Derivation and validation of machine learning models for preoperative estimation of microvascular invasion risk in hepatocellular carcinoma
Highlight box
Key findings
• A novel machine learning model, based on α-fetoprotein, protein induced by vitamin K absence or antagonist-II, and tumor size, provides a convenient approach to predicting microvascular invasion risk before surgical intervention for patients with hepatocellular carcinoma.
What is known and what is new?
• Microvascular invasion is a significant risk factor for hepatocellular carcinoma recurrence and survival after hepatectomy;
• This study provides a preoperative microvascular invasion prediction model based on readily available clinical and radiographic characteristics using machine learning algorithms.
What is the implication, and what should change now?
• This model can be used as a convenient and explainable approach for microvascular invasion prediction before surgical intervention. This may assist clinicians in determining the optimal therapeutic modality and facilitate precision medicine for hepatocellular carcinoma.
Introduction
Hepatocellular carcinoma (HCC) ranks sixth in cancer morbidity and is the fourth leading cause of cancer-related mortality worldwide (1). HCC is refractory to most therapies, and the prognosis remains poor (2). As the backbone of curative therapies for HCC, surgical treatment yields optimal outcomes (3). However, the relatively high rate of recurrence and metastasis after hepatic resection remains a major obstacle (4).
Microvascular invasion (MVI) is defined by the presence of cancer cell clusters in vessels located within the tumor capsule or in the peritumoral liver under microscopy (5). MVI has been well recognized as a risk factor for the early recurrence and survival of HCC after hepatectomy (6,7). Preoperative assessment of MVI is of great value for selecting suitable surgical strategies and improving the survival outcomes of patients with HCC (8). MVI status cannot be determined on imaging and currently can only be reliably confirmed by pathological examination of surgical specimens, limiting its clinical applicability (9). Hence, noninvasive predictors of MVI are urgently needed to accurately evaluate the risk of cancer recurrence and to determine treatment strategy, particularly regarding surgical intervention.
Over the past decade, substantial efforts have been made in the preoperative assessment of MVI. The nomogram provides an accurate, evidence-based, and individualized risk prediction tool and has been widely adopted for model presentation. Recently, machine learning algorithms have been employed to construct prediction models in the field of liver cancer (10). Despite these efforts to construct an accurate MVI prediction model, most studies have not validated the prediction model in external cohorts, decreasing the generalizability of the proposed models.
Herein, we aimed to determine the preoperative risk factors of MVI and to propose an MVI prediction model based on the identified predictors. The prediction model was further validated in internal and external patient cohorts. We present the following article in accordance with the TRIPOD reporting checklist (available at https://atm.amegroups.com/article/view/10.21037/atm-22-2828/rc).
Methods
Patients and study design
The study was conducted in accordance with the Declaration of Helsinki (as revised in 2013). The study was approved by the Ethics Committee of the First Affiliated Hospital of Nanjing Medical University (No. 2020-SRFA-053) and the Ethics Committee of the First Affiliated Hospital of Wannan Medical College (No. 2018-15), and individual consent for this retrospective analysis was waived.
A total of 839 patients who underwent liver resection for histologically confirmed HCC at the First Affiliated Hospital of Nanjing Medical University (Nanjing, China) between January 1, 2020, and March 31, 2022, were retrospectively included. An independent external validation cohort of 291 patients with HCC who underwent hepatectomy at the First Affiliated Hospital of Wannan Medical College (Wuhu, China) from January 1, 2018, to December 31, 2021, was included.
The inclusion criteria were the following: (I) liver function of Child-Pugh class A or B; (II) completion of surgical resection; and (III) postoperative pathological diagnosis of HCC. The exclusion criteria were the following: (I) any preoperative anticancer treatment; (II) extrahepatic metastasis; (III) HCC with macrovascular invasion; (IV) incomplete radical resection; (V) a history of other cancers; and (VI) complications of infectious diseases, blood system diseases, or immune system diseases.
A total of 648 patients from the First Affiliated Hospital of Nanjing Medical University (the FAHNMU cohort) and 140 patients from the First Affiliated Hospital of Wannan Medical College (the FAHWMC cohort) were finally included in this study. The FAHNMU cohort was further randomly assigned to a derivation set and an internal validation set according to a ratio of 7:3 (n in derivation set =466; n in internal validation set =182), and the FAHWMC cohort was used for external validation. A flowchart of the study design is shown in Figure 1.
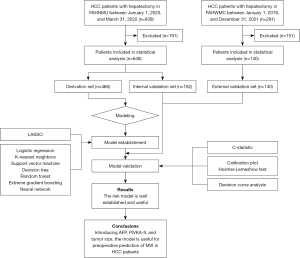
MVI diagnosis relied on the independent judgment of two experienced pathologists using a 7-point sampling protocol (11).
