Prognostic analysis of RAS-related lncRNAs in liver hepatocellular carcinoma
Highlight box
Key findings
• We constructed a signature with 5 RAS-related long noncoding RNAs (lncRNAs) that was highly associated with the prognosis and response to immunotherapy and targeted drugs, which provided novel insights into the personalized treatment and potential drug selection for patients with liver hepatocellular carcinoma (LIHC).
What is known and what is new?
• RAS-related pathways are involved in the cell proliferation, migration, apoptosis, and metabolism in LIHC. LncRNAs also play important roles in the progression and prognosis of LIHC. However, the clinical role, prognostic value, and immune regulation of RAS-related lncRNAs in LIHC remains unclear.
• Here, we developed a novel RAS-related lncRNA signature in LIHC.
What is the implication, and what should change now?
• Our findings provide novel insights into the clinical value of RAS-related lncRNAs in LIHC and contribute refining the precision treatment of patients with LIHC. The experimental validation in vivo and in vitro will further enhance the vigor of our study.
Introduction
Liver hepatocellular carcinoma (LIHC) remains one of the most prevalent diagnosed malignancies and the third most common cancer-related death worldwide, with about 782,000 new diagnosed cases and 746,000 deaths each year (1). The incidence of LIHC is expected to increase in the future. The initiation of LIHC involves a complex multistep process related to persistent inflammatory damage associated with fibrotic deposition, including hepatocyte necrosis and regeneration. When cirrhosis occurs, the risk of LIHC increases in tandem with progressive liver damage (2). As a malignant tumor with considerable molecular heterogeneity, LIHC is characterized by somatic genomic alterations in passenger and driver genes (3,4). The complex molecular pathogenesis of LIHC has prompted the development of molecular targeting drugs (5,6).
Three RAS genes (HRAS, NRAS, and KRAS) encode 4 proteins known as small guanosine triphosphate (GTP) binding proteins (7). RAS proteins play essential roles in the signaling transduction pathways involving cell proliferation, differentiation, and apoptosis (8,9). However, the oncogenic mutations result in the aberrant, constitutive activation of RAS function. The activated RAS then transmits signals downstream through a cytoplasmic protein cascade to regulate cell proliferation, apoptosis, migration, and metabolism in cancers (10,11).
A growing number of studies have indicated that RAS and the members involved in the signaling pathways in LIHC, such as p21, are upregulated while the inhibitors of the RAS pathway are downregulated (4,6,12). Moreover, RAS mutations such as HRAS codon 12, NRAS codon 61, and KRAS codon 12 that result in aberrant, constitutive activation of RAS function have been identified (13). Antisense RNA has been studied in the regulation of RAS pathway to explore the novel therapeutic targets in the treatment of patients with LIHC (14,15). It has already been reported that antisense HRAS treatment can significantly inhibit the hepatocarcinogenesis.
As the key regulators for gene expression, long noncoding RNAs (lncRNAs) play important roles in various disease processes and biological functions, including regulation of RNA transcription, protein translation/modification, and the RNA-protein formation or protein-protein networks (16,17). In previous studies, lncRNA-based prognostic risk signatures have been developed in many cancers, including LIHC (18,19). Xu et al. developed a risk signature with 9 ferroptosis-related lncRNAs (CTD-2116N20.1, CTD-2033A16.3, CTD-2510F5.4, LINC00942, DDX11-AS1, LINC01508, LINC01231, LINC01224, and ZFPM2-AS1) to predict the prognosis and immune response in LIHC (19). Gu et al. developed a robust 6-lncRNA signature (EIF3J-AS1, MSC-AS1, POLR2J4, RMST, SERHL, and PVT1) that was associated with recurrence-free survival for effectively predicting the recurrence risk in LIHC (20). Another prognostic risk signature developed with 4 autophagy-related lncRNAs (ZFPM2-AS1, LUCAT1, AC099850.3, and AC009005.1) identified novel autophagy-related regulatory mechanisms associated with the candidate lncRNAs (21). Protein-coding gene targets of RAS signaling have been characterized; however, the lncRNAs regulated by these processes and the clinical role of RAS-related lncRNAs remain unknown in LIHC.
In this study, we screened RAS-related lncRNAs in patients with LIHC to develop and verify a novel prognostic risk signature. In addition, we also analyzed the correlation between the risk signature with tumor mutation burden, immunotherapy responses, and sensitivity of potential drugs. The findings are expected to promote prognostic prediction and provide novel insights into the mechanism involved in the precision treatment of patients with LIHC. We present the following article in accordance with the TRIPOD reporting checklist (available at https://atm.amegroups.com/article/view/10.21037/atm-22-5827/rc).
Methods
Data collection
The transcriptome profiles and relevant clinical data from LIHC samples and healthy controls were obtained from The Cancer Genome Atlas (TCGA) database on July 28, 2022. The samples with both complete clinical information and overall survival >30 days were finally included and randomly assigned into the training dataset and the testing dataset in a 1:1 ratio. We acquired 16,876 lncRNAs according to the annotation of integrated IDs from the GENCODE website in TCGA database. The study was conducted in accordance with the Declaration of Helsinki (as revised in 2013).
