Analysis and identification of potential key genes in hepatic ischemia-reperfusion injury
Highlight box
Key findings
• A total of 6 key genes (i.e., Krt14, Upk3b, Krt7, Cdh3, Msln, and Gpc3) were identified. Our findings provide novel ideas for the diagnosis and treatment of HIRI.
What is known and what is new?
• HIRI is an unavoidable surgical complication after liver transplantation, but current HIRI treatments cannot achieve satisfactory clinical outcomes.
• In our study, we identified 256 DEGs and 67 DE-lncRNAs between the HIRI and control samples by transcriptome sequencing data. 6 genes (i.e., Krt14, Upk3b, Krt7, Cdh3, Msln, and Gpc3) in the RF model were defined as the key genes. Moreover, a lncRNA-miRNA-mRNA network was constructed with 4 lncRNAs, 5 mRNAs, and 11 miRNAs.
What is the implication, and what should change now?
• In our study, we identified 6 key genes closely related to HIRI via a bioinformatics analysis using transcriptome data. We also constructed lncRNA-miRNA-mRNA networks through a PPI analysis. Our findings provide novel insights into the diagnosis and treatment of HIRI. We will conduct in-depth studies to explore the role and mechanism of 6 key genes in HIRI.
Introduction
Liver transplantation and liver resection are the main treatments for end-stage liver diseases, including liver cancer and cirrhosis (1,2). These procedures require a period of hepatic ischemia, and the partial or total occlusion of hepatic blood flow is a routine procedure. When hepatic blood flow is restored, the already ischemic liver is further damaged, a phenomenon which is known as hepatic ischemia-reperfusion injury (HIRI) (3,4). When HIRI occurs, it rapidly causes an acute inflammatory response in the liver, resulting in significant liver cell damage and liver dysfunction, and multiple organ failure and even death in severe cases (5).
HIRI is a complex and multifactorial process, and its specific mechanism remains unclear. However, recent studies have shown that important factors affecting the occurrence of HIRI include calcium overload, Kupffer cells, or related factors, reactive oxygen species (ROS) production or neutrophil aggregation, endothelin or nitric oxide (NO) concentration imbalance, and complement activation (4,5). Chang et al. found that in liver ischemia, the hepatic cells are in a hypoxic environment, and the consumption of adenosine triphosphate (ATP) increases the level of intracellular free calcium, thereby activating a variety of phospholipases, proteases, ATPases, and endonucleases, which in turn leads to the degradation of membrane proteins, the disruption of membrane structures, and deoxyribonucleic acid (DNA) damage (6). Qiao et al. found that Kupffer cells are important regulators of HIRI, and mediate inflammatory responses by secreting and recruiting inflammatory factors and chemokines, such as tumor necrosis factor alpha and nuclear factor kappa B, causing damage to hepatocytes (7). Abu-Amara et al. found that during HIRI, Kupffer and neutrophils release a large amount of ROS, which causes further damage to hepatocytes (8). Li et al. found that the imbalance of endothelin and NO concentrations further aggravates the constriction of microvascular, and the irreversible disturbance of microcirculation further aggravates the liver damage (9). Marshall et al. found that the membrane attack complex that is formed after complement activation is the main mediator of HIRI, and affects hepatocyte regeneration after HIRI (10). The mechanism of HIRI is complex, and involves multiple lncRNAs and mRNAs. The roles of lncRNAs such as CCAT1, MALAT1and NEAT1 have been established in HIRI. In addition, numerous mRNAs are associated with apoptosis, autophagy, oxidative stress and cellular inflammation that accompany HIRI pathogenesis. Based on the literature, we conclude that four lncRNA-mRNA regulatory networks mediate the pathological progression of HIRI.
At present, the intervention methods for HIRI mainly include ischemic preconditioning, mild hypothermia, hydrogen sulfide, hydrogen gas, and drug preconditioning (7,11). These measures can increase the tolerance of the liver to HIRI to a certain extent, thereby reducing the damage. However, HIRI remains the leading cause of early transplantation dysfunction and the primary non-functioning of the liver. Thus, prevention and treatment targets for HIRI need to be developed and the mechanism of HIRI needs to be further clarified to improve the prognosis of patients.
In the current study, we first identified a total of 256 differentially expressed genes (DEGs) and 67 differentially expressed long non-coding ribonucleic acids (DE-lncRNAs) based on the sequencing results of the HIRI and control samples. Further, we explored the inter-correlation among the DEGs to construct a protein-protein interaction (PPI) network. We then identified 6 key genes [i.e., Keratin-14 (Krt14), Uroplakin 3B (Upk3b), Keratin 7 (Krt7), Cadherin 3 (Cdh3), mesothelin (Msln), and Glypican 3 (Gpc3)] to construct a random forest (RF) model, and analyzed the enrichment functions of these key genes by a gene set enrichment analysis (GSEA). Finally, we successfully constructed a lncRNA-microRNA (miRNA)-messenger RNA (mRNA) network related to HIRI to provide novel ideas for the diagnosis and treatment of HIRI. We present the following article in accordance with the ARRIVE reporting checklist (available at https://atm.amegroups.com/article/view/10.21037/atm-22-6171/rc).