Statistical analysis
Variables associated with the presence of MVI were evaluated a priori according to clinical importance, scientific knowledge, and previously reported predictors. Categorical variables are presented as whole numbers and proportions, and continuous variables are reported as medians with interquartile ranges (IQRs). The cutoff values of continuous variables were determined by the reference ranges. The cutoff values of the neutrophil-to-lymphocyte ratio (NLR) and the platelet-to-lymphocyte ratio (PLR) were determined by the receiver operating characteristic (ROC) curve and the maximum Youden index.
Variables were examined for missing values before the commencement of data analysis. The proportion of missing data ranged between 0% and 10.7%. We imputed missing data by multiple imputation with chained equation methodology, using the multivariate imputation by chained equations (mice) package v. 3.14.0 in R (The R Foundation for Statistical Computing). Baseline characteristics before and after imputation in the FAHNMU and FAHWMC cohorts are listed in Tables S1,S2. Differences between the groups were examined using the Fisher exact test or chi-square test for categorical variables and the Mann-Whitney test for continuous variables.
To select the most useful prediction variables from all characteristics in the derivation cohort, we applied least absolute shrinkage and selection operator (LASSO) analysis by running cyclic coordinate descent with 10-fold cross-validation. Subsequently, we constructed the MVI prediction model using 7 machine learning models, including logistic regression, k-nearest neighbors, support vector machine, decision tree, random forest, extreme gradient boosting, and neural network. After adjusting the hyperparameters (Table S3), we obtained the final models of the derivation and further evaluated the internal and external validation sets. The discrimination ability of the model, which refers to the predictive accuracy of individual outcomes, was assessed by the area under the ROC curve (AUC) or concordance (C)-statistic. The value of the AUC is equivalent to the C-statistic. We calculated and compared the AUC to evaluate the predictive performance of different machine learning algorithms.
The performance of the prediction model was further evaluated using the Hosmer-Lemeshow calibration test and plot. Decision curve analysis (DCA) was performed to investigate the significance of the clinical application of the model by calculating the net benefits at each risk threshold probability. The difference in survival between different groups was assessed using Kaplan-Meier curves.
Statistical analyses were performed in R v. 4.1.3 (http://www.r-project.org). All tests were two-sided, and P<0.05 was considered statistically significant.
Results
Clinicopathologic characteristics
A total of 788 patients who met the inclusion criteria were included in the study and divided into the derivation set (466 patients), internal validation set (182 patients), and external validation set (140 patients).
Characteristics of the derivation, internal, and external validation cohorts are shown in Table 1. No statistically significant difference was found between the 3 cohorts. Histopathologically identified MVI was detected in 224 (48.07%), 89 (48.90%), and 52 (37.14%) of patients in the 3 cohorts, respectively.
Table 1
