Landscape of costimulatory molecule signature in breast cancer and its prognostic significance
Highlight box
Key findings
• The landscape of CMS in BRCA was analyzed, and excellent prognosis models were established in each subtype.
What is known and what is new?
• CMS play an important role in the activation of T cells in tumor immune response, and mature drugs are available at present.
• Based on pathological cognition, an effective prognostic model of costimulatory factors of various subtypes of BRCA was established.
What is the implication, and what should change now?
• The model established in this study can effectively predict the prognosis of patients with different subtypes of BRCA, and put forward treatment suggestions.
Introduction
Breast cancer (BRCA) has become the most common malignant tumor in women worldwide. Due to its heterogeneity, many mature drugs are not effective (1). New directions in therapy include administration of immune checkpoint inhibitors (ICIs), such as programmed cell death protein 1 (PD-1) and PD-1 antibody and programmed death-ligand 1 (PD-L1) antibody. They solve issues that cannot be avoided in the conventional treatment, such as a lack of apparent short-term effect, the inevitable side effects of chemotherapy on the treated patients, the inability to control disease progression over time, and poor outcome (2). ICI-based immunotherapy has developed well, and BRCA prognosis has improved (3,4). However, only a small portion of those with BRCA can obtain good outcomes from this treatment (5). Therefore, means to determining the best candidates for immunotherapy and developing new immune detection targets are urgently needed.
ICI therapy cures tumors by restoring the interference of the tumor microenvironment (TME) on the activation and killing processes of immune cells such as T cells (6,7). Tumor cells are identified by T cell receptor (TCR) of natural killer T cell (NKT) to produce specific signals (8,9). Then, complete activation of T cells is completed by costimulatory molecule signature (CMS) (10). The expression of CMS in an inhibitory TME can change the normal activation process of immune cells (11,12). Therefore, ICI therapy can restore this immune abnormality to treat tumors (13).
CMS affects T cell activation, proliferation, survival, and cytokine secretion and plays an essential role in regulating tumor immunity (14). The B7 family consists of 8 molecules (CD80, CD86, PD-L1, PD-L2, ICOSLG, B7-H3, B7x, and HHLA2) (15), and the CD28 family consists of 5 molecules (CD28, CTLA4, ICOS, PD-1, and TMIGD2) (16). The 2 families come together to form the CD28-B7 family, from which PD-L1/PD-1 and CD86/CTLA4 belong to the immune checkpoint pathway (17,18). The tumor necrosis factor (TNF) ligand superfamily (TNFSF) and TNF receptor superfamily (TNFRSF) (19) compose the TNF family. There are 60 members in all, and some of them have been studied (20-22). However, these members’ combined effect and clinical significance are unclear, so it is necessary to conduct a comprehensive study of these molecules in BRCA patients to find new treatment strategies.
With the great success of other solid tumors targeting ICIs in clinical applications, we seek to find a new CMS with specificity for guiding the clinical treatment of BRCA. The CMSs with tumor-specific expression were selected. Then, through least absolute shrinkage and selection operator (LASSO) regression and cyclic Cox regression analysis of The Cancer Genome Atlas (TCGA) data set, the optimal variables for constructing the model were screened out, and the prognostic risk assessment model of CMS was constructed. However, TCGA within-group validation verification process did not yield good prediction results. We suspect this was due to the numerous molecular types within BRCA. The CMS prognostic model thus cannot accurately describe the overall BRCA prognosis results. Consequently, a CMS with different subtypes and normal tissues was developed. The same prediction model construction method was used to construct the new model, and within-group verification was performed, it has proved that the prognosis model established in various subtypes can effectively predict the prognosis.
For the first time, our work described the panoramic analysis of costimulatory molecules based on B7-CD28 and TNF family in all molecular subtypes of BRCA. A unique and effective prognosis model was established for each subtype, and the potential clinical application of costimulatory factors was emphasized, which provided theoretical support for the prognosis management and immunotherapy of various subtypes of BRCA patients. We present the following article in accordance with the TRIPOD reporting checklist (available at https://atm.amegroups.com/article/view/10.21037/atm-22-6245/rc).
Methods
Public messenger RNA (mRNA) expression datasets
We systematically screened BRCA mRNA sequencing (mRNA-seq) data (TCGA-BRCA, 1,109 BRCA patients and 113 healthy controls’ mRNA-seq data) from TCGA to explore the expression peculiarity, the prognosis information, and the response to treatment of CMS. An external test data set (GSE42568) was used for validation and downloaded from the Gene Expression Omnibus (GEO) database (https://www.ncbi.nlm.nih.gov/geo/). All mRNA data processing standards were in line with public second-generation high-throughput sequencing platforms. Cases without clinical information or those with CMSs with low mRNA expression levels were removed (more than half of the samples had an expression value of 0). We screened the CMS expression of 1,089 BRCA patients to obtain the CMS panoramic and prognostic analysis (Table 1) (23). We further selected 4 recognized molecular subtypes of BRCA (https://xena.ucsc.edu/): basal-like BRCA (BASAL), human epidermal growth factor receptor 2-enriched BRCA (HER2), luminal A BRCA (LUMA), and luminal B BRCA (LUMB) (24). The tumor-specific CMSs of each subtype were screened (P<0.05) and combined with the corresponding survival time information. The study was conducted in accordance with the Declaration of Helsinki (as revised in 2013).
