The impact of hypertension for metabolites in patients with acute coronary syndrome
Highlight box
Key findings
• An evaluation of the changes in circulatory metabolomics in patients with acute coronary syndrome (ACS), as well as the influence of hypertension, was studied.
What is known and what is new?
• Metabolomics has been widely used to identify the disease-specific biomarkers of ACS.
• Metabolic profiling of patients with ACS could be further compromised by hypertension.
What is the implication, and what should change now?
• Using serum metabolomics, it is possible to determine which molecules are differentially metabolized, thus allowing patients with ACS or those with hypertension to be classified.
• Both human and animal serum samples need to be comprehensively analyzed for the targeted screening of differential metabolites.
Introduction
Globally, coronary heart disease (CHD) poses a serious public health threat (1), with China accounting for 38.2% of the increase in deaths caused by CHD worldwide (2). A growing number of Chinese are being afflicted with CHD due to the aging population (3,4). Furthermore, a worsening profile of risk factors, such as an increased prevalence of hypertension, dyslipidemia, obesity, diabetes, and tobacco use, is contributing to the rise in the occurrence of CHD (4,5).
An acute coronary syndrome (ACS) is generally associated with the rupture of atherosclerotic vulnerable plaques and coronary thrombosis (6). With a very wide range of clinical presentations, ACS represents one of the leading causes of disability and death worldwide. With economic development and lifestyle change, the incidence of ACS tends to be younger and the corresponding mortality has been increasing since 2012 in China (7). Early diagnosis of ACS is imperative to provide optimized medical care and reduce case-fatality rates due to acute myocardial ischemia or heart failure (8). Traditional markers of myocardial injury, such as troponin and myocardial enzymology, have been widely adopted in clinical practice. However, the abnormal appearance of such markers often indicates that the disease has entered a severe stage. Such markers focus on myocardial damage itself and cannot describe the situation of the whole body well.
Atherosclerosis is significantly influenced by chronic inflammation during its initiation, progression, and destabilization (9). It is thought that inflammation of the vascular walls, caused by inflammatory leukocytes, resulted in the progression of the atherosclerosis and, ultimately, the destabilization cause plaque rupture and ACS (9). Acute phase proteins, including C-reactive protein, adhesion molecules, selectins, metalloproteinases, interleukins-1 and -6, tumour necrosis factor-a, vascular endothelial growth factor and so on, are important inflammatory mediators in ACS pathogenesis (10). Furthermore, inflammatory pathways not only regulate plaque properties, but also modulate atherosclerosis’ thrombotic complications (11). Chronic inflammatory reaction and subsequent unbalanced lipid metabolism contributes to the pathogenesis of atherosclerosis (12). On the basis of lipid metabolism, there are many biomarkers that can be used to predict ACS (13-15). However, most of these biomarkers have been derived from retrospective analysis, and the predictive accuracy is inconsistent. The development of novel circulatory biomarkers is therefore needed for the early detection of ACS (16,17).
In metabolomics, small-molecular-weight endogenous metabolites can be analyzed qualitatively and quantitatively (18,19). Precision metabolic profiling at the molecular species level has been enabled by advances in mass spectrometry and high-throughput metabolomics techniques (20). Metabolomics have been adopted extensively for identifying new biomarkers and identifying the potential targets of CHD (16,17,21). Paynter et al. (22) indicated that novel metabolites point to the pathophysiological relationship between lipid oxidation and subsequent CHD occurrence in women. Jiang et al. (16) reported that the serum oxyneurine concentration, triglyceride level, and weight are potential biomarkers for CHD diagnosis in the early stages. It is therefore highly desirable to develop serum metabolic biomarkers for diagnosing ACS. Jiang et al. (17) demonstrated that a combined model of serum betaine and ejection fraction could be a promising diagnostic biomarker for plaque vulnerability in patients with ACS.
Treatment and secondary prevention of ischemic coronary events in Croatia (TASPIC-CRO study) found that 66% of the 15,520 patients with established CHD in Croatia had high blood pressure between 1 June, 1998 and 31 March, 2003 (23). The prevalence of hypertension was 70.1% in a total of 1,298 patients with hospitalized CHD in the period of October 1st 2007 until January 7th 2010 at Croatia (24). Croatian patients with CHD continue to have a higher prevalence of hypertension than European average, a trend that is unfavourable (24). Rinkūniene et al. (25) found that the prevalence of hypertension was 47.7% in 606 patients with CHD in the Vilnius University Hospital between 1997 and 2005. Zheng et al. (26) followed 972 young patients (≤50 years of age) with CHD followed for one year. Most of the patients had poor management of risk factors and 64 patients (6.6%) experienced clinical outcomes. The baseline prevalence of hypertension and ACS were 34.3% and 51.2%, respectively (26).
A multifactorial etiology has been uncovered for the pathogenesis of hypertension (27). Genes, environmental effects, and their interactions influence hypertension development. Researchers have discovered a complex interaction of genetics and environment that influences risk-conferring behaviors, such as smoking, not exercising, drinking alcohol and eating unhealthy foods (28). When irreversible pathology has already occurred, hypertension and its underlying pathophysiology may be present for years before clinical diagnosis. As an expression of gene action and environmental exposure, the metabolome represents the result of multiple physiological and metabolic processes. Consequently, metabolomics may provide high-resolution, multifactorial phenotypic signatures of hypertension etiology, manifestation, and pathophysiology (29). Thus, human metabolome is a measure of gene-environment interactions and could identify novel pathways in the etiology of hypertension.
Metabolomics studies on hypertension have provided further insights into the identification of disease-specific biomarkers (30). 896 African American normotensives were investigated in the Atherosclerosis Risk in Communities (ARIC) Study and 38% of subjects developed hypertension during 10-years of follow-up. Baseline 4-hydroxyhippurate, a product of gut microbial fermentation, was associated with 17% higher risk of hypertension after controlling for traditional risk factors and renal function (29). The top-down lipidomics revealed that hypertension was an independent marker of lipidomic alterations caused by obesity and insulin resistance, along with specific reductions in ether lipids and free cholesterol (31). Kulkarni et al. identified the disturbance of diacylglycerol metabolism as an independent biomarker of hypertension (32). The EPIC-Potsdam study identified 127 metabolites related to the occurrence hypertension in a mean follow-up of 9.9 years (33). As ACS is often accompanied by hypertension, the study evaluated the effect of hypertension on the metabolism of ACS and aimed to identify novel circulatory metabolic biomarkers. We present the following article in accordance with the MDAR reporting checklist (available at https://atm.amegroups.com/article/view/10.21037/atm-22-6409/rc).
Methods
Chemicals and reagents
Chemicals and solvents used in this study were analytical grade or high performance liquid chromatography (HPLC) grade. We purchased acetonitrile and methanol from Thermo Fisher Scientific (Waltham, MA, USA). CNW Technologies GmbH (Düsseldorf, Germany) supplied us with pyridine, N-hexane, methoxyamine hydrochloride (97%), and trifluoroacetamide (BSTFA) with 1% trimethylchlorosilane (TMCS). L-2-chlorophenylalanine was obtained from Hengchuang Love Biotechnology Co., Ltd. (Ningbo, China).
