A pan-cancer analysis of the expression and prognostic significance of PDRG1
Highlight box
Key findings
• PDRG1 as a pan-cancer biomarker.
What is known and what is new?
• PDRG1 involved in various physiological regulations of cells.
• PDRG1 may be an important pan-cancer molecular biomarker for diagnosis and prognosis.
What is the implication, and what should change now?
• PDRG1 may application in cancer diagnosis, treatment, and prognosis,and have been preliminarily validated in HCC.
Introduction
According to global cancer statistics, there were approximately 19 million new cancer cases in the world in 2020 and nearly 10 million deaths. Female breast cancer surpassed lung cancer as the most commonly diagnosed cancer, accounting for 11.7% of all new tumor cases, followed by lung cancer, colorectal cancer, and prostate cancer (1). Lung cancer remained the leading cause of death, followed by colorectal cancer, liver cancer, gastric cancer, and female breast cancer. The incidence of cancer in developed countries was higher than that in developing countries, but the mortality rate of breast cancer and cervical cancer in developing countries was significantly higher than that in developed countries. In 2020, China accounted for 24% of the world’s new cancer cases and 30% of global deaths, and total cancer cases worldwide are expected to reach 28.4 million by 2040, an increase of 47% over 2020 (2). Because of population changes, developing countries will have more cancer patients in the future than developed countries. Therefore, early detection and treatment are essential to reduce the burden of cancer.
Tumor markers are a class of molecules produced by tumor tissues or their interaction with the host, which are mostly present in tissues and body fluids, and have important clinical significance for the early diagnosis, treatment, and prognosis of cancer (3). Carcinoembryonic antigen, carbohydrate antigen, oncogene, and tumor suppressor genes are commonly used tumor markers. With the development of research and clinical practice, circulating tumor cells and microRNAs (miRNAs) have been introduced clinically as tumor markers (4). Genome-level biomarkers, such as KRAS mutations and epidermal growth factor receptors, have been used to guide targeted therapies for bowel cancer and non-small cell lung cancer (5,6). In recent years, although immunotherapy and targeted therapy has been successful to a certain extent, the prognosis of patients is still unsatisfactory. Therefore, identifying new tumor markers is necessary to promote early diagnosis and prognostic assessment of tumors, thereby improving the overall survival (OS) rate of patients.
PDRG1, located on the long arm of chromosome 20, encode 133 amino acids in different subcellular compartments in the cytoplasm and are closely related to various cellular physiological activities. These include cell proliferation, growth, apoptosis, cell cycle regulation, and DNA damage repair. In addition, PDRG1 is part of the RNA polymerase II complex, however, its role in tumor remains unclear (7,8). Previous studies have shown that PDRG1 is highly expressed in colorectal and gastric cancer tissues, and silencing PDRG1 expression in cancer cells can affect carcinogenesis growth (9). In addition, PDRG1 may affect the growth of lung cancer cells and their sensitivity to radiotherapy by mediating the ATM-p53 signaling pathway (10). These studies suggest that PDRG1 may be a potential oncogenic molecule. Currently, studies on PDRG1 in tumors are mostly limited to specific types of tumors, and there is no overall pan-cancer evaluation of the significance of PDRG1, and without exploration of the related immune microenvironment and involved pathways. Therefore, we explored the significance of PDRG1 in various malignancies by several different methods. We present the following article in accordance with the REMARK reporting checklist (available at https://atm.amegroups.com/article/view/10.21037/atm-22-5439/rc).
Methods
Differential expression of PDRG1 in tumors
We used the “DiffExp” module in the TIMER2 (http://timer.cistrome.org/) online tool to mine PDRG1 expressions in tumor tissues from the TCGA database.
