Role and progress of artificial intelligence in radiodiagnosing vascular calcification: a narrative review
Introduction
Vascular calcification refers to the ectopic deposition and active remodeling of calcium salts in the arterial walls (1). It often occurs in 2 parts of the arterial walls, the intima and the media. The calcification of the intimal layer is patchy, associated with atherosclerosis (AS), whereas the calcification of the media layer is diffuse and concentric, related to diabetes mellitus (DM), peripheral artery disease (PAD), and chronic kidney disease (CKD) (2,3). For vascular calcification, as the accepted gold standard, radiology can provide simple and usually noninvasive diagnostic methods (4). However, there are increasing defects in this field. Studies have shown that radiologists need to interpret an image in an average of 3–4 seconds (5). Besides, more and more data are being produced by the continuously improving medical imaging technology (6). For instance, chest radiographs are reported to be performed more than 2 billion times worldwide every year (7). The limited human resources are being tasked with handling increasingly complex data within the specified time, resulting in not only a large number of diagnostic errors, but also a huge waste of resources and low work efficiency (7). These harsh facts make radiologists eager to seek tools for improvement. Fortunately, the development of artificial intelligence (AI) has opened up opportunities for radiology departments to address these issues (5).
Hitherto, based on the existing literature, AI has been applied to the diagnostic radiology of 5 types of vascular calcification: coronary artery calcification (CAC), thoracic aortic calcification (TAC), abdominal aortic calcification (AAC), carotid artery calcification, and breast artery calcification (BAC). Consequently, this review focused only on these types. In all, this review introduced the basic concept of AI, summarized the prognostic value of vascular calcification, analyzed the barriers in diagnostic radiology of vascular calcification without AI, narrated the application progress of AI, and put forward the prospect for the possible future direction. We present the following article in accordance with the Narrative Review reporting checklist (available at https://atm.amegroups.com/article/view/10.21037/atm-22-6333/rc).
Methods
Literature retrieval was conducted using the keywords “artificial intelligence”, “machine learning”, “deep learning”, and “vascular calcification” in the databases of PubMed and Web of Science. All papers were published between 2003 and 2022. After the computer automatically generated the search results, the researchers sorted out the literature according to the inclusion and exclusion criteria, focusing on the contents related to diagnostic radiology. When necessary, the researchers conducted a supplementary search for other relevant literature, such as clinical prognostic value of vascular calcification, difficulties faced by radiology department, and related concepts of AI. More details about the retrieval strategy are shown in Table 1.
Table 1
Items | Specification |
---|---|
Date of search | 1 July 2022 to 10 July 2022 |
Databases and other sources searched | PubMed and Web of Science |
Search terms used | “Artificial intelligence”, “machine learning”, “deep learning”, “vascular calcification” |
Timeframe | 2003–2022 |
Inclusion and exclusion criteria | Inclusion criteria: |
(I) The article was about the application of artificial intelligence in vascular calcification | |
(II) The article focused on radiology, including X-ray, CT, and MRI | |
(III) Literature type included review, research article and editorial | |
Exclusion criteria: | |
(I) The article had nothing to do with the application of artificial intelligence in vascular calcification | |
(II) The article focused on other fields, including drug and surgical treatment, mechanism research and other diagnostic tools such as ultrasound | |
Selection process | Two researchers searched the literature, and the third researcher arbitrated in case of differences |
CT, computed tomography; MRI, magnetic resonance imaging.
Discussion
Results of literature search
After searching PubMed and Web of Science, 62 studies published between 2007 and 2022 were included because they were related to the application of AI in diagnostic radiology of artificial intelligence. These studies included 5 types of vascular calcification: CAC, TAC, AAC, carotid artery calcification and BAC, as well as 4 types of radiologic methods: X-ray, invasive angiography, computed tomography (CT) and magnetic resonance imaging (MRI). The main methodology of these studies was to compare the diagnostic ability of human doctors and AI on the same radiological images by the use of sensitivity, specificity, accuracy and speed and other indicators.
Basic concept of AI
The concept of AI originated in 1955, defined as any computing program that imitates and expands human intelligence to perform tasks (6,8). It can automatically make decisions based on input data without manual intervention (9). AI is a very broad and blooming concept, which has different understandings and applications in different fields (10,11).
Machine learning (ML) was introduced as a subset of AI in 1959 (9). Compared with ordinary AI algorithms, ML algorithms need not be explicitly programed for specific tasks, which automatically form rules by learning manually annotated training sets instead (9,12). In the application of ML in the diagnostic radiology of vascular calcification, K-nearest neighbor, support vector machine, and random forest are the representatives of such algorithms (13-15).
Artificial neural network (ANN), which is also called deep learning (DL), is a type of ML inspired by the interconnection of neurons in the human brain (12,16). ANN is composed of interconnected layers, among which the input layers are used to receive input data, the hidden layers are used to extract data patterns, and the output layers are used to generate results (9,17). Compared with traditional ML, ANN can automatically discover features of input without manual annotation (16,17). Convolutional neural network (CNN) is added with filters on the basis of ANN (16,17). If a large number of images are used as input, ordinary ANN will be overloaded due to too much feature information contained in the images, but CNN can select features for learning through filters (16,17). Therefore, CNN is more suitable for medical image analysis. In addition to CNN, there are varieties of new neural networks, such as U-Net and ResNet, which have also been applied in the field of medical imaging because of their excellent performance in image processing (12,16,17). To more vividly show the relationship between different concepts, the related information is depicted in Figure 1.
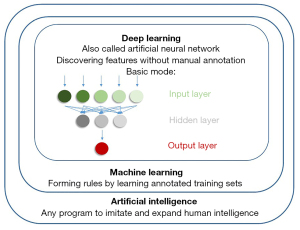
Significance of emphasis on vascular calcifications
Vascular calcification can occur anywhere in the body, having different prognostic value due to different anatomical positions (1). Table 2 briefly summarizes the prognostic value of each type of vascular calcification mentioned below.
