Value of genomics- and radiomics-based machine learning models in the identification of breast cancer molecular subtypes: a systematic review and meta-analysis
Highlight box
Key findings
• Data from this systematic review and meta-analysis support radiomics and gene-transcriptomic ML model analyses, which have ideal predictive accuracy in determining early the BRCA molecular subtypes.
What is known and what is new?
• Machine learning models are increasingly being used to predict the molecular subtypes of breast cancer. However, its application is still controversial.
• This paper comprehensively analyzes the application value of the machine learning prediction model based on radiomics and gene transcriptomics in predicting the molecular subtypes of breast cancer.
What is the implication, and what should change now?
• Our study confirmed the application value of machine learning in predicting the molecular subtypes of breast cancer and providing favorable evidence for finding new ways to improve the clinical treatment of breast cancer, also promoting the development of modern precision medicine.
Introduction
Breast cancer (BRCA) has overtaken lung cancer to become the world’s most prevalent malignant tumor and the top cause of death for women; the number of deaths accounts for 6.6% of all cancer deaths (1). Statistically, the number of BRCA cases constituted one-third of the total female cancer victims in 2022 (2). With increasing populations and increased risk factors, the burden of BRCA is rising substantially worldwide. Based on the incidence trend of BRCA predicted by the GLOBOCAN database, there will be an estimated 9.1 million new cases by 2070 (3). Early screening and accurate diagnosis are currently regarded as the most effective management of BRCA (4). Core needle biopsy or fine-needle aspiration biopsy is extensively used to detect BRCA, and for suspicious cases, tissue biopsy is used to confirm relevant features. However, as high as 31% of BRCA cases are misdiagnosed using the described methods (4). The heterogeneity in the molecular characteristics and cellular composition of BRCA is well known, and increasingly studies have indicated the significance of BRCA molecular subtypes in the diagnosis, treatment, and prognosis of the disease (5-7). The establishment of molecular profiles of adenocarcinomas has generated common molecular subtypes [Luminal A, Luminal B, Basal-like, and human epidermal growth factor receptor 2 (HER2)], which has enabled the phenotypic heterogeneity of BRCA subtypes to be determined and also personalized management regimens using subtype-specific indications (8). Luminal A and B are two relatively mild molecular subtypes of BRCA with better prognoses, and both are sensitive to endocrine therapy. The level of Ki67-labeled standardized proliferation is the most significant feature to distinguish the two (9). HER2-positive BRCA is characterized by its high invasiveness and high recurrence, with increased incidence year by year. Studies have indicated an association of its high recurrence with activation of the PI3K-Akt-mTOR signaling pathway and the stimulation of glycolysis (10). Immunohistochemistry and FISH are widely applied in HER2 molecular subtyping, and new detection assays are emerging. Among them, the more applicable methods include quantum dot-based probes, mass spectrometry, and next-generation sequencing (11).
Heterogeneity is associated with shorter disease-free survival and overall survival outcomes (12). Recent studies have reported that BRCA also presents metastatic heterogeneity, which is inseparable from its receptor status (13), and the receptor status determines the molecular subtype of BRCA. Single-cell gene expression analysis (14), genome and transcriptome analyses (15), and lineage tracing (16) can all be utilized to explore the underlying molecular mechanisms, allowing more systematic and in-depth research on the molecular heterogeneity of BRCA. Parker et al. have developed five intrinsic subtypes using 50 genes (PAM50), which are consistent with the molecular subtypes of BRCA predicted by hierarchical clustering and microarray analyses (17), and they are of great significance in clinical diagnosis and prognostic prediction. Achieving more accurate predictive performance with fewer but more representative genes is also the goal of researchers. Interestingly, the molecular characteristics of each subtype can also be dynamically changed. For example, triple-negative BRCA itself is multi-heterogeneous, with basal-like characteristics in one of its four specific subtypes (18). This indicates that being able to predict changes in the BRCA molecular subtype will assist in identifying novel therapeutic targets and the transformation of refractory BRCA to treatable BRCA. Despite intrinsic molecular subtypes providing the principle biological classifications of BRCA, the subtype assignment of individuals is influenced by the techniques used, as well as the study cohort composition. How to efficiently and precisely predict molecular subtypes of BRCA has emerged as an ongoing focus of study of BRCA at the molecular level.
Specific treatment for different tumor types has always been a challenge, and great efforts have been made to maximize efficacy and minimize the toxicity of therapies. Therefore, improvement in cancer classification is key to advances in cancer treatment. Progress has been achieved in classification prediction technology through the use of machine learning (ML), which integrates computer science, statistics, and biomedical research (19). ML based on the algorithm of the input data as the premise applies computer analysis to identify data attributes and trends, and learns from previous experience to predict output values with a certain degree of accuracy, and as a semi-automated process is both cost- and labor-saving. It is classified into supervised learning and unsupervised learning. The former finds a pattern in the training set to perform known classification and regression of the data set, and the latter clusters and reduces the dimensionality with the data set only (20). ML can handle large-scale, complex, and diverse data, which is attributed to the formulation of algorithms. The algorithms used for ML in the medical field include support vector machine (SVM), neural network (NN), deep learning, and latent variable models (21). ML has been applied in drug research and development (22), robotic surgery and decision-making (23), and imaging diagnosis (24), and multiple current studies have pointed out that ML is vitally significant in cancer research, especially cancers of the lung, colorectum, and prostate (14,25-27). ML has also attracted widespread attention for BRCA diagnosis and management, such as BRCA tumor identification (28), BRCA neoadjuvant efficacy prediction (29), and BRCA medical imaging analyses (radiomics analysis and histopathological image analysis) (30,31).
Radiomics is an emerging field of medical imaging, based on extracting and quantifying high-throughput feature information from medical images that fail in identification using traditional imaging examination methods. It builds a bridge between medical imaging and personalized diagnosis and treatment, and it has the potential to become an alternative to invasive biopsy (32). However, genetic expression evaluation remains the gold standard (32). How to efficiently extract differentially expressed genes from gene databases and assess their impact on prognosis remain to be solved. Meanwhile, exploring more convenient, noninvasive, and accurate genetic detection methods and tumor markers is also a current research focus, leading to various ML models of gene transcriptomics for BRCA being developed. Such models have the potential to improve biomarker identification of the diverse BRCA molecular subtypes, thereby offering novel insight into the differential pathogenesis of these subtypes and the selection and research of more targeted personalized and systemic treatment regimens. Hence, for clinicians, ML models based on radiomics and gene transcriptomics are helpful and significant in improving diagnostic performance and developing reasonable diagnosis and treatment protocols. Nevertheless, the predictive accuracy of current ML models in identifying BRCA molecular subtypes differs due to their diverse mathematical algorithms and the differences in the classifications of modeling variables. Many studies have shown that meta-analysis is of great significant in determining the prediction accuracy of ML models (33,34). Therefore, we conducted this systematic review to analyze the accuracy of various ML models in the identification of BRCA molecular subtypes based on the same classification of modeling variables.
Given the potential of ML in BRCA molecular subtype prediction, this meta-analysis was conducted to explore its application significance of ML, and to provide some practical reference for accurate diagnostic auxiliary system and medically intelligent diagnosis of BRCA. Furthermore, we also compared the prediction accuracy between radiomics-based and genomics-based ML. We present the article in accordance with the MOOSE reporting checklist (available at https://atm.amegroups.com/article/view/10.21037/atm-22-5986/rc).