Role of PDRG1 in tumor prognosis
We downloaded a unified and standardized pan-cancer dataset: The University of California Santa Cruz (UCSC) TCGA Pan-Cancer database (https://xenabrowser.net/), from which we further extracted the expression data of the PDRG1 gene in each sample. We screened the sample sources as follows: Secondary Blood-Derived Cancer-Peripheral Blood (TCGA-LAML). A high-quality prognostic TCGA dataset (TCGA-SKCM metastatic samples) was obtained. Samples with follow-up periods of less than 30 days were excluded. A log2 (x+1) transformation was applied to each expression value, and cancer species with less than 10 samples in a single cancer species were excluded. Finally, the expression data and corresponding samples’ OS, disease-specific survival (DSS), disease-free survival (DFS), progression-free survival (PFS), and prognostic analyses were analyzed for 39 cancer species (TCGA-GBM, TCGA-GBMLGG, TCGA-LGG, TCGA-CESC, TCGA-LUAD, TCGA-LAML, TCGA-BRCA, TCGA-ESCA, TCGA-STES, TCGA-SARC, TCGA-KIRP, TCGA-KIPAN, TCGA-PRAD, TCGA-STAD, TCGA-HNSC, TCGA-KIRC, TCGA-COAD, TCGA-COADREAD, TCGA-LUSC, TCGA-THYm, TCGA-LIHC, TCGA-THCA, TCGA-MESO, TCGA-read, TCGA-SKCM-M, TCGA-SKCM, TCGA-OV, TCGA-TGCT, TCGA-PAAD, TCGA-UCEC, TCGA-PCPG, TCGA-SKCM-P, TCGA-UVM, TCGA-UCS, TCGA -BLCA, TCGA-ACC, TCGA-KICH, TCGA-CHOL, and TCGA-DLBC).
Immune correlation analysis
To evaluate the role of PDRG1 in tumor immune infiltration, we used the R software package IOBR and the CIBERSORT, EPIC, IPS, mcpcounter, quantiseq, TIMER, and xCELL methods (11-18). Immune cell infiltration was reassessed for each patient in each tumor based on gene expression. We further extracted the expression data of 150 marker genes in five types of immune pathways [chemokine, receptor, major histocompatibility complex (MHC), immunoinhibitor, and immunostimulator] in each sample. The expression data of 60 stimulatory and 21 m6A methylation genes and their correlations with PDRG1 were analyzed, and the results were visualized with a heat map.
Genomic heterogeneity and gene expression analysis
From GDC (https://portal.gdc.cancer.gov/), we downloaded the Simple Nucleotide at level 4 of all TCGA samples using MuTect2 software (19). The tumor mutation burden (TMB) function of the R software package “maftools” (version 2.8.05) was used to calculate the TMB of each tumor, and then the TMB and gene expression data of the samples were integrated. The MSI (microsatellite instability) score (20) for each tumor was obtained from previous studies, and a log2 (x+1) transformation was performed for each expression value. We excluded cancer species with less than three samples in a single cancer species and finally obtained expression data for 37 cancer species. Pearson correlations were calculated for each tumor, and the results were visualized with heat maps.
Protein interaction network construction and functional enrichment analysis
The STRING (https://string-db.org/) database was used to screen the proteins interacting with PDRG1. In this study, “PDRG1” was entered, the species was selected as “human”, and the confidence was selected as “medium confidence (0.150)”. The GeneMANIA (http://genemania.org/) database was used for repeated screening to select proteins interacting with PDRG1. Genes obtained were included in Gene Ontology (GO) and Kyoto Encyclopedia of Genes and Genomes (KEGG) analyses. We used the “clusterProfiler” program in R language to analyze the results of the enrichment analysis.
PDRG1 and HCC
We used R software (version 3.6.4) to calculate the expression differences of HCC genes in different clinical stages and grade samples to evaluate the relationship between PDRG1 and clinical information in HCC.
Collection and preservation of data
Written informed consent was obtained from the patient and/or family members before surgery. The study was conducted in accordance with the Declaration of Helsinki (as revised in 2013) and was approved by the Ethics Committee of Liuzhou People’s Hospital (No. KY2022-065-01). Forty HCC samples and adjacent tissues were collected in Liuzhou People’s Hospital. The tissues were immersed in liquid nitrogen within 15 minutes of resection and then transferred to –80 ℃ refrigeration storage until protein extraction.
Western blot
After 20 mg of tissue sample was ground into powder with liquid nitrogen, pre-cooled lysate was added according to the net weight of the tissue, fully lysed, and centrifuged for supernatant. The protein concentration was detected by the BCA Kit. Subsequently, a 10 ug protein sample was loaded and transferred to a polyvinylidene fluoride membrane after SDS-PAGE. PDRG1 (Proteintech Group, 161668-1-AP) and GAPDH (Solarbio, K000026M) were incubated overnight at 4 ℃. After membrane washing, Invitrogen goat anti-rabbit IgG (H+L) secondary antibody HRP (ThermoFisher Scientific, 31460) was added and incubated for 1 h at room temperature. ImageJ software was used to process the images.