Table 2
Type of vascular calcification | Major prognostic value | The most prominent value |
---|---|---|
Coronary artery calcification | Marker of coronary AS | A strong ability to predict CHD and reflect the cardiotoxicity in any population |
Improving risk prediction by FRS | ||
Strong independent predictors of CHD | ||
Predicting CHD in all age groups | ||
Reflecting cardiotoxicity of cancer treatment | ||
Thoracic artery calcification | Marker of increased risk of CHD | Ability to predict cardiovascular and cerebrovascular embolism events |
Higher risk of ischemic stroke | ||
Predicting cerebral infarction | ||
Identifying risk of embolism in surgery | ||
Abdominal artery calcification | Risk of obstructive CHD | Ability to predict pelvic and lower limb skeletal events |
Predicting asymptomatic CHD | ||
Marker of congestive heart failure | ||
Higher risk of skeletal events | ||
Carotid artery calcification | Marker of intracranial AS | Ability to predict cerebrovascular events in young people |
Higher risk of stroke | ||
Predicting cerebrovascular events for youth | ||
Breast artery calcification | Indication of the severity of CHD for women | Ability to predict cardiovascular events in women |
Related to CKD, DM, and bone health |
AS, atherosclerosis; FRS, Framingham risk score; CHD, coronary heart disease; CKD, chronic kidney disease; DM, diabetes mellitus.
CAC
The traditional prediction tool for coronary artery events is Framingham risk score (FRS), which uses traditional risk factors such as age, sex, and blood pressure (18). However, a large prospective study with a follow-up of 7 years reported that high-level coronary artery calcium scoring (CACS) diagnosed by CT could improve the risk prediction obtained only from FRS (18). The description of CACS is shown in Table 3. Another study with a follow-up of 7.6 years demonstrated that not only CACS had a good ability to predict risk, but also CAC volume and density scores were valuable (19). The CAC volume score was independently correlated with coronary heart disease (CHD) events, whereas the CAC density score showed an independent negative correlation with risk (19). It is generally recognized that the elderly are the main risk population of CHD events (19). However, a 12.5-year follow-up showed that young people aged between 32 and 46, even if the CACS score was very low, had an increased risk of CHD and death (7). By analyzing the conclusions of these follow-up studies with long time span, it becomes clear that both CACS and other information related to CAC have a strong ability to predict coronary events in almost all age groups.
Table 3
CACS | Risk classification |
---|---|
0 | Almost none |
1–10 | Low |
11–100 | Medium |
101–400 | Upper-middle |
>401 | High |
CACS, coronary artery calcium scoring.
TAC
A large sample study including 4 ethnicities found that TAC was widespread in the AS population (8). In addition, a study with a follow-up of about 5 years and a 28-year follow-up study of nearly 120,000 participants confirmed that TAC was associated with an increased risk of CHD and death, especially the aortic arch calcification (20,21). The latter study also showed that aortic arch calcification was related with an increased risk of ischemic stroke in women (21). This proof was reinforced by another study of 2,618 subjects, which found that TAC was an effective means to assess the risk of cerebral infarction (22). Moreover, TAC has other prognostic values. A study of patients with indications for cardiovascular surgery found that preoperative CT screening of TAC status was helpful to identify high-risk areas and reduce the risks of aortic embolism and stroke (23). Thus, TAC can not only predict cardiovascular events, but also provide prediction function for cerebrovascular events and perioperative period.
AAC
A study of 58 patients found that the AAC score determined by CT was correlated with the CACS, and the absence of AAC helped to exclude obstructive CHD and improve risk stratification (24). Besides, an analysis of 373 patients and another of lateral lumbar X-rays of 2,467 participants supplemented the value of the AAC score, indicating that the AAC score could be used as a surrogate marker for asymptomatic CHD and an independent risk factor for congestive heart failure (25,26). Prognostic value can also be provided for bone health by AAC. A study that included 2,662 healthy postmenopausal women found that AAC was independently associated with an increased risk of osteoporosis in the proximal femur (27). In addition, a study that included 5,994 65-year-old men confirmed that AAC was an effective indicator to identify the high risk of hip fracture in elderly men (28). Overall, AAC is a common predictor of cardiovascular outcomes and skeletal events.
Carotid artery calcification
Carotid artery calcification is a vital predictor of cerebrovascular events, which has been supported by a series of studies based on a 6-year cohort study of 2,323 patients (29). Carotid artery calcification, especially intracranial carotid artery calcification (ICAC), is an important marker of intracranial AS in various ethnicities worldwide and is closely related to stroke risk (29-31). Notably, a study involving nearly 2,000 patients found that ICAC and other vascular bed calcifications has a prominent difference, that is, ICAC has been widespread in very young individuals, indicating that carotid artery calcification can serve as a significant prognostic factor for cerebral vascular events in the young (32).
BAC
A study involving 213 patients showed that BAC is significantly correlated with CAC and the severity of CHD (33). A cohort study involving 12,239 women and a retrospective study involving 228 women also demonstrated that BAC is related to CKD, DM, bone mineral density, and so on (34,35). However, because BAC is considered unrelated to breast cancer, it has been widely underreported by radiologists (36,37). What is worse, young women have an insufficient understanding of cardiovascular diseases (38). In fact, cardiovascular disease is also one of the most dangerous threats to women’s health, related to their unique non-traditional risk factors which tend to be ignored, such as fertility, contraception, pregnancy complications, menopausal hormone therapy, systemic autoimmune diseases, and so on (38,39). Consequently, given that BAC is an easily available quantitative indicator that can reflect the cardiovascular risk of women, it should not go unheeded by radiologists (37).
Barriers in the absence of AI
A survey from 1999 to 2010 concluded that the volume of medical images is increasing, yet the utilization of images is not proportional, and radiologists need to interpret an image every 3–4 seconds within 8-hour working days (40). Another study based on multiple hospitals found that radiologists interacted with other medical staff an average of 6 times per hour, of which more than 81.2% was found to directly affect the health of patients (41). A study of physical condition of 40 radiologists before and after work found that after reading for 1 day, the diagnostic accuracy was significantly reduced, the attention was decreased, and the symptoms of oculomotor fatigue were increased (42). In general, it is reported that the retrospective error rate of radiological examination is as high as 30%, and the real-time error rate is 3–5%. Besides, 75% of medical malpractice claims related to radiologists link to diagnostic errors (43). These research results reveal the cruel situation of radiology that radiologists play an important role in the decision-making of patient intervention measures, but the increasingly heavy workload increases the diagnostic error rate, and the health of radiologists is also threatened.
