The association between air pollutants and hospitalizations for asthma: an evidence from Chengdu, China
Highlight box
Key findings
• Significant associations between hospitalizations for asthma and air pollutants were found in some population groups, especially in specific age-, gender-, and season-groups.
What is known and what is new?
• Previous works found that SO2 and NO2 were significant risk factors for asthma hospitalization of the elderly. The associations appeared to be more evident in the cool seasons than in warm seasons.
• We observed that the elderly was more sensitive to CO. The effects of O3 were significant in the cold season, whereas SO2 impacted more significantly in the warm season particularly on females and adults.
What is the implication, and what should change now?
• Males are more sensitive to PM2.5 and PM10, whereas the elderly are more sensitive to CO. We should pay more attention to SO2 in the warm season and be more vigilant against harmful effects of O3 in the cold season.
Introduction
According to the global disease burden report released by the World Health Organization (WHO) in 2016, about 300 million people worldwide currently have asthma (the prevalence rate is about 1–18%). The increasing prevalence of asthma has caused substantial economic and health care burdens in most well-developed and developing countries. Asthma is a major public health issue in the USA, affecting over 23 million people (1). In China, the asthmatic population has reached 30 million (2). Data of the recent 20 years show that the prevalence of asthma in China is increasing at the rate of 20–50% every 10 years. By 2025, the asthmatic population is expected to increase by 100–150 million and be responsible for about 1 in 250 deaths per year.
The health effects of air pollution have been subject to intense study in recent years. Outdoor air contaminants consist of primary pollutants emitted directly into the atmosphere, such as sulphur dioxide (SO2), nitrogen dioxide (NO2), carbon monoxide (CO), and particulate matter (PM2.5, PM10), along with secondary pollutants that form in the air as a result of chemical reactions with other pollutants and gases such as ozone (O3), nitrogen oxide species, and some particles (3). Exposure to pollutants such as airborne particulate matter and O3 has been associated with increases in mortality and hospital admissions due to respiratory disease (4,5). Epidemiological and toxicological studies show that expose to allergens, viral respiratory infections, and air pollutants increases the risk of hospitalization in patients with asthma (6-8). Fewer than 1% of the 500 largest cities in China meet the air quality standards (AQS; e.g., PM10, 20 µg/m3 for annual average and 50 µg/m3 for daily average) recommended by the WHO, and 7 of these cities are ranked among the 10 most polluted cities in the world (9). The prevalence and risk factors of asthma in several metropolitan cities in China including Beijing, Chengdu, and Guangzhou have become comparable to those in other developed countries (10).
A recent study pointed out that the prevalence rate of asthma in children aged 0–14 years was 4.56% in Chengdu (11). The city is located in the Sichuan Basin, where the terrain varies greatly, the annual average wind speed is low, and the climate is humid. The location and climatic conditions make it difficult to diffuse pollutants. From China’s Air Quality Assessment Report (12), the average duration of air pollution in the city is 92.4 hours, the days of air pollution accounts for 88% of one year, including 9% of days of extreme air pollution. In addition, the concentration of air pollution in 2014 often exceeded the accepted level of AQS, (i.e., PM2.5 ≤75 µg/m3, PM10 ≤150 µg/m3, NO2 ≤80 µg/m3 and O3 ≤160 µg/m3. Hence, quantitatively investigating the effects of air pollutants on hospitalizations for asthma in this city is of great importance, not only for regulating patients’ activities when the outdoor air pollution levels are high, but also for hospital allocation and scheduling of resources in an effective and timely manner.
The effects of air pollution on asthma have aroused widespread concern since the outbreak of asthma in Japan in 1961 (13). Associations between air pollution and increases in morbidity and mortality in asthma have been observed in many studies in various parts of the world, including Los Angeles (14), Nashville (15), New York (16), Philadelphia (17), New Orleans (18), West Virginia (19), and Leiden (20), and so on. Medina et al. (21) found that the asthma visits for the elderly increased for the increase of PM10 concentration. Sunyer et al. (22) applied Poisson time-series regression models and meta-analysis techniques to study the combined association between air pollution and the number of emergency admissions for asthma in 4 European cities from 1986 to 1992. The results showed that the number of emergency patients aged 15–64 increased by 2.9% for every 50 µg/m3 increase in NO2 concentration. Szyszkowicz (23) applied a generalized linear mixed model to prove the hypothesis that emergency department (ED) visits for asthma are associated with exposure to O3. Cai et al. (24) applied an over-dispersed generalized additive model (GAM) to investigate the acute effect of air pollution on asthma hospitalization in Shanghai, China, and showed that asthma hospitalization was influenced more by SO2 and NO2. The associations appeared to be more evident in cool seasons than in warm seasons. Luo et al. (25) utilized generalized linear Poisson models to estimate the association of asthma hospital admission with air pollution levels, and used a Markov chain to explore the transition probabilities between high-admission states and low-admission states. They found that among PM2.5, PM10, NO2, and SO2, SO2 was a significant risk factor for asthma hospitalization among the elderly. This phenomenon appeared more evidently, especially in cold seasons for females. Halonen et al. (26) analyzed the associations between daily air pollution levels and ED visits with Poisson regression and penalized thin plate regression splines in the GAMs framework; the results showed that children were more sensitive to smaller particles and the elderly to larger particles, suggesting that the effects of air pollution on asthma patients differ by age group. Ko et al. (27) conducted a retrospective ecological study to investigate the effects of air pollution on asthma hospitalization rates in different age groups in Hong Kong, by constructing a GAM using a Poisson distribution with log-link function. They confirmed that children and the elderly were more likely to be hospitalized when exposed to air pollution. Woo et al. (28) built a deep learning algorithm to predict the deterioration of health symptoms among asthmatic children aged 8–12 years, based on peak expiratory flow rates (PEFR), indoor air pollution data, and meteorological data (PM2.5, temperature, and relative humidity). Sousa-Pinto et al. (29) assessed whether web-based searches on common cold (Google Trends) would correlate with and help to predict asthma hospitalizations. The results showed that common cold-related web-based searches display moderate-to-strong correlations with asthma hospitalizations and may be useful in forecasting them. Nadali et al. (30) used a generalized linear model (GLM) and distributed lag model (DLM) with the family of Poisson distribution to investigate the asthma hospitalization associated with air pollution.