Development of a prognostic RAS-related lncRNA signature
We carried out Pearson correlation analysis on these lncRNAs and 3 RAS (HRAS, NRAS, and KRAS) genes to identify the RAS-related lncRNAs with the cutoff value of |Pearson R|>0.4 and P<0.001. The prognostic value of each lncRNA was evaluated by univariate Cox regression analysis in the training dataset. We subsequently conducted least absolute shrinkage and selection operator (LASSO)-penalized regression analysis to avoid overfitting among the included lncRNAs significantly related to the prognosis. Finally, 5 lncRNAs with the coefficients were identified to develop the prognostic risk signature. And, the risk score formula according to the coefficient and the expression value of each lncRNA was then created.
In addition, we evaluated the correlation of these lncRNAs’ expression with the overall survival of patients with LIHC to explore the prognostic value of each lncRNA in the clinical data.
Validation of the prognostic RAS-related lncRNA signature
The samples were then assigned into the high-risk and the low-risk groups according to the median risk score as the cutoff value. Kaplan-Meier survival curve analysis combined with the log-rank test was carried out to compare the overall survival between the 2 risk groups. The receiver operating characteristic (ROC) curve was carried out. The area under the curve (AUC) values were applied to determine the accuracy of the risk signature in estimating the prognosis of the patients with LIHC.
Construction of a predictive nomogram for overall survival
We conducted multivariate Cox regression analysis to explore whether the risk signature was an independent prognostic factor of patients with LIHC. We then developed a predictive nomogram with the risk score and clinical variables to predict 1-, 2-, and 3-year overall survival according to the multivariate Cox regression analysis. The consistency between the predicted survival of the nomogram and the observed one was determined by time-dependent calibration curves. The AUC values of ROC curves were generated to assess the predictive accuracy of the risk signature and clinical variables in predicting survival in LIHC.
Functional enrichment analysis
The differentially expressed genes (DEGs) between the 2 risk groups were determined using the R package “limma” (The R Foundation for Statistical Computing, Vienna, Austria) according to the cutoff criteria set to |log2fold change|>1 and the false discovery rate (FDR) <0.05. Then, the DEGs were subjected to Gene Ontology (GO) enrichment analysis and Kyoto Encyclopedia of Genes and Genomes (KEGG) functional enrichment analyses to clarify the potential biological functions and key cellular pathways underlying the signature. The GO enrichment analysis focused on the following 3 terms: biological processes, cellular components, and molecular functions; meanwhile, KEGG analysis mainly aimed to study the potential biological processes and signaling pathways. Both GO and KEGG analyses were conducted using the R package “clusterProfiler”.
Tumor mutation burden and immunotherapy response related to the signature
We acquired the nucleotide variation data related to patients with LIHC in TCGA database. Then, the differences in tumor mutation burden (TMB) and somatic mutations between the low-risk group and the high-risk group were evaluated. Based on the TMB values, the patients were assigned into the low-TMB group and the high-TMB group. Kaplan-Meier survival curve analysis was subsequently conducted to assess the survival status between the 2 TMB groups and among the combination of TMB value and risk score. To evaluate the correlation of immune cell infiltration with the signature, single-sample gene set enrichment analysis (ssGSEA) was carried out to evaluate the immune function in the tumor microenvironment. In addition, we employed a tumor immune dysfunction and exclusion (TIDE) algorithm analysis to assess the immunotherapy response according to the simulation of the mechanism involved in immune escape. Thus, we could predict the immunotherapy response between the 2 risk groups according to the TIDE score.
Drug sensitivity analysis
Based on the half-maximal inhibitory concentration (IC50) of potential drugs, we evaluated the drug sensitivity of patients with different risk scores using the R package “pRRophetic”. Wilcoxon signed-rank test was used to evaluate the difference in IC50 between the 2 risk groups to facilitate the individualized treatment.
Statistical analysis
All the statistical analyses in our study were carried out on R studio in R software (version 4.1.3). Pearson correlation analysis was used to determine the RAS-related lncRNAs. Moreover, univariate and multivariate analyses were used to explore the independent prognostic factors of patients with LIHC. Kaplan-Meier survival curve analysis combined with the log-rank test was applied to assess the survival status between the groups. Time-dependent ROC and the AUC values were applied to evaluate the sensitivity and reliability of the risk signature.
