Establishment and validation a prediction model for discrimination of invasive adenocarcinomas for patients with peripheral pulmonary subsolid nodules
Highlight box
Key findings
• This study developed and validated a nomogram model to evaluate the risk of the pathological invasiveness for patients with lung SSNs.
What is known and what is new?
• The current strategy for the management of SSNs tends to differ, and until now, there is no effective model to guide the surgical management.
• By the combination of five CT characteristic, we developed a new nomogram model which show robust discrimination performance in the validation cohort.
What is the implication, and what should change now?
• Our model may help clinicians to make individualized treatment for patients with SSNs.
Introduction
Adenocarcinoma is the most common histologic subtype of lung cancer with high heterogeneity (1). Since the International Association for the Study of Lung Cancer/American Thoracic Society/European Respiratory Society (IASLC/ATS/ERS) (1) introduced its multidisciplinary classification system, lung adenocarcinoma has been classified as atypical adenomatous hyperplasia (AAH), adenocarcinoma in situ (AIS), minimally invasive adenocarcinoma (MIA), and invasive adenocarcinoma (IA). At present, sublobar resection is favored for AIS/MIA, while lobectomy and lymph node dissection remain the standard treatment for IA (2,3). However, currently, it is still difficult to make a diagnosis of AIS and MIA with a frozen biopsy section, as the invasive component needs to be precisely evaluated by the entire pathologic specimen (1). In addition, immediate surgical resection could be obviated in cases of preinvasive lesions and MIAs, which may remain stable over several years (4). Subsolid nodules (SSNs), which consist of pure ground glass nodules and part solid nodules, are of growing clinical concern as they are frequently detected by computed tomography (CT) screening. Most common diagnosis for surgical resected SSNs is lung adenocarcinoma. Thus, it is necessary to construct an effective predictive model to evaluate the pathological invasiveness of SSNs.
At present, no definitive strategy has been proposed to determine the risk of pathological invasiveness of SSNs in medical settings. It has been reported that the overall size of the nodule and the solid component size are the 2 most important parameters in evaluating the pathological invasiveness of SSNs (5,6). The Fleischner Society (7) suggests that a 0.5-cm solid component threshold should be used to decide whether to adopt a follow-up strategy or evaluate the need for surgical resection. CT findings of ground glass versus solid opacities tend to correspond to the lepidic and invasive patterns, respectively, which can be observed pathologically. However, this correlation is not absolute. A benign scar or pulmonary vessels can also present as a solid area (8). Yanagawa et al. also claimed that a solid portion >0.8 cm on the lung window setting and/or >0.6 cm on the mediastinal window setting predicted pathologic invasiveness and could be used to differentiate IA from MIA and AIS (9). Discrimination of invasive adenocarcinomas among SSNs solely based on tumor size or solid component size is not always feasible.
Previous studies also have shown that CT attenuation values and CT morphology (10), such as pleura indentation and a bubble-like appearance (11-13), may help to differentiate between SSNs. In this study, we sought to identify the variables associated with the characterization of SSNs and establish a predictive model to evaluate the pathological invasiveness of SSNs based on CT features. We present the following article in accordance with the TRIPOD reporting checklist (available at https://atm.amegroups.com/article/view/10.21037/atm-22-5685/rc).
Methods
Patients
The data of patients with peripheral SSNs who underwent surgical resection from January 2014 to December 2018 at the Department of Thoracic Surgery, Shanghai Renji Hospital were retrospectively analyzed. All the patients had a definite pathological diagnosis. Any patients who had nodules <0.5 or >3 cm were excluded from our study. If a patient had multiple SSNs, the largest sized nodule was analyzed. Ultimately, 1,140 patients were included in the study. The study was conducted in accordance with the Declaration of Helsinki (as revised in 2013). The Ethics Committee of the Renji Hospital, Shanghai Jiaotong University School of Medicine approved the study (No. RA-2019-033), and informed consent was waived because the study was retrospective.