Table 1
ID | Other names | Family | HR | HR.95L | HR.95H | P value |
---|---|---|---|---|---|---|
CD274 | PD-L1, B7-H1 | B7 | 0.90618937 | 0.7867342 | 1.04378223 | 0.1719938 |
CD276 | B7-H3 | B7 | 1.01515928 | 0.99844664 | 1.03215166 | 0.07566421 |
CD80 | B7-1, CD28LG1 | B7 | 1.03571376 | 0.72028877 | 1.48926796 | 0.84980565 |
CD86 | B7-2, CD28LG2 | B7 | 0.97834426 | 0.92597672 | 1.03367339 | 0.43537992 |
HHLA2 | B7-H5 | B7 | 0.00577569 | 3.14E-06 | 10.6219678 | 0.17899279 |
ICOSLG | B7-H2, CD275 | B7 | 1.02407962 | 0.54605798 | 1.9205636 | 0.94088014 |
PDCD1LG2 | PD-L2, B7DC, CD273 | B7 | 0.91609046 | 0.8252223 | 1.01696443 | 0.10010595 |
VTCN1 | B7-H4 | B7 | 1.00004577 | 0.99887692 | 1.00121598 | 0.93886494 |
CD28 | Tp44 | CD28 | 0.9202512 | 0.79042151 | 1.07140591 | 0.28413443 |
CTLA4 | CD152 | CD28 | 0.90690926 | 0.8085523 | 1.01723093 | 0.09525997 |
ICOS | CD278, CVID1 | CD28 | 0.90547788 | 0.79448975 | 1.03197076 | 0.13668113 |
PDCD1 | PD-1, CD279 | CD28 | 0.87735729 | 0.77405834 | 0.99444161 | 0.04064093 |
TMIGD2 | CD28H | CD28 | 0.51849713 | 0.28556182 | 0.94143982 | 0.03090828 |
CD27 | TNFRSF7 | TNFRSF | 0.95358065 | 0.91710989 | 0.99150176 | 0.0168985 |
CD40 | TNFRSF5 | TNFRSF | 0.95739166 | 0.91889399 | 0.99750222 | 0.03758101 |
EDA2R | TNFRSF27, XEDAR | TNFRSF | 1.29181983 | 1.13298695 | 1.47291941 | 0.00013064 |
EDAR | EDA-A1R | TNFRSF | 0.83551011 | 0.48216856 | 1.44778651 | 0.52170969 |
FAS | TNFRSF6, CD95 | TNFRSF | 0.97408207 | 0.93029663 | 1.01992831 | 0.26311239 |
LTBR | TNFRSF3 | TNFRSF | 0.99635411 | 0.98044493 | 1.01252144 | 0.6564975 |
NGFR | TNFRSF16, CD271 | TNFRSF | 0.97383521 | 0.94130712 | 1.00748734 | 0.12611328 |
RELT | TNFRSF19L | TNFRSF | 0.965696 | 0.80680521 | 1.15587846 | 0.70351811 |
TNFRSF10A | TRAILR1, CD261 | TNFRSF | 1.00741762 | 0.94370324 | 1.0754337 | 0.82454599 |
TNFRSF10B | TRAILR2, CD262 | TNFRSF | 0.98437014 | 0.9454612 | 1.02488032 | 0.44391584 |
TNFRSF10C | TRAILR3, CD263 | TNFRSF | 1.05310689 | 0.92669458 | 1.19676336 | 0.42772237 |
TNFRSF10D | TRAILR4, CD264 | TNFRSF | 0.99114626 | 0.90149916 | 1.08970807 | 0.85412494 |
TNFRSF11A | RANK, CD265 | TNFRSF | 0.98592928 | 0.86956738 | 1.11786225 | 0.82497562 |
TNFRSF11B | OPG | TNFRSF | 0.99582722 | 0.96660156 | 1.02593653 | 0.78321104 |
TNFRSF12A | FN14, TWEAKR, CD266 | TNFRSF | 0.99615606 | 0.98849017 | 1.00388139 | 0.32850793 |
TNFRSF13B | TACI, TNFRSF14B, CD267 | TNFRSF | 0.62384324 | 0.37355545 | 1.04182763 | 0.07133195 |
TNFRSF13C | BAFFR, CD268 | TNFRSF | 0.87235258 | 0.74665819 | 1.01920669 | 0.08537683 |
TNFRSF14 | LIGHTR, HVEM, CD270 | TNFRSF | 0.91186061 | 0.86843839 | 0.95745396 | 0.00021014 |
TNFRSF17 | BCMA, TNFRSF13A, CD269 | TNFRSF | 0.94325224 | 0.87913362 | 1.01204727 | 0.10383219 |
TNFRSF18 | GITR, AITR, CD357 | TNFRSF | 0.97597548 | 0.95314141 | 0.99935657 | 0.04408834 |
TNFRSF19 | TROY, TAJ | TNFRSF | 0.96305506 | 0.91754805 | 1.01081906 | 0.12744656 |
TNFRSF1A | TNFR1, CD120A | TNFRSF | 0.99235135 | 0.97760473 | 1.00732041 | 0.31483092 |
TNFRSF1B | TNFR2, CD120B | TNFRSF | 0.97809485 | 0.95894412 | 0.99762803 | 0.02813842 |
TNFRSF21 | DR6, CD358 | TNFRSF | 1.00514255 | 0.99442978 | 1.01597073 | 0.34812118 |
TNFRSF25 | DR3, TNFRSF12 | TNFRSF | 0.92387929 | 0.83601188 | 1.02098183 | 0.12048706 |
TNFRSF4 | OX40, CD134 | TNFRSF | 0.97309554 | 0.89997603 | 1.05215571 | 0.49378071 |
TNFRSF6B | DCR3 | TNFRSF | NA | NA | NA | NA |
TNFRSF8 | CD30 | TNFRSF | 0.48173788 | 0.28956709 | 0.80144252 | 0.0049197 |
TNFRSF9 | 4-1BB, CD137, ILA | TNFRSF | 0.78840241 | 0.61154183 | 1.01641184 | 0.06659995 |
CD40LG | TNFSF5, CD154, CD40L | TNFSF | 0.82331268 | 0.69350165 | 0.977422 | 0.02636278 |
CD70 | TNFSF7, CD27L | TNFSF | 0.84588855 | 0.65764939 | 1.08800747 | 0.19250822 |
EDA | EDA-A1, EDA-A2 | TNFSF | 1.31909047 | 0.95053491 | 1.83054787 | 0.09761617 |
FASLG | TNFSF6, CD95-L | TNFSF | 0.80943956 | 0.65790002 | 0.99588445 | 0.04561293 |
LTA | TNFSF1 | TNFSF | 0.82120529 | 0.66552639 | 1.01330036 | 0.06624502 |
LTB | TNFSF3 | TNFSF | 0.98654754 | 0.97088894 | 1.00245868 | 0.09708761 |
TNF | TNFSF2, TNFA | TNFSF | 0.97181714 | 0.90327616 | 1.04555904 | 0.44362768 |
TNFSF10 | TRAIL, CD253 | TNFSF | 1.00088224 | 0.99833159 | 1.0034394 | 0.4981763 |
TNFSF11 | RANKL, CD254 | TNFSF | 1.00011272 | 0.96997313 | 1.03118882 | 0.99423978 |
TNFSF12 | TWEAK | TNFSF | 0.96807948 | 0.93932971 | 0.99770919 | 0.03493894 |
TNFSF13 | APRIL, CD256 | TNFSF | 0.99666685 | 0.95142363 | 1.04406152 | 0.88798362 |
TNFSF13B | BAFF, CD257 | TNFSF | 0.96769873 | 0.92700724 | 1.01017641 | 0.13412644 |
TNFSF14 | LIGHT, HVEML, CD258 | TNFSF | 0.71933172 | 0.49533846 | 1.04461528 | 0.0835128 |
TNFSF15 | TL1A | TNFSF | 0.94484839 | 0.84370713 | 1.05811417 | 0.32605969 |
TNFSF18 | GITRL | TNFSF | 1.05339076 | 0.70728115 | 1.56886988 | 0.79800763 |
TNFSF4 | OX-40L, CD134L, CD252 | TNFSF | 1.12176372 | 1.02793224 | 1.22416029 | 0.00993467 |
TNFSF8 | CD30L, CD153 | TNFSF | 0.91126814 | 0.76686072 | 1.08286891 | 0.291174 |
TNFSF9 | 4-1BB-L, CD137L | TNFSF | 1.03716052 | 0.94076189 | 1.14343699 | 0.46351416 |
CMS, costimulatory molecule signature; TCGA, The Cancer Genome Atlas; BRCA, breast cancer; HR, hazard ratio; HR.95L, HR with low 95% CI; CI, confidence index; HR.95H, HR with high 95% CI; TNFRSF, TNF receptor superfamily; TNF, tumor necrosis factor; TNFSF, TNF ligand superfamily; NA, not available.