Characteristics | Total patient cohort (n=788) | Patients with MVI (n=365) | Patients without MVI (n=423) | Derivation set (n=466) | Internal validation set (n=182) | External validation set (n=140) | P value |
---|---|---|---|---|---|---|---|
Age per 10 years | 6.1 (5.4–6.9) | 6.0 (5.3–6.9) | 6.3 (5.5–6.9) | 6.1 (5.5–6.9) | 5.9 (5.3–6.9) | 6.4 (5.3–7.1) | 0.155 |
Gender | 0.306 | ||||||
Female | 140 (17.8) | 60 (16.4) | 80 (18.9) | 91 (19.5) | 27 (14.8) | 22 (15.7) | |
Male | 648 (82.2) | 305 (83.6) | 343 (81.1) | 375 (80.5) | 155 (85.2) | 118 (84.3) | |
Lymphocyte | 0.071 | ||||||
≤1.1×109/L | 223 (28.3) | 118 (32.3) | 105 (24.8) | 120 (25.8) | 53 (29.1) | 50 (35.7) | |
>1.1×109/L | 565 (71.7) | 247 (67.7) | 318 (75.2) | 346 (74.2) | 129 (70.9) | 90 (64.3) | |
Monocyte | 0.580 | ||||||
≤0.6×109/L | 640 (81.2) | 290 (79.5) | 350 (82.7) | 382 (82.0) | 143 (78.6) | 115 (82.1) | |
>0.6×109/L | 148 (18.8) | 75 (20.5) | 73 (17.3) | 84 (18.0) | 39 (21.4) | 25 (17.9) | |
Neutrophil | 0.315 | ||||||
≤6.3×109/L | 751 (95.3) | 347 (95.1) | 404 (95.5) | 447 (95.9) | 174 (95.6) | 130 (92.9) | |
>6.3×109/L | 37 (4.7) | 18 (4.9) | 19 (4.5) | 19 (4.1) | 8 (4.4) | 10 (7.1) | |
Platelet | 0.123 | ||||||
≤125×109/L | 322 (40.9) | 141 (38.6) | 181 (42.8) | 183 (39.3) | 71 (39.0) | 68 (48.6) | |
>125×109/L | 466 (59.1) | 224 (61.4) | 242 (57.2) | 283 (60.7) | 111 (61.0) | 72 (51.4) | |
NLR | 0.397 | ||||||
≤2.535 | 510 (64.7) | 223 (61.1) | 287 (67.8) | 304 (65.2) | 122 (67.0) | 84 (60.0) | |
>2.535 | 278 (35.3) | 142 (38.9) | 136 (32.2) | 162 (34.8) | 60 (33.0) | 56 (40.0) | |
PLR | 0.170 | ||||||
≤92.545 | 378 (48.0) | 151 (41.4) | 227 (53.7) | 219 (47.0) | 82 (45.1) | 77 (55.0) | |
>92.545 | 410 (52.0) | 214 (58.6) | 196 (46.3) | 247 (53.0) | 100 (54.9) | 63 (45.0) | |
ALT | 0.070 | ||||||
≤50 U/L | 634 (80.5) | 279 (76.4) | 355 (83.9) | 372 (79.8) | 156 (85.7) | 106 (75.7) | |
>50 U/L | 154 (19.5) | 86 (23.6) | 68 (16.1) | 94 (20.2) | 26 (14.3) | 34 (24.3) | |
AST | 0.158 | ||||||
≤40 U/L | 557 (70.7) | 233 (63.8) | 324 (76.6) | 326 (70.0) | 138 (75.8) | 93 (66.4) | |
>40 U/L | 231 (29.3) | 132 (36.2) | 99 (23.4) | 140 (30.0) | 44 (24.2) | 47 (33.6) | |
AKP | 0.398 | ||||||
≤120 U/L | 617 (78.3) | 264 (72.3) | 353 (83.5) | 357 (76.6) | 147 (80.8) | 113 (80.7) | |
>120 U/L | 171 (21.7) | 101 (27.7) | 70 (16.5) | 109 (23.4) | 35 (19.2) | 27 (19.3) | |
GGT | 0.151 | ||||||
≤60 U/L | 482 (61.2) | 191 (52.3) | 291 (68.8) | 274 (58.8) | 113 (62.1) | 95 (67.9) | |
>60 U/L | 306 (38.8) | 174 (47.7) | 132 (31.2) | 192 (41.2) | 69 (37.9) | 45 (32.1) | |
Total bilirubin | 0.421 | ||||||
≤19 μmol/L | 600 (76.1) | 274 (75.1) | 326 (77.1) | 354 (76.0) | 144 (79.1) | 102 (72.9) | |
>19 μmol/L | 188 (23.9) | 91 (24.9) | 97 (22.9) | 112 (24.0) | 38 (20.9) | 38 (27.1) | |
Albumin | 0.052 | ||||||
≥40 g/L | 265 (33.6) | 124 (34.0) | 141 (33.3) | 167 (35.8) | 63 (34.6) | 35 (25.0) | |
<40 g/L | 523 (66.4) | 241 (66.0) | 282 (66.7) | 299 (64.2) | 119 (65.4) | 105 (75.0) | |
PT | 0.221 | ||||||
≤14 s | 735 (93.3) | 340 (93.2) | 395 (93.4) | 437 (93.8) | 172 (94.5) | 126 (90.0) | |
>14 s | 53 (6.7) | 25 (6.8) | 28 (6.6) | 29 (6.2) | 10 (5.5) | 14 (10.0) | |
APTT | 0.920 | ||||||
≤31.3 s | 704 (89.3) | 323 (88.5) | 381 (90.1) | 423 (90.8) | 163 (89.6) | 118 (84.3) | |
>31.3 s | 84 (10.7) | 42 (11.5) | 42 (9.9) | 43 (9.2) | 19 (10.4) | 22 (15.7) | |
Fibrinogen | 0.588 | ||||||
≥2 g/L | 623 (79.1) | 303 (83.0) | 320 (75.7) | 374 (80.3) | 140 (76.9) | 109 (77.9) | |