Results
Identification of RAS-related lncRNAs with prognostic value in LIHC
The flowchart of this study is shown in Figure 1. The RNA transcriptome data of patients with LIHC was obtained from TCGA database, and 16,876 lncRNAs were acquired. The patients with complete survival data in the entire dataset (n=343) were randomly assigned into the training dataset (n=172) or the testing dataset (n=171) in a 1:1 ratio (Table 1), with no significant difference in terms of any clinical variables between them. We then conducted Pearson correlation analysis in the training dataset to evaluate the association of RAS (HRAS, KRAS, and NRAS) genes and the acquired lncRNAs, and 502 RAS-related lncRNAs significantly related to the prognosis were identified. Subsequently, to avoid overfitting among the lncRNAs, LASSO analysis was then used to eliminate the significantly related lncRNAs, and 5 RAS-related lncRNAs, including LINC01232, AL031985.3, ELFN1-AS1, AC093673.1, and AC244102.4 were finally identified to develop the prognostic risk signature. And, all of them were detrimental factors, with hazard ratios (HRs) >1 (Figure 2). Thus, the formula of the risk score was as follows:
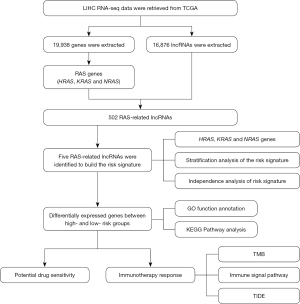
Table 1
Covariates | Type | Total, n (%) | Group, n (%) | P value | |
---|---|---|---|---|---|
Testing | Training | ||||
Age (years) | 0.8554 | ||||
≤65 | 216 (62.97) | 109 (63.74) | 107 (62.21) | ||
>65 | 127 (37.03) | 62 (36.26) | 65 (37.79) | ||
Gender | 0.0895 | ||||
Female | 110 (32.07) | 47 (27.49) | 63 (36.63) | ||
Male | 233 (67.93) | 124 (72.51) | 109 (63.37) | ||
Grade | 0.5389 | ||||
G1 | 53 (15.45) | 26 (15.2) | 27 (15.7) | ||
G2 | 161 (46.94) | 85 (49.71) | 76 (44.19) | ||
G3 | 112 (32.65) | 53 (30.99) | 59 (34.3) | ||
G4 | 12 (3.5) | 4 (2.34) | 8 (4.65) | ||
Unknown | 5 (1.46) | 3 (1.75) | 2 (1.16) | ||
Tumor stage | 0.9135 | ||||
I | 161 (46.94) | 81 (47.37) | 80 (46.51) | ||
II | 77 (22.45) | 38 (22.22) | 39 (22.67) | ||
III | 80 (23.32) | 42 (24.56) | 38 (22.09) | ||
IV | 3 (0.87) | 1 (0.58) | 2 (1.16) | ||
Unknown | 22 (6.41) | 9 (5.26) | 13 (7.56) | ||
T | 0.9097 | ||||
T1 | 168 (48.98) | 82 (47.95) | 86 (50.00) | ||
T2 | 84 (24.49) | 41 (23.98) | 43 (25.00) | ||
T3 | 75 (21.87) | 40 (23.39) | 35 (20.35) | ||
T4 | 13 (3.79) | 6 (3.51) | 7 (4.07) | ||
Unknown | 3 (0.87) | 2 (1.17) | 1 (0.58) | ||
M | 0.9785 | ||||
M0 | 320 (93.29) | 163 (95.32) | 157 (91.28) | ||
M1 | 3 (0.87) | 1 (0.58) | 2 (1.16) | ||
Unknown | 20 (5.83) | 7 (4.09) | 13 (7.56) | ||
N | 0.3922 | ||||
N0 | 307 (89.5) | 157 (91.81) | 150 (87.21) | ||
N1 | 14 (4.08) | 5 (2.92) | 9 (5.23) | ||
Unknown | 22 (6.41) | 9 (5.26) | 13 (7.56) |
LIHC, liver hepatocellular carcinoma.
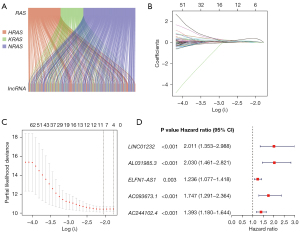
Risk score = (0.4273 × LINC01232) + (0.4079 × AL031985.3) + (0.1893 × ELFN1-AS1) + (0.2475 × AC093673.1) + (0.2373 × AC244102.4).
Evaluation and validation of the RAS-related lncRNA prognostic signature
Subsequently, the patients were assigned into the low-risk group or the high-risk group according to the cutoff value of the median risk score (Figure 3A). As shown in Figure 3B, more deaths were observed among the patients from the high-risk group than among those from the low-risk group, and there was a significantly statistical difference in the lncRNA expression from the signature between the groups (Figure 3C). As expected, the signature lncRNA expression was elevated in high-risk group. The Kaplan-Meier survival curve indicated that the high-risk patients had an unfavorable prognosis compared with the low-risk ones (Figure 3D). The AUC values of ROC in estimating the overall survival rate at 1, 2, and 3 years reached 0.77, 0.70, and 0.73, respectively (Figure 3E).