However, previous studies have had several limitations. It has been uncovered that age, gender, and meteorological factors may have interactive effects on the exposure-effect relationship between air pollutants and human health (31). Some of the previous works either did not consider age, gender, and seasonality as potential risk factors of asthma hospitalization at the same time, or did not involve SO2, CO, NO2, O3, PM10, and PM2.5 as air pollutants and daily average/maximum/minimum temperature, relative humidity, and daily average wind speed as meteorological factors in a study. This study aimed to quantitatively investigate the effects of air pollutants on hospitalizations for asthma, by conducting age-, gender-, and season-specific analyses to achieve a systematic study. Based on our work, asthma intervention and corresponding healthcare resource allocation can be conducted in advance. We present the following article in accordance with the STROBE reporting checklist (available at https://atm.amegroups.com/article/view/10.21037/atm-22-6265/rc).
Methods
Data
Data of hospitalizations for asthma
The studied region of the Sichuan province (Chengdu) is a densely populated city situated in the southwestern China. The total population had reached 21.19 million by the end of 2021 with a population density of 1,478/km2. The city covered a total of 692 hospitals and 80,000 physicians, and 88.28% of the total population has participated in the basic medical insurance. Hence, almost all of the admission records can be searched in the medical insurance system. Approximately 12 million residents were covered from 1 January 2014 to 31 December 2014 (365 days). A total of 7,503 records of asthma hospitalizations for residents during this period were obtained from the Database of Medical Insurance Office. Patient code, age, gender, date of admission, main diagnosis code, and diagnosis description were involved covariates. According to the International Classification of Diseases, Revision 10 (ICD-10), diagnosis of asthma is coded as J45.001, J45.005, J45.901, and J45.903. The data was also classified by gender, age, and season. Warm season was defined as a period from April to September, and cold season was defined as the remaining period in a year. Patients were clustered into 3 age groups: ≤14 years, 14–65 years, and ≥65 years. The study was conducted in accordance with the Declaration of Helsinki (as revised in 2013).
Data of air pollution and meteorology
Daily (24 hours) air pollution concentration data including date, SO2, NO2, O3, particulates with an aerodynamic diameter of less than 10 µm particulate matter (PM10) and 2.5 µm (PM2.5) with unit µg/m3 and CO with unit mg/m3 from 1 January 2014 to 31 December 2014 in the studied region, were extracted from the website of the Environment Monitoring Center (EMC) database. To allow adjustment for the effects of weather on hospital admissions, meteorological data including date, daily average/maximum/minimum temperature (℃), relative humidity (%), and daily average wind speed (m/s) were collected from the website of the Meteorological Bureau.
Statistical analysis
In any given a day or week, only a small group of the population is admitted to hospitals. The number of admissions is a count; that is, it can only take on values limited to the non-negative integers. This indicates that a Poisson process is the underlying mechanism being modeled. In this setting, time-series analysis is often used to study health effects of the short-term fluctuation of air pollutant concentration. We used the date of admission as the specific incidence date of asthma for each patient, as the specific incidence date data cannot be captured in a real-life scenario. Air pollution levels and the number of daily hospitalizations for asthma were linked by date index and therefore could be analyzed in a time-series framework.
The association between hospitalizations for asthma and air pollution levels was evaluated by constructing a core model, which is a GAM using a Poisson distribution with log-link function. The nonparametric regression relaxes the usual assumption of linearity of GLM and enables more flexible data exploration, uncovering nonlinear structure in the data. The GAM regressed the daily count of asthma admissions on the time variable (day) and DOW. The smoothing functions of meteorological factors (daily average/maximum/minimum temperature, relative humidity, and daily average wind speed), seasonality, holiday indicator, and other time-based variables were incorporated in the GAM to adjust for the potential nonlinear confounding effects. Smoothing of the confounding effects was to control for the long-term seasonal patterns of hospitalizations for asthma and interactive effects of meteorological factors on the exposure-effect relationship between air pollutants and asthma, and natural cubic spline were chosen as smoothers. Each core model was identified based on the degree of freedom that derived the minimum Akaike’s Information Criterion (AIC) value. Finally, considering the AICs and the actual situation in China, what really affects the hospital admission of Chinese people is the Spring Festival and the year-end settlement of Chinese hospitals, not the ordinary holidays, so only these 2 factors were considered as holiday variables.
After establishing the core model, the average daily concentrations of PM2.5, PM10, CO, SO2, NO2, and O3 were then added with only 1 pollutant once into the core model to determine the relative risk (RR) of hospitalizations for asthma for a 10 µg/m3 (10 mg/m3 for CO) increase in each of these air pollutants. The concentrations of all the air pollutants for the same day (lag0) up to 7 lag days (lag7) and cumulative lags by 2 (lag01), and 3 (lag02) to 8 days (lag07) were tested in each model. Lag07 corresponds to 8-day moving average of pollutant concentration of the recent 8 days in RR analysis. The lag day (best lag) with the air pollutant concentration that yielded the largest RR value was chosen. We conducted age-, sex-, and season-specific analyses. The statistical significance of differences on air pollution effects on hospitalizations for asthma between subgroups were tested by calculating the 95% confidence interval (95% CI). Multi-pollutant models were constructed for air pollutants that were significant in the single-pollutant analysis, and the lag that had the strongest univariate effect was tested.
The correlation coefficients of the individual air pollutants and meteorological factors were examined. To address the collinearity between different air pollutants, only those air pollutants with correlation less than 0.7 were entered into the model. The null hypothesis of this test, H0, was that there were no differences of air pollution effects on hospitalizations for asthma between subgroups. Permutations of all air pollutants were performed in RR analysis by entering each pairwise of pollutants into the core model.