The accurate determination of calcium score is reasonably demanding for the operators, to achieve which, each calcification within each coronary artery must be manually identified by the operator in turn (13). However, the shape of calcified foci is variable and subject to various disturbances. Therefore, calcium scoring is quite time-consuming and technically demanding (13,44,45). Meanwhile, specific calcium scores, such as CACS, need to be obtained through dedicated cardiac CT loaded with electrocardiogram (ECG) gating: calcium scoring computed tomography (CSCT) (46). The standard scanning parameters of CSCT include 120 kVp tube voltage and variable tube current according to the patients’ body type, equipped with a prospective ECG trigger device, obtaining images in the diastole period, and reconstructed in a slice thickness of 3 mm (9). Moreover, in CSCT, CAC is defined as a lesion with at least 3 consecutive pixels (or 1 mm2) and attenuation ≥130 Hounsfield unit (HU) (9). This means that if the CAC needs to be diagnosed accurately, a special examination must be performed, increasing the economic burden and radiation exposure, which will be reduced if an accurate diagnosis can be made by ordinary equipment (46). In addition, not in all scans, radiologists will evaluate the vascular calcification reflected in the images, resulting in a large number of missed diagnoses (44-47).
Generally, AI fairly needed for radiologists to diagnose vascular calcification. Fortunately, 5 types of vascular calcification have been assisted by AI (1,45,48-50). The anatomical location and radiologic equipment involved in AI of each type of vascular calcification is visualized in Figure 2.
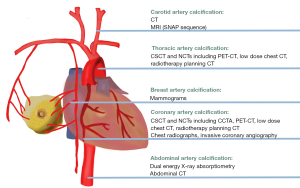
Application progress of AI
CAC
Automatic CACS in CSCT
AI was originally used to diagnose CAC in 2007 (13). A total of 64 features were designed for each candidate of CAC, after which, the K-nearest neighbor was applied to achieve an accuracy rate of 73.8% (13). In the next 3 years, researchers tried various methods to design features, including strength threshold, spatial features, and geometric features (13,51-53). Since it was always a technical problem to select features in the non-contrast images obtained by CSCT (15), in the following years, a more popular method was to register the high-precision coronary artery spatial information in coronary computed tomography angiography (CCTA) with the images from CSCT, which was called the atlas-based method (15,54-57) This idea made a breakthrough in the performance of ordinary ML. An algorithm using support vector machine achieved a sensitivity of 98.9% and a positive predictive value (PPV) of 94.8% (15). Similar ML algorithms were popular until 2016 (15); however, they had obstacles such as the cumbersome manual operation that was required (9).
In order to further improve efficiency, DL became the mainstream choice (5,16). Although the performance of DL was unsatisfactory at first, through continuous improvement of CNN by researchers, it was able to achieve hundreds of times faster efficiency, accurate and robust automatic scoring, and convenience of full automatic detection (58-64). When researchers use DL, the traditional idea is to determine CAC first and then obtain calcium scores (65). However, 1 study omitted the time-consuming step of determining CAC first, but directly regressed Agatston scores, completing the work in less than 0.3 seconds, with almost perfect consistency with manual results (65). U-Net, a more efficient algorithm, also known as fully CNN (FCN), is an extension of CNN to achieve more efficient learning in fewer training sets (66). The performance of DL based on U-Net architecture has been confirmed in a recent study, whose performance is very close to those of other algorithms in the same period (59). In detail, the U-Net proposed in this study correctly classified the risk classification of 86% of cases. In the remaining 14%, most of the errors were controlled in one class (59). Another study enabled the DL based on the U-Net to achieve the end-to-end and pixel-wise performance, that is, to directly input a complete image without losing pixel information (62).
Enabling more devices to diagnose CAC
Compared with CSCT, non-electrocardiogram-gating CTs (NCTs) are more common worldwide (67). If the CAC information obtained by NCT is reliable, it will reduce unnecessary CT examination, not only reducing economic expenses but also reducing radiation exposure (68). It has been shown that the use of NCTs including low-dose chest CT, CCTA, positron emission tomography (PET)/CT, and radiotherapy planning CT for calcium scoring is clinically significant and theoretically feasible (65,67-71). However, more noise and various artifacts make it almost impossible to obtain CAC manually and accurately using NCTs (55,71,72).
AI has played a great role in the task of diagnosing CAC based on NCTs. Ten years ago, an ordinary ML algorithm was reported able to achieve an accuracy of 82.2% in Agatston score in low-dose chest CT (71). In the past 5 years, the application of DL has promoted further development. Many researchers have been committed to noise and artifact reduction, as well as lessening the interference of non-calcified components such as stents on diagnosis, the DL algorithms developed by whom have improved the use value of low-quality images (67,72-74). In the latest study, DL improved the signal-to-noise ratio of low-dose CT by 27.7% (73), and the specificity of detecting CAC has been increased by 41% at most by means of removing blooming artifacts (74). Several other studies have also achieved noteworthy results. In order to solve the problem that the performance of network will be degraded if it is so deep due to a sea of information, a DL algorithm with ResNet architecture was adopted in a study, making complex work easier (68). Moreover, although the correct classification rate of scoring on NCTs in this work was only 70%, the correlation coefficient of the quantification of CAC volume between AI and manual results was as high as 0.923, indicating that quantification of CAC volume is an alternative when CACS are not accurate enough (68). Another study found that when detecting CAC in non-contrast chest CT, the sensitivity, specificity, and area under curve (AUC) of AI exceeded the manual results, suggesting that the performance of AI in NCTs can be better than that of human operators (47). Besides, a study validated the performance of AI in NCTs in 4 different centers, with high sensitivity and PPV in all datasets, further generalizing the achievement (75). In conclusion, the current performance of AI in diagnosing CAC based on NCTs is robust.
Notably, researchers have begun to expand the ability of AI to diagnose CAC in a wider range of radiological devices. Compared with NCTs, chest radiographs are more common (76). A study realized the classification of total calcium scores on chest radiographs using deep CNN, demonstrating the capability of chest radiographs to avoid CT examination in certain patients with regard to screening CAC (76). At length, for the detection of CAC in the study, the AUC reached 0.73 on the frontal radiographs and 0.7 on the lateral (76). In this first attempt in chest radiographs, even if the data was not so excellent, it was still valuable. In addition, a study proposed a deep neural network that enabled the analysis of invasive coronary angiography images within seconds, detecting CAC with a F1 score of 0.802 (77).
