSIII. OR, odds ratio; CI, confidence interval; BMI, body mass index; SBP, systolic blood pressure; PT, prothrombin time; PTT, partial thrombin time; RDW, red blood cell distribution width; SOFA, sequential organ failure assessment; APSIII, acute physiological score.
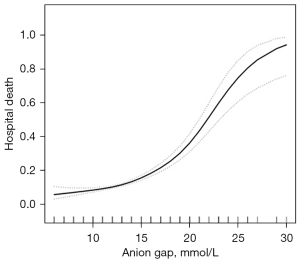
Discussion
In this study, we retrospectively analyzed the data of sepsis patients with acute kidney injury (SA-AKI) obtained from the MIMIC-IV database. A total of 3,684 patients were included based on the inclusion and exclusion criteria. The results showed that the 28-day mortality of patients in the low AG (<18 mmol/L) group was significantly lower than that in the high AG (≥18 mmol/L) group. After adjusting for other risk factors, the increase of AG was still a risk factor for the short-term death of SA-AKI patients. Further subgroup and curve fitting analyses of the AG level showed that when AG ≥14 mmol/L, the risk of short-term death of SA-AKI patients began to increase (Table 4, Figure 1). To our knowledge, this study is the first to analyze the risk factors related to short-term death of SA-AKI patients based on the MIMIC-IV database, and our findings suggested that when the AG was high in the normal range, the risk of death of patients might be increased. Evaluating the prognosis of patients and adjusting the treatment plan promptly is hugely significant in clinical practice.
In severe patients, sepsis and AKI significantly increased the risk of death. When sepsis is complicated with AKI, the risk of death of patients is further increased. The risk of death of SA-AKI patients is 1.48 times that of AKI patients caused by other factors, and the average hospital stay is also markedly prolonged (17). However, some patients have undergone active treatment, and their renal function can be substantially improved or even cured, while at the same time providing notable survival benefits. Some studies have shown that if renal function can be recovered within 24 hours after shock, the risk of death during hospitalization will be significantly reduced (18). However, it should be noted that the renal function of some patients will deteriorate again after recovery (19). Therefore, predicting the death risk of SA-AKI patients more specifically has important clinical significance. A previous study has conducted in-depth analyses of biomarkers related to SA-AKI, demonstrating that the plasma neutral gelatin associated lipocalin (pNGAL) level at discharge can predict the recovery potential of renal function in SA-AKI patients (20). A recent study showed that pNGAL can predict the risk of death of critically ill patients during hospitalization and ICU stay (21). However, for SA-AKI patients, it is unclear whether pNGAL can predict the risk of death. Other SA-AKI-related markers include urinary kidney injury molecule-1 (uKIM-1), urinary live type fat acid binding protein, urinary TIMP-2, and IGFBP7; however, there is no research on predicting the prognosis of SA-AKI patients (7). In the present study, AG was used to predict the prognosis of SA-AKI; the results showed that the AG level was related to the short-term mortality risk of patients.
There are numerous studies on predicting the prognosis of severe patients using the AG. Mitra et al. found that in the emergency department, the rate of patients with elevated AG requiring admission to the ICU and the mortality rate were significantly higher (22). In another cohort study, Mohr et al. found that the AG could predict the risk of death of septic patients during hospitalization (23). Some researchers believe that AG is weak in predicting prognosis, and studied the predictive value of albumin-corrected AG. Kim et al. found that the albumin-corrected AG for admission to the ICU can predict the mortality risk in children (24). Zhang et al. identified that the relationship between AG and 30-day all-cause mortality of severe patients with cardiogenic shock followed a J-shaped curve, and higher AG was associated with an increased risk of 30-, 90-, and 365-day all-cause mortality in these patients (25). In a recent retrospective propensity score-matching analysis, Hu et al. reported that albumin-corrected AG had a high predictive value for the risk of death of septic patients during hospitalization (26). In another albumin-corrected AG study, the authors observed that in critically ill AKI patients receiving continuous renal replacement therapy (CRRT), a higher albumin-corrected AG (>20 mmol/L) level at the beginning of CRRT was significantly associated with all-cause mortality in the ICU (27). In this study, we did not use albumin correction to simplify the use of AG.