Methods
Laboratory animals
We purchased 30 male specific pathogen free (SPF)-grade Sprague-Dawley rats, weighing 250–300 g, aged 10 weeks, from the Department of Laboratory Zoology, Kunming Medical University. Kunming Medical University, where the animal laboratory is located, has a license for the use of experimental animals. This research was approved by the Ethics Review Committee for Animal Experiments of Kunming Medical University (No. 2020-168), and was conducted in compliance with the institutional guidelines for the care and use of animals. A protocol was prepared before the study without registration.
Establishment of a rat model of hepatic ischemia-reperfusion
The rats were fasted for 8–12 hours without water. The rats were anesthetized with a 3% sodium pentobarbital intraperitoneal injection, and fixed in a supine position. The abdomen was then prepared, sterilized, and a drape was laid. An incision 4–5-cm in length (up to the xiphoid process, down to the umbilicus) was made along the midline white line of the abdomen. For the xiphoid process, the cephalad was pulled with vascular forceps, and the liver was probed with a wet cotton swab to fully expose the hepatic hilum. The hepatoduodenal ligament was carefully separated, and the falciform ligament of the liver, the left and right coronary ligament of the liver, the left triangular ligament of the liver, the perihepatic ligament, the hepatogastric ligament, and the teres hepatic ligament were cut out carefully with micro-scissors. The Glisson system (including the portal vein branches, hepatic artery, and bile duct) of the middle lobe and left lobe of the liver was bluntly dissected with microscopic-curved forceps. An atraumatic microvascular clamp was used to create ischemia, but blood flow to the right hepatic lobe was not blocked to prevent portal and gastrointestinal congestion. After blocking, the blocked liver lobes showed dark red changes. After 10 minutes, the non-injured microvascular clip was loosened to perform blood reperfusion in the ischemic area of the liver, and the color of the liver lobe was observed to change from dark red to bright red, which indicated blood recovery. After flushing the abdominal cavity with warm saline, the abdominal cavity was routinely closed layer by layer with a 3-0 line. After the operation, the rats were placed in a small sun and kept warm until they woke up. After waking up, the reactivity of the rats was observed, and they were put into the rat cage and fed normally.
Collection of rat liver tissue and blood samples
Blood samples and liver tissue were collected on the 1st postoperative day. After sampling, the rats were killed using the cervical vertebrae method, and they were centrally incinerated at a qualified institution.
Serum treatment: blood (5 mL) was collected in a gel-facilitated blood collection tube from the inferior vena cava of the rats, and then centrifuged (3,000 rpm, 15 min) to remove the supernatant, dispensed into 1.5 mL eppendorf tubes (EP) tubes and stored in a −80 ℃ refrigerator. After the samples were collected, they were uniformly tested.
Part of the fresh liver tissue block was put into a pre-prepared fixative solution (containing 10% formalin, and Bouin’s fixative solution) to denature and coagulate the tissue proteins to maintain the original morphological structure of the cells. The remaining liver tissue was cut into small pieces of 1 cm × 1 cm, placed in a 5-mL EP tube, and stored in a −80 ℃ refrigerator until enough samples were collected for unified detection.
RNA isolation and library construction
TRIzol reagents (Invitrogen, Carlsbad, CA, USA) were used to extract the total RNA from the Sprague-Dawley rats in this study in accordance with the manufacturer’s procedure. The NanoDrop (ND)-1000 (NanoDrop, Wilmington, DE, USA) was used to quantify the RNA amount and purity of each RNA sample. The Bioanalyzer 2100·(Agilent, CA, USA) with a RIN number >7.0 was applied to estimate the RNA integrity to ensure good RNA quality. Finally, the 2×150 bp paired-end sequencing (PE150) was performed on an illumine NovaseqTM 6000 (LC Biotechnology Co., Ltd., Hangzhou, China) in accordance with the manufacturer’s recommended protocol. Information about the transcriptome sequencing samples is listed in Table 1.
Table 1
Sample ID | Control | HIRI |
---|---|---|
YX1N-L4-A009 | 1 | 0 |
YX2N-L2-A010 | 1 | 0 |
YX3N-L4-A011 | 1 | 0 |
YX4N-L4-A012 | 1 | 0 |
YX5N-L2-A001 | 1 | 0 |
YX6N-L2-A002 | 1 | 0 |
YX7N-L4-A003 | 1 | 0 |
YX8N-L4-A004 | 1 | 0 |
YX9N-L2-A005 | 1 | 0 |
YX10N-L4-A006 | 1 | 0 |
YX1L-L2-A007 | 0 | 1 |
YX2L-L4-A008 | 0 | 1 |
YX3L-L2-A009 | 0 | 1 |
YX4L-L2-A010 | 0 | 1 |
YX5L-L2-A011 | 0 | 1 |
YX6L-L3-A012 | 0 | 1 |
YX7L-L2-A001 | 0 | 1 |
YX8L-L2-A002 | 0 | 1 |
YX9L-L4-A003 | 0 | 1 |
YX10L-L2-A004 | 0 | 1 |
HIRI, hepatic ischemia-reperfusion injury.
Sequencing data processing
The sequencing data quality assessment was performed using the software FastQC (v0.11.9). Next, the raw data were pre-processed using the software Trimmomatic (v0.39) to filter out low-quality data, and the contamination and linker sequences were removed and the clean data were obtained. The filtered sequencing Clean Data were compared with the reference genome (GRCh38) using hisat2 (v2.2.1). After the above analysis, a gene expression matrix was obtained for the subsequent analysis.