The results of all the statistical tests with two-sided P<0.05 were considered statistically significant. All models were fitted using R software (version 3.4.4; r Foundation for Statistical Computing, Vienna, Austria, http://cran.r-project.org/) with the mgcv package.
Results
Figure 1 provides a first view of the data and shows the time-series daily admission volume. The figure suggests a nonstationary fluctuation without obvious trend. It can be clearly found that there was a sharp decline in admissions at the end of the year, possibly resulting from the year-end settlement effect.
Table 1 and Table 2 summarize the basic statistics for our study. From 1 January 2014 to 31 December 2014, a total of 7,503 hospitalizations for asthma were recorded; on average, there were approximate 21 admissions per day in our study area. Among them, females accounted for about 60.55%. The share of the total population for the elderly (≥65 years old), adults (14–65 years old), and children (≤14 years old) was 21.14%, 71.12%, and 7.74%, respectively. The number of hospitalizations for asthma was higher in the warm season (21.02 per day), compared to that in the cold season (20.09 per day). During the study period, the average of daily concentrations was 72.7 µg/m3 for PM2.5, 117.2 µg/m3 for PM10, 18.1 µg/m3 for SO2, 1.1 mg/m3 for CO, 52.7 µg/m3 for NO2, and 82.8 µg/m3 for O3.
Table 1
Population groups | Number of patients |
---|---|
Total | 7,503 |
Male | 2,957 |
Female | 4,546 |
≥65 years old | 1,586 |
14–65 years old | 5,336 |
≤14 years old | 581 |
Warm | 3,846 |
Cold | 3,657 |
Cold season: from October to March; warm season: from April to September.
Table 2
Type | Indicator | Mean (SD) | Mina | P25a | P75a | IQRa | Maxa |
---|---|---|---|---|---|---|---|
Total population | 20.56 (6.52) | 2 | 16 | 25 | 9 | 44 | |
Male | 8.10 (3.51) | 1 | 6 | 10 | 4 | 24 | |
Female | 12.45 (4.65) | 1 | 9 | 15 | 6 | 32 | |
Daily admissions | ≥65 years old | 4.35 (2.33) | 0 | 3 | 6 | 3 | 13 |
14–65 years old | 14.62 (5.18) | 0 | 11 | 18 | 7 | 33 | |
≤14 years old | 1.59 (1.64) | 0 | 0 | 2 | 2 | 9 | |
Warmb | 21.02 (6.27) | 8 | 16 | 25 | 9 | 44 | |
Coldb | 20.09 (6.74) | 2 | 16 | 25 | 9 | 36 | |
PM2.5c | 72.70 (53.38) | 11 | 37 | 89.5 | 52.5 | 396 | |
PM10c | 117.15 (73.21) | 21 | 67 | 148.5 | 81.5 | 562 | |
Air pollutants | SO2c | 18.08 (9.90) | 4 | 11.5 | 21.5 | 10 | 61 |
COc | 1.095 (0.37) | 0.4 | 0.8 | 1 | 1.3 | 2.3 | |
NO2c | 52.68 (15.88) | 20 | 41 | 61 | 20 | 108 | |
O3c | 82.76 (43.38) | 15 | 47 | 112 | 65 | 249 | |
Meteorological measures | Mean.tempd | 18.02 (7.29) | 2 | 12 | 24 | 12 | 30 |
Min.tempd | 14.65 (7.09) | -1 | 9 | 21 | 12 | 26 | |
Max.tempd | 21.39 (7.83) | 4 | 15 | 28 | 13 | 37 | |
Relative.humd | 70.83 (9.44) | 30 | 65 | 78 | 13 | 92 | |
Wind.spd | 4.41 (2.02) | 0 | 3 | 5 | 2 | 15 |
a, Min: minimum; P25: 25th percentile; P75: 75th percentile; IQR: interquartile range (P75–P25); Max: maximum. b, cold season: from October to March; warm season: from April to September. c, PM2.5, PM10, SO2, NO2 and O3 with unit µg/m3, CO with unit mg/m3. d, mean.temp: mean temperature; min.temp: min temperature; Max.temp: max temperature with unit Celsius degree (℃); relative.hum: relative humid with unit %; wind.sp: wind speed with unit meter/second (m/s). CO, carbon monoxide; SO2, sulphur dioxide; NO2, nitrogen dioxide; O3, ozone; PM2.5 and PM10, particulates with an aerodynamic diameter of less than 2.5 and 10 µm particulate matter.
There are 6 levels of air quality. The higher the level, the more serious the air pollution. Levels 1 to 6 represent excellent, good, mildly polluted, moderately polluted, heavily polluted, and severely polluted, respectively. It can be seen from the top panel of Figure 2 that there were 129 days in the studied city that were mildly polluted or above (accounting for 36% of the whole year). Most of the time, air quality was good (accounting for 55% of the whole year), and only 35 days presented excellent air quality. The red lines in the bottom panel of Figure 2 represent the standard concentration of air pollutants according to China AQS. The concentrations of SO2 and CO did not exceed the standard. The concentrations of PM2.5 and PM10 were similar and their over-standard rates were 31.8% and 23.3%, respectively. The over-standard rates of NO2 and O3 were 6% and 4.9%, respectively.
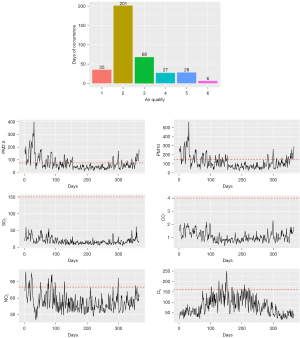
Generally, the correlation coefficients of the individual air pollutants and meteorological factors were examined. From Table 3, PM2.5, PM10, SO2, CO, and NO2 had moderately high correlation coefficients with each other. Particularly, a close correlation between PM10 and PM2.5 was observed, SO2 and NO2 had a strong correlation, and strong correlation relationships between CO and PM2.5, PM10, and NO2 were observed. Meanwhile, air pollutant concentrations showed a certain correlation with daily temperature measures (Mean temperature, Min temperature, and Max temperature). A high positive correlation existed between O3 and Max temperature, whereas the correlation between temperature measures with other air pollutants was significantly negative. These temperature measures also had significantly high correlation coefficients with each other. Relative humidity had no correlation with air pollutants and temperature measures. Wind speed showed a significantly negative correlation with PM2.5, PM10, SO2, CO, and NO2, indicating that concentrations of these 5 air pollutants may decrease with the wind speed. The higher the correlation between air pollutants and meteorological factors is, the stronger the interactive effects of meteorological factors have on the exposure-effect relationship between air pollutants and asthma.