Patients and sample collection
We recruited eligible participants between March 2021 and October 2021 at the First Affiliated Hospital, Zhejiang University Medical School. Exclusion criteria included patients with severe liver and kidney disease, hematological disorders, psychiatric disorders, diabetes, autoimmune diseases, malignancies, thoracalgia due to aortic dissection or pulmonary embolism, and acute pericarditis. On the basis of clinical characteristics, electrocardiogram examinations, cardiac troponin T (cTnT) levels, and coronary angiography, 20 patients were diagnosed with ACS, 21 patients with ACS complicated with hypertension (ACS + HTN), and 26 as healthy controls (HCs) (34) (Figure 1). The serum samples (about 900 µL) were collected and stored at –80 ℃ immediately after collection.
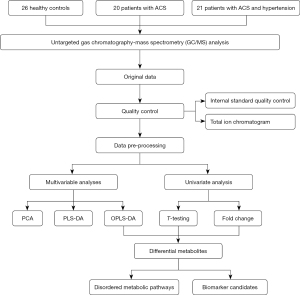
The Research Ethics Committee of the First Affiliated Hospital, Zhejiang University Medical School approved this study (institutional review board approval No. 2022/820). The informed consent of all participants was obtained before they were enrolled in the study. The study was conducted in accordance with the Declaration of Helsinki (as revised in 2013).
Sample preparation
Homogenization of blood samples was performed on patients. We transferred 150 µL of serum to an Eppendorf tube and added 10 µL of L-2-chlorophenylalanine (0.06 mg/mL) dissolved in methanol to serve as an internal standard. We extracted the mixed solution with 450 L of methanol and acetonitrile (2/1, vol/vol) and vortexed it for 30 seconds before placing it at –20 ℃ for 10 minutes. The extract was centrifuged for 10 minutes at 4 ℃ (13,000 rpm). A freeze concentration centrifugal dryer was used to dry 150 µL of supernatant in a glass vial. We prepared a quality control (QC) sample by mixing aliquots from all samples to create a pooled sample. At room temperature, 150 µL of supernatant was transferred to a glass sampling vial for vacuum drying. Following this, 80 µL of 15 mg/mL methoxyamine hydrochloride dissolved in pyridine were added. Following vigorous vortexing for 2 minutes, the mixture was incubated for 90 minutes at 37 ℃. In the mixture, 50 µL of BSTFA (containing 1% TMCS) and 20 µL of N-hexane were added. After vigorous vortexing for 2 minutes, the mixture was derivatized at 70 ℃ for 60 minutes. Before gas chromatography-mass spectrometry (GC/MS) analysis, samples were kept at ambient temperature for 30 minutes.
Untargeted metabolomics and measurement of metabolites
The derivative samples were analyzed with an Agilent 7890B gas chromatography system equipped with an Agilent 5977A MSD system (Agilent Technologies, Inc., Santa Clara, CA, USA). Separating the derivatives was achieved using a DB-5MS fused-silica capillary column (30 mm × 0.25 mm × 0.25 mm, Agilent J & W Scientific, Folsom, CA, USA). In the column, 1 mL/min of helium (>99.999%) flowed as the carrier gas. The temperature of the injector was maintained at 260 ℃ with an injection volume of 1L.
The initial oven temperature was 60 ℃, which was maintained at 60 ℃ for 0.5 minutes; increased to 125 ℃ at a rate of 8 ℃/min, 210 ℃ at a rate of 5 ℃/min, 270 ℃ at a rate of 10 ℃/min, and 305 ℃ at a rate of 20 ℃/min; and then maintained at 305 ℃ for 5 min. A temperature of 150 ℃ was set for the MS quadrupole, while 230 ℃ was set for the ion source (electron impact). Data were acquired in full-scan mode (m/z 50–500) with a collision energy of 70 eV.
Data preprocessing and statistical analysis
For quick retrieval of the raw data, the GC/MS raw data in D format were converted into axon binary format (ABF) format using Analysis Base File Converter software. The data were imported into the software MS-DIAL, which performed peak detection, peak identification, MS2Dec deconvolution, characterization, peak alignment, wave filtering, and missing value interpolation. A database called Lumingbio was used for the characterization of metabolic products. By using internal standards and quality control samples, quality control was assessed (Total Ion Chromatogram, Figure S1) and a data matrix was derived. This 3-dimensional matrix included sample information, peak names, retention times, retention indices, mass:charge ratios, and signal intensities. After screening, all peak signal intensities in each sample were segmented and normalized according to internal standards with a relative standard deviation (RSD) greater than 0.3. The data matrix was obtained by removing redundancies and merging peaks after normalization (available online: https://cdn.amegroups.cn/static/public/atm-22-6409-1.xlsx).
The matrix was imported in Cytoscape software 3.6.1 to carry out principle component analysis (PCA) to observe the overall distribution of samples and the stability of the whole analysis. We used orthogonal partial least-squares-discriminant analysis (OPLS-DA) and partial least-squares-discriminant analysis (PLS-DA) to identify metabolites that differed between groups. The model was evaluated using 7-fold cross-validation and 200 response permutation testing (RPT) to prevent overfitting. Furthermore, Kyoto Encyclopedia of Genes and Genomes (KEGG) provided metabolic pathways related to these metabolites.
Group discrimination was ranked based on the variable influence on projection (VIP) values obtained from the OPLS-DA model. Furthermore, a 2-tailed Student t-test was used to determine whether there were significant differences between groups in metabolites. Differential metabolites were selected if the VIP value was greater than 1.0 and the P value was less than 0.05. In order to improve the accuracy of metabolites screening, the condition of fold change (FC) ≥2 or ≤0.5 was further added (Figure 1).
Results
Clinical characteristics of participants
The clinical indices between the HC, ACS, and ACS-HTN groups are presented in Table 1; the mean ages were 45.96±13.2, 63.46±8.55, and 64.83±12.02 in the three groups, respectively; the prevalence rates of female participants were 69.23%, 25.00%, 28.57% in the three groups, respectively. The mean age, sex, systolic blood pressure (SBP), high-density lipoprotein cholesterol (HDL-C), alanine aminotransferase (ALT), aspartate aminotransferase (AST), serum creatinine, cTnT, creatine kinase-MB (CK-MB), and brain natriuretic peptide (BNP) as well as the prevalence of smokers between the HC and ACS groups were significantly different (all P values <0.05). The ACS and ACS-HTN patient groups showed no obvious differences in these clinical characteristics.