High-resolution CT evaluation
All the patients received a high-resolution CT scan within 1 month before surgical resection. The CT scans were conducted using a 64-detector CT row scanner (Brilliance 64; Philips, Eindhoven, The Netherlands). The baseline chest CT scans were performed from the lung apices to the middle pole of both kidneys. The images were reconstructed using soft tissue and lung algorithms with a thickness of 1 mm. The CT features were evaluated in the following settings: lung window center –600 Hu/lung window width –1,600 Hu; and mediastinal window center –40 Hu/mediastinal window width –350 Hu.
The descriptions of the CT images were evaluated independently by 3 experienced thoracic radiologists (with 25, 20, and 15 years of experience, respectively), who were blind to the pathological diagnoses. Nodule characteristics, including lesion position, diameter, solid component size, solid ratio, mean CT attenuation, multiplicity, lobulation, spiculation, vascular convergence, air bronchogram sign, bubble lucency, and pleural indentation, were assessed and recorded. In our study, the size of the SSNs was determined by measuring the maximal diameter of the SSNs in the transverse plane on the lung window setting using electronic calipers. The solid component sizes were determined by measuring the maximal diameter of the solid components in the lung window settings. If a patient had multiple solid components, the size of the single largest solid component was recorded as recommended by Travis et al. (8). The solid ratio was calculated by dividing the solid component size by the nodule size. Mean CT attenuation was measured using the region-of-interest cursors, which traced the edge of the tumor on the slices containing the region of the lesion with the maximum diameter (5). Multiplicity was defined as the presence of >1 nodule (including both SSNs and solid nodules) that were >5 mm in size.
Pathological diagnosis
All the patients underwent surgical resection using the thoracoscopic approach. All the pathologic specimens were fixed in 10% formalin and embedded in paraffin. The histological evaluations were conducted using hematoxylin and eosin staining slides. A histopathologic analysis was performed using the 2011 IASLC/ATS/ERS multidisciplinary classification system for lung adenocarcinoma (8). NIA includes AAH, AIS, and MIA. Invasive adenocarcinoma includes only IA.
Statistical analysis
Two-thirds of the eligible patients were randomly selected and assigned to the training cohort, while the remaining patients were assigned to the validation cohort. The quantitative variables were evaluated by the independent sample t-test, while the qualitative variables were evaluated by the chi square test. The variables with statistically significant differences (P<0.1) in the univariate analysis were included in the multivariate logistic regression analysis. The nomogram model for differentiating IA from NIA was developed with the variables with a P value <0.05 according to the results of the multivariate analysis.
In the validation cohort, the predictive performance of the nomogram model was evaluated by using the area under the receiver operator characteristic curves (AUC). Generally, AUC values of less than 0.6 were regarded as poor, 0.6–0.9 were rated moderate, and >0.9 were considered excellent. The calibration of the prediction model for the training cohort and validation cohort were performed using visual calibration curves with 1,000 bootstrap resamples. We compared the AUC of our nomogram model to the AUCs of Hyungjin Kim’s model (6), and a “solid size >5 mm” model based on the Fleischner Society’s recommendation (7) that a solid size >5 mm on the CT scan is an indication of pathological invasives.
Finally, we calculated the net benefit (NB) using a decision curve analysis (DCA). The DCA was developed to evaluate and compare diagnostic and prediction models by integrating the clinical consequences of false positives (14). It subtracts the proportion of all patients who are false-positive from the proportion of all patients who are true-positive; thus, weighing the relative harm of a false-positive and a false-negative result (15). The following formula was used:
where n is the total number of patients included in the study and Pt is the probability threshold.
The statistical analysis was performed using SPSS software, version 22 (IBM Corp., Armonk, NY, USA) and R software, version 3.1.0 (http://www.R-project.org; package: rms, ggplot2 and rmda). The statistical tests were two-sided, with P<0.05 indicating statistical significance.