Statistical analysis
The expression of PDRG1 was analyzed by t-test, the survival analysis was performed by the Kaplan-Meier method, and the Pearson test was used for the correlation analysis. A P value <0.05 was considered statistically significant. The data were integrated and analyzed using R software (Version 3.6.4; https://www.R-project.org). The differences between paired groups were analyzed using the unpaired Student’s t-test, and the differences between multiple groups were tested using an analysis of variance (ANOVA).
Results
Expression of PDRG1 in tumors
We first analyzed the expression differences of PDRG1 in 33 types of tissues and adjacent tissues in the TCGA database. The results showed that PDRG1 was upregulated, downregulated, or unchanged in different cancer types (Figure 1). PDRG1 was significantly overexpressed in bladder urothelial carcinoma (BLCA), breast invasive carcinoma (BRCA), cholangiocarcinoma (CHOL), colon adenocarcinoma (COAD), esophageal carcinoma (ESCA), head and neck squamous cell carcinoma (HNSC), HNSC−HPVpos, kidney chromophobe (KICH), kidney renal clear cell carcinoma (KIRC), kidney renal papillary cell carcinoma (KIRP), lung adenocarcinoma (LUAD), lung squamous cell carcinoma (LUSC), rectum adenocarcinoma (READ), stomach adenocarcinoma (STAD), and uterine corpus endometrial carcinoma (UCEC). It was significantly underexpressed in KIRC and thyroid carcinoma (THCA). This suggests that there are intrinsic differences in PDRG1 expression among different tumor types.
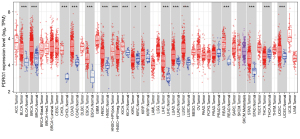
Correlation between PDRG1 expression and tumor prognosis
To explore the correlation between PDRG1 expression and patient outcomes in a pan-cancer analysis, we used gene expression profiling data and univariate analysis with forest mapping. The analysis of DFS showed that PDRG1 was significantly associated with prognosis in prostate adenocarcinoma (PRAD), COAD, colorectal adenocarcinoma (COADREAD), LIHC, testicular germ cell tumors (TGCT), diffuse large B-cell lymphoma (DLBC), and mesothelioma (MESO). The analysis of DSS showed that PDRG1 was significantly associated with prognosis in glioblastoma and low-grade glioma (GBMLGG), lower grade glioma (LGG), LIHC, PRAD, MESO, pheochromocytoma and paraganglioma (PCPG), and uveal melanoma (UVM). PDRG1 was significantly associated with OS prognosis in GBMLGG, LGG, LIHC, MESO, PRAD, acute myeloid leukemia (LAML), and UVM. The PFS analysis showed that PDRG1 was significantly associated with prognosis in GBMLGG, PRAD, LGG, DLBC, and LIHC (Figure 2). These results indicated that the expression of PDRG1 was associated with the survival rates of PRAD, MESO, GBMLGG, LGG, LIHC, and UVM, suggesting that PDRG1 may be a potential pan-cancer prognostic biomarker.
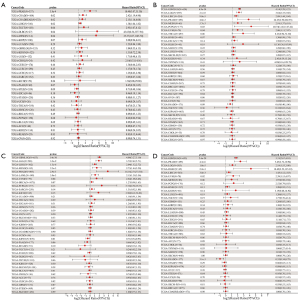
Expression of PDRG1 and analysis of the tumor microenvironment
In our study, various assays were used to assess the association between abnormal PDRG1 expression and immune cell infiltration across cancer types. The results showed that PDRG1 was positively correlated with memory B cells, regulatory T cells, and resting mast cells in various tumors. In addition, PDRG1 was positively correlated with B cells, CD4 T cells, neutrophils, macrophages, and dendritic cells (DC) in LIHC. Subsequently, we explored the correlation between PDRG1 and five types of immune pathways, and the results showed that MHC was significantly positively correlated with GBM, LGG, and UVM and negatively correlated with kidney renal papillary cell carcinoma (KIRP), THCA, and KICH. Effector cells (EC) was significantly positively correlated with UVM, and immunsuppressive cells (SC) and immune checkpoints (CP) were significantly positively correlated with KIPAN and THCA (Figure 3). In addition, we also found significant positive and negative correlations between PDRG1 and m6A methylation and CP in various tumors (Figure 4).