Table 1
Characteristic | Healthy control (N=26) | ACS (N=20) | ACS-HTN (N=21) | P1 value | P2 value |
---|---|---|---|---|---|
Female, n (%) | 18 (69.23%) | 5 (25.00%) | 6 (28.57%) | 0.003 | 0.796 |
Age (years) | 45.96±13.2 | 63.46±8.55 | 64.83±12.02 | 0.000 | 0.386 |
SBP (mmHg) | 119.56±11.44 | 126.92±11.35 | 123.06±14.96 | 0.009 | 0.325 |
DBP (mmHg) | 71.92±9.68 | 76.38±11.38 | 73.06±14.93 | 0.103 | 0.262 |
Heart rate (times/min) | 75.84±9.12 | 78.38±7.92 | 74.28±12.30 | 0.273 | 0.206 |
BMI (kg/m²) | 24.00±3.35 | 23.37±3.31 | 24.22±3.70 | 0.930 | 0.877 |
Smoker, n (%) | 2 (7.69%) | 7 (35.00%) | 9 (42.86%) | 0.029 | 0.606 |
FBG (mmol/L) | 4.69±0.71 | 4.67±0.55 | 5.09±0.75 | 0.747 | 0.115 |
TC (mmol/L) | 4.04±0.52 | 3.92±0.86 | 3.64±0.92 | 0.959 | 0.242 |
TG (mmol/L) | 0.96 (0.82–1.24) | 1.14 (0.79–1.44) | 1.25 (1.05–1.99) | 0.145 | 0.202 |
HDL-C (mmol/L) | 1.08 (0.99–1.39) | 0.99 (0.79–1.17) | 0.88 (0.74–1.07) | 0.019 | 0.165 |
LDL-C (mmol/L) | 2.32±0.45 | 2.21±0.71 | 1.94±0.71 | 0.925 | 0.165 |
ALT (U/L) | 12.00 (9.50–19.50) | 20.00 (13.75–33.75) | 25.00 (14.00–37.00) | 0.023 | 0.718 |
AST (U/L) | 15.00 (11.50–17.00) | 19.50 (16.75–32.75) | 20.50 (17.00–28.50) | 0.002 | 0.906 |
Serum creatinine (μmol/L) | 63.48±13.93 | 83.23±11.65 | 80.28±19.02 | 0.001 | 0.476 |
Cardiac troponin I (ng/mL) | 0.001 (0.000–0.002) | 0.015 (0.0075–5.919) | 0.017 (0.004–0.106) | 0.000 | 0.328 |
CK-MB (U/L) | 13.00 (9.50–17.00) | 16.50 (13.75–22.75) | 15.00 (11.00–19.00) | 0.013 | 0.592 |
SUA (μmol/L) | 291.20±83.59 | 342.69±49.87 | 356.39±91.04 | 0.108 | 0.148 |
CRP (mg/L) | 0.8 (0.39–3.10) | 1.74 (0.7–33.56) | 1.08 (0.6575–5.65) | 0.111 | 0.498 |
BNP (pg/mL) | 5.20 (0.00–21.00) | 78.50 (27.20–219.60) | 54.70 (18.05–158.73) | <0.001 | 0.537 |
Hemoglobin (g/L) | 135.68±16.07 | 130.38±17.29 | 133.11±14.76 | 0.642 | 0.796 |
Continuous variables are presented as the means ± standard deviation or the median (quartiles), as appropriate, and were compared using the Student t-test, or the Mann-Whitney test, depending on whether the quantitative data were consistent with a normal distribution. Categorical variables are presented as count (percentage), and differences between the groups were measured by chi-squared test. All statistical analyses were performed using SPSS 21.0 (IBM Corp., Armonk, NY, USA). All P values are 2-tailed, and P<0.05 was considered statistically significant. P1-value obtained from the healthy control group vs. acute coronary syndrome group. P2-value obtained from the ACS group vs. the ACS-HTN group. SBP, systolic blood pressure; DBP, diastolic blood pressure; BMI, body mass index; FBG, fasting blood glucose; TC, total cholesterol; TG, total triglyceride; HDL-C, high-density lipoprotein cholesterol; LDL-C, low-density lipoprotein cholesterol; AST, aspartate aminotransferase; ALT, alanine aminotransferase; CK-MB, creatine kinase-MB; SUA, serum uric acid; CRP, C-reactive protein; BNP, brain natriuretic peptide; ACS, acute coronary syndrome; HTN, hypertension.
Detection of dysregulated metabolites
Serum metabolites of 26 HCs, 20 patients with ACS, and 21 patients with ACS complicated with HTN were comparatively and qualitatively characterized, with 661 molecular features ultimately being acquired and analyzed (available online: https://cdn.amegroups.cn/static/public/atm-22-6409-2.xlsx). There were differential peak heights between the HCs, ACS, and ACS-HTN groups, as shown in Figure S2A-S2C).
Data on metabolites were statistically analyzed using R software (The R Foundation for Statistical Computing, Vienna, Austria). The PCA score plots derived from 7-fold cross-validation indicated separation tendencies between HC, ACS, and ACS-HTN groups (Figure 2A). Volcano plot indicated the obvious differential expression of metabolites between the ACS and HC groups (Figure 2B). There was an separation between HCs and patients with ACS in the PLS-DA score plots (R2X=0.427, R2Y=0.992, Q2=0.944; Figure S3) and the OPLS-DA score plots (R2X=0.373, R2Y=0.981, Q2=0.926; Figure 2C).
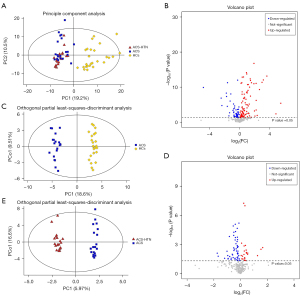
Moreover, the volcano plot indicated the obvious differential expression of metabolites for patients with ACS-HTN compared to those with ACS (Figure 2D). The PLS-DA score plots indicated an obvious trend of separation between ACS and ACS-HTN patients (R2X=0.338, R2Y=0.985, Q2=0.746; Figure S4). The OPLS-DA score plots indicated an obvious trend of separation between ACS and ACS-HTN patients (R2X=0.338, R2Y=0.985, Q2=0.637; Figure 2E).
Model quality was evaluated using 7-fold cross-validation and 200 times RPT to prevent overfitting. PCA score plots derived from 7-fold cross-validation indicated reliable results (Figure 2A). Two hundred times RPT indicated that OPLS-DA model was not overfitting (R2=0.241, Q2=−0.846; Figure S5). The correlation of metabolites between HCs and patients with ACS are presented in Figure S6, which shows the degree of correlation between the discrepant metabolites. The correlation of metabolites between patients with ACS and those with ACS-HTN are illustrated in Figure S7, which also shows the degree of correlation between the discrepant metabolites.
Dysregulated metabolites and metabolic pathways
The analysis of variance (ANOVA) identified 68 dysregulated metabolites between HCs and patients with ACS and 63 dysregulated metabolites between patients with ACS and those with ACS-HTN (Figure 3). There were 51 upregulated metabolites and 17 downregulated metabolites between HCs and patients with ACS. There were 19 upregulated metabolites and 44 downregulated metabolites between patients with ACS and those with ACS-HTN.