<2 g/L | 165 (20.9) | 62 (17.0) | 103 (24.3) | 92 (19.7) | 42 (23.1) | 31 (22.1) | |
HBV | 0.661 | ||||||
No | 235 (29.8) | 99 (27.1) | 136 (32.2) | 140 (30.0) | 50 (27.5) | 45 (32.1) | |
Yes | 553 (70.2) | 266 (72.9) | 287 (67.8) | 326 (70.0) | 132 (72.5) | 95 (67.9) | |
HBV DNA load | 0.080 | ||||||
≤104 IU/mL | 655 (83.1) | 299 (81.9) | 356 (84.2) | 387 (83.0) | 144 (79.1) | 124 (88.6) | |
>104 IU/mL | 133 (16.9) | 66 (18.1) | 67 (15.8) | 79 (17.0) | 38 (20.9) | 16 (11.4) | |
HCV | 0.201 | ||||||
No | 764 (97.0) | 353 (96.7) | 411 (97.2) | 450 (96.6) | 175 (96.2) | 139 (99.3) | |
Yes | 24 (3.0) | 12 (3.3) | 12 (2.8) | 16 (3.4) | 7 (3.8) | 1 (0.7) | |
AFP | 0.174 | ||||||
≤20 ng/mL | 394 (50.0) | 125 (34.3) | 269 (63.6) | 234 (50.2) | 95 (52.2) | 65 (46.4) | |
20–400 ng/mL | 203 (25.8) | 107 (29.3) | 96 (22.7) | 127 (27.3) | 46 (25.3) | 30 (21.5) | |
≥400 ng/mL | 191 (24.2) | 133 (36.4) | 58 (13.7) | 105 (22.5) | 41 (22.5) | 45 (32.1) | |
PIVKA-II | 0.110 | ||||||
≤40 mAu/mL | 304 (38.6) | 83 (22.7) | 221 (52.2) | 166 (35.6) | 76 (41.8) | 62 (44.3) | |
>40 mAu/mL | 484 (61.4) | 282 (77.3) | 202 (47.8) | 300 (64.4) | 106 (58.2) | 78 (55.7) | |
Cirrhosis | 0.110 | ||||||
No | 239 (30.3) | 90 (24.7) | 147 (34.8) | 148 (31.8) | 44 (24.2) | 47 (33.6) | |
Yes | 549 (69.7) | 275 (75.3) | 276 (65.2) | 318 (68.2) | 138 (75.8) | 93 (66.4) | |
Ascites | 0.379 | ||||||
No | 700 (88.8) | 318 (87.1) | 382 (90.3) | 420 (90.1) | 158 (86.8) | 122 (87.1) | |
Yes | 88 (11.2) | 47 (12.9) | 41 (9.7) | 46 (9.9) | 24 (13.2) | 18 (12.9) | |
Tumor encapsulation | 0.098 | ||||||
Incomplete | 89 (11.3) | 50 (13.7) | 39 (9.2) | 44 (9.4) | 23 (12.6) | 22 (15.7) | |
Complete | 699 (88.7) | 315 (86.3) | 384 (90.8) | 422 (90.6) | 159 (87.4) | 118 (84.3) | |
Tumor number | 0.053 | ||||||
Solitary | 699 (88.7) | 325 (89.0) | 374 (88.4) | 405 (86.9) | 162 (89.0) | 132 (94.3) | |
Multiple | 89 (11.3) | 40 (11.0) | 49 (11.6) | 61 (13.1) | 20 (11.0) | 8 (5.7) | |
Tumor size | 0.760 | ||||||
≤5 cm | 490 (62.2) | 157 (43.0) | 333 (78.7) | 287 (61.6) | 112 (61.5) | 91 (65.0) | |
>5 cm | 298 (37.8) | 208 (57.0) | 90 (21.3) | 179 (38.4) | 70 (38.5) | 49 (35.0) |
Continuous data are presented as median (interquartile range), and categorical data are presented as n (%). P value denotes the statistical difference among the derivation, internal validation, and external validation sets, with the Fisher exact test or χ2 test being used for categorical data and the Mann-Whitney test for continuous data. AFP, α-fetoprotein; AKP, alkaline phosphatase; ALT, alanine aminotransferase; APTT, activated partial thromboplastin time; AST, aspartate transaminase; GGT, γ-glutamyl transferase; HBV, hepatitis B virus, HCV, hepatitis C virus; MVI, microvascular invasion; NLR, neutrophil-to-lymphocyte ratio; PIVKA-II, protein induced by vitamin K absence or antagonist-II; PLR, platelet-to-lymphocyte ratio; PT, prothrombin time.
Development of an MVI prediction model
We screened 28 variables to generate the optimal panel of included characteristics to construct the prediction model. Based on LASSO analysis, we identified 3 variables associated with MVI in the derivation set (Figure 2A,2B), including α-fetoprotein (AFP), protein induced by vitamin K absence or antagonist-II (PIVKA-II), and tumor size, with an optimal λ of 0.01611495. These 3 variables had nonzero coefficients in the LASSO analysis and were subsequently used to form the MVI risk estimation models.