In clinical practice, the normal range of AG is generally 8–16 mmol/L, and a study has even expanded the range to 7–18 mmol/L (28). However, most of these commonly used reference values are based on the statistical analysis of healthy people. For patients, especially those with severe diseases, we should be cautious as to whether these normal values can continue to be used. The normal range is only for the whole population, while different individuals have certain variations in their physiological and metabolic processes. For specific individuals, there may be some differences in the appropriate AG levels. The results of this study highlight that the risk of death of SA-AKI patients may increase if the AG exceeds 14 mmo/L, which suggests that doctors should pay attention to the specific AG detection value, rather than just whether it is in the normal range.
Limitations of this study
This is a retrospective study based on the MIMIC database. Therefore, the research results may be influenced by two factors. Firstly, this database comprises data from a famous large medical center in the United States, which is a leading institution in the field of critical care. Their service level and experience with severely ill patients may be significantly superior to many other medical institutions, and their diagnostic and treatment models may also be markedly different from those of other medical institutions. These differences may have a notable impact on the prognosis of patients. Therefore, the representativeness of this sample is limited. Secondly, the retrospective nature of the study meant that many patients were not included in the final analysis due to incomplete clinical data, leading to a certain degree of patient selection bias. Thirdly, this study did not use the albumin-corrected AG for analysis, which may affect the prognostic value of AG in SA-AKI patients.
Conclusions
In this study, we found that AG levels can serve as a valuable predictor of the death risk of SA-AKI patients during hospitalization. We suggest that, in clinical practice, doctors pay some attention to AG when treat SA-AKI patients.
Acknowledgments
Funding: None.
Footnote
Reporting Checklist: The authors have completed the TRIPOD reporting checklist. Available at https://atm.amegroups.com/article/view/10.21037/atm-22-5916/rc
Conflicts of Interest: All authors have completed the ICMJE uniform disclosure form (available at https://atm.amegroups.com/article/view/10.21037/atm-22-5916/coif).The authors have no conflicts of interest to declare.
Ethical Statement: The authors are accountable for all aspects of the work in ensuring that questions related to the accuracy or integrity of any part of the work are appropriately investigated and resolved. The study was conducted in accordance with the Declaration of Helsinki (as revised in 2013).
Open Access Statement: This is an Open Access article distributed in accordance with the Creative Commons Attribution-NonCommercial-NoDerivs 4.0 International License (CC BY-NC-ND 4.0), which permits the non-commercial replication and distribution of the article with the strict proviso that no changes or edits are made and the original work is properly cited (including links to both the formal publication through the relevant DOI and the license). See: https://creativecommons.org/licenses/by-nc-nd/4.0/.
References
- Cecconi M, Evans L, Levy M, et al. Sepsis and septic shock. Lancet 2018;392:75-87. [Crossref] [PubMed]
- Singer M, Deutschman CS, Seymour CW, et al. The Third International Consensus Definitions for Sepsis and Septic Shock (Sepsis-3). JAMA 2016;315:801-10. [Crossref] [PubMed]
- Font MD, Thyagarajan B, Khanna AK. Sepsis and Septic Shock - Basics of diagnosis, pathophysiology and clinical decision making. Med Clin North Am 2020;104:573-85. [Crossref] [PubMed]
- Ronco C, Bellomo R, Kellum JA. Acute kidney injury. Lancet 2019;394:1949-64. [Crossref] [PubMed]
- Adhikari NK, Fowler RA, Bhagwanjee S, et al. Critical care and the global burden of critical illness in adults. Lancet 2010;376:1339-46. [Crossref] [PubMed]