Results
The characteristics of the 1,140 participants with SSNs are described in Table 1. Overall, 327 (28.7%) male participants and 813 (71.3%) females with a mean age of 58.3±11.9 years were included in the study. Among them, 760 patients were assigned to the training cohort, and 380 patients were assigned to the validation cohort. There was no significant difference in terms of the patient or tumor characteristics between the training and validation cohorts.
Table 1
Characteristics | Overall cohort (n=1,140) | Training cohort (n=760) | Validation cohort (n=380) | P |
---|---|---|---|---|
Clinical characters | ||||
Male (%) | 327 (28.7) | 220 (28.9) | 107 (28.2) | 0.781 |
Age (year, mean ± SD) | 57.88±11.91 | 57.67±11.92 | 58.31±11.90 | 0.390 |
Position of lesion | 0.580 | |||
RUL (%) | 402 (35.3) | 271 (35.7) | 131 (34.5) | |
RML (%) | 66 (5.8) | 48 (6.3) | 18 (4.7) | |
RLL (%) | 199 (17.5) | 130 (17.1) | 69 (18.2) | |
LUL (%) | 317 (27.8) | 214 (28.2) | 103 (27.1) | |
LLL (%) | 156 (13.7) | 97 (12.8) | 59 (15.5) | |
C/T | 0.947 | |||
0 (%) | 624 (54.7) | 414 (54.5) | 210 (55.3) | |
≤50% (%) | 358 (31.4) | 239 (31.4) | 119 (31.3) | |
>50% (%) | 158 (13.9) | 107 (14.1) | 51 (13.4) | |
Surgical approach | 0.686 | |||
Lobectomy (%) | 412 (36.1) | 140 (36.8) | 272 (35.8) | |
Segmentectomy (%) | 379 (33.2) | 130 (34.2) | 249 (32.8) | |
Wedge resection (%) | 349 (30.6) | 110 (28.9) | 239 (31.4) | |
Radiologic characters | ||||
Mean CT value (Hu, mean ± SD) | –442.1±175.6 | –442.4±175.3 | –441.4±176.4 | 0.925 |
Mean CT value | 0.695 | |||
<–600 (%) | 141 (18.6) | 77 (20.3) | 218 (19.1) | |
–600 to –300 (%) | 453 (59.6) | 217 (57.1) | 670 (58.8) | |
>–300 (%) | 166 (21.8) | 86 (22.6) | 252 (22.1) | |
Multiplicity | 256 (22.5) | 166 (21.8) | 90 (23.7) | |
Size in lung window (mm, mean ± SD) | 13.5 ± 6.2 | 13.3 ± 6.0 | 14.0 ± 6.4 | 0.060 |
Solid component size (mm, mean ± SD) | 3.21±4.74 | 3.24±4.82 | 3.16±4.59 | 0.792 |
Spiculation (%) | 192 (16.8) | 127 (16.7) | 65 (17.1) | 0.867 |
Lobulation (%) | 234 (20.5) | 160 (21.1) | 74 (19.5) | 0.534 |
Vascular convergence (%) | 711 (62.4) | 235 (61.8) | 476 (62.6) | 0.795 |
Air bronchogram sign (%) | 234 (20.5) | 154 (20.3) | 80 (21.1) | 0.756 |
Bubble lucency (%) | 252 (22.1) | 162 (21.3) | 90 (23.7) | 0.364 |
Pleural indentation (%) | 242 (21.2) | 160 (21.1) | 82 (21.6) | 0.838 |
Pathology | 0.300 | |||
AAH, AIS (%) | 344 (30.2) | 237 (31.2) | 107 (28.2) | |
MIA (%) | 328 (28.8) | 223 (29.3) | 105 (27.6) | |
IA (%) | 468 (41.1) | 300 (39.5) | 168 (44.2) |
SSN, subsolid nodule; RUL, right upper lobe; RML, right middle lobe; RLL, right lower lobe; LUL, left upper lobe; LLL, left lower lobe; AAH, atypical adenomatous hyperplasia; AIS, adenocarcinoma in situ; MIA, minimally invasive adenocarcinoma; IA, invasive adenocarcinoma; C/T, consolidation to tumor ration; CT, computed tomography; Hu, Hounsfield units: SD, standard deviation.