Methods
This project was prospectively registered with PROSPERO (CRD42022333611).
Literature search
We searched the PubMed, Embase, Cochrane, and Web of Science databases from inception to April 15, 2022. No restrictions were set on region or language, and the retrieval method used subject headings plus free words. Subject headings included Breast Neoplasms and Machine Learning. More search strategies are shown in Table S1.
Inclusion and exclusion criteria
We formulated the criteria for inclusion and exclusion of studies according to the PICO principle. Inclusion criteria included: (I) subjects had pathologically confirmed BRCA with relevant molecular subtypes as previously described [Luminal A, Luminal B, HER2 overexpression (Basal-like) and normal]; (II) interventions: (i) ML studies for classifying BRCA molecular subtypes based on radiomics [i.e., mammography (MMG), digital breast tomosynthesis (DBT), ultrasound (US), computed tomography, and magnetic resonance imaging (MRI)], genometrics or other pathological data; (ii) original research designs were cohort studies, case-control and nested case-control studies, or case–cohort studies; (III) the comparison is between different molecular subtypes; (IV) outcomes: the classification performance of the constructed ML model was assessed, and the assessment indicators included one of the following: c-statistic, sensitivity, specificity, calibration curve, accuracy, F1 score, and confusion matrix.
Exclusion criteria were: (I) subjects did not have any of the molecular subtypes as stated in the inclusion criterion; (II) interventions: (i) only difference analysis was performed rather than the construction of an integrated ML model, (ii) studies with small overall samples; (III) outcomes: classification performance of the constructed ML model was not evaluated.
Literature screening and data extraction
We imported the retrieved literature into Endnote, and after ruling out duplicate articles, the original studies that satisfied the criteria were initially screened using titles and abstracts. Selected studies were downloaded for subsequent analysis by intensive reading, and the final included studies for this research were determined.
A standard information extraction spreadsheet was created prior to data extraction, which included article title, author names, publication year, countries of authors, research type, sample source, molecular subtypes, number of samples for training set subtype detection, the total number of samples for the training set, external validation, samples for testing set subtype detection, overall samples for external validation, model types, model overfitting considerations (bootstrap/k-fold cross-validation), and outcome indicators.
Literature screening and data extraction were performed independently by Yiwen Zhang and Guofeng Li, and post-technical cross-validation was performed. Two investigators, Yuzhuo Bai and Wenqing Bian, assisted when there were discrepancies between the first two reviewers.
Quality assessment
Because we adopted gene-transcriptomic and radiomics ML models, we used the Radiomics Quality Score (RQS) [28975929] and PROBAST [PMID: 31585960] for quality assessment of the ML models.
The RQS assesses the quality from the image extraction process to the ML construction process, with 16 assessment items and 36 points in total. The items of the Prospective study registered in a trial database and the Validation are very strict, with 7 and 5 points at most, respectively. If there was no external validation, a penalty of 5 points was given plus no points awarded, which was very severe for radiometrics. Additionally, due to the extraction process of radiomics generating a large number of predictor variables, the assessment of the Feature reduction or adjustment for multiple testing was also very severe. If neither measure was implemented, a penalty of 3 points was given plus no points awarded.
We assessed the quality of the gene- transcriptome ML model using PROBAST, which contains a large number of items involving four distinct aspects: subjects, predictors, outcomes, and statistical analysis, reflecting the overall risk of bias and overall usability. The four domains consisted of 2, 3, 6, and 9 questions with specificity, respectively, each with 3 responses (yes/maybe yes, no/maybe no, and no information). A domain is considered to be at high risk if it contains at ≥1 question with a response of no or maybe no. A low-risk domain is determined when it contains all responses with yes or maybe yes. If each aspect is determined as low risk, the overall risk of bias is rated as low, whereas ≥1 domain is considered as high risk, and the overall risk of bias is judged as high.
Risk of bias assessment/quality assessment was performed by two independent investigators, with post-technical cross-validation. A third investigator assisted in judgment when discrepancies arose between the first two reviewers.
Outcome indicators
In our systematic review, the outcome indicators were c-statistic, reflecting the accuracy of the ML model, and the sensitivity and specificity summed by true positive (TP), false positive (FP), false negative (FN), and true negative (TN). Of these, TP, FP, FN and TN in the original studies can be extracted using the following methods: Method 1—direct report of the original studies (e.g., multi-classification confusion matrix); Method 2—extract the sensitivity and specificity of the original studies, and combine the number of cases with corresponding molecular subtypes and the total number of samples; Method 3—original research provided receiver operating characteristic (ROC) curves, in light of the principle of the optimal Youden index, the sensitivity and specificity were extracted using Origin 2020 software as per Method 2.
Statistical analysis
A meta-analysis was conducted on the indicators (c-statistic, sensitivity, and specificity) to assess the ML models. If c-statistic was depleted 95% confidence interval (CI) and standard error (SE), we referred to the study of Debray et al. (35) to estimate its SE. Given the differences among the variables included in each ML model and inconsistent parameters, For the c-statistic, the random effects model was preferred for meta-analyses. A bi-variable mixed-effect model was used for meta-analysis of sensitivity and specificity. The current meta-analysis was implemented using R4.2.0 (R development Core Team, Vienna, http://www.R-project.org).
Results
Literature search
Our literature search initially identified 3,421 articles from the PubMed, Embase, Cochrane, and Web of Science databases. After deleting 1,093 duplicate articles, 2,328 articles were screened out based on their titles and abstracts, leaving 98 potentially eligible articles. Of them, 10 did not have full text available. No eligible articles were found after searching the references of the remaining 88 articles. After a full-text review of the provisionally eligible articles, 50 were excluded either due to the lack of BRCA molecular subtype or unclear sample size, and so 38 articles were finally included in this study for subsequent systematic review and meta-analysis (Table 1 and Table 2). The screening processes are shown in Figure 1.