TAC
Compared with CAC, the accuracy of detecting TAC by CT cannot be significantly affected by cardiac motion (78) Some 12 years ago, researchers correctly detected 97.9% of TAC by using K-nearest neighbor algorithm combined with feature-based and atlas-based methods, which had a good correlation with manual results (78) In CT, TAC is able to be detected simultaneously during coronary artery examination (50). In recent years, the application of DL has enabled the concurrent quantification of CAC in multiple branches and TAC (62,70,79). A study extended the conclusion above to a variety of CTs including CSCT, low-dose chest CT, PET/CT, radiotherapy plan CT, and so on, with the ICC ranging from 0.68 to 0.98 (80). A recent study succeeded to achieve a time of only 4 seconds per patient with pixel-wise performance (62). It is worth noting that a study using 6 CNNs to detect TAC alone achieved a sensitivity of 98.4%, not only classifying the TAC in ascending aorta, aortic arch and descending aorta respectively, but also quantifying the TAC risk scores (50). Therefore, with the assistance of AI, automatic quantification of CAC and TAC in unison becoming the routine work of radiology department is no longer difficult.
AAC
With the continuous progress of DL, automatic detection of AAC has been realized, which has been supported by 2 studies in recent years (49,81). Dual energy X-ray absorptiometry (DXA) is a device supported by many guidelines as a strategy for fracture risk assessment, the increase in whose use has created opportunities for the application of AAC automatic scoring; however, due to its high technical requirement, DXA has not been used for routine detection of AAC in the clinic (49,82,83). Researchers have achieved satisfactory AAC scores classification using CNN based on DXA, attaining high consistency with manual results, that is, a Kappa score of 0.71 (49). In the future, the ability of CNN to automatically detect AAC based on DXA needs to be verified in more centers. Compared with DXA, CT shows obvious advantages in quantifying the mural calcium of the aorta (81). Researchers successfully realized the automatic detection of AAC using abdominal CT in a population involving more than 9,000 patients, attributing to Mask Region-CNN, a type of CNN accurate in feature extraction (81). On the basis of reliable quantitative results, this study also assessed the population distribution of AAC, increasing the value brought by the assistance of AI (81). To sum up, whether it is X-ray machine or CT, the automatic quantification of AAC can be realized by AI, and then becomes a routine work.
Carotid artery calcification
Both extracranial carotid artery calcification and ICAC can now be automatically detected by CT attributing to AI (44,48). The latest study used 4 DL networks with structures similar to U-Net, with the detection accuracy of ICAC even better than that of trained manual evaluation, with a sensitivity of 83.8% and PPV of 88% (44). Manual annotation of ICAC is time-consuming, error prone, and vulnerable to structures with similar attenuation and appearance, such as bone and dural calcification, so the better accuracy shown by DL is crucial (44).
The most significant difference between carotid artery calcification and other vascular calcification is the application of MRI (14,48). In the past reports, the accuracy of identifying calcification was low when only MRI was used (48). However, the application of simultaneous non-contrast angiography and intraplaque hemorrhage (SNAP) sequence has improved the recognition ability of calcification by MRI (14). The SNAP sequence first inverts all signals by an inversion pulse, and then obtains a highly T1 weighted inversion recovery acquisition and a proton density weighted reference acquisition by 2 gradient echo, having an excellent effect in cranial and cervical vascular imaging (84). Although SNAP succeeds in detecting calcification, it will be affected by motion artifacts and its scanning time is relatively long, which may be solved by Goal-SNAP and fast SNAP (14,85,86). In this study, ordinary ML algorithms such as random forest are similar to ANN regards to the detection of calcification, but DL may perform better in the segmentation of vascular components, which needs further research (14).
BAC
BAC is imaged by mammograms, but its manifestation in images is quite various, which can be bifurcated, overlapped, truncated, and of different intensity (36,45,66,87). Such complexity makes manual quantification of BAC difficult (38). However, more than 40 million women in the United States are examined by mammograms every year, not to mention globally, providing a huge database for the application of AI (45). In the early stage, researchers proposed the ML idea of random walk for the complex expression of BAC, converting the extraction of calcium into a vessel tracking problem (87). In recent years, DL has been widely used, the common idea of which is to cut out mammograms into patches, for the image size is too large for direct input (36,45,66). A 12-layer CNN describes the detection of BAC as a 2-classification problem (36). Although it succeeded in distinguishing whether BAC existed or not, it failed in quantitative evaluation (36). Moreover, it was quite slow due to the need to run separately for each patch (36,66). Subsequently, researchers improved CNN in view of these defects, proposing simple context U-Net (SCU-Net) and dense U-Net (DU-Net) (45,66). SCU-Net is a lightweight U-Net, which reduces the training amount by an order of magnitude on the basis of U-Net, overcoming the problem that BAC accounts for less than 1% of the images, leaving a sea of useless data to generate an army of invalid learning (45). DU-Net is also an improved way to avoid learning redundant features, greatly improving the efficiency of CNN, with an accuracy of 91.47% and sensitivity of 91.22% (66). To sum up, the DL used for automatic detection of BAC has reached an advanced level, but there is no reliable public data set to standardize this field, so relevant researchers need to make further contributions in the future (66).
Possible future directions
As reviewed above, AI has gradually reached the ability to assist radiologists in diagnosing vascular calcification by playing a role in the preliminary screening and speeding up work efficiency. However, it still inevitably has limitations and some areas for additional work remain.
The accuracy of AI for diagnostic radiology mainly associates with the algorithm of AI itself and the characteristics of images. From the conventional ML algorithms to the ever-expanding DL algorithms, the performance of AI itself has made great progress, and the diagnostic performance has improved accordingly (13,62). Over the years, with the progress of imaging technology, the image quality has been continuously upgraded, leading to the improvement of the diagnostic performance (40,84). In other words, AI’s ability is inseparable from high-quality training data, which can also benefit from the establishment of public databases or test platforms. Thus, in the future work, engineers and doctors should try to further promote the diagnostic performance of AI mainly from these two perspectives: AI algorithm itself and image quality. Radiology and AI are mutually reinforcing (1,6,9).
Notably, although AI has shown satisfactory effects in 5 types of vascular calcification, it has potential value for other types of vascular calcification, such as renal artery calcification (RAC), the prognostic value of which in hypertension and proteinuria has been supported (88). Meanwhile, the quantity of research in AAC and carotid artery calcification is low, which needs to be supplemented through future efforts.
Besides the excellent performance of AI reviewed above, AI can be utilized in other links of diagnostic radiology of vascular calcification. AI has been able to assist CT positioning, thus reducing errors caused by manual operation (5). Moreover, it can automatically create views, such as curved multiplanar reformations (CPR), assisting in the intelligent post-processing of images (5). Thus, in future work, AI should be a routine part of the radiology work, with the specific working mode described in Figure 3. Under this new mode, the working efficiency and accuracy of radiologists is hoped to be improved, with the rate of missed diagnosis and misdiagnosis of vascular calcification reduced. Especially for CAC and TAC able to be detected simultaneously, the screening efficiency is likely to be significantly improved. Moreover, AAC, carotid artery calcification and BAC, which are not indicators of routine clinical screening, may have chance to be well utilized as the burden on radiologists is not significantly increased.