Selection of variables for the model
The results of the univariate analysis showed that the patients with IAs were older, and were more common in males than those of NIAs. In terms of the radiological characteristics, the IAs had a larger nodule size (P<0.001), a larger solid component size (P<0.001), and a greater mean CT value (P<0.001) than NIAs (Figure 1). In addition, there were significant differences in the spiculation, lobulation, vascular convergence, air bronchogram sign, bubble lucency, and pleural indentation sign (P<0.05) between the 2 groups.
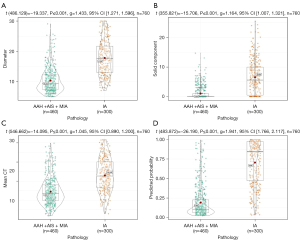
The variables mentioned above were entered into the multivariable logistic regression analysis. In the multivariate logistic regression analysis, we identified 5 independent predictors of pathological invasive SSNs, including the nodule diameter (P<0.001), solid component size (P<0.001), mean CT attenuation (P=0.001), spiculation (P<0.001), and pleura indentation (P=0.011). The results of the univariate and multivariate logistic regression analyses are shown in Table 2. On the basis of the multivariate logistic regression analysis, a nomogram model was developed with 5 independent predictors of pathological invasiveness of SSNs (Figure 2). A total score can be calculated by adding each single score of the 5 predictors, and the probability of the pathological invasiveness of SSNs can be estimated by projecting the total score to the lower total point scale.
Table 2
Characteristics | Univariate analysis | Variables selected for the model | |||||
---|---|---|---|---|---|---|---|
NIA (n=460) | IA (n=300) | P | Beta coefficient | Odds ratio (95% CI) | P | ||
Clinical characters | |||||||
Male (%) | 11 2 (24.3) | 108 (36.0) | 0.001 | ||||
Age (year, mean ± SD) | 55.61±12.25 | 60.83±10.66 | <0.001 | ||||
Position of lesion | 0.487 | ||||||
RUL (%) | 158 (34.3) | 113 (37.7) | |||||
RML (%) | 34 (7.4) | 14 (4.7) | |||||
RLL (%) | 75 (16.3) | 55 (18.3) | |||||
LUL (%) | 133 (28.9) | 81 (27.0) | |||||
LLL (%) | 60 (13.0) | 37 (12.3) | |||||
C/T | <0.001 | ||||||
0 (%) | 339 (73.7) | 75 (25.0) | |||||
≤50% (%) | 100 (21.7) | 139 (46.3) | |||||
>50% (%) | 21 (4.6) | 86 (28.7) | |||||
Radiologic characters | |||||||
Mean CT value | <0.001 | 0.001 | |||||
<–600(%) | 120 (26.1) | 21 (7.0) | Reference | ||||
–600 to –300 (%) | 297 (64.6) | 156 (52.0) | 0.726 | 1.083–3.947 | 0.028 | ||
>–300 (%) | 43 (9.3) | 123 (41.0) | 1.500 | 2.013–9.986 | <0.001 | ||
Multiplicity | 100 (21.7) | 66 (22.0) | 0.932 | ||||
Size in lung window (mm, mean ± SD) | 10.3±4.1 | 17.8±5.8 | <0.001 | 0.206 | 1.170–1.290 | <0.001 | |
Solid component size (mm, mean ± SD) | 1.08±2.19 | 6.56±5.78 | <0.001 | 0.193 | 1.124–1.310 | <0.001 | |
Spiculation (%) | 14 (3.0) | 113 (37.7) | <0.001 | 1.624 | 2.547–10.113 | <0.001 | |
Lobulation (%) | 44 (9.6) | 116 (38.7) | <0.001 | ||||
Vascular convergence (%) | 258 (56.1) | 218 (72.7) | <0.001 | ||||
Air bronchogram sign (%) | 39 (8.5) | 115 (38.3) | <0.001 | ||||
Pleural indentation (%) | 39 (8.5) | 121 (40.3) | <0.001 | 0.715 | 1.181–3.537 | 0.011 |
NIA, non-invasive adenocarcinoma; IA, invasive adenocarcinoma; CI, confidence interval; RUL, right upper lobe; RML, right middle lobe; RLL, right lower lobe; LUL, left upper lobe; LLL, left lower lobe; CT, computed tomography; C/T, consolidation to tumor ration: SD, standard deviation.