Table 1
First author | Publication year | Country/Region | Study design | Molecular subtype | Type of model | Model evaluation metric |
---|---|---|---|---|---|---|
Ma M (36) | 2022 | China | Cohort | TNBC, HER2 | Support vector machines, random forest | Radiomics |
Leithner D (37) | 2020 | USA | Database research | HER2, luminal A, luminal B, TN | Artificial neural network | Radiomics |
Chen L (38) | 2019 | China | Database research | Basal, HER2, luminal A, luminal B | Support vector machines, random forest | Gene |
Zhao Y (39) | 2020 | USA | Database research | Basal, HER2, luminal A, luminal B | Random forest | Gene |
Huang CC (40) | 2013 | Taiwan | UNC Microarray database research | Basal, HER2, luminal A, luminal B | Logistic regression, least square method | Gene |
Yu Z (41) | 2020 | China | TCGA database research | Basal, HER2, luminal A, luminal B, Normal-like | Naive Bayes, random forest, support vector machines | Gene |
Liu T (42) | 2022 | China | TCGA-BRCA database research | Basal, HER2, luminal A, luminal B | Logistic regression, fusion model, CNN, DNN | Gene |
Adabor ES (43) | 2019 | USA | Case-control | HER2 | Naive Bayes, random forest | Gene |
Huang Y (44) | 2021 | China | Database research | HER2 | Support vector machines, logistic regression | Radiomics |
Lopez-Rincon A (45) | 2020 | Netherlands | Database research | TN | Gradient boosting, random forest, logistic regression, passive aggressive, SGDClassifier, support vector machines (linear), ridge regression | Gene |
Wu J (46) | 2021 | USA | Database research | TNBC | K-Nearest neighbors, Naive Bayes, deep learning, support vector machines | Gene |
Mohaiminul Islam M (47) | 2020 | Canada | METABRIC database research | Basal, HER2, luminal A, luminal B | Support vector machines, random forest | Gene |
Wilson TR (48) | 2014 | USA | Database research | HER2 | Random forest | Gene |
Seo MK (49) | 2020 | Korea | Database research | Basal, HER2, luminal A, luminal B | Random forest | Gene |
Couture HD (50) | 2018 | USA | Case-control | Basal, HER2, luminal A, luminal B Normal-like | Deep learning | Radiomics |
Fan M (51) | 2017 | China | Cohort | HER2, luminal A, luminal B Basal-like | Logistic regression | Radiomics |
Jiang M (52) | 2021 | China | Database research | TN, HER2, luminal A, luminal B | Artificial neural network | Radiomics |
Moon WK (53) | 2015 | South Korea | Case-control | TNBC | Support vector machines | Radiomics |
Talari ACS (54) | 2019 | UK | Case-control | TNBC, HER2, luminal A, luminal B | Linear discriminant method | Radiomics |
Nie Z (55) | 2021 | China | Case-control | HER2 | Radiomics | |
Zhou J (56) | 2019 | China | Case-control | HER2 | Logistic regression | Radiomics |
Fan M (57) | 2019 | China | Case-control | Basal, HER2, luminal A, luminal B | Random forest | Radiomics |
Wu M (58) | 2011 | China | Case-control | Basal, HER2, luminal A, luminal B | Deep learning | Radiomics |
Zhou J (59) | 2021 | China | Case-control | HER2 | Support vector machines | Radiomics |
Wu T (60) | 2019 | China | Case-control | TN | Logistic regression | Radiomics |
Ma W (61) | 2019 | China | Case-control | TNBC, HER2, Lum | Naive Bayes | Radiomics |
Wang W (62) | 2022 | China | Case-control | TN, luminal A + B | Logistic regression | Radiomics |
Wu J (63) | 2017 | China | Case-control | Basal, luminal A, luminal B | Logistic regression | Radiomics |
Wu M (64) | 2019 | China | Case-control | Basal, HER2, luminal A, luminal B | Deep learning | Radiomics |
Xie T (65) | 2019 | China | Case-control | TN | Support vector machines | Radiomics |
Yang X (66) | 2020 | China | Case-control | HER2 | Logistic regression | Radiomics |
Zhang X (67) | 2021 | China | Case-control | HER2, TN | Artificial neural network | Radiomics |
Zhang Y (68) | 2021 | USA | Case-control | HER2, TN | Artificial neural network | Radiomics |
Zhou J (69) | 2019 | China | Case-control | HER2 | Support vector machines | Radiomics |
Saha A (70) | 2018 | USA | Case-control | TN, HER2, luminal A, luminal B | Random forest | Radiomics |
Sutton EJ (71) | 2016 | USA | Case-control | TN | Support vector machines | Radiomics |
Wang F (72) | 2022 | China | Case-control | luminal A+B | Support vector machines | Radiomics |
Wang J (73) | 2015 | Japan | Case-control | TN | Support vector machines | Radiomics |
BRCA, breast cancer; TNBC, triple negative breast cancer; HER2, HER2-overexpressed subtypes of breast cancer molecules; CNN, convolutional neural network; DNN, deep neural network.
Table 2
First author | Publication year | No. of subtype samples in training set | No. of samples in training set | No. of subtype samples in validation set | Sample size for external validation |
---|---|---|---|---|---|
Ma M (36) | 2022 | NA | 450 | 71, 64 | 150 |
Leithner D (37) | 2020 | 8, 34, 6, 16 | 64 | 3, 15, 2, 7 | 27 |
Chen L (38) | 2019 | 34, 37, 120, 63 | 254 | NA | NA |
Zhao Y (39) | 2020 | NA | NA | 262, 208, 891, 423 | 1784 |
Huang CC (40) | 2013 | 57, 35, 23, 12 | 139 | 57, 35, 23, 12 | 139 |
Yu Z (41) | 2020 | 192, 82, 564, 207, 40 | 1085 | NA | NA |
Liu T (42) | 2022 | 127, 56, 326, 151 | 660 | 14, 6, 43, 21 | 84 |
Adabor ES (43) | 2019 | 187 | 806 | NA | NA |
Huang Y (44) | 2021 | 25 | 137 | NA | NA |
Lopez-Rincon A (45) | 2020 | 139 | 183 | NA | NA |
Wu J (46) | 2021 | 110 | 1102 | NA | NA |
Mohaiminul Islam M (47) | 2020 | 116, 87, 464, 268 | 935 | 210, 153, 255, 224 | 842 |
Wilson TR (48) | 2014 | 14 | 173 | NA | NA |
Seo MK (49) | 2020 | 15, 10, 38, 21 | 84 | NA | NA |
Couture HD (50) | 2018 | 179 | 571 | NA | 288 |
Fan M (51) | 2017 | 7, 34, 8, 11 | 60 | NA | 36 |
Jiang M (52) | 2021 | NA | 1275 | 18, 42, 116, 229 | 405 |
Moon WK (53) | 2015 | 85 | 169 | NA | NA |
Talari ACS (54) | 2019 | 30, 30, 30, 30 | 120 | NA | NA |
Nie Z (55) | 2021 | 57 | 226 | NA | NA |
Zhou J (56) | 2021 | 63 | 244 | 16 | 62 |
Fan M (57) | 2019 | 39, 37, 54, 80 | 210 | NA | NA |
Wu M (58) | 2011 | 40, 76, 96, 151 | 363 | NA | NA |
Zhou J (59) | 2021 | 53 | 200 | 23 | 106 |
Wu T (60) | 2019 | 23 | 140 | NA | NA |
Ma W (61) | 2019 | 40, 141 | 227 | NA | NA |
Wang W (62) | 2022 | 42, 27, 11 | 84 | 43, 27, 11 | 84 |
Wu J (63) | 2017 | 151, 96, 76, 40 | 363 | NA | NA |
Wu M (64) | 2019 | 134 | 134 | NA | NA |
Xie T (65) | 2019 | 60 | 177 | 57 | 162 |
Yang X (66) | 2020 | 149, 118 | 684 | NA | 122 |
Zhang X (67) | 2021 | 24, 10 | 99 | 19, 10 | 83 |
Zhang Y (68) | 2021 | 63 | 244 | 16 | 62 |
Zhou J (69) | 2019 | 82, 27, 305, 471 | 461 | NA | 461 |
Saha A (70) | 2018 | 48 | 178 | NA | NA |
Sutton EJ (71) | 2016 | 110 | 220 | 43 | 80 |
Wang F (72) | 2022 | 11 | 84 | NA | NA |
Wang F (72) | 2022 | 153 | 220 | 80 | NA |
Wang J (73) | 2015 | 11, 4, 42, 27 | 84 | NA | NA |
Characteristics of the included studies
The 38 included studies recruited 17,913 BRCA patients, with 57 ML models, which were mainly multi-classification models, covering molecular subtypes Luminal A, Luminal B, HER2 overexpression, triple negative, and normal. In 28 studies (43 ML models) the BRCA molecular subtypes were identified based on a radiomics ML, and the remaining 11 studies (14 ML models) identified the molecular subtypes by gene transcriptomics ML. There were 22 case-control studies, 3 cohort studies, and 13 retrospective studies. Among all studies, the training sets of 7 studies adopted ≥2 tools for validation, there were 9 articles covering the 4 molecular subtypes, 5 articles classified HER2 subtype only, 5 classified the triple-negative breast cancer (TNBC) subtype, and 5 covered the HER2 + TNBC subtypes. The testing sets of 3 articles used dual-tool analysis, there were 4 articles covering the 4 molecular subtypes, 3 articles classified the HER2 subtype only, and 1 for the TNBC subtype. The number of registrations in the training set of the validation gene transcriptomics group was 3,019; 4 studies performed external validation, and the total sample size for external validation was 2,849. The number of registrations in the training set of the radiomics group was 4,048; 13 studies conducted external validation, and the total sample size was 2,610. In the training set, 9 studies used the logistic regression (LR) model, 7 used the SVM model, 3 used the Naive Bayes (NB) model, 5 used the random forest (RF) model, and 4 used the artificial neural network (ANN) model; in the testing set, 4 studies used the LR model, 2 used the ANN model, 4 used the SVM model, and 1 used the RF model.