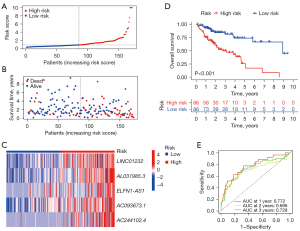
In addition, to validate the favorable accuracy of the prognostic risk signature, we employed this signature in the testing dataset and the entire dataset (Figure 4A-4C). As expected, more deaths were observed in the high-risk groups with high expression level of 5 signature lncRNAs, and the high-risk group had worse prognosis in both the testing and entire datasets (Figure 4D). AUC values in the testing dataset reached 0.76, 0.74, and 0.67, respectively, for estimating the overall survival rate at 1, 2, and 3 years. Similarly, the AUC values in the entire dataset reached 0.77, 0.72, and 0.69 for estimating the overall survival rate at 1, 2, and 3 years, respectively (Figure 4E). These results revealed that the prognostic risk signature had a favorable performance for predicting the prognosis of patients of LIHC.
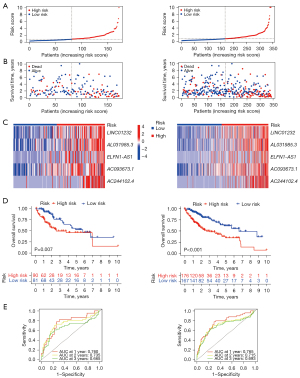
Development of a nomogram for overall survival prediction
The univariate and multivariate analyses showed only the pathological stage and risk score were independent factors for prognosis, and the risk score had a much higher HR (Figure 5A,5B). Furthermore, to assess the potential clinical use of the risk signature according to the RAS-related lncRNAs, we developed a nomogram with the risk score and traditional clinical prognostic factors, including TNM stage, age, gender, and tumor grades, to predict the survival status in patients with LIHC (Figure 5C). As shown in Figure 5D, the calibration curve of the nomogram demonstrated consistency between the predicted and actual overall survival at 1, 2, and 3 years. Moreover, the accuracy of the risk score (AUC =0.77) was superior to that of other clinical variables, such as age (AUC =0.49), gender (AUC =0.51), tumor grade (AUC =0.49), and stage (AUC =0.71), providing a higher practical value in prognostic prediction (Figure 5E).
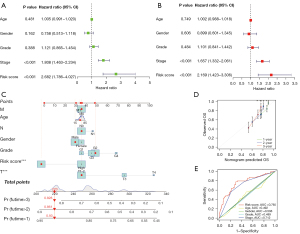
Functional enrichment analysis
To further explore the potential biological mechanisms of the signature, we conducted GO and KEGG functional enrichment analyses on the DEGs between the 2 risk groups. For biological process terms, the DEGs were mainly enriched in the nuclear division, B cell receptor signaling pathway, phagocytosis, and regulation of B cell activation. In regard to cellular component term, they were mainly concentrated in the immunoglobulin complex, chromosomal region, and DNA replication preinitiation complex. For the molecule function term, pathways, including antigen binding, immunoglobulin receptor binding, ATP-dependent activity, DNA helicase activity, CXCR chemokine receptor binding, chemokine pathway, cytokine receptor binding, were significantly associated with the DEGs between the 2 risk groups (Figure 6A). In the KEGG analysis, the DEGs were enriched in the cell cycle; cytokine-cytokine receptor interaction; human T-cell leukemia virus 1 infection; phagosome; type 1 T helper (Th1), Th2, and Th17 cell differentiation; and DNA replication (Figure 6B).
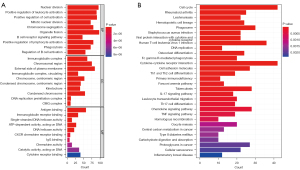
Tumor mutational burden analysis based on the RAS-related lncRNA signature
Based on the nucleotide variation data, the TMB index of the genes in each of the 2 risk groups was estimated. The waterfall plot depicts the top 15 genes with the highest mutation frequency in the mutation profiles of different risk categories. Furthermore, 132 of 172 samples (76.74%) in the high-risk group had the gene mutations (Figure 7A). Moreover, 96 of 162 samples (59.26%) in the low-risk group had the gene mutations (Figure 7B). Longitudinally, gene mutations such as TP53, KMT2D, TTN, MUC16, KDM6A, ARID1A, PIK3CA, RYR2, KMT2C, SYNE1, HMCN1, RB1, FAT4, MACF1, and FLG co-occurred between the two groups. Interestingly, mutation rate of TP53 in the high-risk group was dramatically higher than that in low-risk group (41% vs. 11%). Additionally, the high-risk patients held a significantly higher TMB index than did the low-risk patients (P=0.0026) (Figure 7C). The Kaplan-Meier curve revealed that the high TMB patients had a relatively poor prognosis compared with the low TMB ones (Figure 7D). Furthermore, we combined the TMB and risk score to assess the prognosis of patients with LIHC. The results demonstrated that the group with low risk and low TMB score had the best overall survival rate, and those with high risk and high TMB score had the worst overall survival rate (Figure 7E).