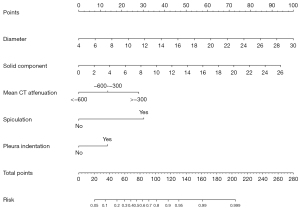
Predictive performance of the nomogram
Based on the receiver operating characteristic analysis, the nomogram showed excellent discrimination performance. The AUCs for the training and validation cohorts were 0.911±0.011 and 0.890±0.017, respectively. The calibration curves for the training and validation cohorts are shown in Figure 3, which show the robust discrimination performance of the nomogram model.
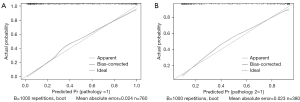
The AUCs of Hyungjin Kim’s model were 0.886±0.012 for the training cohort and 0.872±0.018 for the validation cohort. The AUCs of the “solid size >5 mm” model were 0.746±0.016 for the training cohort and 0.734±0.027 for the validation cohort. Our nomogram model had better predictive performance than both Hyungjin Kim’s model (P=0.036) and the “solid size >5 mm” model (P<0.001) for the validation cohort (Table 3).
Table 3
Model | Training cohort | Validation cohort | |||||
---|---|---|---|---|---|---|---|
AUC | 95% CI | P | AUC | 95% CI | P | ||
Proposed model | 0.911 | 0.900, 0.922 | – | 0.890 | 0.873, 0.907 | – | |
Kim’s model | 0.886 | 0.874, 0.898 | 0.001 | 0.872 | 0.854, 0.890 | 0.036 | |
Solid size >5 mm model | 0.746 | 0.730, 0.762 | <0.001 | 0.734 | 0.707, 0.761 | <0.001 |
AUC, area under the receiver operating characteristic curve; CI, confidence interval.
The sensitivity, specificity, positive predictive value (PPV), negative predictive value (NPV), and accuracy of the “solid size >5 mm” model for the validation cohort were 55.3%, 91.5%, 83.8%, 72.1%, and 75.5%, respectively. At an equally high specificity of 91.5%, the sensitivity, PPV, NPV, and accuracy of Hyungjin Kim’s model were 57.1%, 84.2%, 72.9%, and 76.3%, respectively. The sensitivity, PPV, NPV, and accuracy of the nomogram model were 67.9%, 85.7%, 76.4%, and 79.5%, respectively. The nomogram model was more sensitive than Hyungjin Kim’s model (P=0.036) and the “solid size >5 mm” model (P=0.018) at an equally high specificity of 91.5% (Table 4).
Table 4
Model | Sensitivity (%) | Specificity (%) | PPV (%) | NPV (%) | Accuracy (%) |
---|---|---|---|---|---|
Proposed model | 67.9 | 91.5 | 85.7 | 76.4 | 79.5 |
Kim’s model | 57.1 | 91.5 | 84.2 | 72.9 | 76.3 |
Solid size >5 mm model | 55.3 | 91.5 | 83.8 | 72.1 | 75.5 |
PPV, positive predictive value; NPV, negative predictive value
DCA
The decision curve is shown in Figure 4. The x axis represents the potential thresholds for the pathological invasiveness at which surgical resection should be suggested for patients with SSNs. The y axis represents the clinical NB in terms of the probability of the positive results; that is, removing invasive SSNs, minus the probability of the false-positive results. At a cut-off of 40%, the NBs were 29.1% for the nomogram model, 26.9% for Hyungjin Kim’s model, and 21.8% for the “solid size >5 mm” model. At a cut off of 80%, the NBs were 12.4% for the nomogram model, 7.3% for Hyungjin Kim’s model, and 4.1% for the “solid size >5 mm” model. Across a range of cut-off values, our proposed nomogram model had a larger NB than Kim’s model or the “solid size >5 mm” model.