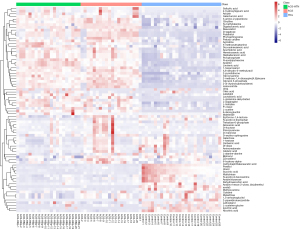
KEGG analyzed 82 metabolic pathways enriched by dysregulated metabolites between HCs and patients with ACS (available online: https://cdn.amegroups.cn/static/public/atm-22-6409-3.xlsx). There were 34 differential metabolites enriched in KEGG pathways between HC and patients with ACS (available online: https://cdn.amegroups.cn/static/public/atm-22-6409-4.xlsx). The 5 most prominent metabolic pathways (Figure 4A) containing differential metabolites between the patients with ACS and HCs were arginine biosynthesis; oxidative phosphorylation; alanine, aspartate, and glutamate metabolism; citrate cycle; and glucagon signaling pathway.
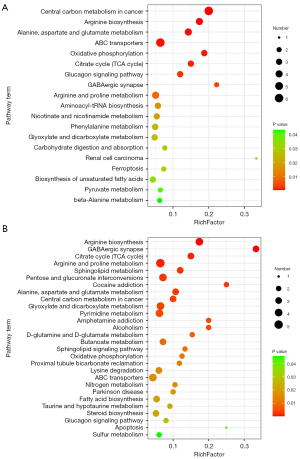
Based on KEGG analysis, 75 metabolic pathways enriched by dysregulated metabolites in patients with ACS and those with ACS-HTN were identified (available online: https://cdn.amegroups.cn/static/public/atm-22-6409-5.xlsx). There were 34 differential metabolites enriched in KEGG pathways between patients with ACS and those with ACS-HTN (available online: https://cdn.amegroups.cn/static/public/atm-22-6409-6.xlsx). The 5 most prominent metabolic pathways (Figure 4B) involved in the differential metabolites between the patients with ACS and those with ACS-HTN were steroid biosynthesis, fatty acid biosynthesis, arginine biosynthesis, primary bile acid biosynthesis, and tyrosine metabolism.
Markedly discrepant metabolites between different groups
To improve metabolite screening accuracy, the condition of FC ≥2 or ≤0.5 was added, as the VIP value was greater than 1.0 and the P value was less than 0.05. From 68 differential metabolites, 12 were clearly upregulated and 6 were significantly downregulated in the ACS group compared to the HC group, among which L-cystine and isocitric acid were the most obviously up- and downregulated, respectively (Table 2). To visualize the relative expression of these discrepant metabolites between the HC and ACS groups, we used box diagrams (Figure 5A-5H; Figure S6A-S6J). There was a high degree of relationality between the metabolites of HCs and those of patients with ACS (Figure S7).
Table 2
Metabolite name | Class | KEGG | RT (min) | VIP | P value | FC | Trend |
---|---|---|---|---|---|---|---|
L-cystine | Carboxylic acids and derivatives | C00491 | 23.04 | 3.32 | 6.75E-14 | 12.19 | ↑ |
D-tagatose | Organooxygen compounds | C00795 | 19.17 | 2.92 | 8.33E-13 | 8.82 | ↑ |
D-fructose | Organooxygen compounds | C02336 | 18.29 | 1.37 | 0.01 | 5.01 | ↑ |
Glycerol 3-phosphate | Glycerophospholipids | C00093 | 17.76 | 2.34 | 8.13E-12 | 4.53 | ↑ |
Arachidonic acid | Fatty acyls | C00219 | 23.57 | 1.85 | 3.70E-08 | 2.70 | ↑ |
Glycocyamine | Carboxylic acids and derivatives | C00581 | 15.23 | 1.96 | 1.20E-12 | 2.69 | ↑ |
Pyrophosphate | Non-metal oxoanionic compounds | C00013 | 16.35 | 1.92 | 4.19E-13 | 2.64 | ↑ |
Linolenic acid | Fatty acyls | C06427 | 23.71 | 1.84 | 9.37E-11 | 2.48 | ↑ |
Docosahexaenoic acid | Fatty acyls | C06429 | 24.63 | 1.52 | 6.96E-06 | 2.40 | ↑ |
Zymosterol | Steroids and steroid derivatives | C05437 | 28.49 | 1.46 | 2.31E-05 | 2.21 | ↑ |
Phytosphingosine | Organonitrogen compounds | C12144 | 16.02 | 1.69 | 1.80E-11 | 2.07 | ↑ |
Agmatine | Organonitrogen compounds | C00179 | 14.51 | 1.45 | 1.15E-05 | 2.02 | ↑ |
Nicotinic acid | Pyridines and derivatives | C00253 | 19.46 | 1.67 | 9.15E-09 | 0.44 | ↓ |
Succinic acid | Carboxylic acids and derivatives | C00042 | 11.05 | 1.7 | 9.57E-07 | 0.41 | ↓ |
Dehydroascorbic acid | Lactones | C05422 | 18.85 | 1.22 | 0.02 | 0.37 | ↓ |
Lathosterol | Steroids and steroid derivatives | C01189 | 28.78 | 2.2 | 5.23E-09 | 0.29 | ↓ |
Pentitol | Organooxygen compounds | C00474 | 16.82 | 2.39 | 1.82E-05 | 0.19 | ↓ |
Isocitric acid | Carboxylic acids and derivatives | C00311 | 10.61 | 3.44 | 1.17E-10 | 0.04 | ↓ |
Metabolites matched with commercially available reference standards. RT, retention time; FC, fold change; VIP, variable importance in the project obtained from the healthy control group vs. the acute coronary syndrome group; KEGG, Kyoto Encyclopedia of Genes and Genomes. ↑, fold change >2; ↓, fold change <0.5.
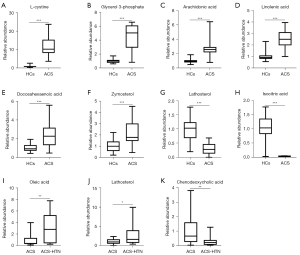
Moreover, 5 metabolites were screened out of the 63 differential metabolites. Compared to the in the ACS group, the ACS-HTN group had 3 metabolites that were obviously upregulated and 2 metabolites that were significantly downregulated, among which oleic acid and chenodeoxycholic acid were the most obviously up- and downregulated, respectively (Table 3). To visualize the relative expression of these discrepant metabolites between the ACS-HTN and ACS groups, we used box diagrams (Figure 5I-5K, Figure S6K-S6L). There was a high degree of relationality between the metabolites of patients with ACS and those with ACS-HTN (Figure S8).
Table 3
Metabolite name | Class | KEGG | RT (min) | VIP | P value | FC | Trend |
---|---|---|---|---|---|---|---|
Oleic acid | Fatty acyls | C00712 | 22.57 | 2.83 | 0.002 | 3.16 | ↑ |
Urea | Organic carbonic acids and derivatives | C00086 | 9.9 | 3.25 | 0.003 | 2.89 | ↑ |
Lathosterol | Steroids and steroid derivatives | C01189 | 22.78 | 2.72 | 0.015 | 2.41 | ↑ |
Dl-dopa | Carboxylic acids and derivatives | C00355 | 12.75 | 2.23 | 0.017 | 0.31 | ↓ |
Chenodeoxycholic acid | Steroids and steroid derivatives | C02528 | 29.71 | 2.95 | 0.008 | 0.30 | ↓ |
Metabolites matched with commercially available reference standards. ACS, acute coronary syndrome; HTN, hypertension; KEGG, Kyoto Encyclopedia of Genes and Genomes; RT, retention time; FC, fold change; VIP, variable importance in the project obtained from the ACS group vs. the ACS-HTN group. ↑: fold change >2; ↓: fold change <0.5.