Validation
According to the above-mentioned tumor subtypes, we screened the mRNA data of tumor-specific CMSs corresponding to each subtype. Then, through LASSO regression and cyclic Cox regression analysis of TCGA-BRCA data set, the optimal variables for constructing the model were screened out, and the prognostic risk assessment model of CMS (BRCA-model) was constructed. The survival rate of each patient was calculated in the form of nomogram. TCGA-BRCA cases were randomly divided into a training group and test group. According to the “Surv-Cutpoint” function in the “Survminer” R software package (The R Foundation for Statistical Computing, USA), the optimal cutpoint was determined. The external validation data set was selected from the GEO database (GSE42568). Patients from all the cohorts were divided into high-risk and low-risk groups. The Kaplan-Meier curve and the double-tailed logarithmic rank-sum test were used to calculate the prognostic model (25). The CMS expression heat map of the model, receiver operating characteristic (ROC) area prediction survival curve (26), and multiple-factor Cox analysis with other clinical traits proved that the model could be used as an independent prognostic predictor. Therefore, our work describes the overall prospect of CMS based on the CD28-B7 and TNF family and highlights the potential clinical application of CMS: supporting the theoretical development of prognosis management and immunotherapy for BRCA subtypes.
Functional enrichment analysis
After building a predictive model, the sample cases were scored and divided into high-risk and low-risk groups for comparison and obtained the difference genes. Gene Ontology (GO) and Kyoto Encyclopedia of Genes and Genomes (KEGG) were used to analyze the target genes’ biochemical reaction, molecular function, and cell component enrichment. It was found that these genes were enriched in biological functions such as monooxygenase, endopeptidase, oxidoreductase activity, and multicellular homeostasis.
Estimation of the characteristics of immune cell infiltration
We used CIBERSORT and the fragments per kilobase million (FPKM) of TCGA-BRCA RNA sequencing (RNA-seq) data to estimate the scores of 22 immune cell types in BRCA’s TME. The leukocyte gene marker matrix LM22 of CIBERSORT software was used to complete the analysis of various immune cell types. LM22 comprises 547 genes, with 22 kinds of immune cells, including different subtypes of B cell type, T cell type, NK cells, plasma cells, and myeloid cells (27).
Biomarkers predicting immune response
The predictive performance of the potential immunotherapy response of CMS was evaluated with the following biomarkers: immune checkpoints, immunosuppressive factors, chemokines, and major histocompatibility complex (MHC) molecules. The bubble diagram suggested that CMS strongly correlated with molecules in each stage of the immune process (28).
Statistical analysis
The outcome of the BRCA-model was not ideal when the ROC curve was used to predict the model effect. This was likely due to the considerable heterogeneity of BRCA. We therefore divided TCGA-BRCA data into BASAL, HER2, LUMA, and LUMB types. The expression of CMS in each subtype was comprehensively analyzed. The prognosis analysis was carried out based on CMS, and the same method was used to construct the CMS prognostic model based on each subtype. Valuable results were obtained from the verification of the ROC curve in the random block. P<0.05 was considered statistically significant for all statistical methods.
Results
CMS panoramic analysis
We examined overall picture and prognostic significance of CMS genes in BRCA. The genes identified in this study included 13 CD28-B7 family members and 47 TNF family members. The co-expression of these molecules is shown in Figure 1. We found that there is a high correlation between CMS. Then, the matched clinical system information was combined with the expression data of 60 CMSs from 1,097 BRCA patients to calculate the prognosis information of CMS (Table 1). We then screened the CMSs that were specifically expressed in tumor tissues (tumor-specific CMSs). In tumor-specific CMSs, there were 10 genes with high-risk factors [hazard ratio (HR) >1] and 32 genes with low risk-factors (HR <1). In addition, we divided BRCA into 4 subtypes, BASAL, HER2, LUMA, and LUMB, according to molecular typing (PAM50) and then compared 60 CMSs with the expression levels of normal tissues (Figure 2). In the subsequent establishment of the prognostic model, CMSs with different subtypes and normal tissues were screened for candidate genes.
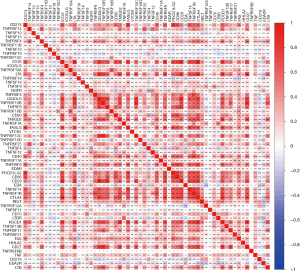
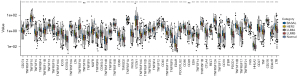
Identification of CMS for prediction
Based on the clinical information of 1,086 patients and the mRNA data of 42 tumor-specific genes, the optimal adjustment parameter λ was determined by the LASSO regression algorithm and cross-validation method, and the optimal variables were determined (TNFRSF14, TNFRSF19, TNFSF9, EDA, EDA2R, TNFSF14) to construct the prediction model (Figure 3A). Then, we used these parameter genes to develop a risk model based on the gene expression level for BRCA patients after several cycles of stepwise Cox regression analysis to predict the survival rate of patients using the following formula: model assessment risk score = (expression level of TNFRSF14 × −0.117170916 + expression level of TNFRSF19 × −0.135304566 + expression level of TNFSF9 × 0.255634182 + expression level of EDA2R × 0.372627814) (Figure 3B, Table 2). For clinical application, we detected the expression level data of these 4 indicators of patients with BRCA with the second-generation high-throughput sequencing technique. Patients’ risks can be calculated by the above model and patients can be divided into high-risk patients and low-risk patients according to the optimal cutpoint. The results of univariate and multivariate Cox analyses of the model score, age, gender, and clinical stage showed that the risk score of model assessment could not independently evaluate the prognostic risk level (Figure 3C,3D). The survival prediction model is given in the form of a nomogram (Figure 3E).
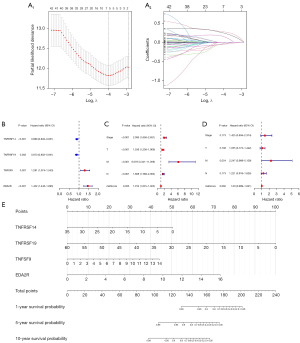
Table 2
ID | Coef | HR | HR.95L | HR.95H | P value |
---|---|---|---|---|---|
TNFRSF14 | −0.1171709 | 0.88943316 | 0.83176602 | 0.95109843 | 0.00061271 |
TNFRSF19 | −0.1353046 | 0.87344985 | 0.80216791 | 0.95106601 | 0.00183919 |
TNFSF9 | 0.25563418 | 1.29128027 | 1.07394771 | 1.55259396 | 0.00655425 |
EDA2R | 0.37262781 | 1.45154399 | 1.24302303 | 1.695045 | 2.48E–06 |
CMS, costimulatory molecule signature; TCGA, The Cancer Genome Atlas; BRCA, breast cancer; coef, coefficient; HR, hazard ratio; HR.95L, HR with low 95% CI; CI, confidence index; HR.95H, HR with high 95% CI; TNFRSF, TNF receptor superfamily; TNF, tumor necrosis factor; TNFSF, TNF ligand superfamily.