Table 3
Air pollutants and meteorological measures | PM2.5 | PM10 | SO2 | CO | NO2 | O3 | Mean.temp | Min.temp | Max.temp | Relative.hum | Wind.sp |
---|---|---|---|---|---|---|---|---|---|---|---|
PM2.5 | 1 | ||||||||||
PM10 | 0.974** | 1 | |||||||||
SO2 | 0.637** | 0.676** | 1 | ||||||||
CO | 0.790** | 0.767** | 0.668** | 1** | |||||||
NO2 | 0.627** | 0.682** | 0.700** | 0.725** | 1 | ||||||
O3 | −0.177* | −0.174* | −0.212* | −0.310** | −0.092 | 1 | |||||
Mean.temp | −0.470** | −0.482** | −0.459** | −0.496** | −0.301** | 0.714** | 1 | ||||
Min.temp | −0.517** | −0.539** | −0.497** | −0.526** | −0.370** | 0.591** | 0.971** | 1 | |||
Max.temp | −0.407** | −0.409** | −0.400** | −0.442** | −0.224* | 0.790** | 0.977** | 0.901** | 1 | ||
Relative.hum | −0.010 | −0.115 | −0.220 | 0.144 | −0.154 | −0.437 | −0.024 | 0.106 | −0.14 | 1 | |
Wind.sp | −0.408** | −0.439** | −0.328* | −0.473** | −0.573** | 0.108 | 0.169 | 0.214 | 0.123 | −0.03 | 1 |
**, the significance level is 0.01; *, the significance level is 0.05. CO, carbon monoxide; SO2, sulphur dioxide; NO2, nitrogen dioxide; O3, ozone; PM2.5 and PM10, particulates with an aerodynamic diameter of less than 2.5 µm and 10 µm particulate matter.
Table 4 summarizes the results of the single-pollutant analysis for hospitalization due to asthma. An increase of 10 µg/m3 (10 mg/m3 for CO) of PM2.5, PM10, SO2, CO, NO2, and O3 was not associated with increased risks for asthma hospitalizations for all the air pollutants at a lag day ranging from 0 to 7 or cumulative lag days ranging from 01 to 07. The effects of these 6 air pollutants on the studied total population of hospitalizations due to asthma was not significant. Table 4 also shows the effects of air pollutants on the different age and gender groups. The increase in the concentrations of PM2.5 and PM10 had no significant effect on the female group, but the RR for hospitalization for every 10 µg/m3 increase in PM2.5 and PM10 for the male group were 1.0121 (95% CI: 1.0012–1.0232) and 1.0075 (95% CI: 1.0001–1.015), respectively. Both NO2 and O3 showed slightly protective effects on the female and male groups, with RR values of 0.9617 with cumulative lags 06 and 0.9872 with lag day 1, respectively. Only an increase in CO significantly increased the hospitalizations of the elderly patients, whereas only NO2 showed slightly protective effects on the elderly patients with a RR value 0.957 (95% CI: 0.9229–0.9922). The elderly (≥65 years old) tended to have a higher RR (1.0022, 95% CI: 1.0001–1.0043) for each 10 mg/m3 increase in CO than other age groups. All 6 pollutants had no significant effects on the group aged 14–65 years, whereas all these pollutants had slightly protective effects on the younger age group (≤14 years old), and the RR for hospitalization due to asthma for every 10 µg/m3 (10 mg/m3 for CO) increase in PM2.5, PM10, SO2, CO, NO2, and O3 were 0.954, 0.9692, 0.8715, 0.9959, 0.9177, and 0.938, respectively. The lag day (best lag) with the air pollutant concentration that yielded the largest RR value was also presented in Table 4.
Table 4
Air pollutants | Groups | Lag effects | Cumulative effects | |||
---|---|---|---|---|---|---|
Lag days | RR (95% CI) | Lag days | RR (95% CI) | |||
PM2.5 | All | – | NS | – | NS | |
Male | 3 | 1.0121 (1.0012, 1.0232)* | – | NS | ||
Female | – | NS | – | NS | ||
≥65 years old | – | NS | – | NS | ||
14–65 years old | – | NS | – | NS | ||
≤14 years old | 0 | 0.954 (0.9237, 0.9854)** | 00 | 0.954 (0.9237, 0.9854)** | ||
PM10 | All | – | NS | – | NS | |
Male | 4 | 1.0075 (1.0001, 1.015)* | – | NS | ||
Female | – | NS | – | NS | ||
≥65 years old | – | NS | – | NS | ||
14–65 years old | – | NS | – | NS | ||
≤14 years old | 0 | 0.9692 (0.9485, 0.9903)** | 00 | 0.9692 (0.9485, 0.9903)** | ||
SO2 | All | – | NS | – | NS | |
Male | – | NS | – | NS | ||
Female | – | NS | – | NS | ||
≥65 years old | – | NS | – | NS | ||
14–65 years old | – | NS | – | NS | ||
≤14 years old | 0 | 0.8715 (0.7688, 0.9878)* | 00 | 0.8715(0.7688, 0.9878)* | ||
CO | All | – | NS | – | NS | |
Male | – | NS | – | NS | ||
Female | – | NS | – | NS | ||
≥65 years old | – | NS | 01 | 1.0022 (1.0001, 1.0043)* | ||
14–65 years old | – | NS | – | NS | ||
≤14 years old | 7 | 0.9959 (0.9928, 0.999)** | – | NS | ||
NO2 | All | – | NS | – | NS | |
Male | – | NS | – | NS | ||
Female | 7 | 0.9789 (0.9584, 0.9998)* | 06 | 0.9617 (0.9263, 0.9984)* | ||
≥65 years old | 6 | 0.957(0.9229, 0.9922)* | – | NS | ||
14–65 years old | – | NS | – | NS | ||
≤14 years old | 0 | 0.9177 (0.8447, 0.9971)* | 00 | 0.9177 (0.8447, 0.9971)* | ||
O3 | All | – | NS | – | NS | |
Male | 1 | 0.9872 (0.9753, 0.9992)* | – | NS | ||
Female | – | NS | – | NS | ||
≥65 years old | – | NS | – | NS | ||
14–65 years old | – | NS | – | NS | ||
≤14 years old | 0 | 0.9544 (0.9257, 0.9839)** | 02 | 0.938 (0.9007, 0.9768)** |
** the significance level is 0.01; * the significance level is 0.05. NS, no significance. CO, carbon monoxide; SO2, sulphur dioxide; NO2, nitrogen dioxide; O3, ozone; PM2.5 and PM10, particulates with an aerodynamic diameter of less than 2.5 and 10 µm particulate matter.