Discrepancies in lipid metabolites between different groups
In view of the close relationship between blood lipids and ACS, we measured the related biomarkers of lipid metabolism. There are many metabolic discrepancies between HCs and patients with ACS. Glycerol 3-phosphate, arachidonic acid, linolenic acid, docosahexaenoic acid, zymosterol, and lathosterol belong to the lipid family or lipid-like molecular classes (Figure 5B-5G, available online: https://cdn.amegroups.cn/static/public/atm-22-6409-7.xlsx). Glycerol-3-phosphate production was most significantly increased in the ACS group, while lathosterol production was most significantly decreased. KEGG analysis showed that the biosynthesis of unsaturated fatty acids and steroid biosynthesis were the main metabolic signaling pathways involved in the differential lipid metabolites between the patients with ACS and the HCs (available online: https://cdn.amegroups.cn/static/public/atm-22-6409-7.xlsx).
There were many discrepancies between the patients with ACS and those with ACS-HTN when it comes to their metabolites. As mentioned above, oleic acid, lathosterol and chenodeoxycholic acid belong to the lipid family or lipid-like molecular classes (Figure 5I-5K, available online: https://cdn.amegroups.cn/static/public/atm-22-6409-8.xlsx). KEGG analysis showed that there were 4 metabolic pathways enriched by dysregulated lipid metabolites between the patients with ACS and those with ACS-HTN, including fatty acid biosynthesis, unsaturated fatty acid biosynthesis, steroid biosynthesis, and primary bile acid biosynthesis (available online: https://cdn.amegroups.cn/static/public/atm-22-6409-8.xlsx).
Discussion
ACS is a medical emergency associated with inflammatory response and oxidative stress (17,35,36). Advancements in metabolomics have provided insights into the potential mechanisms of ACS. We examined whether hypertension affects the metabolism of patients with ACS. From the analysis of baseline data, common clinical metabolites, including total cholesterol, total triglyceride, low-density lipoprotein cholesterol, and uric acid, did not differ significantly between the groups. Compared to the HC group, the ACS group had 12 significantly upregulated metabolites and 6 significantly downregulated metabolites. Among these, L-cystine and isocitric acid differed the most. Compared to the ACS group, the ACS-HTN group had 3 significantly upregulated metabolites and 2 significantly downregulated metabolites, among which oleic acid and chenodeoxycholic acid differed the most. Thus, hypertension might further affect the metabolic profiling of patients with CHD.
The relationship between lipid metabolism and cardiovascular disease has only recently been investigated, and much remains unknown. Newly developed metabolic profiling allows for the integrative analysis of small molecules under physiological or pathological conditions (18,19). The metabolomics capable of predicting cardiovascular diseases like atherosclerosis (16,17,21,22,37-44), dyslipidemia (45), and hypertension (46) has gradually come to the fore of research. In a large European cohort study consisting of 10,741 patients without CHD, there was a significant association between 5 phosphatidylcholines and an increased risk of CHD incidence, and there was comparable discrimination with classic risk factors (38). In a nested case-control study consisting of 4,662 patients, glycoprotein acetyls, ketone bodies, glucose, and docosahexaenoic acid were associated with myocardial infarction, ischemic stroke, and intracerebral hemorrhage (39). The Bioheat-CT study reported novel associations between specific coronary artery disease (CAD) plaque phenotypes, and 4 metabolites: dimethylguanidino valerate, glutamic acid, phenylalanine, and trimethylamine N-oxide (40). The lipid and nucleotide metabolic pathways were independently associated with the presence of CAD (40).
Metabolites associated with atherosclerosis are largely consistent among both coronary and carotid arteries beds, with lipid and carbohydrate metabolism, branched chain and aromatic amino acid metabolism, and oxidative stress and inflammatory pathways all revealed to be dysregulated in both (41).
Atherosclerosis Risk in Communities (ARIC) study identified 19 metabolites that when combined, could improve the risk prediction for CHD, among which theophylline and γ-linolenic acid were the most positive and negative, respectively (42). A comprehensive metabolomics analysis of human plasma from total 28 subjects with stable angina, myocardial infarction, and HCs revealed that the most significantly disturbed pathway was glycerophospholipid metabolism (43). Additionally, a targeted metabolomics approach showed that plasma levels of oxidized phospholipids, isoprostanes, and isomers of prostaglandins of patients with myocardial infarction were significantly elevated (43). An investigation using non-targeted lipidomics profiling aimed at shedding light on potential CHD biomarkers and therapeutics revealed a series of circulating lipids that were significantly altered in patients with CHD (44).
In our study, compared to the HC group, the ACS group had 12 obviously upregulated metabolites and 6 significantly downregulated metabolites, among which L-cystine and isocitric acid were the most obviously up- and downregulated, respectively. Oxidative stress plays a significant role in ACS, and part of its effect is mediated by lipid oxidation (17,35,36). In our research, L-cystine mainly was found to be involved in iron death and cysteine and methionine metabolic pathways. Human plasma contains cysteine and cystine, the 2 main disulfide redox molecules. As a result of L-cystine’s ability to regulate glutathione’s extracellular concentration, it is sensitive to oxidative stress (47). Dysregulation of this redox contributes to cardiovascular disease, including atherosclerosis through proinflammatory signaling (48). Isocitric acid is a more effective antioxidant than ascorbic acid (49). In our study, isocitric acid was mainly involved in the citrate cycle, the glucagon signaling pathway, and the metabolism of glyoxylates and dicarboxylates. Isocitric acid was identified as a novel coronary lesion-specific marker, independent of cholesterol levels and aortic lesions, in WHHLMI rabbits, and might be a promising metric for the early diagnosis of patients with CHD (50). Our hypothesis was that myocardial ischemia reduces isocitric acid levels by enhancing isocitrate dehydrogenase activity in the citrate cycle.
There are many lipid metabolic discrepancies between HCs and patients with ACS. Compared to the HCs, glycerol-3-phosphate was most significantly increased in the ACS group, while lathosterol was most significantly decreased. Glucose and fat metabolism intersect at glycerol-3-phosphate, which is formed during glycolysis, and its availability can influence energy and intermediary metabolism in mammals. Glycerol-3-phosphate phosphatase regulates energy and intermediary metabolism, as well as physiological functions such as insulin secretion, hepatic glucose production, and fat synthesis, storage, and oxidation (51). Metabolic risk factors for ACS may be modulated by disruptions of a single glycolytic enzyme. Hypertriglyceridemia might be explained by a loss of glyceraldehyde-3-phosphate dehydrogenase (GA3PDH) responsiveness to insulin, by causing accumulation of glycolytic intermediates including glycerol-3-phosphate (52).