Quality assessment
Among the 14 ML models based on genomics, we assessed the quality of studies as per PROBAST. The assessment results revealed that the risk of bias was mostly caused by the lack of external validation and the small number of modeling samples, which was reflected in the statistical analysis of PROBAST assessment (Figure 2). Among the 43 ML models based on radiomics, only 14 (32.56%) models conducted external validation, and there was no clearly described Prospective study registered in a trial database. Comprehensive assessment results of other items showed an average score of 14.6 for 43 models (standard deviation: 5.3).
Value of ML for predicting BRCA molecular subtype
C-statistic
Meta-analyses of the c-statistic were conducted using a random effects model, which revealed that the c-statistic values by radiomics ML for Luminal A, Luminal B, Basal-like or TNBC, and HER2 subtypes were 0.76 (95% CI: 0.60–0.96), 0.78 (95% CI: 0.69–0.87) 0.87 (95% CI: 0.83–0.91), and 0.83 (95% CI: 0.81–0.86), respectively.
The c-statistic values of the BRCA molecular subtypes identified by gene-transcriptomic ML were 0.96 (95% CI: 0.93–0.99), 0.93 (95% CI: 0.91–0.95), 0.98 (95% CI: 0.95–1.00), and 0.97 (95% CI: 0.96–0.98), respectively (Table 3, Figure 3).
Table 3
Modeling variable | Subtype | n | C-statistic | n | Sensitivity | Specificity |
---|---|---|---|---|---|---|
Radiomics | Luminal | 12 | 0.77 [0.71, 0.83] | 12 | 0.79 [0.72, 0.84] | 0.85 [0.73, 0.92] |
Luminal A | 4 | 0.76 [0.60, 0.96] | 5 | 0.76 [0.68, 0.82] | 0.86 [0.62, 0.96] | |
Luminal B | 4 | 0.78 [0.69, 0.87] | 5 | 0.84 [0.69, 0.93] | 0.86 [0.64, 0.96] | |
Mixed | 4 | 0.76 [0.73, 0.80] | 2 | NA | NA | |
Basal-like or TNBC | 15 | 0.87 [0.83, 0.91] | 19 | 0.82 [0.75, 0.87] | 0.86 [0.78, 0.91] | |
HER2 | 16 | 0.83 [0.81, 0.86] | 13 | 0.84 [0.81, 0.87] | 0.83 [0.71, 0.90] | |
Gene | Luminal | 10 | 0.94 [0.92, 0.96] | 16 | 0.86 [0.80, 0.90] | 0.96 [0.92, 0.97] |
Luminal A | 5 | 0.96 [0.93, 0.99] | 8 | 0.89 [0.87, 0.92] | 0.95 [0.89, 0.98] | |
Luminal B | 5 | 0.93 [0.91, 0.95] | 8 | 0.79 [0.64, 0.89] | 0.96 [0.91, 0.98] | |
Mixed | NA | NA | NA | NA | NA | |
Basal-like or TNBC | 5 | 0.98 [0.95, 1.00] | 9 | 0.97 [0.93, 0.98] | 0.99 [0.96, 1.00] | |
HER2 | 6 | 0.97 [0.96, 0.98] | 11 | 0.92 [0.85, 0.96] | 0.96 [0.94, 0.97] |
TNBC, triple negative breast cancer; HER2, human epidermal growth factor receptor 2; NA, not available.
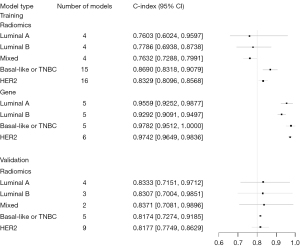
In the testing cohort, statistical analysis was conducted on the data available and the results showed that the predicted c-index values for molecular subtypes of BRCA exceeded 80% for both sets of the ML models.
Sensitivity and specificity
A bivariate mixed-effects model was used for the meta-analysis of TP, FP, FN, and TN, and the sensitivity of the ML method for each molecular subtype was summarized. The sensitivity and specificity of radiometrics ML for molecular subtype identification of Luminal A were 0.79 (95% CI: 0.72–0.84) and 0.85 (95% CI: 0.73–0.92), for Luminal B were 0.84 (95% CI: 0.69–0.93) and 0.86 (95% CI: 0.64–0.96), for Basal-like or TNBC were 0.82 (95% CI: 0.75–0.87) and 0.86 (95% CI: 0.78–0.91), for HER2 were 0.84 (95% CI: 0.81–0.87) and 0.83 (95% CI: 0.71–0.90), and for Luminal subtypes (Luminal A + Luminal B) were 0.79 (95% CI: 0.72–0.84) and 0.85 (95% CI: 0.73–0.92), respectively.
The sensitivity and specificity of the gene-transcriptomic. ML for the identification of Luminal A were 0.89 (95% CI: 0.87–0.92) and 0.95 (95% CI: 0.89–0.98), for Luminal B were 0.79 (95% CI: 0.64–0.89) and 0.86 (95% CI: 0.91–0.98), for Basal-like or TNBC were 0.97 (95% CI: 0.93–0.98) and 0.99 (95% CI: 0.96–1.00), for HER2 were 0.92 (95% CI: 0.85–0.96) and 0.96 (95% CI: 0.94–0.97), and for Luminal subtypes (Luminal A + Luminal B) were 0.86 (95% CI: 0.80–0.90) and 0.96 (95% CI: 0.92–0.97), respectively (Table 4, Figures 4,5).