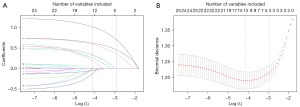
Next, we employed several machine learning algorithms, including logistic regression, k-nearest neighbors, support vector machine, decision tree, random forest, extreme gradient boosting, and neural network to construct and optimize the MVI prediction model. As shown in Table 2, we found that the logistic regression model achieved the largest AUC. Therefore, we developed the MVI risk nomogram based on the logistic regression algorithm (Figure 3A). For instance, a patient with HCC and PIVKA-II greater than 40 mAu/mL, tumor size larger than 5 cm, and an AFP level of 20–400 ng/mL had an estimated probability of MVI of 76.2% (Figure 3B). Furthermore, we deployed a user-friendly, online calculator (https://generalsurgery.shinyapps.io/MVIprediction/) that allowed real-time MVI probability prediction using the nomogram (Figure 3C).
Table 2
Algorithm | AUC (95% CI) | |
---|---|---|
Internal validation set | External validation set | |
Logistic regression | 0.771 (0.703–0.839) | 0.812 (0.734–0.891) |
K-nearest neighbors | 0.667 (0.601–0.733) | 0.710 (0.631–0.789) |
Support vector machine | 0.673 (0.607–0.739) | 0.742 (0.665–0.818) |
Decision tree | 0.676 (0.618–0.733) | 0.762 (0.691–0.833) |
Random forest | 0.660 (0.598–0.723) | 0.732 (0.655–0.809) |
Extreme gradient boosting | 0.738 (0.672–0.813) | 0.810 (0.732–0.890) |
Neural network | 0.630 (0.561–0.699) | 0.749 (0.674–0.824) |
AUC, area under the receiver operating characteristic curve; CI, confidence interval.
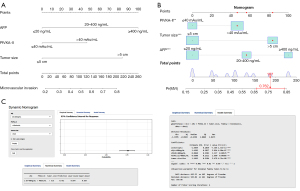
Discrimination of the MVI prediction model
As shown in Figure 4A-4C, the discrimination of the proposed model showed moderate to good performance for the derivation set [C-statistic 0.745; 95% confidence interval (CI): 0.701–0.790], internal validation set (C-statistic 0.771; 95% CI: 0.703–0.839), and external validation set (C-statistic 0.812; 95% CI: 0.734–0.891).
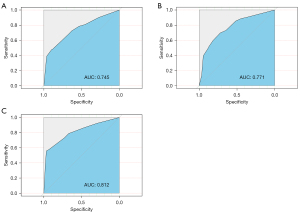
Model calibration
The Hosmer-Lemeshow calibration test was not significant for all 3 sets (derivation set: χ2=7.8775, P=0.4455; internal validation set: χ2=2.2515, P=0.9723; external validation set: χ2=3.6957, P=0.8835), indicating a good fit. Consistently, the calibration plots of the nomogram for these 3 cohorts showed moderate to good performance (Figure 5A-5C).
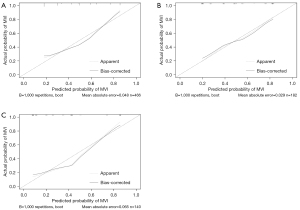
Clinical usefulness
According to the DCA results, the derivation set, internal validation set, and external validation set showed relatively good performance for the model in terms of clinical application compared with the strategies of treating all patients or treating no patients (Figure 6A-6C).
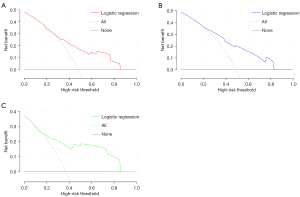
Furthermore, we compared patient survival between the two groups with or without MVI in the external validation set. As demonstrated in Figure 7A,7B, results from survival analyses showed that patients with either pathologically confirmed or model-predicted MVI exhibited poorer overall survival (P<0.001 for pathologically confirmed MVI and P=0.009 for model-predicted MVI), confirming the value of this study in the clinical context.
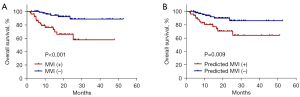
Discussion
The presence of MVI markedly worsens the survival outcomes of patients diagnosed with HCC (12). It is essential to determine the risk factors and develop prediction models for MVI to facilitate optimal therapeutic decisions. Herein, we incorporated clinical and radiographic characteristics to obtain a 3-feature-based MVI prediction model which was further validated in 2 independent patient cohorts. Based on the LASSO results, we demonstrated that 3 noninvasive preoperative variables, including AFP, PIVKA-II, and tumor size, were major factors associated with MVI. We compared the performance of several machine learning algorithms and constructed the prediction model using logistic regression, which exhibited the largest AUC among all algorithms tested.
In an attempt to identify the most important variables of MVI risk, we evaluated 28 noninvasive preoperative indicators, including clinical characteristics, imaging examination features, and laboratory test results. According to the LASSO results, we selected 3 major factors (AFP, PIVKA-II, and tumor size) to build the prediction model. AFP has been acknowledged as an important tumor biomarker that is associated with the risk of MVI in HCC (13,14). Reportedly, PIVKA-II may be more effective than AFP for early HCC diagnosis and is a predictive biomarker of MVI (15). Previous studies have confirmed the significance of tumor size in MVI risk prediction (16-18). Large tumor size is associated with an increased risk of MVI and HCC metastasis (19,20). Although tumor size, AFP, and PIVKA-II are significant factors related to MVI, clinical prediction models comprising only these 3 indicators are scant and have only been applied to patients with early HCC with single nodule disease (21-23).
