A gene signature driven by abnormally methylated DEGs was developed for TP53 wild-type ovarian cancer samples by integrative omics analysis of DNA methylation and gene expression data
Highlight box
Key finding
• A 5 genes prognostic model based on DNA methylation may be useful for accurately predicting prognosis for OC.
What is known and what is new?
• DNA methylation has become a hot topic to improve OC treatment.
• A novel 5 genes signature related DNA methylation was constructed to predicting prognosis of OC.
What is the implication, and what should change now?
• A 5 genes signature based on DNA methylation may be used for clinical application in OC to help clinicians develop personalized treatment.
IntroductionOther Section
Ovarian cancer (OC) is the second leading cause of death related to gynecological malignant tumors worldwide, which is more common in women aged over 50 years and is usually diagnosed late, with a mortality rate of about 63% (1,2). About 90–95% of OCs are primary ovarian malignant tumors, and 5–10% are primary metastatic ovarian malignant tumors that are detected in other proximal sites (3). The most common subtype of OC is epithelial carcinoma, which originates from epithelial cells on the surface of the ovary or fallopian tube (4). The standard treatment for OC is radical tumor reduction surgery combined with chemotherapy (5). Despite improved survival owing to current standard nursing therapy, the majority of patients with OC relapse (6). Our understanding of the molecular pathways that drive the development and progression of human cancer has advanced in recent decades with the advent of targeted anticancer therapies. Targeted drugs block the growth and survival of cancer cells by specifically targeting the molecular and signaling cascades needed for tumorigenesis, and thus, these therapies may have better efficacy and fewer off-target side effects than chemotherapy drugs (7). Hence, identifying the relevant targets of OC is crucial for early screening and improving the prognosis of patients.
The occurrence and progression of cancer are regulated by epigenetic and genetic events. Epigenetic characteristics based on abnormal DNA methylation are considered to be powerful biomarkers for early diagnosis and prognosis of cancer (8,9). Also, the sensitivity of this epigenetic feature in cancer diagnosis may be superior to that of somatic mutation. There are three potential reasons for this. First, abnormal DNA methylation occurs in the early stages of tumorigenesis and may be of tissue and cancer-specific types. Second, DNA methylation patterns are widespread in the entire tumor tissue and the same tumor type, while somatic mutations are usually limited to tumor cell subsets. Third, DNA methylation is consistent in larger genomic regions; thus, multiple cytosine-guanine (CpG_ dinucleotides can be used for detection (10). Some studies have reported that patterns of DNA methylation in tumor cells treated with drugs can alter and support the acquisition of resistance to treatments such as radiation and chemotherapy (11,12). Altered methylation status of multiple genes leads to dysregulation of Wnt canonical and PI3K/AKT/mTOR signaling pathways, which is associated with resistance of many cancers to current therapies (13,14). Analysis of methyl groups reveals global patterns of methylation and provides gene expression signatures of methylated region-specific DNA that can be used to predict therapeutic outcomes of anti-cancer therapeutic responses (15). In demethylated tumors with hypermethylated CpG island promoters, immunomodulatory pathway genes are concentrated in these regions and repressed transcriptionally. Global methylation loss is associated with immune escape signals, and genomic hypomethylation is associated with immune escape features of tumors. Changes in DNA methylation suggest epigenetic regulation in precise immunotherapy (16).
Recent studies by Lønning and Knappskog (17) have shown that the inactivation of tumor protein53 (TP53) can effectively predict the resistance of breast cancer patients to DNA-damaging drugs (18). Currently, sequencing of all the encoding exons of TP53 (exon 2-11) has been established as the gold standard for evaluating TP53 mutation status. In addition to evaluating TP53 mutation status, The function of TP53 can also be better evaluated by evaluating key genes in the TP53 pathway and their redundant pathways to compensate for the deletion of TP53 (17). The high prevalence of TP53 mutations in human cancers has also facilitated the development of targeted therapies using the TP53 pathway (19), such as adenovirus vector delivery of wild-type TP53, TP53-based vaccines to attract t cells, and the use of siRNA to knockout the negative regulators of TP53. Small molecules (e.g., PRIMA-1, RITA) restore the activity of TP53.
Recent technological advances have allowed the identification of methylation regions and characteristics as novel biomarkers (20). Genome-wide interrogation of DNA methylation signatures, in conjunction with machine learning methods, has great prospects for the detection and classification of cancer (21). Dong et al. identified a new model composed of six DNA methylation sites for lung adenocarcinoma, with strong survival prediction ability (22). Bisarro dos Reis et al. developed a model based on 21 CpGs that was able to predict poor prognosis in patients with well-differentiated thyroid carcinoma with high specificity (23). These findings imply that aberrant methylation is also a feature of OC; however, a comprehensive analysis of DNA methylation in this disease as well as reliable and stable diagnostic and prognostic classifiers modulated by DNA methylation are still lacking.
Bioinformatics contributes to the research of targeted therapies for diseases (24,25). In this study, we performed differential analysis by integrating the DNA methylation and gene expression data from TP53 wild-type OC samples and normal samples to identify differentially expressed genes (DEGs) with abnormal methylation. A network medicine framework was utilized as an OC to identify potential therapeutic drugs and was also applied in conjunction with machine learning methods to construct a prognostic gene model based on DEGs with aberrant methylation. We present the following article in accordance with the STREGA reporting checklist (available at https://atm.amegroups.com/article/view/10.21037/atm-22-5764/rc).