Next, we randomly divided the BRCA samples into a training group and a test group, and a high-risk group and a low-risk group according to the best cutoff point of the prediction model score. Patients in the high-risk group showed significantly worse prognoses (Figure 4A) (18). Further, we use ROC curve to verify and evaluate the prediction effect of the model (Figure 4B); area under the curve (AUC) training =0.665; AUC test =0.711; AUC >0.5 indicates that the prediction results of the model are reliable (19). In order to determine whether the model gene expression trend was the same as that for a random block, the model gene expression of the model was extracted to draw a heat map (Figure 4C). Patients in each group were ranked according to the risk score from low to high, with a higher risk score indicating a higher probability of death (Figure 4D).
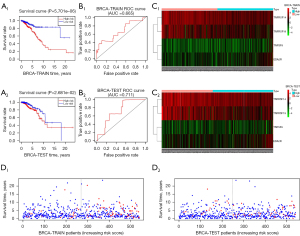
External dataset validation
GSE42568 was performed by GPL570 (Affymetrix Human Genome U133 Plus 2.0 array) including 104 BRCA tumor samples and 17 nontumor samples as normal controls. The consistent statistical method mentioned above was used for validation (Figure 5). Although the prognostic model we developed proved to be effective after verification, the actual effect was not as good as expected. Because of the pathological background, the results were attributed to the tumor heterogeneity of BRCA, so BRCA was grouped according to molecular typing (PAM50).
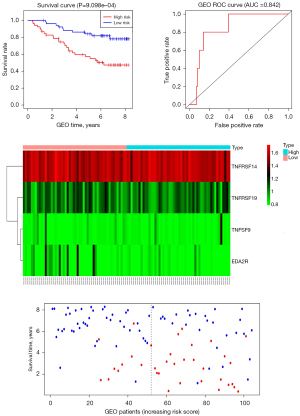
Construction and validation of the CMS in the 4 subtypes
According to the clinical data of TCGA-BRCA collected by UCSC Xena (https://xena.ucsc.edu/), 1,089 patients were divided into BASAL (n=139), HER2 (n=69), LUMA (n=415), and LUMB (n=185) subtypes. The CMS prognosis model of each subtype was established and verified using the same statistical method. Each subtype has a good predictive effect, which confirms the speculation that the heterogeneity of BRCA leads to a poor prognosis model. The results are shown in Figure 6. There were significant differences in the low-risk groups of the high-risk groups in each subtype, and they could be used as independent prognostic factors to evaluate the prognosis.
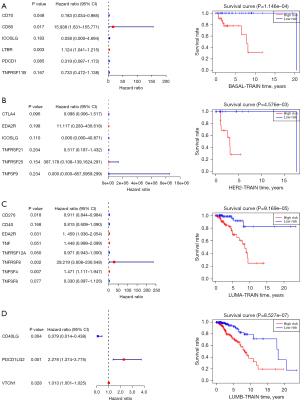
Biological processes and pathways related to CMS
The CMS prognostic models of each subtype [BASAL (n=139), HER2 (n=69), LUMA (n=415), and LUMB (n=185)] confirmed that CMS could clearly and effectively predict the prognosis, so we studied the biological mechanism further. According to the median of the model assessment risk score, the patients were divided into high-risk patients and low-risk patients. The differential analysis of the two groups of patients screened 75 differentially expressed genes and then selected these selected genes for GO and KEGG analysis. The results showed that the characteristic genes were concentrated in the redox reaction and the oxidoreductase activity acting on complement binding (Figure 7A). These results were furthered confirmed by KEGG analysis (Figure 7B).
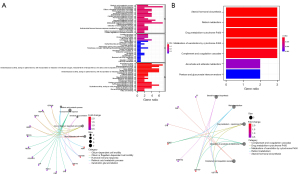
CMS-related immune cell infiltration and inflammatory activity
We used the combination of CIBERSORT and LM22 to calculate the estimated scores of different immune cells in the TME of BRCA (Figure 8A). According to the prognostic model score, the patients were divided into high-risk and low-risk patients. The results showed that B memory cells, activated dendritic cells (DCs) and M0 macrophages were highly infiltrated in high-risk patients, but this was not obvious. The ratio of CD8+ T cells and activated mast cells in low-risk patients is higher than that in high-risk patients (Figure 8B).
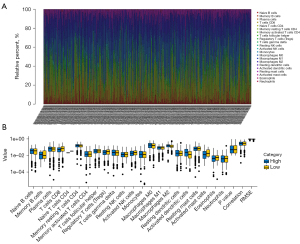
CMS and biomarkers for predicting immunotherapy response
The predictive performance of the immunotherapy response of CMS was evaluated by the following biomarkers: MHC molecules, immune-stimulating factors, immune checkpoints, immunosuppressive factors, and chemokines. The bubble diagram (Figure 9) suggested that CMS strongly correlated with the molecules in each stage of the immune process; that is, the model gene may in fact play a greater role in clinical application than indicated by the data.
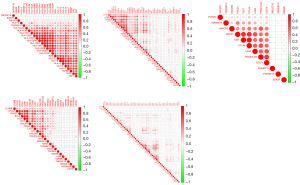
Immunotherapy response analysis of characteristic costimulatory factor EDA2R
Macroscopically, we found that the high-risk costimulatory factor EDA2R had adverse prognostic significance and appeared in many prediction models. BRCA-TCGA data were used for single-gene analysis, and we speculated that EDA2R had crucial biological significance based on the data results. The two groups of samples were evaluated with the Wilcoxon test. We used a forest map (Figure 10), with R version 4.2.1 software for statistical analysis, to show each variable [including HR, 95% confidence interval (CI), and P value]. Each subtype showed statistical significance (if not otherwise stated, the rank-sum test detected two sets of data, with a P value <0.05 being considered statistically significant). Nonetheless, the HR values were not the same, and it is indicated that the mechanism may be different in different cancers (29).
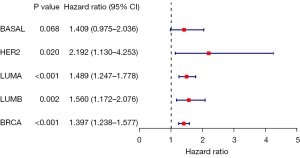
The expression and distribution of EDA2R in different subtypes of tumor tissues and normal tissues (Figure 11). The transverse axis represents different tumor tissues, the longitudinal axis represents the expression and distribution of the EDA2R gene, and different colors represent different groups. *P<0.05, **P<0.01, ***P<0.001, asterisks (*) stand for significance levels (30).