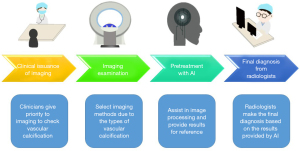
AI promotes the joint development of multiple disciplines, attributed to the automatic diagnosis of vascular calcification. Recently, the automatic quantification of CAC and TAC has been used to promote the mechanism research of cardiotoxicity caused by cancer treatment (89-91). In the future, the research efficiency of more cardiovascular diseases is bound to be improved.
Last but not least, the solution to the black box nature should also be a vital goal of future work, which means AI fails to interpret the logic of its output, and outputs based only on its learning from features (12). A solution may be better visualization techniques performed by DL, showing heat maps to help describe the principles (6,65). Moreover, AI’s such lack of the ability to use the emotional brain to conduct clinical analysis of calculation results makes it doom to be only an aid to radiologists, rather than replace them. Meanwhile, even though AI can surpass humans in speed and accuracy, it can never give patients the humanistic care that real doctors can give for now (6,10,11).
Conclusions
AI has performed brilliantly in the diagnostic radiology of vascular calcification. Besides improving accuracy and efficiency, the pixel-wise performance is more powerful than the naked eyes. The comparison of AI in the diagnostic radiology of each type of vascular calcification is shown in Table 4. In general, AI has achieved the ability to assist radiologists in automatically diagnosing vascular calcification, but there is still room for improvement. It is hoped that radiology can be better developed with the help of AI.
Table 4
Type of vascular calcification | Quantity of research | Whether X-ray is involved | Whether CT is involved | Whether MRI is involved | Latest application of DL |
---|---|---|---|---|---|
Coronary artery calcification | Great and sufficient | Yes | Yes | No | Pixel-wise and end-to-end DL together with GAN reduces noise and artifacts makes reliable automatic CACS based on CSCT and enables credible CAC quantification in NCTs and more radiological device |
Thoracic artery calcification | Medium | No | Yes | No | DL enables automatic TAC quantification together with CAC, as well as quantification in sections including the ascending aorta, aortic arch, and descending aorta |
Abdominal artery calcification | Few, needs more | Yes | Yes | No | More advanced DLs including Mask R-CNN enable accurate AAC quantification in both DXA and CT, with contributions to the population study of AAC |
Carotid artery calcification | Quite few, needs more | No | Yes | Yes | The performance of DL seems to be close to that of ML, but surpasses that of humans, with the first and only attempt to involve MRI |
Breast artery calcification | Medium | Yes | No | No | U-Net, SCU-Net, DU-Net and other advanced DLs overcome the complexity of manual BAC evaluation |
AI, artificial intelligence; CT, computed tomography; MRI, magnetic resonance imaging; DL, deep learning; GAN, generative adversarial network; CACS, coronary artery calcium scoring; CSCT, calcium scoring computed tomography; CAC, coronary artery calcification; NCTs, non-electrocardiogram-gating CTs; TAC, thoracic aortic calcification; Mask R-CNN, mask region-convolutional neural network; AAC, abdominal aortic calcification; DXA, dual energy X-ray absorptiometry; SCU-Net, simple context U-Net; DU-Net, dense U-Net.
Acknowledgments
Funding: This work was supported by the Key Research and Development Project of Jiangsu Province (No. BE2022780); the National Natural Science Foundation of China (No. 82070455); and Medical Innovation Team Project of Jiangsu Province (No. CXTDA2017010).
Footnote
Reporting Checklist: The authors have completed the Narrative Review reporting checklist. Available at https://atm.amegroups.com/article/view/10.21037/atm-22-6333/rc
Conflicts of Interest: All authors have completed the ICMJE uniform disclosure form (available at https://atm.amegroups.com/article/view/10.21037/atm-22-6333/coif). The authors have no conflicts of interest to declare.
Ethical Statement: The authors are accountable for all aspects of the work in ensuring that questions related to the accuracy or integrity of any part of the work are appropriately investigated and resolved.
Open Access Statement: This is an Open Access article distributed in accordance with the Creative Commons Attribution-NonCommercial-NoDerivs 4.0 International License (CC BY-NC-ND 4.0), which permits the non-commercial replication and distribution of the article with the strict proviso that no changes or edits are made and the original work is properly cited (including links to both the formal publication through the relevant DOI and the license). See: https://creativecommons.org/licenses/by-nc-nd/4.0/.