The single-pollutant model’s estimates of season-specific effects for each pollutant are summarized in Table 5. In the cold season, the concentrations of PM2.5 and PM10 were associated with the higher hospitalizations for the elderly (RR 1.0226, 95% CI: 1.0023–1.0434) and the males (RR 1.0097, 95% CI: 1.0008–1.0186), respectively; an increase in O3 in the cold season would increase the hospitalizations of all the groups, except for that of the younger age group (≤14 years old); CO and NO2 played a slightly protective role on the most groups, and SO2 only impacted on the elderly group with a slightly protective effect. In the warm season, concentration increases in PM10 led to the increase of hospitalizations of males (RR 1.0193, 95% CI: 1.0038–1.035) and the adult age group (RR 1.0165, 95% CI: 1.0013–1.0318). Different from the cold season, SO2 had significant effects on all other groups, such as females (RR 1.1523, 95% CI: 1.0042–1.3222), the adult age group (RR 1.1739, 95% CI: 1.0349–1.3317), and the younger age group (RR 0.7318, 95% CI: 0.5547–0.9653). CO only impacted on males with an increased risk of hospitalization in the warm season. Both PM2.5 and O3 had a protective effect on the younger age group, whereas NO2 had no significant effect on all the specific groups. The cold season was associated with a higher risk of hospital admissions for asthma compared with the warm season, given the same 10 µg/m3 increase in the level of O3. Stronger associations were observed in the warm season for SO2.
Table 5
Season | Pollutant | All | Male | Female | ≥65 years old | 14–65 years old | ≤14 years old | |||||||||||
---|---|---|---|---|---|---|---|---|---|---|---|---|---|---|---|---|---|---|
Lag days | RR (95% CI) | Lag days | RR (95% CI) | Lag days | RR (95% CI) | Lag days | RR (95% CI) | Lag days | RR (95% CI) | Lag days | RR (95% CI) | |||||||
Warm | PM2.5 | – | NS | – | NS | 3 | 0.9787 (0.9603, 0.9974)* |
– | NS | – | NS | 0 | 0.8902 (0.8266, 0.9587)** |
|||||
PM10 | – | NS | 1 | 1.0193 (1.0038, 1.035)* |
3 | 0.9848 (0.9732, 0.9965)* |
– | NS | 1 | 1.0165 (1.0013, 1.0318)* |
0 | 0.9349 (0.8931, 0.9786)** |
||||||
SO2 | 2 | 1.1353 (1.019, 1.265)* |
– | NS | 2 | 1.1523 (1.0042, 1.3222)* |
– | NS | 2 | 1.1739 (1.0349, 1.3317)* |
0 | 0.7318 (0.5547, 0.9653)* |
||||||
CO | – | NS | 1 | 1.0038 (1.0013, 1.0063)** |
– | NS | – | NS | – | NS | – | NS | ||||||
NO2 | – | NS | – | NS | – | NS | – | NS | – | NS | – | NS | ||||||
O3 | 1 | 0.988 (0.9768, 0.9993)* |
1 | 0.9816 (0.9677, 0.9957)* |
– | NS | – | NS | – | NS | 1 | 0.9534 (0.911, 0.9977)* |
||||||
Cold | PM2.5 | – | NS | – | NS | – | NS | 3 | 1.0226 (1.0023, 1.0434)* |
– | NS | 6 | 0.966 (0.9334, 0.9998)* |
|||||
PM10 | – | NS | 4 | 1.0097 (1.0008, 1.0186)* |
– | NS | – | NS | – | NS | – | NS | ||||||
SO2 | – | NS | – | NS | – | NS | 6 | 0.9058 (0.843, 0.9733)* |
– | NS | – | NS | ||||||
CO | 6 | 0.9987 (0.9976, 0.9998)* |
7 | 0.996 (0.9926, 0.9994)* |
6 | 0.9984 (0.997, 0.9998)* |
6 | 0.9975 (0.9953, 0.9997)* |
– | NS | 7 | 0.995 (0.9913, 0.9988)* |
||||||
NO2 | 6 | 0.9727 (0.9494, 0.9965)* |
6 | 0.9202 (0.8608, 0.9838)* |
– | NS | 6 | 0.9501 (0.9092, 0.9927)* |
– | NS | – | NS | ||||||
O3 | 6 | 1.0438 (1.0063, 1.0826)* |
6 | 1.0327 (1.0076, 1.0584)* |
6 | 1.0221 (1.0001, 1.0447)* |
6 | 1.0438 (1.0063, 1.0826)* |
6 | 1.0276 (1.0066, 1.0489)** |
0 | 0.9428 (0.8895, 0.9993)* |
**, the significance level is 0.01; *, the significance level is 0.05. NS, no significance; CO, carbon monoxide; SO2, sulphur dioxide; NO2, nitrogen dioxide; O3, ozone; PM2.5 and PM10, particulates with an aerodynamic diameter of less than 2.5 µm and 10 µm particulate matter; rR, relative risk; CI, confidence interval.