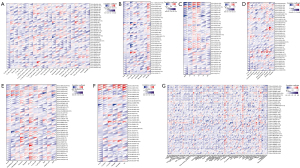
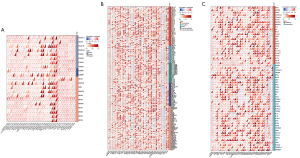
Correlation between PDRG1 expression and tumor TMB and MSI
We also explored the correlation between PDRG1 and the TMB and MSI of tumors. The results showed that PDRG1 was significantly correlated with TMB in LIHC, KICH, CHOL, uterine carcinosarcoma (UCS), and 33 other common tumors. PDRG1 was significantly correlated with MSI in COAD, READ, LIHC, and KIPAN (Figure 5).
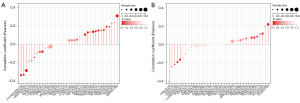
Protein interaction network construction and functional enrichment analysis
We used GeneMANIA and STRING to further analyze the related molecules and mechanisms of PDRG1. The results showed that PDRG1 interacted with URI1, PFDN2, PFDN4, and UXT, mainly in protein folding, protein phosphatase type 1 complex, and chaperone binding. It may play a role via messenger RNA (mRNA) surveillance, insulin resistance, insulin signaling, and other pathways (Figure 6).
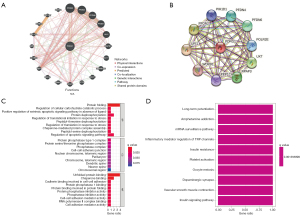
PDRG1 and HCC
We further evaluated the expression of PDRG1 in different clinical stages of HCC and found that PDRG1 expression was different among different clinical stages of HCC (Figure 7). Moreover, the western blot results of the clinical samples confirmed that PDRG1 was significantly overexpressed in HCC tissues (Figure 8).
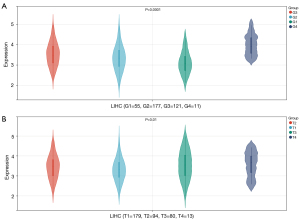
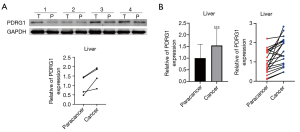
Discussion
By analyzing gene expression, prognosis, mutation, and function in different tumors using multiple databases, a pan-cancer analysis can reveal similarities and differences between different tumors, providing a new perspective for developing targeted drugs, tumor prevention, and personalized treatment (21). An increasing number of studies have focused on genome-wide pan-cancer analyses to mine mutated genes and tumor driver genes associated with tumorigenesis and progression, which is important for early diagnosis of tumors and identification of biomarkers (22,23). To have a more systematic and comprehensive understanding of PDRG1, we explored the expression pattern of PDRG1 using a pan-cancer analysis. We also focused on PDRG1’s diagnostic and prognostic value in HCC and explored whether it is involved in different types of tumorigenesis and development.
Although current studies on the expression and function of PDRG1 indicate that it is an oncogene, it may play different roles in different types of cancer. Studies have shown that PDRG1 is highly expressed in colorectal cancer tissues, and the expression level is positively correlated with clinical stage and prognosis. The higher the expression level, the worse the prognosis, and PDRG1 has also been identified as an independent prognostic factor for CRC (24). PDRG1 is significantly increased in gastric cancer tissues and is associated with lower pathological differentiation, more severe lymph node metastasis, and a higher tumor stage (25). In addition, PDRG1 is overexpressed in glioblastoma multiforme and esophageal cancer and is associated with poor prognosis (26,27). Although these findings are consistent with our results, many tumors remain unexamined. The expression of PDRG1 is high in most tumors but low in some tumor tissues, suggesting that PDRG1 may have opposite effects in different tumors or even in different subtypes of the same tumor. This difference in human tumors may be due to the complexity and heterogeneity of cancer.