Table 4
Modeling variables | Subtype | n | C-statistic | n | Sensitivity | Specificity |
---|---|---|---|---|---|---|
Radiomics | Luminal | 9 | 0.84 [0.77, 0.91] | 8 | 0.86 [0.71, 0.94] | 0.76 [0.51, 0.91] |
Luminal A | 4 | 0.83 [0.72, 0.97] | 3 | NA | NA | |
Luminal B | 3 | 0.83 [0.70, 0.98] | 3 | NA | NA | |
Mixed | 2 | 0.84 [0.71, 0.99] | 2 | NA | NA | |
Basal-like or TNBC | 5 | 0.82 [0.73, 0.92] | 2 | NA | NA | |
HER2 | 9 | 0.82 [0.77, 0.86] | 7 | 0.66 [0.53, 0.77] | 0.83 [0.70, 0.91] | |
Gene | Luminal | NA | NA | 8 | 0.76 [0.70, 0.81] | 0.93 [0.91, 0.95] |
Luminal A | NA | NA | 4 | 0.80 [0.76, 0.84] | 0.93 [0.91, 0.95] | |
Luminal B | NA | NA | 4 | 0.69 [0.61, 0.76] | 0.93 [0.89, 0.96] | |
Mixed | NA | NA | NA | NA | NA | |
Basal-like or TNBC | NA | NA | 4 | 0.94 [0.80, 0.98] | 0.99 [0.97, 1] | |
HER2 | NA | NA | 4 | 0.71 [0.65, 0.76] | 0.96 [0.94, 0.97] |
TNBC, triple negative breast cancer; HER2, human epidermal growth factor receptor 2; NA, not available.
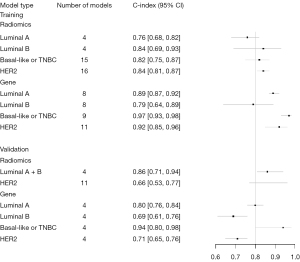
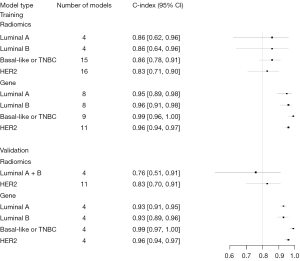
Discussion
This systematic review and meta-analysis indicated that ML based on radiomics and genomics can achieve satisfactory performance in predicting BRCA molecular subtypes. The accuracy of gene-transcriptomic ML was superior to that of radiomics ML, but the diagnostic accuracy of identification of BRCA molecular subtypes using radiological data analysis has theoretical support. We also found that ML was more accurate in identifying the Basal-like/TNBC subtype than other BRCA molecular subtypes.
The gene transcriptome data analyzed in the present study clarified the ability of ML to identify the intrinsic biological subtypes of TNBC, HER2+, and luminal BRCA using whole-gene database analysis, with good accuracy. Genetic diagnosis is the gold standard for cancer diagnosis, and whole genomic amplification provides an opportunity to conduct in-depth investigations of the role of epigenetic processes in cancer. Unfortunately, as epigenome map and cancer sample data accumulates, the analysis and utilization of such data become difficult. ML greatly improves efficiency due to its flexibility and learning ability to latent structures (74). For example, partial least squares (PLS) regression has been applied to BRCA intrinsic taxonomy and five distinct molecular subtypes were successfully identified (40). Wilson et al. used multiple model classifiers to determine estrogen receptor (ER), progesterone receptor (PR), and HER2 phenotypes and introduced a new median complement method, which is beneficial for the development of higher sensitivity and a lower FP rate (48). Additionally, ML helps scientists discover new biomarkers that can aid patient prognosis. As for the heterogeneity of BRCA, a single tumor marker is not sufficient. Many studies have focused on determining cancer prognosis and the subtypes of gene characteristics based on gene expression data, which are easily affected by noise signals, reducing accuracy (75). BRCA diagnosis is not limited to such parameters, with more genetic modification patterns, microRNAs and methylation and genetic variants being identified and potentially available. DNA methylation data are more stable than those of gene expression in cancer prognosis (76), and microRNA also shows several advantages in cancer prediction, prognosis, and therapeutic targets, and it can be obtained from body fluids thereby being noninvasive (77). Unfortunately, due to the existence of large number of differentially methylated genes and microRNAs, human verification is not yet available, but the present study showed how this can be effectively solved by using ML. For example, ML distinguished studies on Basal-like/TNBC from other molecular subtypes using both microRNA data and gene methylation data analysis with a sensitivity and specificity of 87%, 80%, and 100%, and 99%, respectively (38,45). This suggests a bright future for ML in the identification of BRCA molecular subtypes and also provides theoretical support for microRNA, especially gene methylation, as a predictor of BRCA prognosis.
BRCA screening is typically conducted by a variety of medical diagnostic imaging methods, including US, X-ray radiography, and MRI. However, defects in imaging techniques lead to relatively low average sensitivity and specificity (»70%) for clinical diagnosis (78,79). The ability of radiomics to analyze images to obtain various quantitative features from single or multiple imaging patterns and highlight such characteristics that are not visible to the naked eye greatly enhances the discriminative and predictive potential of medical imaging. Presently, radiomics can not only differentiate between benign and malignant tumors, tumor types and grades, but also support the prediction of response to neoadjuvant chemotherapy and recurrence (80). Our study revealed that the sensitivity and specificity of radiomics-based ML models in predicting BRCA molecular subtypes were as high as 80%, and some even reached 90%. The therapeutic benefit was similar to that of the gene-transcriptomics ML models, which is effectively avoiding the invasive procedure of needle biopsy for traditional diagnosis of the BRCA molecular subtype. ML diagnosis also overcomes the issue of inexperience of radiologists, and takes full advantage of image analysis, such as subtype analysis of H&E-stained histological images of BRCA, distinguishing Basal-like from non-Basal-like BRCA molecular subtypes with 75–80% accuracy, thus reducing the high cost of RNA-based genomic testing (55). In our study, the number of included studies of radiomics was more than that of genomics, indicating that radiomics is an emerging field receiving extensive attention. It can be used to maximize information extraction from almost all of the medical imaging modalities. Twelve such studies involved MRI, which is known to have higher resolution than X-ray or US. MRI has the best specificity and sensitivity of all imaging tools available, and we can take advantage of this technique to develop more accurate ML models. It is worth noting that our study results indicated that the average c-index value of radiomics in the prediction of BRCA molecular subtypes was 0.796, while that for transcriptomics was 0.956, implying that further efforts should be made to improve the accuracy of radiometric prediction models.
TNBC lacks the ER, PR, and HER2. It is characterized by young onset, high invasiveness, high recurrence rate, and poor prognosis (81). Studies have shown that neoadjuvant therapy for TNBC is highly effective and improves the pathological complete remission rate (82). The current study revealed that, regardless of gene-transcriptomic or radiomic analysis, ML was more effective in identifying Basal-like/TNBC BRCA, especially with the application of a radiomics ML model (Figure 3). As TNBC and Basal-like BRCA have many similarities in their clinical manifestation, histological morphology, and immunophenotype (83), and because of the limited number of study cases, we regarded Basal-like and TNBC as similar. However, further research has raised the question of whether Basal-like and TNBC can be compared (84). TNBC can be subdivided into several subtypes, and studies have shown that its spectrum of germline variation differs from other subtypes, and ethnic differences have been discovered (85). Using the promising effect of ML revealed in this research, we can develop more ML models to investigate the difference between Basal-like and TNBC subtypes, and distinguish the different subtypes of TNBC as a breakthrough in the treatment of refractory BRCA and precision treatment, such actively adopting neoadjuvant treatment preoperatively.