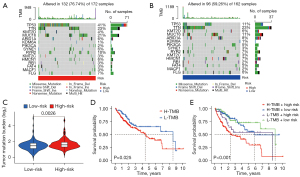
Association of immune status and escape with risk score
Furthermore, the ssGSEA was used to analyze the differences in 13 types of the immune signal pathways. The heatmap showed that the APC_co_stimulation, CCR, Check-point, and MHC_class_I immune pathways were activated, while, the type II interferon (IFN) response was suppressed in the high-risk group (Figure 8A). In addition, the evaluation of immune escape potential between the 2 risk groups was further explored according to the TIDE algorithm. The results revealed that the low-risk group had a higher TIDE level than did the high-risk group, which indicated that the high-risk patients tended to have a lower immune escape rate and better immunotherapy efficacy (Figure 8B).
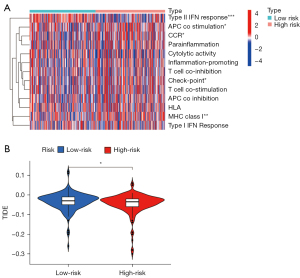
Significance of the signature in small molecular drug response evaluation
To explore the potential application of the signature in the precision treatment of patients with LIHC, we evaluated the correlation of the IC50 of potential drugs and risk scores. Patients with different levels of risk tended to respond to different antitumor drugs. A significant difference was observed in drug sensitivity between the 2 risk groups. For S-trityl-L-cysteine, paclitaxel, sunitinib, GW843682X, BI-2536, FR-180204, gemcitabine, roscovitine, and epothilone B, the IC50 for patients with high-risk scores were lower than that for patients with low-risk scores (Figure 9). The results demonstrated that the risk signature can be used to guide treatment and drug selection in patients of different risk subgroups.
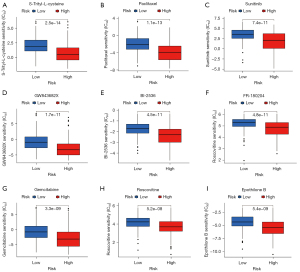
Discussion
As LIHC remains one of the most prevalent malignancies with a high morbidity and mortality worldwide, especially in China, it is of great value to identify reliable and accurate biomarkers that can estimate the outcomes of patients with LIHC.
It is evident that the lncRNAs have the capacity to regulate RAS expression in human cancers (22). As a regulator of KRAS, lncRNA MALAT1 knockdown suppresses the MEK and ERK1/2 phosphorylation induced by downregulating KRAS protein expression in cancers (23). As a commonly considered pseudogene of KRAS, lncRNA KRAS1P could potentially act as an oncogenic lncRNA to suppress the degradation of KRAS transcript in cancers (24). Therefore, it is of great value to comprehensively analyze the clinical role of the RAS-related lncRNAs to explore novel lncRNA-based strategies for diagnosis and targeted therapies in cancers. In our study, we first conducted a comprehensive analysis on the RAS-related lncRNAs in LIHC, and developed a novel 5 RAS-related lncRNA signature with encouraging sensitivity and specificity to predict the prognosis of patients with LIHC (Figures 3D,4D). We found that the patients with high-risk scores calculated by the signature were related to worse survival outcomes. All of these 5 RAS-related lncRNAs including LINC01232, AL031985.3, ELFN1-AS1, AC093673.1, and AC244102.4 were over-expressed in the high-risk cohort (Figures 3C,4C), which were consistent with the result of Figure 2D, suggesting they appear to be detrimental factors, with HRs >1. Furthermore, the Kaplan-Meier analysis showed that the samples with higher expression of LINC01232, AL031985.3, ELFN1-AS1, AC093673.1, and AC244102.4 had an unfavorable prognosis (Figure S1). The above results suggest that these 5 lncRNAs could be the candidate targets for the treatment of LIHC.
The biological functions of these candidate lncRNAs in cancers had been investigated in the previous studies. Results of this research suggests that LINC01232 plays important roles in the alternative splicing of A-Raf by suppressing HNRNPA2B1 degradation and by regulating the MAPK/ERK signaling pathway to promote pancreatic cancer metastasis, thus providing a potential therapeutic target (25). LINC01232 was also found to maintain carcinogenic properties in tumor progression via regulation of TM9SF2 in pancreatic adenocarcinoma (26). Additionally, the increased expression of LINC01232 was shown to sponge miR-204-5p and upregulate RAB22A to promote disease progression in clear cell renal cell carcinoma (27). Other research indicates that LINC01232 sequesters microRNA (miRNA)-654-3p and consequently promotes hepatoma-derived growth factor expression to contribute to the tumor progression in esophageal squamous cell carcinoma (28). Moreover, LINC01232 has been proven to promote cell proliferation, migration, and invasion by modulating the miR-370-5p–PIM3 axis in bladder cancer (29). Interestingly, AL031985.3 has been included in several lncRNA-based signatures related to ferroptosis, pyroptosis, immune, and autophagy in predicting the outcome, immune cell infiltration, and response to immunotherapy in patients with LIHC (30-33). The results demonstrated that AL031985.3 is significantly associated with the progression of LIHC. However, the related biological functions remain to be further clarified. MEIS1 downregulation was shown to promote tumorigenesis and oxaliplatin resistance through the ELFN1-AS1-EZH2-DNMT3a axis in colorectal cancer (34). ELFN1-AS1 can accelerate cell proliferation, migration, and invasion through regulating the miR-497-3p-CLDN4 axis in ovarian cancer (35). Furthermore, ELFN1-AS1 can promote cell proliferation and migration via the miR-191-5p–special AT-rich sequence-binding protein 1 axis in colon cancer (36). The exosome ELFN1-AS1 was shown to mediate M2 macrophage polarization to facilitate tumorigenesis in osteosarcoma (37). However, the biological functions of lncRNA AC093673.1 and AC244102.4 remain unknown, and it is hoped that our results can illuminate their functions and mechanisms.