Screening of DEGs and DE-lncRNAs
Transcriptome mRNA sequencing data and lncRNA sequencing data were used to identify DEGs and DE-lncRNAs between the HIRI and control (HIRI vs. control) samples using the DESeq2 package (version 1.33.5). The screening criteria were as follows: a |log2 fold change (FC)| value ≥0.5, and a P value <0.05. Volcano maps were drawn using the ggplot2 package (version 3.3.3) (H. Wickham. Ggplot2: Elegant Graphics for Data Analysis, Springer-Verlag New York, 2016) to show the DEGs and DE-lncRNAs, and heatmaps were drawn using the pheatmap package (Raivo Kolde, 2019, Pheatmap: Pretty Heatmaps. R package version 1.0.12. https://CRAN.R-project.org/package=pheatmap) to show the expression patterns of the top 50 DEGs and DE-lncRNAs.
Functional enrichment analyses of the DEGs
To further explore the pathways targeted by the DEGs and their functions, Gene Ontology (GO) and Kyoto Encyclopedia of Genes and Genomes (KEGG) enrichment analyses were performed on the DEGs using the clusterProfiler package (version 3.18.0) (12) (a P value <0.05, and a count value ≥2). The enrichplot package (version 1.10.2) and ggplot2 package (version 3.3.3) (H. Wickham. Ggplot2: Elegant Graphics for Data Analysis, Springer-Verlag New York, 2016) were used to visualize the enrichment results by plotting bar graphs and bubble plots.
The construction of the PPI network and the screening of the key genes
To investigate the interactions between the DEGs, a PPI network was constructed for the DEGs using the Search Tool for the Retrieval of Interacting Genes/Proteins (STRING; https://www.string-db.org/) database. The network structure was further analyzed using the cytoHubba plugins. The top 30 genes were identified separately using the maximal clique centrality (MCC), maximum neighborhood component (MNC), Degree and Closeness algorithms, and the intersection was taken between these 4 algorithms using UpSetR (version 1.4.0) {Nils Gehlenborg [2019]. UpSetR: A More Scalable Alternative to Venn and Euler Diagrams for Visualizing Intersecting Sets. R package version 1.4.0. https://CRAN.R-project.org/package=UpSetR}. The intersecting genes were defined as the candidate genes. VennDiagram (version 1.6.20) was used to draw the Venn diagram for display, and Cytoscape software (13) was used to visualize the network.
The screening of the key genes by machine learning
Based on the mRNA expression data and grouping information, the receiver operating characteristic (ROC) curves of the 21 candidate genes were plotted using pROC (version 1.17.0.1) (14) to evaluate the diagnostic value of the candidate genes for HIRI. Further, a RF model, support vector machine (SVM) model and generalized linear model (GLM) were constructed based on the candidate genes with areas under the curve (AUCs) >0.8 using the “caret” package (version 6.0-86). Additionally, the 3 models were analyzed interpretively using the explain function of the DALEX package (version 2.3.0), and the model performance distribution was visualized using the plot function. The cumulative residual distribution and the box plot distribution were both plotted, and the optimal model was obtained based on the data set.
GSEA and the functional network of the key genes
To identify the enriched pathways in the high expression groups, the R language clusterProfiler (version 3.18.1) (12) and the org.Hs.eg.db (version 3.12.0) packages were used for the GSEA of the key genes in the high expression groups. First, a correlation analysis was performed between the key genes and other genes, and the correlation coefficients between the genes were obtained and ranked. Next, a GSEA was conducted using the sequencing results to obtain the GSEA enrichment results for the high expression risk group of the key genes. The results of GSEA enrichment results were visualized using enrichplot (version 1.10.2).
The construction of the lncRNA-miRNA-mRNA network
To predict the miRNA-mRNA network of the key genes, the miRWalk database (http://mirwalk.umm.uni-heidelberg.de/) was used to predict the miRNA-mRNA targeting relationships (bindingp =1). A Pearson correlation analysis was performed of the key genes and DE-lncRNAs, and the relationships with a |correlation| value >0.4 and a P value <0.05 were screened for co-expression network construction for imported Cytoscape.
To predict the lncRNA-miRNAmiRNA-mRNA network of the key genes, the targeting relationship between lncRNA-miRNA was predicted using miRanda. The downregulated lncRNAs in the lncRNA-mRNA network were extracted, and the fasta sequences were downloaded from The National Center for Biotechnology Information (NCBI, https://www.ncbi.nlm.nih.gov/), and the fasta sequences of miRNAs in the miRNA-mRNA network were also downloaded. Next, the binding sites between lncRNA and miRNA were predicted using the linux system (energy <−2,000). Finally, the targeting relationship between lncRNA/miRNA/mRNA obtained from this study was introduced into Cytoscape (version 3.8.2) to construct the lncRNA-miRNA-mRNA network.