The multi-pollutant model was conducted with the results shown in Table 6. As the levels of PM2.5, PM10, SO2, NO2, and CO were highly correlated (Table 3), only 1 of them was selected in the multi-pollutant model. In addition, as the negative effect of the 6 pollutants on females, adults, and the younger age group was very weak, even protective, the multi-pollutant models only considering the male and the elderly groups were conducted. From Table 4, PM2.5 were chosen into the multi-pollutant model with the male group as it had higher RR for asthma admissions when compared with PM10. O3 was also chosen in the model because its correlation with other 5 air pollutants was low.
Table 6
Multi-pollutant model | Male | ≥ 65 years old |
---|---|---|
The first pollutant | PM2.5 (lag1) | CO (lag01) |
1.1026 (1.004, 1.2109)* | 1.0098 (1.0005, 1.0191)* | |
The second pollutant | + O3 (lag1) | + O3 (lag6) |
0.8948 (0.8023, 1.0125)* | 1.0471 (0.973, 1.1268)* |
* the significance level is 0.05. CO, carbon monoxide; O3, ozone; PM2.5, particulates with an aerodynamic diameter of less than 2.5 µm particulate matter.
Discussion
Our study has shown that air pollutants have adverse effects on hospitalizations due to asthma, especially in specific age-, gender-, and season-group. Such effects could be found in males, females, the elderly, adults, and children, and the effects of different air pollutants on asthma admissions varies with different groups.
The strength of our study is that data we used for research were derived from the reliable institution of Medical Insurance Office, which captured the medical information of people who use medical insurance in the studied city. In addition, the corresponding air pollutants and meteorological data were synthetically considered in this study. Previous studies that assessed the effects of air pollutants on hospitalizations related asthma focused on the elderly group aged above 65 years old in the city (25), and the younger group aged below 18 years old (32). A study estimated the associations between ambient particulate matter pollution and hospital admissions from respiratory diseases and cardiovascular diseases, but unfortunately, it was difficult for us to understand the effects of air pollutants on asthma admissions alone from the merged data. Although many studies have performed similar investigations in other areas, the air pollutants or risk factors they considered were not comprehensive (24,33-35).
According to the classification standard of AQI, air quality is acceptable when the AQI reaches level 2, but some pollutants may have adverse effects on a very small number of abnormally sensitive people. When the AQI reaches level 3 or above, people with respiratory diseases may experience worsening symptoms. Asthma patients are extremely sensitive to air pollutants. However, there were only 35 days in 2014 that air quality was excellent, which is not good for asthma patients. The problem related to air pollution in the studied city is not optimistic. The sharp decline in hospitalizations at the end of the year was most likely due to the year-end settlement of Chinese hospitals. Many people will be discharged at the end of the year and hospitalized in time for the next year, as can be seen from the rebound in admissions on the first day of year 2014. In the course of modeling, we found that there was no significant difference in hospitalizations between general holidays and non-general holidays, but the impact of Spring Festival and the year-end settlement was worth noticing, which is a result with China-specific characteristics. Hence, we finally only chose the 2 periods as holiday variables.
As the GAM model was repeatedly tested and successfully constructed, we found that the effects of air pollution on hospitalizations of the total studied population was not significant. Such results were different from many previous studies and were contrary to our common sense. Wilson et al. (36) proposed that all studies they reviewed reported a significant relationship with at least one pollutant species. This may be partly influenced by publication bias, explicitly, the tendency for studies that yield positive results to be published, whereas those that determine no significant effects to remain “in the file drawer” (37). Considering that the time span of our data was not large enough, we continued our research by grouping patients. To observe whether the effects of air pollutants were consistent in the cold and warm seasons, we also divided the year into 2 seasons. Fortunately, significant associations were found in some specific groups and the fact that some air pollutants played different roles in different seasons was also found.
We observed that every 10 µg/m3 increase of PM2.5 and PM10 was significantly associated with increased hospitalizations for males. Besides, the adverse impact of PM10 on males existed in both cold and warm seasons. The results of multi-pollutant models also showed that PM2.5 had a positive association with hospitalizations of males. This means that male patients were susceptible to PM2.5 and PM10 in this study. A similar finding was reported in a study in Kalmar, Sweden, which noted that risk for asthma in men would increase when occupational exposure to air pollution from dust or smoke (similar to ambient particulate matter pollution), whereas no risk was seen in women (38). When patients were grouped by age, we noted that every 10 mg/m3 increase in CO significantly increased the hospitalizations of the elderly. The same result could also be seen in the result of multi-pollution model. Koskela (39) found a significant correlation between the incidence of angina pectoris and CO. In the study of Pan et al. (40), it was found that there was a significant correlation between the number of asthma outpatients and admissions and CO. As the function of heart and respiratory systems in the elderly is relatively weak when compared with the younger population, we can reasonably guess that the elderly are more sensitive to CO compared to the younger group. We noted that 6 pollutants have slight protective effects on the younger age group (≤14 years old), which was a rather strange result. This could be because children aged 4–14 years old usually attend school, mainly indoors. For children under 4 years old, when air pollution is serious, their parents usually take adequate protective measures (not going out or wearing masks).
In this study, O3 had more adverse effects in the cold season compared with the warm season on all age-based and gender-based groups (except for the younger age group), whereas SO2 impacted more significantly in the warm season particularly on females and adults. Sun Hong, doctor of Jiangsu Province Centers for Disease Control, and his team chose data from 9 cities in Jiangsu Province to study the impact of short-term ozone exposure on human health. On 9 November 2017, Hong said at the special report of “Environment and Health” in Nanjing, “Studies in other countries (41,42) have found that the adverse effects of ozone on human health were mainly in summer and warm season, but this does not match the reality of China. As the incidence of respiratory diseases often increases in autumn and winter and stimulation of ozone can easily cause discomfort in patients, the effects of ozone in China were significant in the cold season”. Similarly, they found that the effects of ozone were not significant throughout the year or in the warm season. In addition, a study in Harris County Texas observed no association between increased O3 levels and children diagnosed with asthma in colder months. Tramuto et al. (43) studied data from January 2004 to December 2007 in Palermo, Italy, and observed that SO2 demonstrated a clear adverse effect on emergency room admissions due to asthma in the warm season, yet no similar risk was seen in the cold season. Both CO and NO2 were observed to play a slightly protective role on the most groups in the cold season in our current study. Similarity, Tramuto et al. (43) also did not find adverse effects of CO and NO2 in the cold season. The protective effects we found in this study were very weak, so further study is needed in the near future.