GO and KEGG analyses showed that the nuclear division and DNA replication were significantly enriched and that active nuclear division and DNA replication were predisposed to gene mutation (Figure 6). Therefore, we next calculated the TMB for each patient. The results showed that TMB indices were 76.74% and 59.26% in the high-risk group and the low-risk group, respectively. Consistent with the previous studies, our results showed that higher TMB tends to predict worse survival. In addition, the group with high risk and high TMB score had the worst overall survival rate (Figure 7).
Mutations are processed into the neoantigens and are presented to the T cells by the major histocompatibility complexes (MHCs). Cancer cells use immune checkpoints to inhibit T cell reactivity and evade immune eradication (38). Immune checkpoint inhibitors (ICIs) enable T cell reactivation and have revolutionized cancer treatment. However, as most cancer patients do not benefit from the ICIs, identifying accurate and reliable biomarkers that can estimate the response to ICIs are required. Higher TMB could lead to more neoantigens, which increases the chance for T cell recognition and clinical results in better ICI responses (39). Consistent with this conclusion, we found that in LIHC, patients with high-TMB had higher levels of CD8 T cells and follicular helper T cells, which can form T cell immunogenicity (Figure S2). However, TMB is not a perfect biomarker for estimating the ICI response. The composite predictors consisting of critical variables including the T-cell receptor repertoire and MHC are needed (40). We further analyzed the differences in 13 types of immune signal pathways, the results of which showed MHC_class_I, APC_co_stimulation, Check-point, and CCR immune pathways were activated in the high-risk group. Taken together, these results suggest that the high-risk group had better immune activity. In addition, the high-risk group had the lower TIDE level, which indicated that the high-risk patients tended to have a lower immune escape rate and better immunotherapy efficacy (Figure 8). In the past few years, ICIs have revolutionized the management of LIHC (41), but despite these major advances in the immunotherapy of patients LIHC, the underlying molecular biological mechanisms governing immune evasion and responses remain unclear. Thus conducted this study to garner insights into the immune status and genomic signature underlying immunotherapy response or resistance in patients with LIHC.
In the course of disease, about 50% of patients with LIHC receive systemic therapy, including lenvatinib or sorafenib as the first-line treatment and cabozantinib, ramucirumab, or regorafenib as the second-line treatment. However, the problem of drug resistance and relapse is significant, and thus accurate and reliable biomarkers need to be developed to guide the treatment and drug selection for patients across different risk subgroups. Drug sensitivity results demonstrated that the group with high risk was more sensitive to S-trityl-L-cysteine, paclitaxel, sunitinib, GW843682X, BI-2536, FR-180204, gemcitabine, roscovitine, and epothilone B (Figure 9).
However, several limitations exist in this study. We only used integrative bioinformatic analysis based on the data from a public database, and in vitro or in vivo experimental validation of our findings is currently lacking. The accuracy of the prognostic risk signature in estimating the prognosis and immune landscape of patients with LIHC in the clinic still needs further validation in clinical trials. In addition, there are some problems and challenges that need to be overcome in the clinical application of lncRNA, such as the complex regulatory network of lncRNA has not been fully understood and the stability of RNA extracted from serum or plasma tend to be low.
Conclusions
We constructed an accurate and reliable prognostic risk signature with 5 RAS-related lncRNAs for predicting the prognosis and responses to immunotherapy of patients with LIHC. We also evaluated the correlation of the risk signature and specific immune cell populations based on the immune analysis. Thus, our findings offer novel insights into the clinical value of RAS-related lncRNAs in LIHC and contribute refining the precision treatment of patients with LIHC.
Acknowledgments
The authors gratefully acknowledge the contributors to The Cancer Genome Atlas (TCGA) database.
Funding: None.
Footnote
Reporting Checklist: The authors have completed the TRIPOD reporting checklist. Available at https://atm.amegroups.com/article/view/10.21037/atm-22-5827/rc
Conflicts of Interest: All authors have completed the ICMJE uniform disclosure form (available at https://atm.amegroups.com/article/view/10.21037/atm-22-5827/coif). The authors have no conflicts of interest to declare.
Ethical Statement: The authors are accountable for all aspects of the work in ensuring that questions related to the accuracy or integrity of any part of the work are appropriately investigated and resolved. The study was conducted in accordance with the Declaration of Helsinki (as revised in 2013).