Quantitative real-time polymerase chain reaction (qRT-PCR)
The total RNA was extracted from the whole blood of the HIRI and control samples by TRIzol Reagent (Thermo Fisher, Shanghai, China). The sweScript RT I First strad cDNA SynthesisAll-in-OneTM First-Strand cDNA Synthesis Kit (Servicebio, Wuhan, China) was used for reverse transcription to form the complementary DNA (cDNA). Finally, PCR reactions were performed with the 2xUniversal Blue SYBR Green quantitative PCR (qPCR) Master Mix (Servicebio, Wuhan, China). The primers of the 6 genes were as Table 2.
Table 2
Gene | Sequence |
---|---|
Krt14-F | CAGAGCGGCAAGAGCGAGAT |
Krt14-R | TGGGCAGATGAAAGGTGAGC |
Upk3b-F | CACCCAAGGCTGAAACGAAG |
Upk3b-R | CCACCACAGGCTGGAGAAAC |
Krt7-F | CCATCCACTTCAGCTCTCGCT |
Krt7-R | TTGATCTGCTCACGCTCTTCC |
Msln-F | CAGGGTTTGCTGGTAGTGTTG |
Msln-R | CAGGCTTTCTGTTCTGTGTCC |
Gpc3-F | TAATCAACACCACCGACCACC |
Gpc3-R | TCGATCTCTACCACACCTGCC |
GAPDH-F | GACCCCTTCATTGACCTCAAC |
GAPDH-R | GCCATCACGCCACAGCTTTCC |
Results
Screening of the DEGs and DE-lncRNAs
A total of 256 DEGs were identified between the HIRI and control samples, of which 151 genes were upregulated in HIRI and 105 genes were downregulated in HIRI (Figure 1). In addition, 67 DE-lncRNAs were identified between the HIRI and control samples, of which 40 lncRNAs were upregulated in HIRI and 27 lncRNAs were downregulated in HIRI (Figure 2).
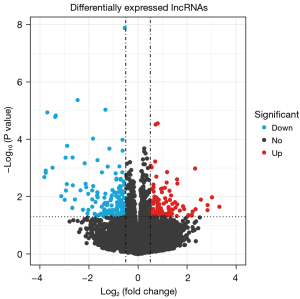
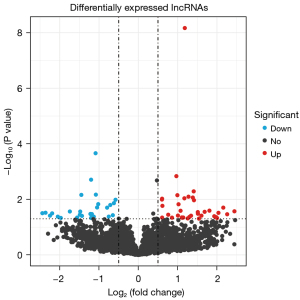
Functional enrichment analyses of the DEGs
These DEGs were enriched in 35 GO terms [26 GO-biological process (GO-BP) terms, 8 GO-molecular function (GO-MF) terms and 1 GO-cellular component (GO-CC) terms], and 23 KEGG pathways (Figure 3). In relation to the GO-BP terms, the DEGs were mainly enriched in the regulation of animal organ morphogenesis, cell-cell adhesion via plasma-membrane adhesion, and the retinoic acid metabolic process. In relation to the GO-CC terms, the DE-mRNAs were mainly enriched in. In relation to the GO-MF terms, the DE-mRNAs were mainly enriched in retinoic acid binding, cell adhesion molecule binding, and heparin binding. The KEGG pathways of the DEGs were mainly enriched in various immune, metabolism and disease pathways, such as the interleukin (IL)-17 signaling pathway, lipid and atherosclerosis, and retinol metabolism.
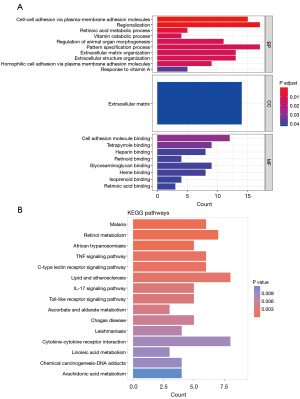
The construction of the PPI network and the screening of the key genes
To explore the interactions between the DEGs, a PPI network of 130 DEGs, including 130 nodes and 192 edges, was constructed according to the confidence =0.4 (Figure 4A). Further, 21 intersecting genes were obtained using the MCC, MNC, Degree and Closeness algorithms (Figure 4B-4D and Table 3). These 21 intersecting genes were regarded as the candidate genes.