We predicted the related genes and constructed signaling pathways based on PDRG1 and found that PDRG1 may play a role via the mRNA surveillance, insulin resistance, and insulin signaling pathways. DNA loss repair pathways play an important role in maintaining genome integrity, and cellular stress can damage RNA to produce faulty and/or nonfunctional proteins (28,29). Three mRNA monitoring pathways—non-decaying (NGD), uninterrupted decay (NSD), and nonsense-mediated mRNA decay (NMD)—play important roles in mRNA translation (30). Insulin resistance is caused by a decreased response of peripheral target tissues to the action of insulin and is the beginning of the insidious process of type 2 diabetes mellitus (31,32). One of the most prominent features of tumor metabolism is abnormal glucose metabolism. The relationship between insulin resistance and cancer is complex and has not been comprehensively studied. Current studies have shown that insulin receptor (INSR) is highly expressed in human malignant tumors, and INSR can activate a variety of signaling pathways and play a role in the occurrence and development of tumors (33,34). Insulin binds to insulin receptor tyrosine kinase (IRTK) and functions through the PI3K/AKT signaling pathway, which is closely related to human colorectal, breast, and prostate cancers and can directly affect various biological manifestations of tumor cells (35-37).
B cells are the key immune cells in the adaptive anti-tumor immune response. After activation, B cells differentiate into plasma cells, which can produce antibodies and directly exert anti-tumor effects. Because of the relatively small number of B cells infiltrating tumors, their role in tumor immunity is often overlooked. With the development of technology, previously underestimated B-cell heterogeneity and clonal diversity can be further revealed by single-cell sequencing and immune bank sequencing (38). In different types of tumors, tumor-infiltrating B cells also exhibit anti-tumor or pro-tumor effects based on immune stimulatory or immunosuppressive effects. Studies have shown that the number of B cells in breast cancer, non-small cell lung cancer, HCC, CHOL, and other tumor tissues is positively correlated with better prognosis, and the reduction of the number and diversity of B cells in renal cancer is correlated with poor overall survival. The number of IL-10+ regulatory B cells (Breg) is positively associated with shorter total survival time in bladder and breast cancer (39,40). Similar results were observed in our study, suggesting that different B cells or different B cell states are closely related to both tumorigenesis and immunotherapy responsiveness. No in-depth functional studies of PDRG1 were performed, which is a limitation of our study.
Conclusions
In this review, PDRG1 is abnormally upregulated in different tumor tissues, and its abnormal expression is related to the prognosis of tumors. The abnormal expression of PDRG1 is related to the infiltration, methylation, and expression of TMB and MSI in immune cells. PDRG1 is a potential pan-cancer biomarker, and our results may provide a theoretical basis for its future clinical application in cancer diagnosis, treatment, and prognosis.
Acknowledgments
Funding: The study was supported by Innovative Ability Construction of Liuzhou Key Laboratory of Hepatocellular Carcinoma Research (No. 2018DB20502).
Footnote
Reporting Checklist: The authors have completed the REMARK reporting checklist. Available at https://atm.amegroups.com/article/view/10.21037/atm-22-5439/rc
Data Sharing Statement: Available at https://atm.amegroups.com/article/view/10.21037/atm-22-5439/dss
Conflicts of Interest: All authors have completed the ICMJE uniform disclosure form (available at https://atm.amegroups.com/article/view/10.21037/atm-22-5439/coif). The authors have no conflicts of interest to declare.
Ethical Statement: The authors are accountable for all aspects of the work in ensuring that questions related to the accuracy or integrity of any part of the work are appropriately investigated and resolved. The study was conducted in accordance with the Declaration of Helsinki (as revised in 2013). This study was approved by the Ethics Committee of Liuzhou People’s Hospital (No. KY2022-065-01), and written informed consent was obtained from the patient and/or family members before surgery.
Open Access Statement: This is an Open Access article distributed in accordance with the Creative Commons Attribution-NonCommercial-NoDerivs 4.0 International License (CC BY-NC-ND 4.0), which permits the non-commercial replication and distribution of the article with the strict proviso that no changes or edits are made and the original work is properly cited (including links to both the formal publication through the relevant DOI and the license). See: https://creativecommons.org/licenses/by-nc-nd/4.0/.