- Murugan R, Karajala-Subramanyam V, Lee M, et al. Acute kidney injury in non-severe pneumonia is associated with an increased immune response and lower survival. Kidney Int 2010;77:527-35. [Crossref] [PubMed]
- Peerapornratana S, Manrique-Caballero CL, Gómez H, et al. Acute kidney injury from sepsis: current concepts, epidemiology, pathophysiology, prevention and treatment. Kidney Int 2019;96:1083-99. [Crossref] [PubMed]
- Xu X, Nie S, Liu Z, et al. Epidemiology and Clinical Correlates of AKI in Chinese Hospitalized Adults. Clin J Am Soc Nephrol 2015;10:1510-8. [Crossref] [PubMed]
- Vincent JL, Sakr Y, Sprung CL, et al. Sepsis in European intensive care units: results of the SOAP study. Crit Care Med 2006;34:344-53. [Crossref] [PubMed]
- Gómez H, Kellum JA. Sepsis-induced acute kidney injury. Curr Opin Crit Care 2016;22:546-53. [Crossref] [PubMed]
- Kellum JA, Wen X, de Caestecker MP, et al. Sepsis-Associated Acute Kidney Injury: A Problem Deserving of New Solutions. Nephron 2019;143:174-8. [Crossref] [PubMed]
- Kotake Y. Unmeasured anions and mortality in critically ill patients in 2016. J Intensive Care 2016;4:45. [Crossref] [PubMed]
- Kraut JA, Madias NE. Treatment of acute metabolic acidosis: a pathophysiologic approach. Nat Rev Nephrol 2012;8:589-601. [Crossref] [PubMed]
- Farwell WR, Taylor EN. Serum anion gap, bicarbonate and biomarkers of inflammation in healthy individuals in a national survey. CMAJ 2010;182:137-41. [Crossref] [PubMed]
- Sun T, Cai C, Shen H, et al. Anion Gap Was Associated with Inhospital Mortality and Adverse Clinical Outcomes of Coronary Care Unit Patients. Biomed Res Int 2020;2020:4598462. [Crossref] [PubMed]
- Liu X, Feng Y, Zhu X, et al. Serum anion gap at admission predicts all-cause mortality in critically ill patients with cerebral infarction: evidence from the MIMIC-III database. Biomarkers 2020;25:725-32. [Crossref] [PubMed]
- Bagshaw SM, Uchino S, Bellomo R, et al. Septic acute kidney injury in critically ill patients: clinical characteristics and outcomes. Clin J Am Soc Nephrol 2007;2:431-9. [Crossref] [PubMed]
- Sood MM, Shafer LA, Ho J, et al. Early reversible acute kidney injury is associated with improved survival in septic shock. J Crit Care 2014;29:711-7. [Crossref] [PubMed]
- Kellum JA, Sileanu FE, Bihorac A, et al. Recovery after Acute Kidney Injury. Am J Respir Crit Care Med 2017;195:784-91. [Crossref] [PubMed]
- Srisawat N, Murugan R, Lee M, et al. Plasma neutrophil gelatinase-associated lipocalin predicts recovery from acute kidney injury following community-acquired pneumonia. Kidney Int 2011;80:545-52. [Crossref] [PubMed]
- Duda I, Krzych Ł. Plasma Neutrophil Gelatinase-Associated Lipocalin Is Useful for Predicting Mortality in Critically Ill Patients. J Clin Med 2021;10:2576. [Crossref] [PubMed]
- Mitra B, Roman C, Charters KE, et al. Lactate, bicarbonate and anion gap for evaluation of patients presenting with sepsis to the emergency department: A prospective cohort study. Emerg Med Australas 2020;32:20-4. [Crossref] [PubMed]
- Mohr NM, Vakkalanka JP, Faine BA, et al. Serum anion gap predicts lactate poorly, but may be used to identify sepsis patients at risk for death: A cohort study. J Crit Care 2018;44:223-8. [Crossref] [PubMed]
- Kim MJ, Kim YH, Sol IS, et al. Serum anion gap at admission as a predictor of mortality in the pediatric intensive care unit. Sci Rep 2017;7:1456. [Crossref] [PubMed]
- Zhang T, Wang J, Li X. Association Between Anion Gap and Mortality in Critically Ill Patients with Cardiogenic Shock. Int J Gen Med 2021;14:4765-73. [Crossref] [PubMed]
- Hu T, Zhang Z, Jiang Y. Albumin corrected anion gap for predicting in-hospital mortality among intensive care patients with sepsis: A retrospective propensity score matching analysis. Clin Chim Acta 2021;521:272-7. [Crossref] [PubMed]
- Zhong L, Xie B, Ji XW, et al. The association between albumin corrected anion gap and ICU mortality in acute kidney injury patients requiring continuous renal replacement therapy. Intern Emerg Med 2022; Epub ahead of print. [Crossref]
- Ayala-Lopez N, Harb R. Interpreting Anion Gap Values in Adult and Pediatric Patients: Examining the Reference Interval. J Appl Lab Med 2020;5:126-35. [Crossref] [PubMed]
(English Language Editor: A. Kassem)