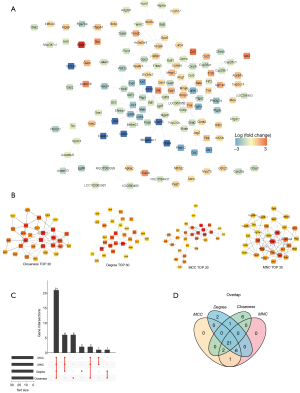
Table 3
Symbol | Log2 fold change | P value | P adj | Type |
---|---|---|---|---|
Ugt1a6 | 1.528962085 | 0.024128389 | 0.998514705 | Up |
Spp1 | 1.508182756 | 0.015504317 | 0.998514705 | Up |
Mmp12 | 1.401334208 | 0.017821287 | 0.998514705 | Up |
Mmp3 | 1.277583905 | 0.035363335 | 0.998514705 | Up |
Sele | 1.274716295 | 0.032317535 | 0.998514705 | Up |
Fos | 1.249888268 | 0.025874774 | 0.998514705 | Up |
Mmp9 | 0.728013312 | 0.034385731 | 0.998514705 | Up |
Gdnf | 0.620393431 | 0.017690685 | 0.998514705 | Up |
Ccl5 | −0.514414926 | 0.026197814 | 0.998514705 | Down |
Sulf1 | −0.63205502 | 0.043960916 | 0.998514705 | Down |
LOC367858 | −0.712485169 | 0.002550126 | 0.68878135 | Down |
Cdh3 | −0.718163935 | 0.022239937 | 0.998514705 | Down |
Wt1 | −0.747485202 | 0.03790684 | 0.998514705 | Down |
Gpc3 | −1.16752821 | 0.000211195 | 0.259249051 | Down |
Ptgs2 | −1.367480022 | 0.027630595 | 0.998514705 | Down |
Hoxb7 | −1.370483735 | 0.010785835 | 0.964075675 | Down |
Msln | −1.870466387 | 0.006056879 | 0.870234374 | Down |
Lhx1 | −2.026005136 | 0.028870994 | 0.998514705 | Down |
Upk3b | −2.465412969 | 4.28E-06 | 0.027744913 | Down |
Krt7 | −2.893247391 | 0.000167845 | 0.237733743 | Down |
Krt14 | −4.259830967 | 4.52E-06 | 0.027744913 | Down |
The screening of the key genes by machine learning
The AUCs of the 21 candidate genes obtained from the PPI network were explored. Among these 21 genes, the AUCs of 13 genes were >0.7 (Figure 5A). Further, the RF model, SVM model, and GLM were constructed based on these 13 genes, of which the RF model was the best model (Figure 5B,5C). These 6 genes (i.e., Krt14, Upk3b, Krt7, Cdh3, Msln, and Gpc3) of the RF model were defined as the key genes (Figure 5D).
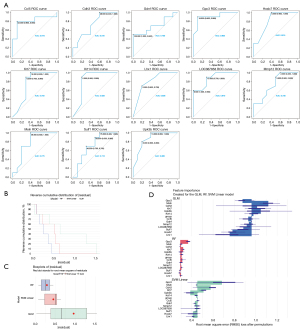
The GSEA and functional network of the key genes
A GO enrichment analysis was conducted to explore the potential functions of the key genes (Figure 6A). The extracellular structure organization and extracellular matrix organization were enriched in the high expression groups of Cdh3, Krt14, and Gpc3. The isoprenoid metabolic process, terpenoid metabolic process, and diterpenoid metabolic process were enriched in the high expression groups of Krt14, Krt7, Msln, and Upk3b. The fatty acid metabolic process and nucleoside bisphosphate metabolic process were enriched in the high expression groups of Krt7 and Upk3b.
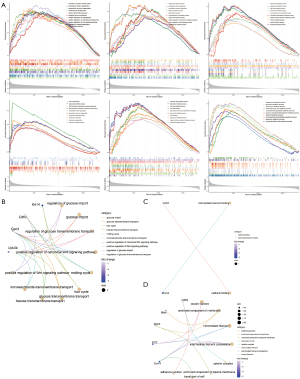
Moreover, in the GO-BP functional network of the key genes, 127 terms were enriched. The regulation of glucose import and the regulation of glucose transmembrane transport were enriched by GPC3 and UPK3B (Figure 6B). In the GO-MF functional network of the key genes, cadherin binding was enriched by CDH3, and intermediate filament binding was enriched by Krt14 (Figure 6C). In the GO-CC functional network of the key genes, a total of 8 terms were enriched. The keratin filament, intermediate filament and intermediate filament cytoskeleton were enriched by KRT7 and Krt14 (Figure 6D).
A lncRNA-miRNA-mRNA network was constructed for the 6 key genes
In total, 145 miRNAs were predicted by 5 key genes (Figure 7A). Additionally, 16 lncRNAs were predicted by 6 key genes (Figure 7B). Finally, a lncRNA-miRNA-mRNA network was constructed with 4 lncRNAs, 5 mRNAs, and 11 miRNAs (Figure 7C and Table 4). A total of 4 lncRNAs were shared among the 6 genes, such as LOC120102987-rno-miRNA-331-3P-Cdh3, LOC1201029870-rno-miRNA-128-5p-UPK3B, LOC120094223-rno-miRNA-92b-5p-KRT7.
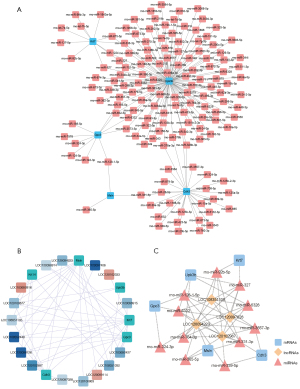
Table 4
Symbol | Log2 fold change | P value | P adj | Type |
---|---|---|---|---|
LOC120094223 | −1.218956169 | 0.033514812 | 0.993315091 | lncRNA |
LOC120097028 | −0.649070342 | 0.037224884 | 0.993315091 | lncRNA |
LOC108351105 | −0.752412323 | 0.041814558 | 0.993315091 | lncRNA |
LOC120102987 | −1.972318409 | 0.046572122 | 0.993315091 | lncRNA |
Upk3b | −2.465412969 | 4.28E-06 | 0.027744913 | mRNA |
Krt14 | −4.259830967 | 4.52E-06 | 0.027744913 | mRNA |
Krt7 | −2.893247391 | 0.000167845 | 0.237733743 | mRNA |
Gpc3 | −1.16752821 | 0.000211195 | 0.259249051 | mRNA |
Msln | −1.870466387 | 0.006056879 | 0.870234374 | mRNA |
Cdh3 | −0.718163935 | 0.022239937 | 0.998514705 | mRNA |
mRNA, messenger ribonucleic acid; lncRNA, long non-coding RNA.
qRT-PCR
A qRT-PCR was performed to verify the expression of the 5 key genes (Krt14, Upk3b, Krt7, Msln, and Gpc3). The expression of Krt14, Upk3b, Msln, and Gpc3 were more highly expressed in the control samples than the HIRI samples, while the expression of Krt7 was not significant (Figure 8).