It has been reported that multiomics data have also been evaluated in MVI prediction models. A recent study identified a transcriptomic signature to predict MVI in biopsy samples (24). However, liver biopsy is an invasive procedure and not routinely performed on patients with HCC who intend to undergo hepatectomy. With the rapid development of radiomics, a number of MVI prediction models have included multiscale and multiparametric data generated from radiomic techniques (25-27). Nonetheless, an acknowledged limitation of radiomics is the lack of reproducibility and repeatability of the radiomic variables generated (28). The results of radiomics may vary between different radiomics or statistical analysis software, from feature selection to model evaluation (29). In addition, some parameters in radiomics models are too specialized, and therefore the process of using these models is potentially cumbersome. In contrast, routine laboratory tests and clinical parameters are more commonly used and convenient for standardization, and data from different sources are comparable and accurate (30). In the present study, our model incorporated multidimensional predictors to successfully predict MVI risk before surgery using routine clinical and radiographic data. Moreover, we established an online calculator that could automatically and promptly calculate the risk probability of MVI.
Recently, several studies have used machine learning techniques in the preoperative risk estimation of MVI (31,32). Most machine learning algorithms analyze large amounts of heterogeneous data without predefined rules and have been characterized as “black box” models (33). This “black box” inexplicability can hardly help physicians fulfill their ethical duties. A lack of interpretability in prediction models can undermine the trust of patients and healthcare providers (34). In this study, we also evaluated several “black box” machine learning algorithms, including k-nearest neighbors, support vector machine, decision tree, random forest, extreme gradient boosting, and neural network. However, none of these algorithms yielded a larger AUC than that of the logistic regression model. Therefore, we focused on the logistic regression algorithm, which is explainable and convenient for clinicians.
The advantages of our study are as follows. First, we incorporated multidimensional variables to build the prediction model, and all variables have the advantages of convenient data acquisition, objectivity, and ready availability. Second, our study had a large sample size of 788 patients, and the enrolled patients were not restricted to those with early HCC and solitary, small-sized HCC. Third, both internal and external validation cohorts were used to confirm the prediction model. Effective training of the prediction model ensured that the model had sufficient predictive power in real-world situations. Fourth, we transformed the prediction model into a user-friendly, online calculator based on the 3 selected common clinical and radiographic variables. Hence, the prediction model can facilitate rapid risk detection and be easily adopted in regions with limited medical resources. Fifth, this study compared various machine learning algorithms to select the optimal model.
Several limitations with regard to this study should be noted. First, the retrospective nature of the present study introduced a potential for selection bias. Further prospective studies are needed to confirm the reliability and reproducibility of the model. Second, the patients recruited in this study were Chinese, and the inclusion of patients with HCC of different ethnicities is necessary in future studies to build external validation datasets. Third, a combination of clinical, radiographic, and multiomics signatures might further improve the prediction of MVI in patients with HCC.
Conclusions
We proposed a novel model based on AFP, PIVKA-II, and tumor size, which provided a convenient approach to predicting MVI before surgical intervention. According to the results of internal and external validation and the DCA curves, our model may assist clinicians in determining the optimal therapeutic modality by precisely predicting the MVI risk in patients with HCC.
Acknowledgments
Funding: This work was supported by the National Natural Science Foundation of China (Nos. 82002556, 82172651, 81972768, 31930020); the Natural Science Foundation of Jiangsu Province (No. BK20201083); the Natural Science Foundation of Anhui Province (No. 2108085MH287); the Support Plan for Outstanding Young Talents of Anhui Education Department (No. gxyq2021257); the Key Research and Development Project of Anhui Province (No. 202104j07020019); the Talent Introduction Science Foundation of Yijishan Hospital, Wannan Medical College (No. YR202110); and the Funding of “Peak” Training Program for Scientific Research of Yijishan Hospital, Wannan Medical College (No. GF2019J08).
Footnote
Reporting Checklist: The authors have completed the TRIPOD reporting checklist. Available at https://atm.amegroups.com/article/view/10.21037/atm-22-2828/rc
Data Sharing Statement: Available at https://atm.amegroups.com/article/view/10.21037/atm-22-2828/dss
Conflicts of Interest: All authors have completed the ICMJE uniform disclosure form (available at https://atm.amegroups.com/article/view/10.21037/atm-22-2828/coif). XW serves as an Editor-in-Chief of Annals of Translational Medicine from August 2019 to July 2024. The other authors have no conflicts of interest to declare.