Human serum lathosterol concentration is a measure of whole-body cholesterol synthesis. Gas chromatography analysis of serum lathosterol could be identified as the marker of cholesterol synthesis to predict the efficacy of simvastatin in patients with CHD (53). The highest plasma lathosterol concentration was associated with a lack of response of plasma cholesterol during the phytosterols treatment period (54). Lathosterol synthesis was decreased in patients with aortic stenosis who had a positive family history of cardiovascular disease and concomitant CHD (55). Patients who did not receive lipid-lowering agents had increased cardiovascular event risk and excess all-cause mortality when serum lathosterol was low (56). The serum level of lanosterol was closely associated with CVD risk factors, while the ratio of lathosterol to cholesterol might reflect statin response (57).
Compared to the ACS group, the ACS-HTN group had 3 metabolites that were obviously upregulated and 6 metabolites that were significantly downregulated among which oleic acid and chenodeoxycholic acid were the most obviously up- and downregulated, respectively. In the metabolism of fatty acids, oleic acid belongs to the monounsaturated group of fatty acids (58). Several studies have confirmed its potential as a target for infections, and inflammatory, immune, and cardiovascular diseases (58). Monounsaturated fatty acids have protective effects for the human body, including the lowering of cholesterol and blood glucose and the improvement of insulin sensitivity and endothelial dysfunction (59). However, it also has potential adverse effects, such as the promotion of lipid peroxidation and inhibition of immune function (60). There is evidence that free fatty acids (FFAs), such as linoleic acid and stearic acid, can modulate microvascular function and contribute to obesity-related insulin resistance and hypertension (61). Ceramide, triacylglycerol, total glycolipids, and oleic acid levels have been found to be positively associated with longitudinal diastolic pressure change (62). Furthermore, oleic acid was found to have a close association with pulmonary arterial hypertension occurrence (63). Our hypothesis was that the increased oleic acid was the body’s self-protective mechanism against hypertension.
Primary bile acids (BAs) such as chenodeoxycholic acid are essential for the digestive-absorptive process of cholesterol, triglycerides, and liposoluble vitamin (64). Furthermore, BAs can regulate epithelial cell proliferation and glycolipid metabolism in the enterohepatic cycle as signaling molecules (64). Chenodeoxycholic acid can activate type 2 iodothyronine deiodinase (D2) in brown adipose tissue via the G protein-coupled bile acid receptor 1 (TGR5), leading to enhancive oxygen consumption and energy expenditure (65). Chenodeoxycholic acid prevents high-fat diet-induced obesity and hyperlipidemia via the activation of TGR5 and the inhibition of proliferator-activated receptor γ pathway (66). We speculated that the decreased chenodeoxycholic acid present in patients with ACS was caused by the pathophysiological pathways that induce hypertension.
KEGG metabolic pathway analysis showed that these differential metabolites were mainly concentrated in oxidative phosphorylation, amino acid metabolism, fatty acid metabolism, sphingolipid metabolism, and citrate cycle. In particular, the discrepant lipids metabolites between HCs and patients with ACS included glycerol 3-phosphate, arachidonic acid, linolenic acid, docosahexaenoic acid, docosahexaenoic acid, zymosterol, and lathosterol. Discrepant lipid metabolites between patients with ACS and ACS-HTN included oleic acid, lathosterol, and chenodeoxycholic acid. KEGG analysis showed that biosynthesis of unsaturated fatty acids, steroid biosynthesis fatty acid, biosynthesis, and primary bile acid biosynthesis were the main metabolic pathways enriched by these dysregulated lipid metabolites. Reactive oxygen species might be increased by FFAs, leading to oxidative stress and insulin resistance as a result of signaling pathways related to FFAs (67). These findings suggest that these dysregulated metabolites may be involved in the metabolic-related signaling pathways that contributed to ACS pathogenesis.
This study has some limitations that should be noted. Considering that this study was validated in a small sample, its accuracy should be scrutinized by further investigation. Our experiment only preliminarily screened out the possible differential metabolites between patients with ACS and HCs through non-targeted metabolomics. Further targeting of the screened differential metabolites and enlargement of the sample size are needed, along with a comprehensive data analysis of human serum and animal samples. It would be useful to conduct a prospective cohort-based metabolomics study to confirm the differential expression of these metabolites among patients with ACS and those with hypertension complications.
Conclusions
A comprehensive analysis of the circulatory metabolomics change in patients with ACS was conducted and hypertension’s influence on its metabolism was examined. A serum metabolomics test can be used to identify differentially metabolized molecules and allow for the classification of patients with ACS or those complicated with hypertension.
Acknowledgments
This work was supported by the Alibaba-Zhejiang University Joint Research Center of Future Digital Healthcare and Alibaba Cloud.
Funding: This work was supported by a grant from the National Natural Science Foundation of China (No. 82170489); a grant from the Natural Science Foundation of Zhejiang Province (Nos. LR22H020001, LY23H010007); and a grant from the Project of Medical Science Research Foundation from the Health Department of Zhejiang Province (No. WKJ-ZJ-2312).
Footnote
Reporting Checklist: The authors have completed the MDAR reporting checklist. Available at https://atm.amegroups.com/article/view/10.21037/atm-22-6409/rc
Data Sharing Statement: Available at https://atm.amegroups.com/article/view/10.21037/atm-22-6409/dss
Conflicts of Interest: All authors have completed the ICMJE uniform disclosure form (available at https://atm.amegroups.com/article/view/10.21037/atm-22-6409/coif). The authors have no conflicts of interest to declare.
Ethical Statement: The authors are accountable for all aspects of the work in ensuring that questions related to the accuracy or integrity of any part of the work are appropriately investigated and resolved. The Research Ethics Committee of the First Affiliated Hospital, Zhejiang University Medical School approved this study (institutional review board approval No. 2022/820). The informed consent of all participants was obtained before they were enrolled in the study. The study was conducted in accordance with the Declaration of Helsinki (as revised in 2013).
Open Access Statement: This is an Open Access article distributed in accordance with the Creative Commons Attribution-NonCommercial-NoDerivs 4.0 International License (CC BY-NC-ND 4.0), which permits the non-commercial replication and distribution of the article with the strict proviso that no changes or edits are made and the original work is properly cited (including links to both the formal publication through the relevant DOI and the license). See: https://creativecommons.org/licenses/by-nc-nd/4.0/.