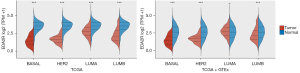
Verification of the immune correlation of EDA2R in various subtypes. Spearman correlation analysis hot map of EDA2R immune correlation and EDA2R gene expression in multiple tumor tissues (Figure 12A). The transverse axis represents different tumor tissues, the longitudinal axis represents different immune scores, and the different colors represent the correlation coefficient. The negative value represents negative correlation, and the positive value represents positive correlation; the stronger the correlation is, the deeper the color (31).
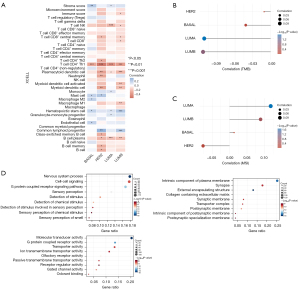
Mutation analysis of EDA2R and each subtype. Relationship with tumor mutation load: Spearman correlation analysis of tumoral mutational burden (TMB) and EDA2R gene expression (Figure 12B). The horizontal axis represents the correlation coefficient between the gene and TMB, and the ordinate is the different tumors. The dot size represents the correlation coefficient, and the different colors represent the P value; the bluer the color is, the smaller is the P value (32).
Relationship between EDA2R and microsatellite instability (MSI). Spearman correlation analysis of MSI and EDA2R gene expression (Figure 12C). The horizontal axis represents the correlation coefficient between the gene and TMB, and the ordinate is the different tumors. The dot size represents the correlation coefficient, and the different colors represent the P value the darker the color is, the smaller is the P value (33).
The gene expression data of EDA2R in TCGA-BRCA were selected, and BRCA patients were divided into high group and low groups according to the median expression data. Differentiated genes were obtained by difference analysis between the high and low groups. The functional enrichment analysis of these differential genes was performed (Figure 12D). Combined with the correlation of immune cells, we found that EDA2R was related to type 1 helper (Th1) CD4+ T cells and NKT cells in different degrees. We believe that EDA2R plays an important role in the immune signal mediation of these immune cells in BRCA immune microenvironment.
Drug sensitivity analysis of the CMS prognostic model
We predicted the chemotherapeutic response for each sample based on the largest publicly available pharmacogenomics database [the Genomics of Drug Sensitivity in Cancer (GDSC); https://www.cancerrxgene.org]. The prediction process was implemented by the R package “pRRophetic”. The samples’ half-maximal inhibitory concentration (IC50) was estimated by ridge regression. All parameters were set as the default values. Using the batch effect of combat and tissue type of all tissues, we summarized the duplicate gene expression as the mean value. As calculated by this model, we found that some drugs may be therapeutically effective in high-risk patients (IC50 high risk < IC50 low risk) (Figure 13).
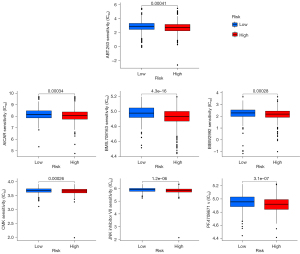
Benefits of ICIs treatment
We use the PD-L1 expression level to assess the potential impact of ICIs therapy on BRCA patients under our risk model. The results showed that the PD-L1 expression level of high-risk subgroup was lower than that of low-risk subgroup. This means that patients in low-risk group benefit more from ICIs treatment than patients in high-risk group (Figure 14).
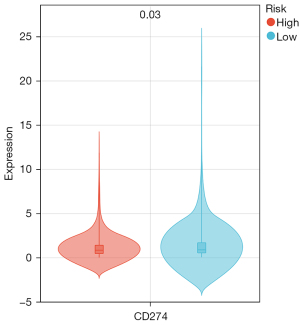
Discussion
A large body of evidence shows that physical and biochemical depletion of T cells is the main feature of an inhibitory TME (34). CMS plays an essential role in the killing of tumor by immune cells. In addition, PD-L1/PD-1 drugs have received good results in solid tumors, such as bladder cancer and lung cancer (35,36). CMS is mainly composed of the CD28-B7 and TNF families. In this study, the expression patterns of 60 CMSs in BRCA patients were detected. Based on the expression of CMS, we screened the expression data of tumor-specific CMSs in TCGA data and developed a new survival prediction model. However, the results were unsatisfactory in the TCGA-BRCA samples random validation. From the perspective of clinicopathology, this can be attributed to the substantial heterogeneity of BRCA. Subsequently, according to the clinical information provided by the UCSC, we divide the cases into 4 subtypes: BASAL, LUMA, LUMB, and HER2. According to the same statistical method and packages from R, the 4 subtypes were used to establish a predictive model with tumor-specific CMSs. We verified the model within the random group. The CMS prediction model risk score was an independent risk factor for BRCA subtypes. We also explored the immune landscape (including immune cell distribution and inflammatory activity) of CMS high-risk and low-risk patients. We found that CMS scores were positively correlated with different immunotherapy biomarkers. Our study is the first and most comprehensive study to date to describe the predictive value of CMS for prognosis and immunotherapy response in BRCA patients.
The results of the CMS model of each subtype of BRCA showed that TNFRSF14+, TNFRSF4+, TNFRSF19+, TNFRSF10A+, LTA+, TNFSF9+, TNFRSF8+, TNFSF4+, EDA+, TNFSF14+ T, NFRSF1B+, LTBR+, TNFSF13B+, TNFRSF17+, CD27+, EDA2R, and CD40LG all belonged to the TNF family. This indicates that costimulatory signals and pathways in the TNF family have greater prognostic value in BRCA patients than do those of the CD28-B7 family. Among them, EDA2R+, CD40LG+, PDCD1+, and TNFRSF14+ showed prognostic significance in the panoramic analysis of CMS and repeatedly appeared in the prediction models of each subtype. Their biological functional significance also warrants further attention.
EDA2R, also known as TNFRSF27, or XEDAR, has been studied previously, but its function and signal mechanism are still unclear. XEDAR can activate typical nuclear factor-κB (NF-κB) pathways and atypical NF-κB signaling pathways by direct interaction with TRAF3 and TRAF6 (37). Some researchers have found that EDA2R can reduce the inflammatory effect of cell injury through the NF-κB pathway (38). The atypical NF-κB signal induced by XEDAR involves NF-κB inducing kinase (NIK) and inhibitor of kappa B kinase α (IKKα) and is negatively regulated by TRAF3, cIAP, and A20 (39). It had also been reported that in most BRCAs, XEDAR expression is downregulated via promoter methylation blocking the XEDAR-mediated cell death pathway. Restoring XEDAR expression by demethylation therapy may be a novel approach for BRCA treatment (40). EDA2R, as a high-risk immunostimulatory factor, appeared in the single-factor Cox, BRCA, and LUMA prediction model. Its actual prognostic and therapeutic value in BRCA, has not been entirely clarified. This study provides data for directing the further study of EDA2R in LUMA.