Ethical Statement: The authors are accountable for all aspects of the work in ensuring that questions related to the accuracy or integrity of any part of the work are appropriately investigated and resolved. The study was conducted in accordance with the Declaration of Helsinki (as revised in 2013). The study was approved by the Ethics Committee of the First Affiliated Hospital of Nanjing Medical University (No. 2020-SRFA-053) and the Ethics Committee of the First Affiliated Hospital of Wannan Medical College (No. 2018-15), and individual consent for this retrospective analysis was waived.
Open Access Statement: This is an Open Access article distributed in accordance with the Creative Commons Attribution-NonCommercial-NoDerivs 4.0 International License (CC BY-NC-ND 4.0), which permits the non-commercial replication and distribution of the article with the strict proviso that no changes or edits are made and the original work is properly cited (including links to both the formal publication through the relevant DOI and the license). See: https://creativecommons.org/licenses/by-nc-nd/4.0/.
References
- Llovet JM, Kelley RK, Villanueva A, et al. Hepatocellular carcinoma. Nat Rev Dis Primers 2021;7:6. [Crossref] [PubMed]
- Zhou Q, Zhou C, Yin Y, et al. Development and validation of a nomogram combining hematological and imaging features for preoperative prediction of microvascular invasion in hepatocellular carcinoma patients. Ann Transl Med 2021;9:402. [Crossref] [PubMed]
- Gunasekaran G, Bekki Y, Lourdusamy V, et al. Surgical Treatments of Hepatobiliary Cancers. Hepatology 2021;73:128-36. [Crossref] [PubMed]
- Kim HI, An J, Kim JY, et al. Postresection Period-Specific Hazard of Recurrence as a Framework for Surveillance Strategy in Patients with Hepatocellular Carcinoma: A Multicenter Outcome Study. Liver Cancer 2022;11:141-51. [Crossref] [PubMed]
- Zhou J, Sun H, Wang Z, et al. Guidelines for the Diagnosis and Treatment of Hepatocellular Carcinoma (2019 Edition). Liver Cancer 2020;9:682-720. [Crossref] [PubMed]
- Ivanics T, Murillo Perez CF, Claasen MPAW, et al. Dynamic risk profiling of HCC recurrence after curative intent liver resection. Hepatology 2022;76:1291-301. [Crossref] [PubMed]
- Kang I, Jang M, Lee JG, et al. Subclassification of Microscopic Vascular Invasion in Hepatocellular Carcinoma. Ann Surg 2021;274:e1170-8. [Crossref] [PubMed]
- Lee S, Kang TW, Song KD, et al. Effect of Microvascular Invasion Risk on Early Recurrence of Hepatocellular Carcinoma After Surgery and Radiofrequency Ablation. Ann Surg 2021;273:564-71. [Crossref] [PubMed]
- Erstad DJ, Tanabe KK. Prognostic and Therapeutic Implications of Microvascular Invasion in Hepatocellular Carcinoma. Ann Surg Oncol 2019;26:1474-93. [Crossref] [PubMed]
- Calderaro J, Seraphin TP, Luedde T, et al. Artificial intelligence for the prevention and clinical management of hepatocellular carcinoma. J Hepatol 2022;76:1348-61. [Crossref] [PubMed]
- Sheng X, Ji Y, Ren GP, et al. A standardized pathological proposal for evaluating microvascular invasion of hepatocellular carcinoma: a multicenter study by LCPGC. Hepatol Int 2020;14:1034-47. [Crossref] [PubMed]
- Lin WP, Xing KL, Fu JC, et al. Development and Validation of a Model Including Distinct Vascular Patterns to Estimate Survival in Hepatocellular Carcinoma. JAMA Netw Open 2021;4:e2125055. [Crossref] [PubMed]
- Wang H, Lu Y, Liu R, et al. A Non-Invasive Nomogram for Preoperative Prediction of Microvascular Invasion Risk in Hepatocellular Carcinoma. Front Oncol 2021;11:745085. [Crossref] [PubMed]
- Lee JC, Hung HC, Wang YC, et al. Risk Score Model for Microvascular Invasion in Hepatocellular Carcinoma: The Role of Tumor Burden and Alpha-Fetoprotein. Cancers (Basel) 2021;13:4403. [Crossref] [PubMed]
- Ryu T, Takami Y, Wada Y, et al. A Clinical Scoring System for Predicting Microvascular Invasion in Patients with Hepatocellular Carcinoma Within the Milan Criteria. J Gastrointest Surg 2019;23:779-87. [Crossref] [PubMed]