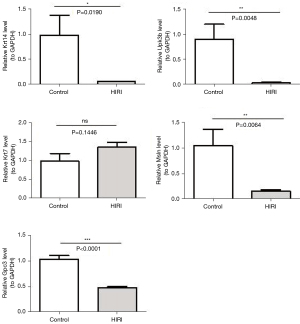
Discussion
HIRI is an inevitable complication of liver transplantation and liver resection, and severe cases can lead to acute liver dysfunction and liver failure after surgery (1,9). In the early ischemic phase, HIRI-induced liver damage results from metabolic disturbances. During the reperfusion phase, cytokines secreted after neutrophil accumulation and Kupffer cell activation aggravate HIRI injury (15-17). In the prevention and treatment of HIRI, targeted therapies, such as ischemic preconditioning, mild hypothermia treatment, antioxidant, anti-inflammatory and anti-apoptotic drugs, have not yet been integrated into HIRI therapy (16). Great efforts have been made to examine the mechanism of HIRI; however, the specific mechanism of HIRI is still unknown. Thus, it is of great importance that novel molecular regulatory targets for the diagnosis and treatment of HIRI are identified.
In our study, based on the 6 key genes (i.e., Krt14, Upk3b, Krt7, Cdh3, Msln, and Gpc3) identified from the HIRI and control samples, an RF model related to the diagnosis and prognosis of HIRI was constructed. Further, we also successfully constructed a lncRNA-miRNA-mRNA network, which provides further research directions for the molecular network of HIRI occurrence.
Krt14 is an intermediate filament protein that is normally expressed in basal epithelial progenitor cells located in the epithelial niche. These cells exhibit migratory behavior and self-renewal, produce multiple differentiated cells, and regulate branching structure during organogenesis (18,19). A study on breast cancer have clearly demonstrated that a subset of cells expressing Krt14 modulate polyclonal tumor deposit formation in vitro and in vivo (20). Upk3b encodes an integral membrane protein of the tetraspanin superfamily (21). Upks play an important role in the expansion and stabilization of the apical surface in the urothelium and contribute to mammalian urothelial permeability barrier function (22). Krt7 is a gene encoding type II keratin and is mainly expressed in epithelial cells. The major roles of keratin include maintaining cellular integrity and regulating cell growth, migration, and apoptosis in normal tissues and cancer tissues (23). Several studies have identified Krt7 as an immunohistochemical biomarker, and the overexpression of Krt7 is considered a poor prognostic factor in the variety of cancers, such as renal tumors, lung cancer, and intrahepatic cholangiocarcinoma (24,25). Cadherins are a large superfamily comprising 350 members. Currently, they are known to be involved in many BPs, such as cell signaling, cell recognition, morphogenesis, and angiogenesis. They play key roles in cell differentiation, growth and migration. Similar to other members of the cadherin family, Cdh3 plays a key role in cell growth and migration (26). Cdh3 is the key factor in the occurrence and progression of various malignant tumors, such as breast cancer, pancreatic cancer, and gastric cancer (27). MSLN is a cell surface glycoprotein expressed on normal mesothelial cells and mesothelioma (28). MSLN abnormally regulates tumor growth, invasion, and metastasis in tumors, such as mesothelioma, pancreatic cancer, and ovarian cancer (29,30). At present, it has become a hot target for tumor therapy, especially chimeric antigen receptor T-cell immunotherapy (CAR-T) cell immunotherapy for mesothelioma (31). GPC3 is a membrane-bound heparan sulfate proteoglycan located on chromosome Xq26. GPC3 is highly expressed during the fetal period, and its expression level is reduced after birth. GPC3 is overexpressed in hepatocellular carcinoma (HCC), embryonal tumors, melanoma, and some germ cell tumors, and is closely related to tumorigenesis and prognosis (32,33). These key genes play an important role in a variety of diseases, but their function in HIRI is still unknown.
In this study, we analyzed the functions of 6 key genes. We found that in terms of MFs, these genes are mainly involved in the regulation of extracellular structure organization and matrix organization and are closely related to the metabolism of various substances, such as fatty acid metabolism. Further, in terms of BFs, these genes are key molecules in the formation of cadherin and keratin, as well as the transport and regulation of glucose molecules. Thus, further exploration of the function and mediating mechanism of core genes will extend understandings of the molecular mechanism of HIRI. In addition, we successfully constructed HIRI-related lncRNA-miRNA-mRNA networks, such as LOC1201029870-rno-miRNA-331-3P-CDH3, LOC1201029870-rno-miRNA-128-5p-UPK3B, and LOC120094223-rno-miRNA-92b-5p-KRT7. These molecular networks may play an important role in HIRI, and further investigations are needed.