References
- Rogers MA, Aikawa E. Cardiovascular calcification: artificial intelligence and big data accelerate mechanistic discovery. Nat Rev Cardiol 2019;16:261-74. [Crossref] [PubMed]
- Desai MY, Cremer PC, Schoenhagen P. Thoracic Aortic Calcification: Diagnostic, Prognostic, and Management Considerations. JACC Cardiovasc Imaging 2018;11:1012-26. [Crossref] [PubMed]
- Rocha-Singh KJ, Zeller T, Jaff MR. Peripheral arterial calcification: prevalence, mechanism, detection, and clinical implications. Catheter Cardiovasc Interv 2014;83:E212-20. [Crossref] [PubMed]
- Mori H, Torii S, Kutyna M, et al. Coronary Artery Calcification and its Progression: What Does it Really Mean? JACC Cardiovasc Imaging 2018;11:127-42. [Crossref] [PubMed]
- Sharma P, Suehling M, Flohr T, et al. Artificial Intelligence in Diagnostic Imaging: Status Quo, Challenges, and Future Opportunities. J Thorac Imaging 2020;35:S11-6. [Crossref] [PubMed]
- van Assen M, Muscogiuri G, Caruso D, et al. Artificial intelligence in cardiac radiology. Radiol Med 2020;125:1186-99. [Crossref] [PubMed]
- Carr JJ, Jacobs DR Jr, Terry JG, et al. Association of Coronary Artery Calcium in Adults Aged 32 to 46 Years With Incident Coronary Heart Disease and Death. JAMA Cardiol 2017;2:391-9. [Crossref] [PubMed]
- Takasu J, Katz R, Nasir K, et al. Relationships of thoracic aortic wall calcification to cardiovascular risk factors: the Multi-Ethnic Study of Atherosclerosis (MESA). Am Heart J 2008;155:765-71. [Crossref] [PubMed]
- Lee H, Martin S, Burt JR, et al. Machine Learning and Coronary Artery Calcium Scoring. Curr Cardiol Rep 2020;22:90. [Crossref] [PubMed]
- Hamet P, Tremblay J. Artificial intelligence in medicine. Metabolism 2017;69S:S36-40. [Crossref] [PubMed]
- Topol EJ. High-performance medicine: the convergence of human and artificial intelligence. Nat Med 2019;25:44-56. [Crossref] [PubMed]
- Singh G, Al'Aref SJ, Van Assen M, et al. Machine learning in cardiac CT: Basic concepts and contemporary data. J Cardiovasc Comput Tomogr 2018;12:192-201. [Crossref] [PubMed]
- Isgum I, Rutten A, Prokop M, et al. Detection of coronary calcifications from computed tomography scans for automated risk assessment of coronary artery disease. Med Phys 2007;34:1450-61. [Crossref] [PubMed]
- Zhang Q, Qiao H, Dou J, et al. Plaque components segmentation in carotid artery on simultaneous non-contrast angiography and intraplaque hemorrhage imaging using machine learning. Magn Reson Imaging 2019;60:93-100. [Crossref] [PubMed]
- Yang G, Chen Y, Ning X, et al. Automatic coronary calcium scoring using noncontrast and contrast CT images. Med Phys 2016;43:2174. [Crossref] [PubMed]
- Jiang B, Guo N, Ge Y, et al. Development and application of artificial intelligence in cardiac imaging. Br J Radiol 2020;93:20190812. [Crossref] [PubMed]
- Mazurowski MA, Buda M, Saha A, et al. Deep learning in radiology: An overview of the concepts and a survey of the state of the art with focus on MRI. J Magn Reson Imaging 2019;49:939-54. [Crossref] [PubMed]
- Greenland P, LaBree L, Azen SP, et al. Coronary artery calcium score combined with Framingham score for risk prediction in asymptomatic individuals. JAMA 2004;291:210-5. [Crossref] [PubMed]
- Criqui MH, Denenberg JO, Ix JH, et al. Calcium density of coronary artery plaque and risk of incident cardiovascular events. JAMA 2014;311:271-8. [Crossref] [PubMed]
- Eisen A, Tenenbaum A, Koren-Morag N, et al. Calcification of the thoracic aorta as detected by spiral computed tomography among stable angina pectoris patients: association with cardiovascular events and death. Circulation 2008;118:1328-34. [Crossref] [PubMed]
- Iribarren C, Sidney S, Sternfeld B, et al. Calcification of the aortic arch: risk factors and association with coronary heart disease, stroke, and peripheral vascular disease. JAMA 2000;283:2810-5. [Crossref] [PubMed]
- Itani Y, Watanabe S, Masuda Y. Relationship between aortic calcification and stroke in a mass screening program using a mobile helical computed tomography unit. Circ J 2006;70:733-6. [Crossref] [PubMed]
- Lee R, Matsutani N, Polimenakos AC, et al. Preoperative noncontrast chest computed tomography identifies potential aortic emboli. Ann Thorac Surg 2007;84:38-41; discussion 42. [Crossref] [PubMed]
- Zweig BM, Sheth M, Simpson S, et al. Association of abdominal aortic calcium with coronary artery calcium and obstructive coronary artery disease: a pilot study. Int J Cardiovasc Imaging 2012;28:399-404. [Crossref] [PubMed]
- An C, Lee HJ, Lee HS, et al. CT-based abdominal aortic calcification score as a surrogate marker for predicting the presence of asymptomatic coronary artery disease. Eur Radiol 2014;24:2491-8. [Crossref] [PubMed]
- Walsh CR, Cupples LA, Levy D, et al. Abdominal aortic calcific deposits are associated with increased risk for congestive heart failure: the Framingham Heart Study. Am Heart J 2002;144:733-9. [Crossref] [PubMed]
- Bagger YZ, Tankó LB, Alexandersen P, et al. Radiographic measure of aorta calcification is a site-specific predictor of bone loss and fracture risk at the hip. J Intern Med 2006;259:598-605. [Crossref] [PubMed]
- Szulc P, Blackwell T, Schousboe JT, et al. High hip fracture risk in men with severe aortic calcification: MrOS study. J Bone Miner Res 2014;29:968-75. [Crossref] [PubMed]
- Bos D, Portegies ML, van der Lugt A, et al. Intracranial carotid artery atherosclerosis and the risk of stroke in whites: the Rotterdam Study. JAMA Neurol 2014;71:405-11. [Crossref] [PubMed]