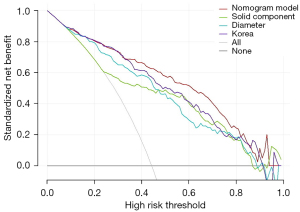
Discussion
The optimal management of patients with SSNs is of growing clinical concern. It should be noted that most persistent SSNs belong to 1 of the following 4 categories of the adenocarcinoma spectrum: AAH, AIS, MIA, and IA (16). Evaluating the pathological invasiveness of SSNs is important as clinical management strategies differ substantially for preinvasive and invasive lesions. By retrospectively analyzing the radiological characteristics of the 760 patients from the training cohort, we developed an intuitive graphic model that showed excellent discrimination performance for the validation cohort.
In the present study, we identified the diameter, solid component size, mean CT attenuation, presence of spiculation, and pleura indentation as independent predictors of the pathological invasiveness of SSNs. The diameter and solid component size were the 2 greatest contributors to the risk of IA in our study. Generally, as the lesion size and solid component increase, the possibility of pathological invasiveness increases. However, our study clearly showed overlaps between invasive and non-invasive SSNs in terms of nodule diameter and solid component size.
Solid components on CT images not only include cancer cells, but also include myofibroblastic stroma, fibrosis, inflammatory cells, alveolar collapse, and pathologic mucus (9). Discrimination solely based on nodule size or solid component size is not always feasible. Previous study has shown that morphologic CT features, including lesion margin and lesion border, can help to differentiate between preinvasive lesions and IAs (17). Our study clearly showed a better discrimination performance and more NBs than Kim’s model, which mainly uses lesion size and solid component size in combination with more CT characteristics. Our results provide evidence that a meticulous CT and a comprehensive evaluation, not only of the lesion size, but also of the morphologic features, are important in the management of SSNs.
Previous reported models (5,6) incorporated the solid ratio as an independent predictor of the pathological invasiveness of SSNs. However, the solid ratio was not adopted in the present study as a predictive variable in the univariate and multivariate analyses. One reason for this was that multicollinearity was observed when the diameter, solid component size, and solid ratio were entered into the multivariate logistic regression simultaneously. As multicollinearity increases, coefficients remain unbiased, but standard errors increase, and the likelihood of model convergence decreases. Another important reason for this was that for non-mucinous lung adenocarcinomas with a lepidic component, which manifests as SSNs on CT scans, the size of the invasive component should be used as the tumor(T) descriptors, regardless of the lepidic components in the 8th tumor, node, and metastasis (TNM) staging project of lung cancer (8). Accordingly, the long axis of the largest solid portion of the SSNs is proposed to be the T descriptors of clinical TNM stage. This highlights the importance and necessity of measuring solid components when making decisions for patients with SSNs.
An important issue in the management of SSNs is to avoid overdiagnosis and overtreatment in clinical practice. It has been estimated that 20–25% screening-detected lung cancers are overdiagnosed (18). Compared to solid nodules, SSNs have a more indolent growth rate, which shows no difference in prognosis even after years of observation (19). The treatment of SSNs should include a comprehensive evaluation of the surgical risks, patient’s psychology, life expectancy, cancer progress, and the socio-economic burden (2,6). Based on the CT features, our proposed nomogram model provides more individualized probability predictions of SSNs, which may help patients and clinicians in the treatment decision-making process. Given the high PPV (89.1%) and specificity (95.3%) at the cut-off of 80%, we believe our model could be used to help mitigate the overtreatment of SSNs. The false-negative results mainly occurred when the low-risk cut-off points were used. However, we believe a follow-up CT scan at 3–6-month intervals could safely be used to monitor the growth of SSNs.