Our systematic review and meta-analysis comprehensively and systematically assessed the potential of gene transcriptome and radiomics-based ML models for predicting BRCA molecular subtypes, and demonstrated that the major advantage of ML is the ability to learn and develop algorithms without human intervention. Additionally, this research supported the application of virtual network deep learning and convolutional neural networks (CNN) to diagnose BRCA molecular subtypes using more fundamental artificial intelligence approaches. However, our study also has several limitations. The number of samples was small and there needs to be further continuous mining of relevant research to prove the accuracy of ML predictions. It also lacked external validation and enough testing sets for validation. To further improve the accuracy of ML for identifying molecular subtypes of BRCA, we need to be aware of the following. First, the reduction of data noise before ML application can improve model accuracy. Studies have shown that dimensionality reduction through the number of principal components for cross-validation, and the use of noise reduction techniques can significantly improve model accuracy (31). In the existing research, the application of cross-validation is not yet comprehensive, and noise reduction technology needs to be further improved. Second, the indications used for ML model training should be increased and function models should be continuously fine-tuned. One task of an ML model is to propose some predictors or features, and the other is to determine a function that can be used to associate eigenvalueswith disease prediction (class assignment) (86). Feature selection is the core of ML, and sufficient predictors guarantee improved accuracy of ML models, but this was limited by our lack of exploration of the mechanism of disease occurrence and development. Despite there being many options for functional models, the selection of optimal free parameter values to fit the model is not easy, implying that we need more training examples. This is both an opportunity and a challenge for ML to predict the development of molecular subtypes of BRCA. The treatment of BRCA is developing in the direction of precision medicine, and ML models are of great significance for de-escalation therapy of minimally invasive techniques, surgery, and chemotherapy.
Conclusions
Data from this systematic review and meta-analysis support radiomics and gene-transcriptomic ML model analyses, which have ideal predictive accuracy in determining early the BRCA molecular subtypes. However, compared with radiomics, gene transcriptomics yielded better results although radiomics is simpler and more convenient in operation from a clinical point of view. As the paradigm shifts to individualized medicine with minimally invasive techniques, prospective translational oncology research may focus on the development of radiomic techniques.
Acknowledgments
Funding: None.
Footnote
Reporting Checklist: The authors have completed the MOOSE reporting checklist. Available at https://atm.amegroups.com/article/view/10.21037/atm-22-5986/rc
Conflicts of Interest: All authors have completed the ICMJE uniform disclosure form (available at https://atm.amegroups.com/article/view/10.21037/atm-22-5986/coif). The authors have no conflicts of interest to declare.
Ethical Statement: The authors are accountable for all aspects of the work in ensuring that questions related to the accuracy or integrity of any part of the work are appropriately investigated and resolved.
Open Access Statement: This is an Open Access article distributed in accordance with the Creative Commons Attribution-NonCommercial-NoDerivs 4.0 International License (CC BY-NC-ND 4.0), which permits the non-commercial replication and distribution of the article with the strict proviso that no changes or edits are made and the original work is properly cited (including links to both the formal publication through the relevant DOI and the license). See: https://creativecommons.org/licenses/by-nc-nd/4.0/.
References
- Sung H, Ferlay J, Siegel RL, et al. Global Cancer Statistics 2020: GLOBOCAN Estimates of Incidence and Mortality Worldwide for 36 Cancers in 185 Countries. CA Cancer J Clin 2021;71:209-49. [Crossref] [PubMed]
- Siegel RL, Miller KD, Fuchs HE, et al. Cancer statistics, 2022. CA Cancer J Clin 2022;72:7-33. [Crossref] [PubMed]
- Soerjomataram I, Bray F. Planning for tomorrow: global cancer incidence and the role of prevention 2020-2070. Nat Rev Clin Oncol 2021;18:663-72. [Crossref] [PubMed]
- Bleyer A, Welch HG. Effect of three decades of screening mammography on breast-cancer incidence. N Engl J Med 2012;367:1998-2005. [Crossref] [PubMed]
- de Kruijf EM, Bastiaannet E, Rubertá F, et al. Comparison of frequencies and prognostic effect of molecular subtypes between young and elderly breast cancer patients. Mol Oncol 2014;8:1014-25. [Crossref] [PubMed]
- Zhao S, Ma D, Xiao Y, et al. Molecular Subtyping of Triple-Negative Breast Cancers by Immunohistochemistry: Molecular Basis and Clinical Relevance. Oncologist 2020;25:e1481-91. [Crossref] [PubMed]
- Ades F, Zardavas D, Bozovic-Spasojevic I, et al. Luminal B breast cancer: molecular characterization, clinical management, and future perspectives. J Clin Oncol 2014;32:2794-803. [Crossref] [PubMed]
- Comprehensive molecular portraits of human breast tumours. Nature 2012;490:61-70. [Crossref] [PubMed]
- Finsterbusch K, Decker T, van Diest PJ, et al. Luminal A versus luminal B breast cancer: MammaTyper mRNA versus immunohistochemical subtyping with an emphasis on standardised Ki67 labelling-based or mitotic activity index-based proliferation assessment. Histopathology 2020;76:650-60. [Crossref] [PubMed]
- Holloway RW, Marignani PA. Targeting mTOR and Glycolysis in HER2-Positive Breast Cancer. Cancers (Basel) 2021.
- Chen Y, Liu L, Ni R, et al. Advances in HER2 testing. Adv Clin Chem 2019;91:123-62. [Crossref] [PubMed]
- Hamilton E, Shastry M, Shiller SM, et al. Targeting HER2 heterogeneity in breast cancer. Cancer Treat Rev 2021;100:102286. [Crossref] [PubMed]
- Song L, Chen X, Mi L, et al. Icariin-induced inhibition of SIRT6/NF-κB triggers redox mediated apoptosis and enhances anti-tumor immunity in triple-negative breast cancer. Cancer Sci 2020;111:4242-56. [Crossref] [PubMed]
- Bartoschek M, Oskolkov N, Bocci M, et al. Spatially and functionally distinct subclasses of breast cancer-associated fibroblasts revealed by single cell RNA sequencing. Nat Commun 2018;9:5150. [Crossref] [PubMed]
- Curtis C, Shah SP, Chin SF, et al. The genomic and transcriptomic architecture of 2,000 breast tumours reveals novel subgroups. Nature 2012;486:346-52. [Crossref] [PubMed]