Open Access Statement: This is an Open Access article distributed in accordance with the Creative Commons Attribution-NonCommercial-NoDerivs 4.0 International License (CC BY-NC-ND 4.0), which permits the non-commercial replication and distribution of the article with the strict proviso that no changes or edits are made and the original work is properly cited (including links to both the formal publication through the relevant DOI and the license). See: https://creativecommons.org/licenses/by-nc-nd/4.0/.
References
- Forner A, Reig M, Bruix J. Hepatocellular carcinoma. Lancet 2018;391:1301-14. [Crossref] [PubMed]
- Villanueva A. Hepatocellular Carcinoma. N Engl J Med 2019;380:1450-62. [Crossref] [PubMed]
- Xu LX, He MH, Dai ZH, et al. Genomic and transcriptional heterogeneity of multifocal hepatocellular carcinoma. Ann Oncol 2019;30:990-7. [Crossref] [PubMed]
- Teufel A, Staib F, Kanzler S, et al. Genetics of hepatocellular carcinoma. World J Gastroenterol 2007;13:2271-82. [Crossref] [PubMed]
- Dhanasekaran R, Nault JC, Roberts LR, et al. Genomic Medicine and Implications for Hepatocellular Carcinoma Prevention and Therapy. Gastroenterology 2019;156:492-509. [Crossref] [PubMed]
- Rebouissou S, Nault JC. Advances in molecular classification and precision oncology in hepatocellular carcinoma. J Hepatol 2020;72:215-29. [Crossref] [PubMed]
- Yan C, Theodorescu D. RAL GTPases: Biology and Potential as Therapeutic Targets in Cancer. Pharmacol Rev 2018;70:1-11. [Crossref] [PubMed]
- Li S, Balmain A, Counter CM. A model for RAS mutation patterns in cancers: finding the sweet spot. Nat Rev Cancer 2018;18:767-77. [Crossref] [PubMed]
- Moore AR, Rosenberg SC, McCormick F, et al. RAS-targeted therapies: is the undruggable drugged? Nat Rev Drug Discov 2020;19:533-52. [Crossref] [PubMed]
- Murugan AK, Grieco M, Tsuchida N. RAS mutations in human cancers: Roles in precision medicine. Semin Cancer Biol 2019;59:23-35. [Crossref] [PubMed]
- Chen K, Zhang Y, Qian L, et al. Emerging strategies to target RAS signaling in human cancer therapy. J Hematol Oncol 2021;14:116. [Crossref] [PubMed]
- Moon H, Ro SW. MAPK/ERK Signaling Pathway in Hepatocellular Carcinoma. Cancers (Basel) 2021;13:3026. [Crossref] [PubMed]
- Muñoz-Maldonado C, Zimmer Y, Medová M. A Comparative Analysis of Individual RAS Mutations in Cancer Biology. Front Oncol 2019;9:1088. [Crossref] [PubMed]
- Wu B, Yuan Y, Liu J, et al. Single-cell RNA sequencing reveals the mechanism of sonodynamic therapy combined with a RAS inhibitor in the setting of hepatocellular carcinoma. J Nanobiotechnology 2021;19:177. [Crossref] [PubMed]
- Akula SM, Abrams SL, Steelman LS, et al. RAS/RAF/MEK/ERK, PI3K/PTEN/AKT/mTORC1 and TP53 pathways and regulatory miRs as therapeutic targets in hepatocellular carcinoma. Expert Opin Ther Targets 2019;23:915-29. [Crossref] [PubMed]
- Tan YT, Lin JF, Li T, et al. LncRNA-mediated posttranslational modifications and reprogramming of energy metabolism in cancer. Cancer Commun (Lond) 2021;41:109-20. [Crossref] [PubMed]
- Peng WX, Koirala P, Mo YY. LncRNA-mediated regulation of cell signaling in cancer. Oncogene 2017;36:5661-7. [Crossref] [PubMed]
- Li D, Liang J, Cheng C, et al. Identification of m6A-Related lncRNAs Associated With Prognoses and Immune Responses in Acute Myeloid Leukemia. Front Cell Dev Biol 2021;9:770451. [Crossref] [PubMed]
- Xu Z, Peng B, Liang Q, et al. Construction of a Ferroptosis-Related Nine-lncRNA Signature for Predicting Prognosis and Immune Response in Hepatocellular Carcinoma. Front Immunol 2021;12:719175. [Crossref] [PubMed]
- Gu JX, Zhang X, Miao RC, et al. Six-long non-coding RNA signature predicts recurrence-free survival in hepatocellular carcinoma. World J Gastroenterol 2019;25:220-32. [Crossref] [PubMed]
- Wu H, Liu T, Qi J, et al. Four Autophagy-Related lncRNAs Predict the Prognosis of HCC through Coexpression and ceRNA Mechanism. Biomed Res Int 2020;2020:3801748. [Crossref] [PubMed]
- Saliani M, Mirzaiebadizi A, Javadmanesh A, et al. KRAS-related long noncoding RNAs in human cancers. Cancer Gene Ther 2022;29:418-27. [Crossref] [PubMed]