Our study had several limitations. First, we only included patients who underwent surgical resection at our department, and those who did not undergo surgical resection were excluded, which represents a selection bias in our study. The SSNs of the patients who received CT surveillance may be smaller in size and solid component size than those who received surgical resection. We believe a substantial portion of them may be IAs, even though no changes in size or morphology after years of observation were observed. However, studies based on follow-up CT surveillance could not be carried out due to lack of histology diagnoses. The results of our study may not be applied to SSNs detected at screening tests. Second, only CT features were retrospectively analyzed in our study. We did not investigate whether clinical factors, such as a smoking history, previous malignancy, tumor biomarkers, and a positron emission tomography examination, could help differentiate between SSNs. In addition, the longitudinal assessment of SSNs using CT to assess changes in size or morphology might be more important in differential diagnosis (7). Third, our study was a single-center retrospective study with a limited number of patients. Thus, the generalizability of this model still requires external validation in other databases to confirm its diagnostic value in differentiating between SSNs.
Conclusions
Evaluating the pathological invasiveness of SSNs is important as clinical management strategies differ substantially for NIA and IA. Based on CT characteristics, including diameter, solid component size, mean CT attenuation, the presence of spiculation, and pleural indentation, we developed and internally validated a nomogram model to predict the risk of IAs for patients with SSNs. These 5 characteristics can be easily observed on CT images. Our proposed model may help clinicians to make individualized predictions for patients with SSNs and to discriminate between IAs and preinvasive lesions and MIAs.
Acknowledgments
We would like to thank our many colleagues for their support in preparing the manuscript.
Funding: This work was supported by the Shanghai Municipal Health Commission (grant No. 201840342) and the Wu JiePing Medical Foundation (grant No. 320.2730.1878).
Footnote
Reporting Checklist: The authors have completed the TRIPOD reporting checklist. Available at https://atm.amegroups.com/article/view/10.21037/atm-22-5685/rc
Data Sharing Statement: Available at https://atm.amegroups.com/article/view/10.21037/atm-22-5685/dss
Conflicts of Interest: All authors have completed the ICMJE uniform disclosure form (available at https://atm.amegroups.com/article/view/10.21037/atm-22-5685/coif). The authors have no conflicts of interest to declare.
Ethical Statement: The authors are accountable for all aspects of the work in ensuring that questions related to the accuracy or integrity of any part of the work are appropriately investigated and resolved. The study was approved by Ethics Committee of the Renji Hospital, Shanghai Jiaotong University School of Medicine (No. RA-2019-033), and all aspects of the study complied with the Declaration of Helsinki (as revised in 2013). Individual consent for this retrospective analysis was waived.
Open Access Statement: This is an Open Access article distributed in accordance with the Creative Commons Attribution-NonCommercial-NoDerivs 4.0 International License (CC BY-NC-ND 4.0), which permits the non-commercial replication and distribution of the article with the strict proviso that no changes or edits are made and the original work is properly cited (including links to both the formal publication through the relevant DOI and the license). See: https://creativecommons.org/licenses/by-nc-nd/4.0/.