- Yan Y, Zhou Q, Zhang M, et al. Integrated Nomograms for Preoperative Prediction of Microvascular Invasion and Lymph Node Metastasis Risk in Hepatocellular Carcinoma Patients. Ann Surg Oncol 2020;27:1361-71. [Crossref] [PubMed]
- Zhang C, Zhao R, Chen F, et al. Preoperative prediction of microvascular invasion in non-metastatic hepatocellular carcinoma based on nomogram analysis. Transl Oncol 2021;14:100875. [Crossref] [PubMed]
- Tang M, Zhou Q, Huang M, et al. Nomogram development and validation to predict hepatocellular carcinoma tumor behavior by preoperative gadoxetic acid-enhanced MRI. Eur Radiol 2021;31:8615-27. [Crossref] [PubMed]
- Hong SB, Choi SH, Kim SY, et al. MRI Features for Predicting Microvascular Invasion of Hepatocellular Carcinoma: A Systematic Review and Meta-Analysis. Liver Cancer 2021;10:94-106. [Crossref] [PubMed]
- Xia W, Peng T, Guan R, et al. Development of a novel prognostic nomogram for the early recurrence of liver cancer after curative hepatectomy. Ann Transl Med 2021;9:1541. [Crossref] [PubMed]
- Li H, Li T, Hu J, et al. A nomogram to predict microvascular invasion in early hepatocellular carcinoma. J Cancer Res Ther 2021;17:652-7. [Crossref] [PubMed]
- Yamashita YI, Imai K, Yusa T, et al. Microvascular invasion of single small hepatocellular carcinoma ≤3 cm: Predictors and optimal treatments. Ann Gastroenterol Surg 2018;2:197-203. [Crossref] [PubMed]
- Imai K, Yamashita YI, Yusa T, et al. Microvascular Invasion in Small-sized Hepatocellular Carcinoma: Significance for Outcomes Following Hepatectomy and Radiofrequency Ablation. Anticancer Res 2018;38:1053-60. [PubMed]
- Beaufrère A, Caruso S, Calderaro J, et al. Gene expression signature as a surrogate marker of microvascular invasion on routine hepatocellular carcinoma biopsies. J Hepatol 2022;76:343-52. [Crossref] [PubMed]
- Li Y, Zhang Y, Fang Q, et al. Radiomics analysis of [18F]FDG PET/CT for microvascular invasion and prognosis prediction in very-early- and early-stage hepatocellular carcinoma. Eur J Nucl Med Mol Imaging 2021;48:2599-614. [Crossref] [PubMed]
- Xu X, Zhang HL, Liu QP, et al. Radiomic analysis of contrast-enhanced CT predicts microvascular invasion and outcome in hepatocellular carcinoma. J Hepatol 2019;70:1133-44. [Crossref] [PubMed]
- Meng XP, Wang YC, Zhou JY, et al. Comparison of MRI and CT for the Prediction of Microvascular Invasion in Solitary Hepatocellular Carcinoma Based on a Non-Radiomics and Radiomics Method: Which Imaging Modality Is Better? J Magn Reson Imaging 2021;54:526-36. [Crossref] [PubMed]
- Mitchell-Hay RN, Ahearn TS, Murray AD, et al. Investigation of the Inter- and Intrascanner Reproducibility and Repeatability of Radiomics Features in T1-Weighted Brain MRI. J Magn Reson Imaging 2022;56:1559-68. [Crossref] [PubMed]
- Chong HH, Yang L, Sheng RF, et al. Multi-scale and multi-parametric radiomics of gadoxetate disodium-enhanced MRI predicts microvascular invasion and outcome in patients with solitary hepatocellular carcinoma ≤ 5 cm. Eur Radiol 2021;31:4824-38. [Crossref] [PubMed]
- Wang L, Jin YX, Ji YZ, et al. Development and validation of a prediction model for microvascular invasion in hepatocellular carcinoma. World J Gastroenterol 2020;26:1647-59. [Crossref] [PubMed]
- Chen G, Wang R, Zhang C, et al. Integration of pre-surgical blood test results predict microvascular invasion risk in hepatocellular carcinoma. Comput Struct Biotechnol J 2021;19:826-34. [Crossref] [PubMed]
- Liu W, Zhang L, Xin Z, et al. A Promising Preoperative Prediction Model for Microvascular Invasion in Hepatocellular Carcinoma Based on an Extreme Gradient Boosting Algorithm. Front Oncol 2022;12:852736. [Crossref] [PubMed]
- Rudin C. Stop Explaining Black Box Machine Learning Models for High Stakes Decisions and Use Interpretable Models Instead. Nat Mach Intell 2019;1:206-15. [Crossref] [PubMed]
- McCradden MD, Anderson JA, A, Stephenson E, et al. A Research Ethics Framework for the Clinical Translation of Healthcare Machine Learning. Am J Bioeth 2022;22:8-22. [Crossref] [PubMed]
(English Language Editors: K. Gilbert and J. Gray)