CD40LG, also known as CD40L, TNFSF5, or CD154, is a membrane-bound protein belonging to the TNFSF family. It is mainly expressed after activating CD4+ T cells and binds to CD40 molecules on the surface of mature B cells. It assists in the maturation and activation of B cells and plays a vital role in immune response. A study suggests that the characteristics of CD40LG triggering Th1 immune response can be used as a new therapeutic target (41). It was found that P2Y12-dependent CD40L, which is dependent on platelet-releasing cells, can further activate the CD40 receptors on the surface of CD8+ T cells and inhibit tumor growth, thus providing a new therapeutic direction for platelets control liver tumor (42). It has been reported that the expression of the CD40LG-CD40 axis in lung cancer can significantly improve prognosis of lung cancer patients (43). Some cytokines can be secreted to enhance the immune response after binding with CD40 expressed by B cells and tumor cells. For example, after CD40LG combines with Hodgkin lymphoma cells, protumor inflammatory factors such as interleukin-8 (IL-8) and IL-6 are produced. DCs can produce more IL-12 than can CD40L or interferon-γ (IFN-γ) alone under the combined action of CD40L and IFN-γ (44). CD40LG, after binding, interacts with surviving c-MYC, hTERT, and other apoptosis-related genes to induce apoptosis (45). An in-depth study of the biochemical mechanism of CD40 ligand-induced tumor cell apoptosis may provide a new direction for clinical tumor treatment.
The inhibitory receptor on T cells activated by the PDCD1 antigen is the mechanism for organisms to avoid excessive immunity in evolution, which plays a crucial role in inducing and maintaining immune tolerance (46). Inhibitory signals are released when combined with CD274/PDCD1L1 and CD273/PDCD1LG2 (47). After TCR is involved, PDCD1 is associated with CD3-TCR in immune synapses and directly inhibits T cell activation (through similarity) (48). After ligand binding, PDCD1 is phosphorylated, and then PTPN11/SHP-2 is recruited to mediate the dephosphorylation of ZAP70, PRKCQ/PKCtheta, and CD247/CD3zeta, resulting in inhibition of T-cell activation (49). Long-term stimulation of tumor antigen leads to the continuous secretion of inflammatory factors, which can continuously increase the expression of PD-1 in T cells, leading to long-term activation of T cells and functional depletion (50). PDCD1 is highly expressed in the BASAL type, which is an important reason why BASAL has a more potent immune escape effect than do other subtypes of BRCA. As the treatment for PDCD1 locus in the BASAL subtype is unknown, we will further investigate it in future studies.
TNFRSF14 (also known as LIGHTER), TNFSF14 (LIGHT), LTA/lymphotoxin-α, immunoglobulin member BTLA, and CD160 constitute a complex stimulation inhibition signal network (51). TNFRSF14 acts as a ligand for BTLA in mucosa-associated lymphoid tissue, and its inactivation can release the activity of T cells and promote its killing of malignant B cells (52). TNFRSF14 can also promote the survival and differentiation of immune cells through the TRAF2-TRAF3 E3 ligase signaling pathway (53). The LIGHT expressed in DCs stimulates the activation and proliferation of T cells. In contrast, LIGHTER stimulates the NF-κB pathway in T cells and preferentially induces the expression of (54). Interaction with CD160 on NK cells enhances IFN-γ production and antitumor immune response (55). TNFRSF14 may induce apoptosis and inhibit the proliferation of bladder cancer cells in vitro (56). The mutation rate of TNFRSF14 in follicular lymphoma is as high as 39%. It is speculated that the lack of tumor suppressor function of TNFRSF14 leads to the formation of follicular lymphoma (57). In our study, TNFRSF14 was found to be a low-risk factor (HR =0.889<1). We speculate that TNFRSF14 also plays a tumor-suppressing role in BRCA, which may be useful for future BRCA treatment.
This study highlights that CMSs may predict the response of BRCA patients to therapy. Since immune checkpoint targets (PD-L1/PD-1, CD86/CTLA4) are CMSs, CMSs may have the ability to predict ICI immunotherapy response. Due to the lack of details about mRNA expression in the immune microenvironment, we must evaluate this relationship indirectly. We collected the TMB, MSI, and immune correlation. TMB is one of the classic biomarkers of immunotherapy response, and TMB always increases the load of new antigens. MSI is a predictive immunotherapy biomarker that is considered more accurate than TMB or PD-L1 expression. From the data of TMB and MSI, we found that the low-risk patients under our model may have a better response to immunotherapy, which is the same as the result of immunocytogram analysis.
In the later research, PD-L1, the best index of immunotherapy, was predicted to evaluate the guiding significance of this risk model for immunotherapy. We calculated the risk score of patients with TCGA-BRCA by the risk model in our study and divided them into high-risk group and low-risk group by median. At the same time, PD-L1 was extracted from the patient mRNA data. Through the comparison between the high-risk group and the low-risk group, we found that the expression level of PD-L1 in the low-risk group was higher, which shows that the low-risk patients calculated by this model will have a better effect on immunotherapy. This result is consistent with previous infiltration of immune cell: There is a higher degree of CD8+ T cell infiltration in low-risk patients. This result also means that low-risk patients have a more obvious effect on immunotherapy. In addition, in the previous discussion, we analyzed the key factor EDA2R, and found that EDA2R was negatively correlated with the Th1 CD4+ T cells, and Th1 CD4+ T cells mainly promoted tumor immunity, which further confirmed that the low-risk patients calculated by our risk model would gain more benefits from immunotherapy than those with high risk.
Generally speaking, we believe that the higher the TMB and MSI of a case are, the higher is the immunogenicity of the solid tumor in this case. The key genes in this model were negatively correlated with these indices in various subtypes, so we speculate that the low-risk patients calculated by this model algorithm have better response and receive greater effects from immunotherapy. In addition, we performed a drug sensitivity analysis for this prognostic model and found that 7 drugs (JNK inhibitor VIII, PF.4708671, CMK, BMS.708163, BIBW2992, AICAR, ABT.263) were sensitive and effective in high-risk patients. Comparing the model CMS with these different validated biomarkers, we can roughly speculate that different risk patients might be suitable for immunotherapy or combination therapy. These findings provide us with additional confidence in asserting that both the CMS score and the biological characteristics of the CMS itself may serve as novel predictive biomarkers for immunotherapy responses.
This study has some limitations. First, we only verified the reliability of the prognostic models at the data level. Secondly, the CMS-specific immune landscape was clarified by bioinformatics methods of RNA-seq data and thus might have been affected by data deletion errors. Thirdly, the mRNA expression data of patients after immunotherapy could not be obtained. CMS’s ability to predict immunotherapy effects could only be reflected indirectly. With the accumulation and enrichment of future research data, CMS’ significance and clinical application in the breast will increase.
Conclusions
In a word, we comprehensively analyzed the expression of costimulatory factors in BRCA patients, and established costimulatory factor-related models for different subtypes of BRCA to predict the prognosis of BRCA patients. We also generated a nomogram to predict the survival rate of patients. We analyzed the characteristics of immune cell infiltration, immunotherapy response index and chemosensitivity of BRCA patients with different risk levels. Finally, we summarize the results, and screen out the potential treatment methods for patients with different risk levels under the model.