References
- Sung H, Ferlay J, Siegel RL, et al. Global Cancer Statistics 2020: GLOBOCAN Estimates of Incidence and Mortality Worldwide for 36 Cancers in 185 Countries. CA Cancer J Clin 2021;71:209-49. [Crossref] [PubMed]
- Cao W, Chen HD, Yu YW, et al. Changing profiles of cancer burden worldwide and in China: a secondary analysis of the global cancer statistics 2020. Chin Med J (Engl) 2021;134:783-91. [Crossref] [PubMed]
- Lin J, Ma L, Zhang D, et al. Tumour biomarkers-Tracing the molecular function and clinical implication. Cell Prolif 2019;52:e12589. [Crossref] [PubMed]
- Boonstra PA, Wind TT, van Kruchten M, et al. Clinical utility of circulating tumor DNA as a response and follow-up marker in cancer therapy. Cancer Metastasis Rev 2020;39:999-1013. [Crossref] [PubMed]
- Van Poznak C, Somerfield MR, Bast RC, et al. Use of Biomarkers to Guide Decisions on Systemic Therapy for Women With Metastatic Breast Cancer: American Society of Clinical Oncology Clinical Practice Guideline. J Clin Oncol 2015;33:2695-704. [Crossref] [PubMed]
- Wolff AC, Hammond MEH, Allison KH, et al. HER2 Testing in Breast Cancer: American Society of Clinical Oncology/College of American Pathologists Clinical Practice Guideline Focused Update Summary. J Oncol Pract 2018;14:437-41. [Crossref] [PubMed]
- Luo X, Huang Y, Sheikh MS. Cloning and characterization of a novel gene PDRG that is differentially regulated by p53 and ultraviolet radiation. Oncogene 2003;22:7247-57. [Crossref] [PubMed]
- Pajares MÁ. PDRG1 at the interface between intermediary metabolism and oncogenesis. World J Biol Chem 2017;8:175-86. [Crossref] [PubMed]
- Jiang L, Luo X, Shi J, et al. PDRG1, a novel tumor marker for multiple malignancies that is selectively regulated by genotoxic stress. Cancer Biol Ther 2011;11:567-73. [Crossref] [PubMed]
- Tao Z, Chen S, Mao G, et al. The PDRG1 is an oncogene in lung cancer cells, promoting radioresistance via the ATM-P53 signaling pathway. Biomed Pharmacother 2016;83:1471-7. [Crossref] [PubMed]
- Aran D, Hu Z, Butte AJ. xCell: digitally portraying the tissue cellular heterogeneity landscape. Genome Biol 2017;18:220. [Crossref] [PubMed]
- Becht E, Giraldo NA, Lacroix L, et al. Estimating the population abundance of tissue-infiltrating immune and stromal cell populations using gene expression. Genome Biol 2016;17:218. [Crossref] [PubMed]
- Charoentong P, Finotello F, Angelova M, et al. Pan-cancer Immunogenomic Analyses Reveal Genotype-Immunophenotype Relationships and Predictors of Response to Checkpoint Blockade. Cell Rep 2017;18:248-62. [Crossref] [PubMed]
- Finotello F, Mayer C, Plattner C, et al. Molecular and pharmacological modulators of the tumor immune contexture revealed by deconvolution of RNA-seq data. Genome Med 2019;11:34. [Crossref] [PubMed]
- Li T, Fan J, Wang B, et al. TIMER: A Web Server for Comprehensive Analysis of Tumor-Infiltrating Immune Cells. Cancer Res 2017;77:e108-10. [Crossref] [PubMed]
- Newman AM, Liu CL, Green MR, et al. Robust enumeration of cell subsets from tissue expression profiles. Nat Methods 2015;12:453-7. [Crossref] [PubMed]
- Racle J, de Jonge K, Baumgaertner P, et al. Simultaneous enumeration of cancer and immune cell types from bulk tumor gene expression data. Elife 2017;6:e26476. [Crossref] [PubMed]
- Zeng D, Ye Z, Shen R, et al. IOBR: Multi-Omics Immuno-Oncology Biological Research to Decode Tumor Microenvironment and Signatures. Front Immunol 2021;12:687975. [Crossref] [PubMed]
- Beroukhim R, Mermel CH, Porter D, et al. The landscape of somatic copy-number alteration across human cancers. Nature 2010;463:899-905. [Crossref] [PubMed]
- Bonneville R, Krook MA, Kautto EA, et al. Landscape of Microsatellite Instability Across 39 Cancer Types. JCO Precis Oncol 2017;2017:PO.17.00073.