Currently, research has largely focused on the molecular targets and their mediated signaling pathways in HIRI. For example, SET8 alleviates mouse HIRI by inhibiting the microtubule affinity-regulating kinase 4 (MARK4)/nucleotide-binding oligomerization domain, leucine-rich repeat, and pyrin domain containing 3 (NLRP3) inflammasome pathway (34), and ARRB2 alleviates HIRI by activating the phosphatidylinositol 3 kinase (PI3K)/protein kinaseB (AKT) signaling pathway (35). Through a transcriptome analysis, our research identified the 6 key molecules closely related to HIRI, and successfully constructed the RF model of HIRI; in addition, we also successfully established a lncRNA-miRNA-mRNA network using a variety of bioinformatics analysis tools. Our findings provide novel ideas for the in-depth exploration of the diagnosis and treatment of HIRI. However, this study also had some limitations. The conclusions we draw are only based on transcriptome analysis, bioinformatics analysis and qRT-PCR experiments. The evidence is relatively weak, and a large number of molecular experiments and animal experiments are needed to verify our conclusion.
Conclusions
In our study, we identified 6 key genes closely related to HIRI via a bioinformatics analysis using transcriptome data. We also constructed lncRNA-miRNA-mRNA networks through a PPI analysis. Our findings provide novel insights into the diagnosis and treatment of HIRI.
Acknowledgments
Funding: None.
Footnote
Reporting Checklist: The authors have completed the ARRIVE reporting checklist. Available at https://atm.amegroups.com/article/view/10.21037/atm-22-6171/rc
Data Sharing Statement: Available at https://atm.amegroups.com/article/view/10.21037/atm-22-6171/dss
Conflicts of Interest: All authors have completed the ICMJE uniform disclosure form (available at https://atm.amegroups.com/article/view/10.21037/atm-22-6171/coif).The authors have no conflicts of interest to declare.
Ethical Statement: The authors are accountable for all aspects of the work in ensuring that questions related to the accuracy or integrity of any part of the work are appropriately investigated and resolved. This research was approved by the Ethics Review Committee for Animal Experiments of Kunming Medical University (No. 2020-168), and was conducted in compliance with the institutional guidelines for the care and use of animals.
Open Access Statement: This is an Open Access article distributed in accordance with the Creative Commons Attribution-NonCommercial-NoDerivs 4.0 International License (CC BY-NC-ND 4.0), which permits the non-commercial replication and distribution of the article with the strict proviso that no changes or edits are made and the original work is properly cited (including links to both the formal publication through the relevant DOI and the license). See: https://creativecommons.org/licenses/by-nc-nd/4.0/.
References
- Cannistrà M, Ruggiero M, Zullo A, et al. Hepatic ischemia reperfusion injury: A systematic review of literature and the role of current drugs and biomarkers. Int J Surg 2016;33:S57-70. [Crossref] [PubMed]
- Ye L, He S, Mao X, et al. Effect of Hepatic Macrophage Polarization and Apoptosis on Liver Ischemia and Reperfusion Injury During Liver Transplantation. Front Immunol 2020;11:1193. [Crossref] [PubMed]
- Guan Y, Yao W, Yi K, et al. Nanotheranostics for the Management of Hepatic Ischemia-Reperfusion Injury. Small 2021;17:e2007727. [Crossref] [PubMed]
- Saidi RF, Kenari SK. Liver ischemia/reperfusion injury: an overview. J Invest Surg 2014;27:366-79. [Crossref] [PubMed]
- Jiménez-Castro MB, Cornide-Petronio ME, Gracia-Sancho J, et al. Inflammasome-Mediated Inflammation in Liver Ischemia-Reperfusion Injury. Cells 2019;8:1131. [Crossref] [PubMed]
- Chang WJ, Chehab M, Kink S, et al. Intracellular calcium signaling pathways during liver ischemia and reperfusion. J Invest Surg 2010;23:228-38. [Crossref] [PubMed]
- Qiao YL, Qian JM, Wang FR, et al. Butyrate protects liver against ischemia reperfusion injury by inhibiting nuclear factor kappa B activation in Kupffer cells. J Surg Res 2014;187:653-9. [Crossref] [PubMed]
- Abu-Amara M, Yang SY, Tapuria N, et al. Liver ischemia/reperfusion injury: processes in inflammatory networks--a review. Liver Transpl 2010;16:1016-32. [Crossref] [PubMed]
- Li J, Li RJ, Lv GY, et al. The mechanisms and strategies to protect from hepatic ischemia-reperfusion injury. Eur Rev Med Pharmacol Sci 2015;19:2036-47.