- Kim JT, Yoo SH, Kwon JH, et al. Subtyping of ischemic stroke based on vascular imaging: analysis of 1,167 acute, consecutive patients. J Clin Neurol 2006;2:225-30. [Crossref] [PubMed]
- Wong LK. Global burden of intracranial atherosclerosis. Int J Stroke 2006;1:158-9. [Crossref] [PubMed]
- Kockelkoren R, De Vis JB, de Jong PA, et al. Intracranial Carotid Artery Calcification From Infancy to Old Age. J Am Coll Cardiol 2018;72:582-4. [Crossref] [PubMed]
- Huang Z, Xiao J, Xie Y, et al. The correlation of deep learning-based CAD-RADS evaluated by coronary computed tomography angiography with breast arterial calcification on mammography. Sci Rep 2020;10:11532. [Crossref] [PubMed]
- Kemmeren JM, Beijerinck D, van Noord PA, et al. Breast arterial calcifications: association with diabetes mellitus and cardiovascular mortality. Work in progress. Radiology 1996;201:75-8. [Crossref] [PubMed]
- Reddy J, Bilezikian JP, Smith SJ, et al. Reduced bone mineral density is associated with breast arterial calcification. J Clin Endocrinol Metab 2008;93:208-11. [Crossref] [PubMed]
- Wang J, Ding H, Bidgoli FA, et al. Detecting Cardiovascular Disease from Mammograms With Deep Learning. IEEE Trans Med Imaging 2017;36:1172-81. [Crossref] [PubMed]
- Molloi S, Mehraien T, Iribarren C, et al. Reproducibility of breast arterial calcium mass quantification using digital mammography. Acad Radiol 2009;16:275-82. [Crossref] [PubMed]
- Trimboli RM, Codari M, Guazzi M, et al. Screening mammography beyond breast cancer: breast arterial calcifications as a sex-specific biomarker of cardiovascular risk. Eur J Radiol 2019;119:108636. [Crossref] [PubMed]
- Wenger NK. Transforming cardiovascular disease prevention in women: time for the Pygmalion construct to end. Cardiology 2015;130:62-8. [Crossref] [PubMed]
- McDonald RJ, Schwartz KM, Eckel LJ, et al. The effects of changes in utilization and technological advancements of cross-sectional imaging on radiologist workload. Acad Radiol 2015;22:1191-8. [Crossref] [PubMed]
- Dhanoa D, Dhesi TS, Burton KR, et al. The evolving role of the radiologist: the Vancouver workload utilization evaluation study. J Am Coll Radiol 2013;10:764-9. [Crossref] [PubMed]
- Krupinski EA, Berbaum KS, Caldwell RT, et al. Long radiology workdays reduce detection and accommodation accuracy. J Am Coll Radiol 2010;7:698-704. [Crossref] [PubMed]
- Lee CS, Nagy PG, Weaver SJ, et al. Cognitive and system factors contributing to diagnostic errors in radiology. AJR Am J Roentgenol 2013;201:611-7. [Crossref] [PubMed]
- Bortsova G, Bos D, Dubost F, et al. Automated Segmentation and Volume Measurement of Intracranial Internal Carotid Artery Calcification at Noncontrast CT. Radiol Artif Intell 2021;3:e200226. [Crossref] [PubMed]
- Guo X, O'Neill WC, Vey B, et al. SCU-Net: A deep learning method for segmentation and quantification of breast arterial calcifications on mammograms. Med Phys 2021;48:5851-61. [Crossref] [PubMed]
- Waltz J, Kocher M, Kahn J, et al. The Future of Concurrent Automated Coronary Artery Calcium Scoring on Screening Low-Dose Computed Tomography. Cureus 2020;12:e8574. [Crossref] [PubMed]
- Yacoub B, Kabakus IM, Schoepf UJ, et al. Performance of an Artificial Intelligence-Based Platform Against Clinical Radiology Reports for the Evaluation of Noncontrast Chest CT. Acad Radiol 2022;29:S108-17. [Crossref] [PubMed]
- van Engelen A, Niessen WJ, Klein S, et al. Atherosclerotic plaque component segmentation in combined carotid MRI and CTA data incorporating class label uncertainty. PLoS One 2014;9:e94840. [Crossref] [PubMed]
- Reid S, Schousboe JT, Kimelman D, et al. Machine learning for automated abdominal aortic calcification scoring of DXA vertebral fracture assessment images: A pilot study. Bone 2021;148:115943. [Crossref] [PubMed]
- Guilenea FN, Casciaro ME, Pascaner AF, et al. Thoracic Aorta Calcium Detection and Quantification Using Convolutional Neural Networks in a Large Cohort of Intermediate-Risk Patients. Tomography 2021;7:636-49. [Crossref] [PubMed]
- Kurkure U, Chittajallu DR, Brunner G, et al. A supervised classification-based method for coronary calcium detection in non-contrast CT. Int J Cardiovasc Imaging 2010;26:817-28. [Crossref] [PubMed]
- Brunner G, Chittajallu DR, Kurkure U, et al. Toward the automatic detection of coronary artery calcification in non-contrast computed tomography data. Int J Cardiovasc Imaging 2010;26:829-38. [Crossref] [PubMed]
- Brunner G, Kurkure U, Chittajallu DR, et al. Toward unsupervised classification of calcified arterial lesions. Med Image Comput Comput Assist Interv 2008;11:144-52.
- Takx RA, de Jong PA, Leiner T, et al. Automated coronary artery calcification scoring in non-gated chest CT: agreement and reliability. PLoS One 2014;9:e91239. [Crossref] [PubMed]
- Wolterink JM, Leiner T, Takx RA, et al. Automatic Coronary Calcium Scoring in Non-Contrast-Enhanced ECG-Triggered Cardiac CT With Ambiguity Detection. IEEE Trans Med Imaging 2015;34:1867-78. [Crossref] [PubMed]
- Saur SC, Alkadhi H, Desbiolles L, et al. Automatic detection of calcified coronary plaques in computed tomography data sets. Med Image Comput Comput Assist Interv 2008;11:170-7.
- Shahzad R, van Walsum T, Schaap M, et al. Vessel specific coronary artery calcium scoring: an automatic system. Acad Radiol 2013;20:1-9. [Crossref] [PubMed]
- Cano-Espinosa C, González G, Washko GR, et al. Automated Agatston Score Computation in non-ECG Gated CT Scans Using Deep Learning. Proc SPIE Int Soc Opt Eng 2018;10574:105742K.