References
- Abreu D, Sousa P, Matias-Dias C, et al. Impact of public health initiatives on acute coronary syndrome fatality rates in Portugal. Rev Port Cardiol 2020;39:27-34. (Engl Ed). [Crossref] [PubMed]
- Roth GA, Mensah GA, Johnson CO, et al. Global Burden of Cardiovascular Diseases and Risk Factors, 1990-2019: Update From the GBD 2019 Study. J Am Coll Cardiol 2020;76:2982-3021. [Crossref] [PubMed]
- Wang W, Jiang B, Sun H, et al. Prevalence, Incidence, and Mortality of Stroke in China: Results from a Nationwide Population-Based Survey of 480 687 Adults. Circulation 2017;135:759-71. [Crossref] [PubMed]
- Zhang XH, Lu ZL, Liu L. Coronary heart disease in China. Heart 2008;94:1126-31. [Crossref] [PubMed]
- DiNicolantonio JJ, Lucan SC, O'Keefe JH. The Evidence for Saturated Fat and for Sugar Related to Coronary Heart Disease. Prog Cardiovasc Dis 2016;58:464-72. [Crossref] [PubMed]
- Goodacre S, Pett P, Arnold J, et al. Clinical diagnosis of acute coronary syndrome in patients with chest pain and a normal or non-diagnostic electrocardiogram. Emerg Med J 2009;26:866-70. [Crossref] [PubMed]
- Report on Cardiovascular Health and Diseases in China 2021: An Updated Summary. Biomed Environ Sci 2022;35:573-603. [PubMed]
- Reihani H, Sepehri Shamloo A, Keshmiri A. Diagnostic Value of D-Dimer in Acute Myocardial Infarction Among Patients With Suspected Acute Coronary Syndrome. Cardiol Res 2018;9:17-21. [Crossref] [PubMed]
- Sager HB, Nahrendorf M. Inflammation: a trigger for acute coronary syndrome. Q J Nucl Med Mol Imaging 2016;60:185-93. [PubMed]
- Owczarek A, Babińska M, Szyguła-Jurkiewicz B, et al. Chronic inflammation in patients with acute coronary syndrome and chronic kidney disease. Kardiol Pol 2011;69:388-93. [PubMed]
- Libby P, Tabas I, Fredman G, et al. Inflammation and its resolution as determinants of acute coronary syndromes. Circ Res 2014;114:1867-79. [Crossref] [PubMed]
- Liao M, Hu F, Qiu Z, et al. Pim-2 kinase inhibits inflammation by suppressing the mTORC1 pathway in atherosclerosis. Aging (Albany NY) 2021;13:22412-31. [Crossref] [PubMed]
- Ridker PM. C-reactive protein and the prediction of cardiovascular events among those at intermediate risk: moving an inflammatory hypothesis toward consensus. J Am Coll Cardiol 2007;49:2129-38. [Crossref] [PubMed]
- Wang Y, Li L, Tan HW, et al. Transcoronary concentration gradient of sCD40L and hsCRP in patients with coronary heart disease. Clin Cardiol 2007;30:86-91. [Crossref] [PubMed]
- Antoniades C, Bakogiannis C, Tousoulis D, et al. The CD40/CD40 ligand system: linking inflammation with atherothrombosis. J Am Coll Cardiol 2009;54:669-77. [Crossref] [PubMed]
- Jiang H, Li L, Chen W, et al. Application of Metabolomics to Identify Potential Biomarkers for the Early Diagnosis of Coronary Heart Disease. Front Physiol 2021;12:775135. [Crossref] [PubMed]
- Jiang H, Jiang H, Zhang J, et al. The Serum Metabolic Biomarkers in Early Diagnosis and Risk Stratification of Acute Coronary Syndrome. Front Physiol 2020;11:776. [Crossref] [PubMed]
- Nicholson JK, Lindon JC. Systems biology: Metabonomics. Nature 2008;455:1054-6. [Crossref] [PubMed]
- Johnson CH, Gonzalez FJ. Challenges and opportunities of metabolomics. J Cell Physiol 2012;227:2975-81. [Crossref] [PubMed]
- Zampieri M, Sekar K, Zamboni N, et al. Frontiers of high-throughput metabolomics. Curr Opin Chem Biol 2017;36:15-23. [Crossref] [PubMed]
- Deidda M, Noto A, Cadeddu Dessalvi C, et al. Metabolomic correlates of coronary atherosclerosis, cardiovascular risk, both or neither. Results of the 2 × 2 phenotypic CAPIRE study. Int J Cardiol 2021;336:14-21. [Crossref] [PubMed]
- Paynter NP, Balasubramanian R, Giulianini F, et al. Metabolic Predictors of Incident Coronary Heart Disease in Women. Circulation 2018;137:841-53. [Crossref] [PubMed]
- Reiner Z, Mihatov S, Milicić D, et al. Treatment and secondary prevention of ischemic coronary events in Croatia (TASPIC-CRO study). Eur J Cardiovasc Prev Rehabil 2006;13:646-54. [Crossref] [PubMed]
- Vrazić H, Sikić J, Lucijanić T, et al. The prevalence of hypertension among Croatian hospitalized coronary heart disease patients. Coll Antropol 2012;36:217-21. [Crossref] [PubMed]
- Rinkūniene E, Petrulioniene Z, Laucevicius A, et al. Prevalence of conventional risk factors in patients with coronary heart disease. Medicina (Kaunas) 2009;45:140-6. [Crossref] [PubMed]
- Zheng R, Liu Y, Hao Z, et al. Clinical Characteristics and Prognosis of Young Patients with Coronary Heart Disease. Med Sci Monit 2020;26:e922957. [Crossref] [PubMed]
- Rossier BC, Bochud M, Devuyst O. The Hypertension Pandemic: An Evolutionary Perspective. Physiology (Bethesda) 2017;32:112-25. [Crossref] [PubMed]
- Padmanabhan S, Caulfield M, Dominiczak AF. Genetic and molecular aspects of hypertension. Circ Res 2015;116:937-59. [Crossref] [PubMed]
- Zheng Y, Yu B, Alexander D, et al. Metabolomics and incident hypertension among blacks: the atherosclerosis risk in communities study. Hypertension 2013;62:398-403. [Crossref] [PubMed]
- Au A, Cheng KK, Wei LK. Metabolomics, Lipidomics and Pharmacometabolomics of Human Hypertension. Adv Exp Med Biol 2017;956:599-613. [Crossref] [PubMed]
- Graessler J, Schwudke D, Schwarz PE, et al. Top-down lipidomics reveals ether lipid deficiency in blood plasma of hypertensive patients. PLoS One 2009;4:e6261. [Crossref] [PubMed]
- Kulkarni H, Meikle PJ, Mamtani M, et al. Plasma lipidomic profile signature of hypertension in Mexican American families: specific role of diacylglycerols. Hypertension 2013;62:621-6. [Crossref] [PubMed]
- Dietrich S, Floegel A, Weikert C, et al. Identification of Serum Metabolites Associated With Incident Hypertension in the European Prospective Investigation into Cancer and Nutrition-Potsdam Study. Hypertension 2016;68:471-7. [Crossref] [PubMed]
- Zhang M, Wu J, Zhang X, et al. Prevalence and control of hypertension in adults in China, 2018. Zhonghua Liu Xing Bing Xue Za Zhi 2021;42:1780-9. [PubMed]
- Pouralijan Amiri M, Khoshkam M, Salek RM, et al. Metabolomics in early detection and prognosis of acute coronary syndrome. Clin Chim Acta 2019;495:43-53. [Crossref] [PubMed]