Acknowledgments
The results of this study are based on the data from TCGA (https://www.cancer.gov/tcga) and UCSC Genome Database. We thank all the authors who provided the data for these databases.
Funding: This article was supported by the Science and Technology Major Project of Inner Mongolia, China (No. 2019GG083).
Footnote
Reporting Checklist: The authors have completed the TRIPOD reporting checklist. Available at https://atm.amegroups.com/article/view/10.21037/atm-22-6245/rc
Conflicts of Interest: All authors have completed the ICMJE uniform disclosure form (available at https://atm.amegroups.com/article/view/10.21037/atm-22-6245/coif). The authors have no conflicts of interest to declare.
Ethical Statement: The authors are accountable for all aspects of the work in ensuring that questions related to the accuracy or integrity of any part of the work are appropriately investigated and resolved. The study was conducted in accordance with the Declaration of Helsinki (as revised in 2013).
Open Access Statement: This is an Open Access article distributed in accordance with the Creative Commons Attribution-NonCommercial-NoDerivs 4.0 International License (CC BY-NC-ND 4.0), which permits the non-commercial replication and distribution of the article with the strict proviso that no changes or edits are made and the original work is properly cited (including links to both the formal publication through the relevant DOI and the license). See: https://creativecommons.org/licenses/by-nc-nd/4.0/.
References
- Bauer KR, Brown M, Cress RD, et al. Descriptive analysis of estrogen receptor (ER)-negative, progesterone receptor (PR)-negative, and HER2-negative invasive breast cancer, the so-called triple-negative phenotype: a population-based study from the California cancer Registry. Cancer 2007;109:1721-8. [Crossref] [PubMed]
- Keenan TE, Tolaney SM. Role of Immunotherapy in Triple-Negative Breast Cancer. J Natl Compr Canc Netw 2020;18:479-89. [Crossref] [PubMed]
- El Bairi K, Haynes HR, Blackley E, et al. The tale of TILs in breast cancer: A report from The International Immuno-Oncology Biomarker Working Group. NPJ Breast Cancer 2021;7:150. [Crossref] [PubMed]
- Henriques B, Mendes F, Martins D. Immunotherapy in Breast Cancer: When, How, and What Challenges? Biomedicines 2021;9:1687. [Crossref] [PubMed]
- Kwa MJ, Adams S. Checkpoint inhibitors in triple-negative breast cancer (TNBC): Where to go from here. Cancer 2018;124:2086-103. [Crossref] [PubMed]
- Nurieva R, Thomas S, Nguyen T, et al. T-cell tolerance or function is determined by combinatorial costimulatory signals. EMBO J 2006;25:2623-33. [Crossref] [PubMed]
- Singh S, Hassan D, Aldawsari HM, et al. Immune checkpoint inhibitors: a promising anticancer therapy. Drug Discov Today 2020;25:223-9. [Crossref] [PubMed]
- Davari K, Holland T, Prassmayer L, et al. Development of a CD8 co-receptor independent T-cell receptor specific for tumor-associated antigen MAGE-A4 for next generation T-cell-based immunotherapy. J Immunother Cancer 2021;9:e002035. [Crossref] [PubMed]
- Sun L, Kees T, Almeida AS, et al. Activating a collaborative innate-adaptive immune response to control metastasis. Cancer Cell 2021;39:1361-74.e9. [Crossref] [PubMed]
- Bluestone JA. New perspectives of CD28-B7-mediated T cell costimulation. Immunity 1995;2:555-9. [Crossref] [PubMed]
- Goronzy JJ, Weyand CM. T-cell co-stimulatory pathways in autoimmunity. Arthritis Res Ther 2008;10:S3. [Crossref] [PubMed]
- Sanmamed MF, Chen L. A Paradigm Shift in Cancer Immunotherapy: From Enhancement to Normalization. Cell 2019;176:677. [Crossref] [PubMed]
- Fife BT, Bluestone JA. Control of peripheral T-cell tolerance and autoimmunity via the CTLA-4 and PD-1 pathways. Immunol Rev 2008;224:166-82. [Crossref] [PubMed]
- Ward-Kavanagh LK, Lin WW, Šedý JR, et al. The TNF Receptor Superfamily in Co-stimulating and Co-inhibitory Responses. Immunity 2016;44:1005-19. [Crossref] [PubMed]
- Mohammadi A, Najafi S, Amini M, et al. The potential of B7-H6 as a therapeutic target in cancer immunotherapy. Life Sci 2022;304:120709. [Crossref] [PubMed]
- Ren X, Li Y, Nishimura C, et al. Crosstalk between the B7/CD28 and EGFR pathways: Mechanisms and therapeutic opportunities. Genes Dis 2022;9:1181-93. [Crossref] [PubMed]
- Janakiram M, Chinai JM, Zhao A, et al. HHLA2 and TMIGD2: new immunotherapeutic targets of the B7 and CD28 families. Oncoimmunology 2015;4:e1026534. [Crossref] [PubMed]
- Zhang C, Zhang Z, Li F, et al. Large-scale analysis reveals the specific clinical and immune features of B7-H3 in glioma. Oncoimmunology 2018;7:e1461304. [Crossref] [PubMed]
- Dostert C, Grusdat M, Letellier E, et al. The TNF Family of Ligands and Receptors: Communication Modules in the Immune System and Beyond. Physiol Rev 2019;99:115-60. [Crossref] [PubMed]
- Croft M, Benedict CA, Ware CF. Clinical targeting of the TNF and TNFR superfamilies. Nat Rev Drug Discov 2013;12:147-68. [Crossref] [PubMed]
- Schildberg FA, Klein SR, Freeman GJ, et al. Coinhibitory Pathways in the B7-CD28 Ligand-Receptor Family. Immunity 2016;44:955-72. [Crossref] [PubMed]
- Benhamouda N, Sam I, Epaillard N, et al. Plasma CD27, a Surrogate of the Intratumoral CD27-CD70 Interaction, Correlates with Immunotherapy Resistance in Renal Cell Carcinoma. Clin Cancer Res 2022;28:4983-94. [Crossref] [PubMed]
- Chen Z, Yang F, Liu H, et al. Identification of a nomogram based on an 8-lncRNA signature as a novel diagnostic biomarker for childhood acute lymphoblastic leukemia. Aging (Albany NY) 2021;13:15548-68. [Crossref] [PubMed]
- Parker JS, Mullins M, Cheang MC, et al. Supervised risk predictor of breast cancer based on intrinsic subtypes. J Clin Oncol 2009;27:1160-7. [Crossref] [PubMed]
- Zhang Z, Lin E, Zhuang H, et al. Construction of a novel gene-based model for prognosis prediction of clear cell renal cell carcinoma. Cancer Cell Int 2020;20:27. [Crossref] [PubMed]