- Gogin N, Viti M, Nicodème L, et al. Automatic coronary artery calcium scoring from unenhanced-ECG-gated CT using deep learning. Diagn Interv Imaging 2021;102:683-90. [Crossref] [PubMed]
- Wolterink JM, Leiner T, de Vos BD, et al. Automatic coronary artery calcium scoring in cardiac CT angiography using paired convolutional neural networks. Med Image Anal 2016;34:123-36. [Crossref] [PubMed]
- Wang W, Wang H, Chen Q, et al. Coronary artery calcium score quantification using a deep-learning algorithm. Clin Radiol 2020;75:237.e11-6. [Crossref] [PubMed]
- Singh G, Al'Aref SJ, Lee BC, et al. End-to-End, Pixel-Wise Vessel-Specific Coronary and Aortic Calcium Detection and Scoring Using Deep Learning. Diagnostics (Basel) 2021;11:215. [Crossref] [PubMed]
- Martin SS, van Assen M, Rapaka S, et al. Evaluation of a Deep Learning-Based Automated CT Coronary Artery Calcium Scoring Algorithm. JACC Cardiovasc Imaging 2020;13:524-6. [Crossref] [PubMed]
- Zhang N, Yang G, Zhang W, et al. Fully automatic framework for comprehensive coronary artery calcium scores analysis on non-contrast cardiac-gated CT scan: Total and vessel-specific quantifications. Eur J Radiol 2021;134:109420. [Crossref] [PubMed]
- de Vos BD, Wolterink JM, Leiner T, et al. Direct Automatic Coronary Calcium Scoring in Cardiac and Chest CT. IEEE Trans Med Imaging 2019;38:2127-38. [Crossref] [PubMed]
- AlGhamdi M, Abdel-Mottaleb M, Collado-Mesa F. DU-Net: Convolutional Network for the Detection of Arterial Calcifications in Mammograms. IEEE Trans Med Imaging 2020;39:3240-9. [Crossref] [PubMed]
- Zhang Y, van der Werf NR, Jiang B, et al. Motion-corrected coronary calcium scores by a convolutional neural network: a robotic simulating study. Eur Radiol 2020;30:1285-94. [Crossref] [PubMed]
- van Assen M, Martin SS, Varga-Szemes A, et al. Automatic coronary calcium scoring in chest CT using a deep neural network in direct comparison with non-contrast cardiac CT: A validation study. Eur J Radiol 2021;134:109428. [Crossref] [PubMed]
- Fischer AM, Eid M, De Cecco CN, et al. Accuracy of an Artificial Intelligence Deep Learning Algorithm Implementing a Recurrent Neural Network With Long Short-term Memory for the Automated Detection of Calcified Plaques From Coronary Computed Tomography Angiography. J Thorac Imaging 2020;35:S49-57. [Crossref] [PubMed]
- Lessmann N, van Ginneken B, Zreik M, et al. Automatic Calcium Scoring in Low-Dose Chest CT Using Deep Neural Networks With Dilated Convolutions. IEEE Trans Med Imaging 2018;37:615-25. [Crossref] [PubMed]
- Isgum I, Prokop M, Niemeijer M, et al. Automatic coronary calcium scoring in low-dose chest computed tomography. IEEE Trans Med Imaging 2012;31:2322-34. [Crossref] [PubMed]
- Wolterink JM, Leiner T, Viergever MA, et al. Generative Adversarial Networks for Noise Reduction in Low-Dose CT. IEEE Trans Med Imaging 2017;36:2536-45. [Crossref] [PubMed]
- Klug M, Shemesh J, Green M, et al. A deep-learning method for the denoising of ultra-low dose chest CT in coronary artery calcium score evaluation. Clin Radiol 2022;77:e509-17. [Crossref] [PubMed]
- Sun Z, Ng CKC. Artificial Intelligence (Enhanced Super-Resolution Generative Adversarial Network) for Calcium Deblooming in Coronary Computed Tomography Angiography: A Feasibility Study. Diagnostics (Basel) 2022.
- Eng D, Chute C, Khandwala N, et al. Automated coronary calcium scoring using deep learning with multicenter external validation. NPJ Digit Med 2021;4:88. [Crossref] [PubMed]
- Kamel PI, Yi PH, Sair HI, et al. Prediction of Coronary Artery Calcium and Cardiovascular Risk on Chest Radiographs Using Deep Learning. Radiol Cardiothorac Imaging 2021;3:e200486. [Crossref] [PubMed]
- Du T, Xie L, Zhang H, et al. Training and validation of a deep learning architecture for the automatic analysis of coronary angiography. EuroIntervention 2021;17:32-40. [Crossref] [PubMed]
- Isgum I, Rutten A, Prokop M, et al. Automated aortic calcium scoring on low-dose chest computed tomography. Med Phys 2010;37:714-23. [Crossref] [PubMed]
- de Vos BD, Lessmann N, de Jong PA, et al. Deep Learning-Quantified Calcium Scores for Automatic Cardiovascular Mortality Prediction at Lung Screening Low-Dose CT. Radiol Cardiothorac Imaging 2021;3:e190219. [Crossref] [PubMed]
- van Velzen SGM, Lessmann N, Velthuis BK, et al. Deep Learning for Automatic Calcium Scoring in CT: Validation Using Multiple Cardiac CT and Chest CT Protocols. Radiology 2020;295:66-79. [Crossref] [PubMed]
- Graffy PM, Liu J, O'Connor S, et al. Automated segmentation and quantification of aortic calcification at abdominal CT: application of a deep learning-based algorithm to a longitudinal screening cohort. Abdom Radiol (NY) 2019;44:2921-8. [Crossref] [PubMed]
- Lems WF, Paccou J, Zhang J, et al. Vertebral fracture: epidemiology, impact and use of DXA vertebral fracture assessment in fracture liaison services. Osteoporos Int 2021;32:399-411. [Crossref] [PubMed]
- Schousboe JT, Wilson KE, Kiel DP. Detection of abdominal aortic calcification with lateral spine imaging using DXA. J Clin Densitom 2006;9:302-8. [Crossref] [PubMed]
- Li D, Qiao H, Han Y, et al. Histological validation of simultaneous non-contrast angiography and intraplaque hemorrhage imaging (SNAP) for characterizing carotid intraplaque hemorrhage. Eur Radiol 2021;31:3106-15. [Crossref] [PubMed]
- Chen S, Ning J, Zhao X, et al. Fast simultaneous noncontrast angiography and intraplaque hemorrhage (fSNAP) sequence for carotid artery imaging. Magn Reson Med 2017;77:753-8. [Crossref] [PubMed]
- Qi H, Sun J, Qiao H, et al. Carotid Intraplaque Hemorrhage Imaging with Quantitative Vessel Wall T1 Mapping: Technical Development and Initial Experience. Radiology 2018;287:276-84. [Crossref] [PubMed]
- Cheng JZ, Cole EB, Pisano ED, et al. Detection of arterial calcification in mammograms by random walks. Inf Process Med Imaging 2009;21:713-24. [Crossref] [PubMed]
- Roseman DA, Hwang SJ, Manders ES, et al. Renal artery calcium, cardiovascular risk factors, and indexes of renal function. Am J Cardiol 2014;113:156-61. [Crossref] [PubMed]
- Gal R, van Velzen SGM, Hooning MJ, et al. Identification of Risk of Cardiovascular Disease by Automatic Quantification of Coronary Artery Calcifications on Radiotherapy Planning CT Scans in Patients With Breast Cancer. JAMA Oncol 2021;7:1024-32. [Crossref] [PubMed]
- Gernaat SAM, van Velzen SGM, Koh V, et al. Automatic quantification of calcifications in the coronary arteries and thoracic aorta on radiotherapy planning CT scans of Western and Asian breast cancer patients. Radiother Oncol 2018;127:487-92. [Crossref] [PubMed]
- van Velzen SGM, Gal R, Teske AJ, et al. AI-Based Radiation Dose Quantification for Estimation of Heart Disease Risk in Breast Cancer Survivors After Radiation Therapy. Int J Radiat Oncol Biol Phys 2022;112:621-32. [Crossref] [PubMed]
(English Language Editor: J. Jones)