- Yang D, Jones MG, Naranjo S, et al. Lineage tracing reveals the phylodynamics, plasticity, and paths of tumor evolution. Cell 2022;185:1905-1923.e25. [Crossref] [PubMed]
- Parker JS, Mullins M, Cheang MC, et al. Supervised risk predictor of breast cancer based on intrinsic subtypes. J Clin Oncol 2009;27:1160-7. [Crossref] [PubMed]
- Mavrommati I, Johnson F, Echeverria GV, et al. Subclonal heterogeneity and evolution in breast cancer. NPJ Breast Cancer 2021;7:155. [Crossref] [PubMed]
- Golub TR, Slonim DK, Tamayo P, et al. Molecular classification of cancer: class discovery and class prediction by gene expression monitoring. Science 1999;286:531-7. [Crossref] [PubMed]
- Handelman GS, Kok HK, Chandra RV, et al. eDoctor: machine learning and the future of medicine. J Intern Med 2018;284:603-19. [Crossref] [PubMed]
- Greener JG, Kandathil SM, Moffat L, et al. A guide to machine learning for biologists. Nat Rev Mol Cell Biol 2022;23:40-55. [Crossref] [PubMed]
- Patel L, Shukla T, Huang X, et al. Machine Learning Methods in Drug Discovery. Molecules 2020;25:5277. [Crossref] [PubMed]
- Kassahun Y, Yu B, Tibebu AT, et al. Surgical robotics beyond enhanced dexterity instrumentation: a survey of machine learning techniques and their role in intelligent and autonomous surgical actions. Int J Comput Assist Radiol Surg 2016;11:553-68. [Crossref] [PubMed]
- Borstelmann SMMachine Learning Principles for Radiology Investigators. Acad Radiol 2020;27:13-25. [Crossref] [PubMed]
- Gould MK, Huang BZ, Tammemagi MC, et al. Machine Learning for Early Lung Cancer Identification Using Routine Clinical and Laboratory Data. Am J Respir Crit Care Med 2021;204:445-53. [Crossref] [PubMed]
- Liu Z, Liu L, Weng S, et al. Machine learning-based integration develops an immune-derived lncRNA signature for improving outcomes in colorectal cancer. Nat Commun 2022;13:816. [Crossref] [PubMed]
- Nketiah GA, Bathen TF. Editorial for "MRI Radiomics-Based Machine Learning for Predict of Clinically Significant Prostate Cancer in Equivocal PI-RADS 3 Lesions". J Magn Reson Imaging 2021;54:1474-5. [Crossref] [PubMed]
- Ahuja A, Al-Zogbi L, Krieger A. Application of noise-reduction techniques to machine learning algorithms for breast cancer tumor identification. Comput Biol Med 2021;135:104576. [Crossref] [PubMed]
- Sammut SJ, Crispin-Ortuzar M, Chin SF, et al. Multi-omic machine learning predictor of breast cancer therapy response. Nature 2022;601:623-9. [Crossref] [PubMed]
- Li X, Qin G, He Q, et al. Digital breast tomosynthesis versus digital mammography: integration of image modalities enhances deep learning-based breast mass classification. Eur Radiol 2020;30:778-88. [Crossref] [PubMed]
- Alom MZ, Yakopcic C, Nasrin MS, et al. Breast Cancer Classification from Histopathological Images with Inception Recurrent Residual Convolutional Neural Network. J Digit Imaging 2019;32:605-17. [Crossref] [PubMed]
- Davey MG, Davey MS, Boland MR, et al. Radiomic differentiation of breast cancer molecular subtypes using pre-operative breast imaging - A systematic review and meta-analysis. Eur J Radiol 2021;144:109996. [Crossref] [PubMed]
- Fleuren LM, Klausch TLT, Zwager CL, et al. Machine learning for the prediction of sepsis: a systematic review and meta-analysis of diagnostic test accuracy. Intensive Care Med 2020;46:383-400. [Crossref] [PubMed]
- Bellou V, Belbasis L, Konstantinidis AK, et al. Prognostic models for outcome prediction in patients with chronic obstructive pulmonary disease: systematic review and critical appraisal. BMJ 2019;367:l5358. [Crossref] [PubMed]
- Debray TP, Damen JA, Riley RD, et al. A framework for meta-analysis of prediction model studies with binary and time-to-event outcomes. Stat Methods Med Res 2019;28:2768-86. [Crossref] [PubMed]
- Ma M, Liu R, Wen C, et al. Predicting the molecular subtype of breast cancer and identifying interpretable imaging features using machine learning algorithms. Eur Radiol 2022;32:1652-62. [Crossref] [PubMed]
- Leithner D, Mayerhoefer ME, Martinez DF, et al. Non-Invasive Assessment of Breast Cancer Molecular Subtypes with Multiparametric Magnetic Resonance Imaging Radiomics. J Clin Med 2020;9:1853. [Crossref] [PubMed]
- Chen L, Zeng T, Pan X, et al. Identifying Methylation Pattern and Genes Associated with Breast Cancer Subtypes. Int J Mol Sci 2019;20:4269. [Crossref] [PubMed]
- Zhao Y, Pan Z, Namburi S, et al. CUP-AI-Dx: A tool for inferring cancer tissue of origin and molecular subtype using RNA gene-expression data and artificial intelligence. EBioMedicine 2020;61:103030. [Crossref] [PubMed]
- Huang CC, Tu SH, Huang CS, et al. Multiclass prediction with partial least square regression for gene expression data: applications in breast cancer intrinsic taxonomy. Biomed Res Int 2013;2013:248648. [Crossref] [PubMed]
- Yu Z, Wang Z, Yu X, et al. RNA-Seq-Based Breast Cancer Subtypes Classification Using Machine Learning Approaches. Comput Intell Neurosci 2020;2020:4737969. [Crossref] [PubMed]
- Liu T, Huang J, Liao T, et al. A hybrid deep learning model for predicting molecular subtypes of human breast cancer using multimodal data. IRBM 2022;43:62-74. [Crossref]
- Adabor ES, Acquaah-Mensah GK. Machine learning approaches to decipher hormone and HER2 receptor status phenotypes in breast cancer. Brief Bioinform 2019;20:504-14. [Crossref] [PubMed]
- Huang Y, Wei L, Hu Y, et al. Multi-Parametric MRI-Based Radiomics Models for Predicting Molecular Subtype and Androgen Receptor Expression in Breast Cancer. Front Oncol 2021;11:706733. [Crossref] [PubMed]
- Lopez-Rincon A, Mendoza-Maldonado L, Martinez-Archundia M, et al. Machine Learning-Based Ensemble Recursive Feature Selection of Circulating miRNAs for Cancer Tumor Classification. Cancers (Basel) 2020;12:1785. [Crossref] [PubMed]
- Wu J, Hicks C. Breast Cancer Type Classification Using Machine Learning. J Pers Med 2021;11:61. [Crossref] [PubMed]
- Mohaiminul Islam M, Huang S, Ajwad R, et al. An integrative deep learning framework for classifying molecular subtypes of breast cancer. Comput Struct Biotechnol J 2020;18:2185-99. [Crossref] [PubMed]
- Wilson TR, Xiao Y, Spoerke JM, et al. Development of a robust RNA-based classifier to accurately determine ER, PR, and HER2 status in breast cancer clinical samples. Breast Cancer Res Treat 2014;148:315-25. [Crossref] [PubMed]
- Seo MK, Paik S, Kim S. An Improved, Assay Platform Agnostic, Absolute Single Sample Breast Cancer Subtype Classifier. Cancers (Basel) 2020;12:3506. [Crossref] [PubMed]
- Couture HD, Williams LA, Geradts J, et al. Image analysis with deep learning to predict breast cancer grade, ER status, histologic subtype, and intrinsic subtype. NPJ Breast Cancer 2018;4:30. [Crossref] [PubMed]
- Fan M, Li H, Wang S, et al. Radiomic analysis reveals DCE-MRI features for prediction of molecular subtypes of breast cancer. PLoS One 2017;12:e0171683. [Crossref] [PubMed]