- Brentnall AR, Cuzick J. Use of the concordance index for predictors of censored survival data. Stat Methods Med Res 2018;27:2359-73. [Crossref] [PubMed]
- Newman AM, Liu CL, Green MR, et al. Robust enumeration of cell subsets from tissue expression profiles. Nat Methods 2015;12:453-7. [Crossref] [PubMed]
- Jiang P, Gu S, Pan D, et al. Signatures of T cell dysfunction and exclusion predict cancer immunotherapy response. Nat Med 2018;24:1550-8. [Crossref] [PubMed]
- Izzi V, Davis MN, Naba A. Pan-Cancer Analysis of the Genomic Alterations and Mutations of the Matrisome. Cancers (Basel) 2020;12:2046. [Crossref] [PubMed]
- Frost FG, Cherukuri PF, Milanovich S, et al. Pan-cancer RNA-seq data stratifies tumours by some hallmarks of cancer. J Cell Mol Med 2020;24:418-30. [Crossref] [PubMed]
- Sturm G, Finotello F, Petitprez F, et al. Comprehensive evaluation of transcriptome-based cell-type quantification methods for immuno-oncology. Bioinformatics 2019;35:i436-45. [Crossref] [PubMed]
- Thorsson V, Gibbs DL, Brown SD, et al. The Immune Landscape of Cancer. Immunity 2018;48:812-30.e14. Erratum in: Immunity 2019;51:411-2. [Crossref] [PubMed]
- Zhang Q, Huang R, Hu H, et al. Integrative Analysis of Hypoxia-Associated Signature in Pan-Cancer. iScience 2020;23:101460. [Crossref] [PubMed]
- Hanahan D, Weinberg RA. Hallmarks of cancer: the next generation. Cell 2011;144:646-74. [Crossref] [PubMed]
- Turley SJ, Cremasco V, Astarita JL. Immunological hallmarks of stromal cells in the tumour microenvironment. Nat Rev Immunol 2015;15:669-82. [Crossref] [PubMed]
- Topalian SL, Taube JM, Pardoll DM. Neoadjuvant checkpoint blockade for cancer immunotherapy. Science 2020;367:eaax0182. [Crossref] [PubMed]
- Yan M, Wang LC, Hymowitz SG, et al. Two-amino acid molecular switch in an epithelial morphogen that regulates binding to two distinct receptors. Science 2000;290:523-7. [Crossref] [PubMed]
- Jia N, Jia Y, Yang F, et al. Knockdown of EDA2R alleviates hyperoxia-induced lung epithelial cell injury by inhibiting NF-κB pathway. Allergol Immunopathol (Madr) 2022;50:84-90. [Crossref] [PubMed]
- Mikkola ML. The Edar subfamily in hair and exocrine gland development. Adv Exp Med Biol 2011;691:23-33. [Crossref] [PubMed]
- Punj V, Matta H, Chaudhary PM. X-linked ectodermal dysplasia receptor is downregulated in breast cancer via promoter methylation. Clin Cancer Res 2010;16:1140-8. [Crossref] [PubMed]
- Xu W, Li Y, Yuan WW, et al. Membrane-Bound CD40L Promotes Senescence and Initiates Senescence-Associated Secretory Phenotype via NF-κB Activation in Lung Adenocarcinoma. Cell Physiol Biochem 2018;48:1793-803. [Crossref] [PubMed]
- Ma C, Fu Q, Diggs LP, et al. Platelets control liver tumor growth through P2Y12-dependent CD40L release in NAFLD. Cancer Cell 2022;40:986-98.e5. [Crossref] [PubMed]
- Mu CY, Qin PX, Qu QX, et al. Soluble CD40 in plasma and malignant pleural effusion with non-small cell lung cancer: A potential marker of prognosis. Chronic Dis Transl Med 2015;1:36-41. [PubMed]
- Zarnegar B, He JQ, Oganesyan G, et al. Unique CD40-mediated biological program in B cell activation requires both type 1 and type 2 NF-kappaB activation pathways. Proc Natl Acad Sci U S A 2004;101:8108-13. [Crossref] [PubMed]
- Mosca PJ, Hobeika AC, Clay TM, et al. A subset of human monocyte-derived dendritic cells expresses high levels of interleukin-12 in response to combined CD40 ligand and interferon-gamma treatment. Blood 2000;96:3499-504. [Crossref] [PubMed]
- Majidpoor J, Mortezaee K. The efficacy of PD-1/PD-L1 blockade in cold cancers and future perspectives. Clin Immunol 2021;226:108707. [Crossref] [PubMed]
- Xu Y, Wu Y, Zhang S, et al. A Tumor-Specific Super-Enhancer Drives Immune Evasion by Guiding Synchronous Expression of PD-L1 and PD-L2. Cell Rep 2019;29:3435-47.e4. Erratum in: Cell Rep 2020;32:108000. [Crossref] [PubMed]
- Fife BT, Pauken KE. The role of the PD-1 pathway in autoimmunity and peripheral tolerance. Ann N Y Acad Sci 2011;1217:45-59. [Crossref] [PubMed]
- Li J, Jie HB, Lei Y, et al. PD-1/SHP-2 inhibits Tc1/Th1 phenotypic responses and the activation of T cells in the tumor microenvironment. Cancer Res 2015;75:508-18. [Crossref] [PubMed]
- Pauken KE, Sammons MA, Odorizzi PM, et al. Epigenetic stability of exhausted T cells limits durability of reinvigoration by PD-1 blockade. Science 2016;354:1160-5. [Crossref] [PubMed]
- Mauri DN, Ebner R, Montgomery RI, et al. LIGHT, a new member of the TNF superfamily, and lymphotoxin alpha are ligands for herpesvirus entry mediator. Immunity 1998;8:21-30. [Crossref] [PubMed]
- Wu F, Watanabe N, Tzioni MM, et al. Thyroid MALT lymphoma: self-harm to gain potential T-cell help. Leukemia 2021;35:3497-508. [Crossref] [PubMed]
- Cheung TC, Oborne LM, Steinberg MW, et al. T cell intrinsic heterodimeric complexes between HVEM and BTLA determine receptivity to the surrounding microenvironment. J Immunol 2009;183:7286-96. [Crossref] [PubMed]
- Tamada K, Shimozaki K, Chapoval AI, et al. LIGHT, a TNF-like molecule, costimulates T cell proliferation and is required for dendritic cell-mediated allogeneic T cell response. J Immunol 2000;164:4105-10. [Crossref] [PubMed]
- Šedý JR, Bjordahl RL, Bekiaris V, et al. CD160 activation by herpesvirus entry mediator augments inflammatory cytokine production and cytolytic function by NK cells. J Immunol 2013;191:828-36. [Crossref] [PubMed]
- Zhu YD, Lu MY. Increased expression of TNFRSF14 indicates good prognosis and inhibits bladder cancer proliferation by promoting apoptosis. Mol Med Rep 2018;18:3403-10. [Crossref] [PubMed]
- Boice M, Salloum D, Mourcin F, et al. Loss of the HVEM Tumor Suppressor in Lymphoma and Restoration by Modified CAR-T Cells. Cell 2016;167:405-18.e13. [Crossref] [PubMed]
(English Language Editor: J. Gray)