- Liu P, Yang H, Zhang J, et al. The lncRNA MALAT1 acts as a competing endogenous RNA to regulate KRAS expression by sponging miR-217 in pancreatic ductal adenocarcinoma. Sci Rep 2017;7:5186. [Crossref] [PubMed]
- Poliseno L, Salmena L, Zhang J, et al. A coding-independent function of gene and pseudogene mRNAs regulates tumour biology. Nature 2010;465:1033-8. [Crossref] [PubMed]
- Meng LD, Shi GD, Ge WL, et al. Linc01232 promotes the metastasis of pancreatic cancer by suppressing the ubiquitin-mediated degradation of HNRNPA2B1 and activating the A-Raf-induced MAPK/ERK signaling pathway. Cancer Lett 2020;494:107-20. [Crossref] [PubMed]
- Li Q, Lei C, Lu C, et al. LINC01232 exerts oncogenic activities in pancreatic adenocarcinoma via regulation of TM9SF2. Cell Death Dis 2019;10:698. [Crossref] [PubMed]
- Liu Q, Lei C. LINC01232 serves as a novel biomarker and promotes tumour progression by sponging miR-204-5p and upregulating RAB22A in clear cell renal cell carcinoma. Ann Med 2021;53:2153-64. [Crossref] [PubMed]
- Zhao M, Cui H, Zhao B, et al. Long intergenic non coding RNA LINC01232 contributes to esophageal squamous cell carcinoma progression by sequestering microRNA 654 3p and consequently promoting hepatoma derived growth factor expression. Int J Mol Med 2020;46:2007-18. [Crossref] [PubMed]
- Feng F, Yang J, Chen A, et al. Long non-coding RNA long intergenic non-protein coding RNA 1232 promotes cell proliferation, migration and invasion in bladder cancer via modulating miR-370-5p/PIM3 axis. J Tissue Eng Regen Med 2022;16:575-85. [Crossref] [PubMed]
- Chen ZA, Tian H, Yao DM, et al. Identification of a Ferroptosis-Related Signature Model Including mRNAs and lncRNAs for Predicting Prognosis and Immune Activity in Hepatocellular Carcinoma. Front Oncol 2021;11:738477. [Crossref] [PubMed]
- Wu ZH, Li ZW, Yang DL, et al. Development and Validation of a Pyroptosis-Related Long Non-coding RNA Signature for Hepatocellular Carcinoma. Front Cell Dev Biol 2021;9:713925. [Crossref] [PubMed]
- Zhou P, Lu Y, Zhang Y, et al. Construction of an Immune-Related Six-lncRNA Signature to Predict the Outcomes, Immune Cell Infiltration, and Immunotherapy Response in Patients With Hepatocellular Carcinoma. Front Oncol 2021;11:661758. [Crossref] [PubMed]
- Jia Y, Chen Y, Liu J. Prognosis-Predictive Signature and Nomogram Based on Autophagy-Related Long Non-coding RNAs for Hepatocellular Carcinoma. Front Genet 2020;11:608668. [Crossref] [PubMed]
- Li Y, Gan Y, Liu J, et al. Downregulation of MEIS1 mediated by ELFN1-AS1/EZH2/DNMT3a axis promotes tumorigenesis and oxaliplatin resistance in colorectal cancer. Signal Transduct Target Ther 2022;7:87. [Crossref] [PubMed]
- Jie Y, Ye L, Chen H, et al. ELFN1-AS1 accelerates cell proliferation, invasion and migration via regulating miR-497-3p/CLDN4 axis in ovarian cancer. Bioengineered 2020;11:872-82. [Crossref] [PubMed]
- Du Y, Hou Y, Shi Y, et al. Long Non-Coding RNA ELFN1-AS1 Promoted Colon Cancer Cell Growth and Migration via the miR-191-5p/Special AT-Rich Sequence-Binding Protein 1 Axis. Front Oncol 2020;10:588360. [Crossref] [PubMed]
- Wang B, Wang X, Li P, et al. Osteosarcoma Cell-Derived Exosomal ELFN1-AS1 Mediates Macrophage M2 Polarization via Sponging miR-138-5p and miR-1291 to Promote the Tumorgenesis of Osteosarcoma. Front Oncol 2022;12:881022. [Crossref] [PubMed]
- Chalmers ZR, Connelly CF, Fabrizio D, et al. Analysis of 100,000 human cancer genomes reveals the landscape of tumor mutational burden. Genome Med 2017;9:34. [Crossref] [PubMed]
- Büttner R, Longshore JW, López-Ríos F, et al. Implementing TMB measurement in clinical practice: considerations on assay requirements. ESMO Open 2019;4:e000442. [Crossref] [PubMed]
- Jardim DL, Goodman A, de Melo Gagliato D, et al. The Challenges of Tumor Mutational Burden as an Immunotherapy Biomarker. Cancer Cell 2021;39:154-73. [Crossref] [PubMed]
- Llovet JM, Castet F, Heikenwalder M, et al. Immunotherapies for hepatocellular carcinoma. Nat Rev Clin Oncol 2022;19:151-72. [Crossref] [PubMed]
(English Language Editor: J. Gray)