- Jiang M, Zhang D, Tang SC, et al. Deep learning with convolutional neural network in the assessment of breast cancer molecular subtypes based on US images: a multicenter retrospective study. Eur Radiol 2021;31:3673-82. [Crossref] [PubMed]
- Moon WK, Huang YS, Lo CM, et al. Computer-aided diagnosis for distinguishing between triple-negative breast cancer and fibroadenomas based on ultrasound texture features. Med Phys 2015;42:3024-35. [Crossref] [PubMed]
- Talari ACS, Rehman S, Rehman IU. Advancing cancer diagnostics with artificial intelligence and spectroscopy: identifying chemical changes associated with breast cancer. Expert Rev Mol Diagn 2019;19:929-40. [Crossref] [PubMed]
- Nie Z, Wang J, Ji XC. Retracted: Microcalcification-associated breast cancer: HER2-enriched molecular subtype is associated with mammographic features. Br J Radiol 2021; Epub ahead of print. [Crossref] [PubMed]
- Zhou J, Jin AQ, Zhou SC, et al. Application of preoperative ultrasound features combined with clinical factors in predicting HER2-positive subtype (non-luminal) breast cancer. BMC Med Imaging 2021;21:184. [Crossref] [PubMed]
- Fan M, Zhang P, Wang Y, et al. Radiomic analysis of imaging heterogeneity in tumours and the surrounding parenchyma based on unsupervised decomposition of DCE-MRI for predicting molecular subtypes of breast cancer. Eur Radiol 2019;29:4456-67. [Crossref] [PubMed]
- Wu M, Liu L, Chan C. Identification of novel targets for breast cancer by exploring gene switches on a genome scale. BMC Genomics 2011;12:547. [Crossref] [PubMed]
- Zhou J, Tan H, Li W, et al. Radiomics Signatures Based on Multiparametric MRI for the Preoperative Prediction of the HER2 Status of Patients with Breast Cancer. Acad Radiol 2021;28:1352-60. [Crossref] [PubMed]
- Wu T, Sultan LR, Tian J, et al. Machine learning for diagnostic ultrasound of triple-negative breast cancer. Breast Cancer Res Treat 2019;173:365-73. [Crossref] [PubMed]
- Ma W, Zhao Y, Ji Y, et al. Breast Cancer Molecular Subtype Prediction by Mammographic Radiomic Features. Acad Radiol 2019;26:196-201. [Crossref] [PubMed]
- Wang W, Zhang X, Zhu L, et al. Prediction of Prognostic Factors and Genotypes in Patients With Breast Cancer Using Multiple Mathematical Models of MR Diffusion Imaging. Front Oncol 2022;12:825264. [Crossref] [PubMed]
- Wu J, Sun X, Wang J, et al. Identifying relations between imaging phenotypes and molecular subtypes of breast cancer: Model discovery and external validation. J Magn Reson Imaging 2017;46:1017-27. [Crossref] [PubMed]
- Wu M, Zhong X, Peng Q, et al. Prediction of molecular subtypes of breast cancer using BI-RADS features based on a "white box" machine learning approach in a multi-modal imaging setting. Eur J Radiol 2019;114:175-84. [Crossref] [PubMed]
- Xie T, Wang Z, Zhao Q, et al. Machine Learning-Based Analysis of MR Multiparametric Radiomics for the Subtype Classification of Breast Cancer. Front Oncol 2019;9:505. [Crossref] [PubMed]
- Yang X, Wu L, Zhao K, et al. Evaluation of human epidermal growth factor receptor 2 status of breast cancer using preoperative multidetector computed tomography with deep learning and handcrafted radiomics features. Chin J Cancer Res 2020;32:175-85. [Crossref] [PubMed]
- Zhang X, Li H, Wang C, et al. Evaluating the Accuracy of Breast Cancer and Molecular Subtype Diagnosis by Ultrasound Image Deep Learning Model. Front Oncol 2021;11:623506. [Crossref] [PubMed]
- Zhang Y, Chen JH, Lin Y, et al. Prediction of breast cancer molecular subtypes on DCE-MRI using convolutional neural network with transfer learning between two centers. Eur Radiol 2021;31:2559-67. [Crossref] [PubMed]
- Zhou J, Tan H, Bai Y, et al. Evaluating the HER-2 status of breast cancer using mammography radiomics features. Eur J Radiol 2019;121:108718. [Crossref] [PubMed]
- Saha A, Harowicz MR, Grimm LJ, et al. A machine learning approach to radiogenomics of breast cancer: a study of 922 subjects and 529 DCE-MRI features. Br J Cancer 2018;119:508-16. [Crossref] [PubMed]
- Sutton EJ, Dashevsky BZ, Oh JH, et al. Breast cancer molecular subtype classifier that incorporates MRI features. J Magn Reson Imaging 2016;44:122-9. [Crossref] [PubMed]
- Wang F, Wang D, Xu Y, et al. Potential of the Non-Contrast-Enhanced Chest CT Radiomics to Distinguish Molecular Subtypes of Breast Cancer: A Retrospective Study. Front Oncol 2022;12:848726. [Crossref] [PubMed]
- Wang J, Kato F, Oyama-Manabe N, et al. Identifying Triple-Negative Breast Cancer Using Background Parenchymal Enhancement Heterogeneity on Dynamic Contrast-Enhanced MRI: A Pilot Radiomics Study. PLoS One 2015;10:e0143308. [Crossref] [PubMed]
- Arslan E, Schulz J, Rai K. Machine Learning in Epigenomics: Insights into Cancer Biology and Medicine. Biochim Biophys Acta Rev Cancer 2021;1876:188588. [Crossref] [PubMed]
- Li J, Lenferink AE, Deng Y, et al. Identification of high-quality cancer prognostic markers and metastasis network modules. Nat Commun 2010;1:34. [Crossref] [PubMed]
- Licht JD. DNA Methylation Inhibitors in Cancer Therapy: The Immunity Dimension. Cell 2015;162:938-9. [Crossref] [PubMed]
- Davey MG, Davies M, Lowery AJ, et al. The Role of MicroRNA as Clinical Biomarkers for Breast Cancer Surgery and Treatment. Int J Mol Sci 2021;22:8290. [Crossref] [PubMed]
- Badu-Peprah A, Adu-Sarkodie Y. Accuracy of clinical diagnosis, mammography and ultrasonography in preoperative assessment of breast cancer. Ghana Med J 2018;52:133-9. [Crossref] [PubMed]
- Weledji EP, Tambe J. Breast cancer detection and screening. Med Clin Rev 2018;4:8. [Crossref]
- From The American Association Of Neurological Surgeons (AANS). Multisociety Consensus Quality Improvement Revised Consensus Statement for Endovascular Therapy of Acute Ischemic Stroke. Int J Stroke 2018;13:612-32. [PubMed]
- Garrido-Castro AC, Lin NU, Polyak K. Insights into Molecular Classifications of Triple-Negative Breast Cancer: Improving Patient Selection for Treatment. Cancer Discov 2019;9:176-98. [Crossref] [PubMed]
- Miglietta F, Dieci MV, Griguolo G, et al. Neoadjuvant approach as a platform for treatment personalization: focus on HER2-positive and triple-negative breast cancer. Cancer Treat Rev 2021;98:102222. [Crossref] [PubMed]
- Kreike B, van Kouwenhove M, Horlings H, et al. Gene expression profiling and histopathological characterization of triple-negative/basal-like breast carcinomas. Breast Cancer Res 2007;9:R65. [Crossref] [PubMed]
- Borri F, Granaglia A. Pathology of triple negative breast cancer. Semin Cancer Biol 2021;72:136-45. [Crossref] [PubMed]
- Erratum to. Molecular features and functional implications of germline variants in triple-negative breast cancer. J Natl Cancer Inst 2022;114:482. [Crossref] [PubMed]
- Deo RC. Machine Learning in Medicine. Circulation 2015;132:1920-30. [Crossref] [PubMed]