References
- Travis WD, Brambilla E, Noguchi M, et al. International association for the study of lung cancer/american thoracic society/european respiratory society international multidisciplinary classification of lung adenocarcinoma. J Thorac Oncol 2011;6:244-85. [Crossref] [PubMed]
- Fang W, Xiang Y, Zhong C, et al. The IASLC/ATS/ERS classification of lung adenocarcinoma-a surgical point of view. J Thorac Dis 2014;6:S552-60. [Crossref] [PubMed]
- Tsutani Y, Miyata Y, Nakayama H, et al. Appropriate sublobar resection choice for ground glass opacity-dominant clinical stage IA lung adenocarcinoma: wedge resection or segmentectomy. Chest 2014;145:66-71. [Crossref] [PubMed]
- MacMahon H, Naidich DP, Goo JM, et al. Guidelines for Management of Incidental Pulmonary Nodules Detected on CT Images: From the Fleischner Society 2017. Radiology 2017;284:228-43. [Crossref] [PubMed]
- Jin C, Cao J, Cai Y, et al. A nomogram for predicting the risk of invasive pulmonary adenocarcinoma for patients with solitary peripheral subsolid nodules. J Thorac Cardiovasc Surg 2017;153:462-469.e1. [Crossref] [PubMed]
- Kim H, Goo JM, Park CM. A simple prediction model using size measures for discrimination of invasive adenocarcinomas among incidental pulmonary subsolid nodules considered for resection. Eur Radiol 2019;29:1674-83. [Crossref] [PubMed]
- Naidich DP, Bankier AA, MacMahon H, et al. Recommendations for the management of subsolid pulmonary nodules detected at CT: a statement from the Fleischner Society. Radiology 2013;266:304-17. [Crossref] [PubMed]
- Travis WD, Asamura H, Bankier AA, et al. The IASLC Lung Cancer Staging Project: Proposals for Coding T Categories for Subsolid Nodules and Assessment of Tumor Size in Part-Solid Tumors in the Forthcoming Eighth Edition of the TNM Classification of Lung Cancer. J Thorac Oncol 2016;11:1204-23.
- Yanagawa M, Kusumoto M, Johkoh T, et al. Radiologic-Pathologic Correlation of Solid Portions on Thin-section CT Images in Lung Adenocarcinoma: A Multicenter Study. Clin Lung Cancer 2018;19:e303-12. [Crossref] [PubMed]
- Kitami A, Sano F, Hayashi S, et al. Correlation between histological invasiveness and the computed tomography value in pure ground-glass nodules. Surg Today 2016;46:593-8. [Crossref] [PubMed]
- Baldwin DR, Callister MEGuideline Development Group. The British Thoracic Society guidelines on the investigation and management of pulmonary nodules. Thorax 2015;70:794-8. [Crossref] [PubMed]
- Zhang P, Li T, Tao X, et al. HRCT features between lepidic-predominant type and other pathological subtypes in early-stage invasive pulmonary adenocarcinoma appearing as a ground-glass nodule. BMC Cancer 2021;21:1124. [Crossref] [PubMed]
- Chu ZG, Li WJ, Fu BJ, et al. CT Characteristics for Predicting Invasiveness in Pulmonary Pure Ground-Glass Nodules. AJR Am J Roentgenol 2020;215:351-8. [Crossref] [PubMed]
- Steyerberg EW, Vergouwe Y. Towards better clinical prediction models: seven steps for development and an ABCD for validation. Eur Heart J 2014;35:1925-31. [Crossref] [PubMed]
- Allyn J, Allou N, Augustin P, et al. A Comparison of a Machine Learning Model with EuroSCORE II in Predicting Mortality after Elective Cardiac Surgery: A Decision Curve Analysis. PLoS One 2017;12:e0169772. [Crossref] [PubMed]
- Kim H, Park CM, Jeon S, et al. Validation of prediction models for risk stratification of incidentally detected pulmonary subsolid nodules: a retrospective cohort study in a Korean tertiary medical centre. BMJ Open 2018;8:e019996. [Crossref] [PubMed]
- Lee SM, Park CM, Goo JM, et al. Invasive pulmonary adenocarcinomas versus preinvasive lesions appearing as ground-glass nodules: differentiation by using CT features. Radiology 2013;268:265-73. [Crossref] [PubMed]
- Esserman LJ, Thompson IM, Reid B, et al. Addressing overdiagnosis and overtreatment in cancer: a prescription for change. Lancet Oncol 2014;15:e234-42. [Crossref] [PubMed]
- Lee JH, Park CM, Kim H, et al. Persistent part-solid nodules with solid part of 5 mm or smaller: Can the 'follow-up and surgical resection after interval growth' policy have a negative effect on patient prognosis? Eur Radiol 2017;27:195-202. [Crossref] [PubMed]
(English Language Editor: L. Huleatt)