- Aran D, Sirota M, Butte AJ. Systematic pan-cancer analysis of tumour purity. Nat Commun 2015;6:8971. [Crossref] [PubMed]
- Deng M, Peng L, Li J, et al. PPP1R14B Is a Prognostic and Immunological Biomarker in Pan-Cancer. Front Genet 2021;12:763561. [Crossref] [PubMed]
- Wu Y, Yu S, Qiao H. Understanding the functional inflammatory factors involved in therapeutic response to immune checkpoint inhibitors for pan-cancer. Front Pharmacol 2022;13:990445. [Crossref] [PubMed]
- Xu Y, Liu J, Jiang T, et al. PDRG1 predicts a poor prognosis and facilitates the proliferation and metastasis of colorectal cancer. Exp Cell Res 2021;409:112924. [Crossref] [PubMed]
- Zhang YJ, Li JQ, Li HZ, et al. PDRG1 gene silencing contributes to inhibit the growth and induce apoptosis of gastric cancer cells. Pathol Res Pract 2019;215:152567. [Crossref] [PubMed]
- Sun J, Xu Y, Liu J, et al. PDRG1 promotes the proliferation and migration of GBM cells by the MEK/ERK/CD44 pathway. Cancer Sci 2022;113:500-16. [Crossref] [PubMed]
- Tao Z, Zhang Y, Zhu S, et al. Knockdown of PDRG1 Could Inhibit the Wnt Signaling Pathway in Esophageal Cancer Cells. Ann Clin Lab Sci 2019;49:794-803. [PubMed]
- Galluzzi L, Yamazaki T, Kroemer G. Linking cellular stress responses to systemic homeostasis. Nat Rev Mol Cell Biol 2018;19:731-45. [Crossref] [PubMed]
- Yan LL, Zaher HS. How do cells cope with RNA damage and its consequences? J Biol Chem 2019;294:15158-71. [Crossref] [PubMed]
- Morris C, Cluet D, Ricci EP. Ribosome dynamics and mRNA turnover, a complex relationship under constant cellular scrutiny. Wiley Interdiscip Rev RNA 2021;12:e1658. [Crossref] [PubMed]
- Defronzo RA. Banting Lecture. From the triumvirate to the ominous octet: a new paradigm for the treatment of type 2 diabetes mellitus. Diabetes 2009;58:773-95. [Crossref] [PubMed]
- Reaven GM. Banting lecture 1988. Role of insulin resistance in human disease. Diabetes 1988;37:1595-607. [Crossref] [PubMed]
- Nead KT, Sharp SJ, Thompson DJ, et al. Evidence of a Causal Association Between Insulinemia and Endometrial Cancer: A Mendelian Randomization Analysis. J Natl Cancer Inst 2015;107:djv178. [Crossref] [PubMed]
- Paonessa F, Foti D, Costa V, et al. Activator protein-2 overexpression accounts for increased insulin receptor expression in human breast cancer. Cancer Res 2006;66:5085-93. [Crossref] [PubMed]
- Yang J, Nie J, Ma X, et al. Targeting PI3K in cancer: mechanisms and advances in clinical trials. Mol Cancer 2019;18:26. [Crossref] [PubMed]
- Kang BW, Chau I. Molecular target: pan-AKT in gastric cancer. ESMO Open 2020;5:e000728. [Crossref] [PubMed]
- Lee SH, Park SY, Choi CS. Insulin Resistance: From Mechanisms to Therapeutic Strategies. Diabetes Metab J 2022;46:15-37. [Crossref] [PubMed]
- Glass DR, Tsai AG, Oliveria JP, et al. An Integrated Multi-omic Single-Cell Atlas of Human B Cell Identity. Immunity 2020;53:217-232.e5. [Crossref] [PubMed]
- Ye J, Lee PP. B cell receptor signaling strength modulates cancer immunity. J Clin Invest 2022;132:e157665. [Crossref] [PubMed]
- Yi E. Studying extrachromosomal DNA with the ecTag method. Nat Rev Cancer 2022;22:320-1. [Crossref] [PubMed]