- Marshall KM, He S, Zhong Z, et al. Dissecting the complement pathway in hepatic injury and regeneration with a novel protective strategy. J Exp Med 2014;211:1793-805. [Crossref] [PubMed]
- Long Y, Wei H, Li J, et al. Prevention of Hepatic Ischemia-Reperfusion Injury by Carbohydrate-Derived Nanoantioxidants. Nano Lett 2020;20:6510-9. [Crossref] [PubMed]
- Yu G, Wang LG, Han Y, et al. clusterProfiler: an R package for comparing biological themes among gene clusters. OMICS 2012;16:284-7. [Crossref] [PubMed]
- Shannon P, Markiel A, Ozier O, et al. Cytoscape: a software environment for integrated models of biomolecular interaction networks. Genome Res 2003;13:2498-504. [Crossref] [PubMed]
- Robin X, Turck N, Hainard A, et al. pROC: an open-source package for R and S+ to analyze and compare ROC curves. BMC Bioinformatics 2011;12:77. [Crossref] [PubMed]
- Kim D, Choi JW, Han S, et al. Ischemic Preconditioning Protects Against Hepatic Ischemia-Reperfusion Injury Under Propofol Anesthesia in Rats. Transplant Proc 2020;52:2964-9. [Crossref] [PubMed]
- Jaeschke H, Woolbright BL. Current strategies to minimize hepatic ischemia-reperfusion injury by targeting reactive oxygen species. Transplant Rev (Orlando) 2012;26:103-14. [Crossref] [PubMed]
- Dai Q, Jiang W, Liu H, et al. Kupffer cell-targeting strategy for the protection of hepatic ischemia/reperfusion injury. Nanotechnology 2021; [Crossref]
- Paraskevopoulou V, Papafotiou G, Klinakis A. KRT14 marks bladder progenitors. Cell Cycle 2016;15:3161-2. [Crossref] [PubMed]
- Jankowski M, Wertheim-Tysarowska K, Jakubowski R, et al. Novel KRT14 mutation causing epidermolysis bullosa simplex with variable phenotype. Exp Dermatol 2014;23:684-7. [Crossref] [PubMed]
- Bilandzic M, Rainczuk A, Green E, et al. Keratin-14 (KRT14) Positive Leader Cells Mediate Mesothelial Clearance and Invasion by Ovarian Cancer Cells. Cancers (Basel) 2019;11:1228. [Crossref] [PubMed]
- Kanamori-Katayama M, Kaiho A, Ishizu Y, et al. LRRN4 and UPK3B are markers of primary mesothelial cells. PLoS One 2011;6:e25391. [Crossref] [PubMed]
- Rudat C, Grieskamp T, Röhr C, et al. Upk3b is dispensable for development and integrity of urothelium and mesothelium. PLoS One 2014;9:e112112. [Crossref] [PubMed]
- Li Y, Su Z, Wei B, et al. KRT7 Overexpression is Associated with Poor Prognosis and Immune Cell Infiltration in Patients with Pancreatic Adenocarcinoma. Int J Gen Med 2021;14:2677-94. [Crossref] [PubMed]
- Wang W, Wang J, Yang C, et al. MicroRNA-216a targets WT1 expression and regulates KRT7 transcription to mediate the progression of pancreatic cancer-A transcriptome analysis. IUBMB Life 2021;73:866-82. [Crossref] [PubMed]
- Huang B, Song JH, Cheng Y, et al. Long non-coding antisense RNA KRT7-AS is activated in gastric cancers and supports cancer cell progression by increasing KRT7 expression. Oncogene 2016;35:4927-36. [Crossref] [PubMed]
- Zhou Y, Chi Y, Bhandari A, et al. Downregulated CDH3 decreases proliferation, migration, and invasion in thyroid cancer. Am J Transl Res 2020;12:3057-67.
- Xu Y, Zhao J, Dai X, et al. High expression of CDH3 predicts a good prognosis for colon adenocarcinoma patients. Exp Ther Med 2019;18:841-7. [Crossref] [PubMed]
- Sotoudeh M, Shirvani SI, Merat S, et al. MSLN (Mesothelin), ANTXR1 (TEM8), and MUC3A are the potent antigenic targets for CAR T cell therapy of gastric adenocarcinoma. J Cell Biochem 2019;120:5010-7. [Crossref] [PubMed]
- Yang X, Huang M, Zhang Q, et al. Metformin Antagonizes Ovarian Cancer Cells Malignancy Through MSLN Mediated IL-6/STAT3 Signaling. Cell Transplant 2021;30:9636897211027819. [Crossref] [PubMed]
- Li Y, Tian W, Zhang H, et al. MSLN Correlates With Immune Infiltration and Chemoresistance as a Prognostic Biomarker in Ovarian Cancer. Front Oncol 2022;12:830570. [Crossref] [PubMed]
- Schoutrop E, El-Serafi I, Poiret T, et al. Mesothelin-Specific CAR T Cells Target Ovarian Cancer. Cancer Res 2021;81:3022-35. [Crossref] [PubMed]
- Ning J, Jiang S, Li X, et al. GPC3 affects the prognosis of lung adenocarcinoma and lung squamous cell carcinoma. BMC Pulm Med 2021;21:199. [Crossref] [PubMed]
- Nishida T, Kataoka H. Glypican 3-Targeted Therapy in Hepatocellular Carcinoma. Cancers (Basel) 2019;11:1339. [Crossref] [PubMed]
- Luo Y, Huang Z, Mou T, et al. SET8 mitigates hepatic ischemia/reperfusion injury in mice by suppressing MARK4/NLRP3 inflammasome pathway. Life Sci 2021;273:119286. [Crossref] [PubMed]
- Chen X, Zhang J, Xia L, et al. β-Arrestin-2 attenuates hepatic ischemia-reperfusion injury by activating PI3K/Akt signaling. Aging (Albany NY) 2020;13:2251-63. [Crossref] [PubMed]
(English Language Editor: L. Huleatt)