- Gianazza E, Brioschi M, Martinez Fernandez A, et al. Lipid Peroxidation in Atherosclerotic Cardiovascular Diseases. Antioxid Redox Signal 2021;34:49-98. [Crossref] [PubMed]
- Jing Z, Liu L, Shi Y, et al. Association of Coronary Artery Disease and Metabolic Syndrome: Usefulness of Serum Metabolomics Approach. Front Endocrinol (Lausanne) 2021;12:692893. [Crossref] [PubMed]
- Cavus E, Karakas M, Ojeda FM, et al. Association of Circulating Metabolites With Risk of Coronary Heart Disease in a European Population: Results From the Biomarkers for Cardiovascular Risk Assessment in Europe (BiomarCaRE) Consortium. JAMA Cardiol 2019;4:1270-9. [Crossref] [PubMed]
- Holmes MV, Millwood IY, Kartsonaki C, et al. Lipids, Lipoproteins, and Metabolites and Risk of Myocardial Infarction and Stroke. J Am Coll Cardiol 2018;71:620-32. [Crossref] [PubMed]
- Vernon ST, Tang O, Kim T, et al. Metabolic Signatures in Coronary Artery Disease: Results from the BioHEART-CT Study. Cells 2021;10:980. [Crossref] [PubMed]
- Tzoulaki I, Castagné R, Boulangé CL, et al. Serum metabolic signatures of coronary and carotid atherosclerosis and subsequent cardiovascular disease. Eur Heart J 2019;40:2883-96. [Crossref] [PubMed]
- Wang Z, Zhu C, Nambi V, et al. Metabolomic Pattern Predicts Incident Coronary Heart Disease. Arterioscler Thromb Vasc Biol 2019;39:1475-82. [Crossref] [PubMed]
- Lu J, Chen B, Chen T, et al. Comprehensive metabolomics identified lipid peroxidation as a prominent feature in human plasma of patients with coronary heart diseases. Redox Biol 2017;12:899-907. [Crossref] [PubMed]
- Yang L, Wang L, Deng Y, et al. Serum lipids profiling perturbances in patients with ischemic heart disease and ischemic cardiomyopathy. Lipids Health Dis 2020;19:89. [Crossref] [PubMed]
- Sun JH, Liu X, Cong LX, et al. Metabolomics study of the therapeutic mechanism of Schisandra Chinensis lignans in diet-induced hyperlipidemia mice. Lipids Health Dis 2017;16:145. [Crossref] [PubMed]
- Ameta K, Gupta A, Kumar S, et al. Essential hypertension: A filtered serum based metabolomics study. Sci Rep 2017;7:2153. [Crossref] [PubMed]
- McBean GJ, Flynn J. Molecular mechanisms of cystine transport. Biochem Soc Trans 2001;29:717-22. [Crossref] [PubMed]
- Go YM, Jones DP. Cysteine/cystine redox signaling in cardiovascular disease. Free Radic Biol Med 2011;50:495-509. [Crossref] [PubMed]
- Morgunov IG, Karpukhina OV, Kamzolova SV, et al. Investigation of the effect of biologically active threo-Ds-isocitric acid on oxidative stress in Paramecium caudatum. Prep Biochem Biotechnol 2018;48:1-5. [Crossref] [PubMed]
- Shiomi M, Takeda H, Irino Y, et al. Identification of novel serum markers for the progression of coronary atherosclerosis in WHHLMI rabbits, an animal model of familial hypercholesterolemia. Atherosclerosis 2019;284:18-23. [Crossref] [PubMed]
- Possik E, Madiraju SRM, Prentki M. Glycerol-3-phosphate phosphatase/PGP: Role in intermediary metabolism and target for cardiometabolic diseases. Biochimie 2017;143:18-28. [Crossref] [PubMed]
- Leyva F, Wingrove CS, Godsland IF, et al. The glycolytic pathway to coronary heart disease: a hypothesis. Metabolism 1998;47:657-62. [Crossref] [PubMed]
- Wu WF, Wang QH, Zhang T, et al. Gas chromatography analysis of serum cholesterol synthesis and absorption markers used to predict the efficacy of simvastatin in patients with coronary heart disease. Clin Biochem 2013;46:993-8. [Crossref] [PubMed]
- Nunes VS, Ilha AOG, Ferreira GDS, et al. Plasma lathosterol measures rates of cholesterol synthesis and efficiency of dietary phytosterols in reducing the plasma cholesterol concentration. Clinics (Sao Paulo) 2022;77:100028. [Crossref] [PubMed]
- Weingärtner O, Weingärtner N, Scheller B, et al. Alterations in cholesterol homeostasis are associated with coronary heart disease in patients with aortic stenosis. Coron Artery Dis 2009;20:376-82. [Crossref] [PubMed]
- Weingärtner O, Lütjohann D, Meyer S, et al. Low serum lathosterol levels associate with fatal cardiovascular disease and excess all-cause mortality: a prospective cohort study. Clin Res Cardiol 2019;108:1381-5. [Crossref] [PubMed]
- Kwon GE, Hyun MH, Byun DJ, et al. Metabolic signatures of cholesterol biosynthesis and absorption in patients with coronary artery disease. J Steroid Biochem Mol Biol 2021;212:105940. [Crossref] [PubMed]
- Sales-Campos H, Souza PR, Peghini BC, et al. An overview of the modulatory effects of oleic acid in health and disease. Mini Rev Med Chem 2013;13:201-10. [PubMed]
- Kazaz S, Miray R, Lepiniec L, et al. Plant monounsaturated fatty acids: Diversity, biosynthesis, functions and uses. Prog Lipid Res 2022;85:101138. [Crossref] [PubMed]
- Plötz T, Krümmel B, Laporte A, et al. The monounsaturated fatty acid oleate is the major physiological toxic free fatty acid for human beta cells. Nutr Diabetes 2017;7:305. [Crossref] [PubMed]
- de Jongh RT, Serné EH, Ijzerman RG, et al. Free fatty acid levels modulate microvascular function: relevance for obesity-associated insulin resistance, hypertension, and microangiopathy. Diabetes 2004;53:2873-82. [Crossref] [PubMed]
- Lin YT, Salihovic S, Fall T, et al. Global Plasma Metabolomics to Identify Potential Biomarkers of Blood Pressure Progression. Arterioscler Thromb Vasc Biol 2020;40:e227-37. [Crossref] [PubMed]
- Chen C, Luo F, Wu P, et al. Metabolomics reveals metabolite changes of patients with pulmonary arterial hypertension in China. J Cell Mol Med 2020;24:2484-96. [Crossref] [PubMed]
- Di Ciaula A, Garruti G, Lunardi Baccetto R, et al. Bile Acid Physiology. Ann Hepatol 2017;16:s4-s14. [Crossref]
- Broeders EP, Nascimento EB, Havekes B, et al. The Bile Acid Chenodeoxycholic Acid Increases Human Brown Adipose Tissue Activity. Cell Metab 2015;22:418-26. [Crossref] [PubMed]
- Chen X, Yan L, Guo Z, et al. Chenodeoxycholic acid attenuates high-fat diet-induced obesity and hyperglycemia via the G protein-coupled bile acid receptor 1 and proliferator-activated receptor γ pathway. Exp Ther Med 2017;14:5305-12. [Crossref] [PubMed]
- Christiansen E, Hudson BD, Hansen AH, et al. Development and Characterization of a Potent Free Fatty Acid Receptor 1 (FFA1) Fluorescent Tracer. J Med Chem 2016;59:4849-58. [Crossref] [PubMed]
(English Language Editor: J. Gray)