Our study has some limitations. First, as in many other similar studies, our study could not assess precise exposure to 1 specific pollutant. The interaction effect among air pollutants was the difficulty we could not avoid. Secondly, despite the volume of data, the data we studied only covered 1 year. We deleted some insignificant confounding variables in the modeling process, and we found that air pollutants were not significant in the whole population. The reason that those variables or air pollutants were not significant may be that they are really not significant, or that the data was insufficient. Hence, we need more data to validate our results in the future. Also, we did not locate relevant literature to explain why the increase of air pollutant concentration had reduced the number of children admitted to hospital. We have only made some reasonable guesses.
Conclusions
In summary, except for NO2, the other 5 air pollutants were significantly associated with increased asthma hospitalizations of some specific groups in the studied city. Males were more sensitive to PM2.5 and PM10, whereas the elderly were more sensitive to CO. We should pay more attention to the harmful effects of SO2 in the warm season and be more proactive against the harmful effects of O3 in the cold season. As reducing respiratory diseases caused by air pollution could reduce the financial burden of governments and individuals, and improve the living quality of residents, measures to improve air quality are urgently needed. Our work, a fundamental study on asthma resource management, not only explores the relationship between air pollution and hospitalizations due to asthma, but also is of great significance in the implementation of asthma intervention and the allocation of corresponding healthcare resources. Besides, the findings in this study bear meaning for asthma patients and other residents to reduce the harm caused by air pollution.
Acknowledgments
Funding: This research was supported by National Natural Science Foundation of China (No. 71532007).
Footnote
Reporting Checklist: The authors have completed the STROBE reporting checklist. Available at https://atm.amegroups.com/article/view/10.21037/atm-22-6265/rc
Conflicts of Interest: All authors have completed the ICMJE uniform disclosure form (available at https://atm.amegroups.com/article/view/10.21037/atm-22-6265/coif). The authors have no conflicts of interest to declare.
Ethical Statement: The authors are accountable for all aspects of the work in ensuring that questions related to the accuracy or integrity of any part of the work are appropriately investigated and resolved. The study was conducted in accordance with the Declaration of Helsinki (as revised in 2013).
Open Access Statement: This is an Open Access article distributed in accordance with the Creative Commons Attribution-NonCommercial-NoDerivs 4.0 International License (CC BY-NC-ND 4.0), which permits the non-commercial replication and distribution of the article with the strict proviso that no changes or edits are made and the original work is properly cited (including links to both the formal publication through the relevant DOI and the license). See: https://creativecommons.org/licenses/by-nc-nd/4.0/.
References
- Estes TS. Moving towards effective chronic illness management: asthma as an exemplar. Chron Respir Dis 2011;8:163-70. [Crossref] [PubMed]
- Chen ZH, Wang PL, Shen HH. Asthma research in China: a five-year review. Respirology 2013;18:10-9. [Crossref] [PubMed]
- Holguin F. Traffic, outdoor air pollution, and asthma. Immunol Allergy Clin North Am 2008;28:577-88. viii-ix. [Crossref] [PubMed]
- Zanobetti A, Schwartz J, Dockery DW. Airborne particles are a risk factor for hospital admissions for heart and lung disease. Environ Health Perspect 2000;108:1071-7. [Crossref] [PubMed]
- Brunekreef B, Holgate ST. Air pollution and health. Lancet 2002;360:1233-42. [Crossref] [PubMed]
- Moghtaderi M, Aleyasin S, Amin R, et al. Skin test reactivity to fungal aeroallergens in asthmatic children in southern iran. Iran J Pediatr 2010;20:242-3. [PubMed]
- Matsumoto K, Inoue H. Viral infections in asthma and COPD. Respir Investig 2014;52:92-100. [Crossref] [PubMed]
- Gharehchahi E, Mahvi AH, Amini H, et al. Health impact assessment of air pollution in Shiraz, Iran: a two-part study. J Environ Health Sci Eng 2013;11:11. [Crossref] [PubMed]
- ADB. Toward an environmentally sustainable future country environmental analysis of the people’s republic of China, Adb Reports (2014). Available online: https://www.adb.org/zh/publications/toward-environmentally-sustainable-future-country-environmental-analysis-peoples.
- Chen YZNational Cooperation Group On Childhood Asthma. A nationwide survey in China on prevalence of asthma in urban children. Zhonghua Er Ke Za Zhi 2003;41:123-7. [PubMed]
- Li M, Zhang Q, Shi WJ, et al. Epidemiological survey and analysis of asthma in children aged 0-14 years old in urban and rural areas of Chengdu region. Transl Pediatr 2013;2:160-6. [PubMed]
- Available online: http://www.cnemc.cn/jcbg/kqzlzkbg/
- Phelps HW, Sobel GW, Fisher NE. Air pollution asthma among military personnel in Japan. JAMA 1961;175:990-3. [Crossref] [PubMed]
- Schoettlin CE, Landau E. Air pollution and asthmatic attacks in the Los Angeles area. Public Health Rep (1896) 1961;76:545-8. [Crossref] [PubMed]
- Landau E, Prindle RA, Ziedberg LD. The nashville air pollution study. Archives of Environmental Health An International Journal 1967;15:237-48. [Crossref] [PubMed]
- Greenburg L, Field F, Reed JI, et al. Asthma and temperature change. an epidemiological study of emergency clinic visits for asthma in three large New York hospitals. Arch Environ Health 1964;8:642-7. [Crossref] [PubMed]
- Girsh LS, Shubin E, Dick C, et al. A study on the epidemiology of asthma in children in Philadelphia: The relation of weather and air pollution to peak incidence of asthmatic attacks. Journal of Allergy 1967;39:347-57. [Crossref]
- Salvaggio J, Hasselblad V, Seabury J, et al. New Orleans asthma. II. Relationship of climatologic and seasonal factors to outbreaks. J Allergy 1970;45:257-65. [Crossref] [PubMed]
- Cohen AA, Bromberg S, Buechley RW, et al. Asthma and air pollution from a coal-fueled power plant. Am J Public Health 1972;62:1181-8. [Crossref] [PubMed]
- Tromp SW. Biometeorological analysis of the frequency and degree of asthma attacks in the western part of the Netherlands (period 1953-1959), Biometeorology (1962) 477–483. Available online: https://www.researchgate.net/publication/280993027_Biometeorological_analysis_of_the_frequency_and_degree_of_asthma_attacks_in_the_western_part_of_the_Netherlands_periods_1953-1959.
- Medina S, Le Tertre A, Quénel P, et al. Air pollution and doctors' house calls: results from the ERPURS system for monitoring the effects of air pollution on public health in Greater Paris, France, 1991-1995. Evaluation des Risques de la Pollution Urbaine pour la Santé. Environ Res 1997;75:73-84. [Crossref] [PubMed]
- Sunyer J, Spix C, Quénel P, et al. Urban air pollution and emergency admissions for asthma in four European cities: the APHEA Project. Thorax 1997;52:760-5. [Crossref] [PubMed]
- Szyszkowicz M. Ambient air pollution and daily emergency department visits for ischemic stroke in Edmonton, Canada. Int J Occup Med Environ Health 2008;21:295-300. [Crossref] [PubMed]
- Cai J, Zhao A, Zhao J, et al. Acute effects of air pollution on asthma hospitalization in Shanghai, China. Environ Pollut 2014;191:139-44. [Crossref] [PubMed]
- Luo L, Zhang F, Zhang W, et al. Markov Chain-Based Acute Effect Estimation of Air Pollution on Elder Asthma Hospitalization. J Healthc Eng 2017;2017:2463065. [Crossref] [PubMed]
- Halonen JI, Lanki T, Yli-Tuomi T, et al. Urban air pollution, and asthma and COPD hospital emergency room visits. Thorax 2008;63:635-41. [Crossref] [PubMed]
- Ko FW, Tam W, Wong TW, et al. Effects of air pollution on asthma hospitalization rates in different age groups in Hong Kong. Clin Exp Allergy 2007;37:1312-9. [Crossref] [PubMed]
- Woo J, Lee JH, Kim Y, et al. Forecasting the Effects of Real-Time Indoor PM2.5 on Peak Expiratory Flow Rates (PEFR) of Asthmatic Children in Korea: A Deep Learning Approach. IEEE Access 2022;10:19391-400.
- Sousa-Pinto B, Halonen JI, Antó A, et al. Prediction of Asthma Hospitalizations for the Common Cold Using Google Trends: Infodemiology Study. J Med Internet Res 2021;23:e27044. [Crossref] [PubMed]
- Nadali A, Leili M, Karami M, et al. The short-term association between air pollution and asthma hospitalization: a time-series analysis Air Qual Atmos Health 2022;15:1153-67. [Crossref]
- Peng RD, Dominici F, Pastor-Barriuso R, et al. Seasonal analyses of air pollution and mortality in 100 US cities. Am J Epidemiol 2005;161:585-94. [Crossref] [PubMed]
- Ding L, Zhu D, Peng D, et al. Air pollution and asthma attacks in children: A case-crossover analysis in the city of Chongqing, China. Environ Pollut 2017;220:348-53. [Crossref] [PubMed]
- Hsieh NH, Liao CM. Fluctuations in air pollution give risk warning signals of asthma hospitalization Atmospheric Environment 2013;75:206-216. [Crossref]
- Tian Y, Xiang X, Juan J, et al. Fine particulate air pollution and hospital visits for asthma in Beijing, China. Environ Pollut 2017;230:227-33. [Crossref] [PubMed]
- Delamater PL, Finley AO, Banerjee S. An analysis of asthma hospitalizations, air pollution, and weather conditions in Los Angeles County, California. Sci Total Environ 2012;425:110-8. [Crossref] [PubMed]
- Wilson AM, Salloway JC, Wake CP, et al. Air pollution and the demand for hospital services: a review. Environ Int 2004;30:1109-18. [Crossref] [PubMed]
- Scargle JD, Devincenzi D. Publication bias (the “file-drawer problem”) in scientific inference. Physics 1999;62:91-106.
- Graff P, Fredrikson M, Jönsson P, et al. Non-sensitising air pollution at workplaces and adult-onset asthma in the beginning of this millennium. Int Arch Occup Environ Health 2011;84:797-804. [Crossref] [PubMed]
- Koskela RS. Cardiovascular diseases among foundry workers exposed to carbon monoxide. Scand J Work Environ Health 1994;20:286-93. [Crossref] [PubMed]
- Pan HH, Chen CT, Sun HL, et al. Comparison of the effects of air pollution on outpatient and inpatient visits for asthma: a population-based study in Taiwan. PLoS One 2014;9:e96190. [Crossref] [PubMed]
- Schildcrout JS, Sheppard L, Lumley T, et al. Ambient air pollution and asthma exacerbations in children: an eight-city analysis. Am J Epidemiol 2006;164:505-17. [Crossref] [PubMed]
- Silverman RA, Ito K. Age-related association of fine particles and ozone with severe acute asthma in New York City. J Allergy Clin Immunol 2010;125:367-373.e5. [Crossref] [PubMed]
- Tramuto F, Cusimano R, Cerame G, et al. Urban air pollution and emergency room admissions for respiratory symptoms: a case-crossover study in Palermo, Italy. Environ Health 2011;10:31. [Crossref] [PubMed]
(English Language Editor: J. Jones)