MethodsOther Section
Collection of OC gene expression and methylation data
The Cancer Genome Atlas (TCGA) was interviewed to query OC data, including the latest expression profile, methylation data, and clinical follow-up information. After downloading these data, a total of 374 primary tumor samples were selected, of which 359 were TP53 wild-type samples and 15 were TP53 mutant samples. The normal sample data obtained from the Genotype-Tissue Expression (GTEx) project were used as the control data of primary tumor samples in TCGA. The study was conducted in accordance with the Declaration of Helsinki (as revised in 2013).
Differential methylation analysis
The Illumina Infinium HumanMethylation27 BeadChip array was employed to measure the DNA methylation of the original epigenetic range, involving a total of 26,956 probes. The original methylation data were represented by the methylation intensity β value, which was converted into an M value using the formula: M = log2[β/(1 − β)]. The differentially methylated CpG site (DMS) between the OC and normal control samples was screened according to the cutoff criteria of the false discovery rate (FDR) <0.01 and logFC >2 adjusted by the Benjamini & Hochberg (BH) method. The matching files of the CpG site and genes were acquired from the Illumina website (https://www.illumina.com/). The M value of different gene regions was then calculated, including TSS1500, TSS200, 5'-untranslated regions (UTR), first exon, genebody, 3'-UTR, and the intergenic region. The average M value of CpGs in all gene regions was applied to measure the methylation level of a particular gene. The screening criteria for differentially methylated genes (DMGs) between the OC samples and normal controls were adjusted according to FDR <0.01 and log2|FC| >1. Differential methylation analysis was performed using the “limma” R package (26).
Differential expression analysis
The initial expression data obtained in TCGA were transformed by log2, and then the differentially expressed genes (DEGs) between the OC and normal control samples were also analyzed using “limma”. The significance threshold was set as FDR <0.01 and log2|FC| >1 adjusted using the BH method. The DEGs within the threshold of FDR <0.01 and log2FC >1 were the differentially down-regulated genes in the OC versus normal samples, while the DEGs within the threshold of FDR <0.01 and log2FC <−1 were the differentially up-regulated genes in the OC versus normal samples.
Functional annotation analysis
The ClusterProfiler package (27) was used for Gene Ontology (GO) analysis of the DMGs and DEGs, which mainly includes three aspects: GO biological process (BP), GO molecular function (MF), and cellular component (CC). The ClusterProfiler package was also employed to conduct the Kyoto Encyclopedia of Genes and Genomes (KEGG) terms annotation analysis of the DMGs and DEGs. P<0.05 was utilized as the standard of filtering terms in both the GO and KEGG analyses.
Screening and analysis of abnormally methylated DEGs
Venn Diagram Online Software (http://bioinformatics.psb.ugent.be/webtools/Venn/) was utilized to select the abnormally methylated DEGs by intersecting DEGs and DMGs. The samples were classified by principal component analysis (PCA) and linear discriminate analysis (LDA) using the expression profile of abnormally methylated DEGs and the methylation data of genebody, TSS200, and TSS1500. The quality of the classification model was evaluated by the area under the receiver operating characteristic (ROC) curve (AUC) generated by the “pROC” package.
Screening of therapeutic agents targeting abnormally methylated DEGs
NetworkAnalyst3.0 (https://www.networkanalyst.ca/) is an analysis platform for users to create cell type- or tissue-specific protein-protein interaction (PPI) networks, gene regulatory networks, gene co-expression networks, and toxicogenomics and pharmacogenomics research networks (28). The relationship pairs of drug-aberrant methylated DEGs were determined using the NetworkAnalyst 3.0 tool based on the DrugBank’s drug-target interaction information.
The simulated reference distance distribution of drugs was constructed by assessing the network length between S and T. A group of protein nodes was randomly extracted from the human PPI group and their number (R) was the same as that of the target. The distance d (S, R) between the simulated drug target protein and the abnormally methylated DEGs was calculated iteratively.
Molecular docking
AutodockVina software (29) was used to conduct molecular docking simulations. Information about the compounds was obtained from the PubChem database (https://pubchem.ncbi.nlm.nih.gov). The Protein Data Bank ID of the target protein was obtained from the Protein Data Bank (PDB) database (https://www1.rcsb.org/). OpenBabel (30) was applied to add polar hydrogen, distributed charge, and minimized energy to small molecules. The protein system was processed with AutoDockTools to add polar hydrogen and calculate the charge, which was stored in Protein Data Bank, Partial Charge (Q), & Atom Type (T) (PDBQT) format. The key compounds were used as ligands, and the appropriate grid box coordination and size were set according to the target protein. The active pocket of the target protein was determined by the inhibitor site in its crystal structure or the active pocket of the homologous protein. The binding conformation with the lowest binding free energy was selected and imported into PyMOL (31) for visualization.
Molecular dynamics simulation
Molecular dynamics (MD) simulations were carried out using the CHARMM36 force field (32). The Str file of the ligand was generated using the CHARMM general force field (CgenFF). The protein was dissolved in the dodecahedron filled with transferable intermolecular potential 3 point (TIP3P) water molecules, and sodium and chloride ions were added to the system to neutralize the charge. The system was energy minimized by 5,000 steps of the steepest descent. The global electrostatic interaction was calculated using the particle-mesh Ewald (PME) algorithm. Next, the optimized system was subjected to 100 ps position-restrained dynamics simulations, including isochoric-isothermal (NVT) and isobaric-isothermal (NPT) equilibriums, and was equilibrated at 300 K and 1 bar. The production simulation of each system was carried out at 100 ns under periodic boundary conditions. The coordinates and energy of production MD were saved every 10 ps and run for a total of 80 ns. The stability of the structure during the MD simulation was evaluated by determining root-mean-square deviation (RMSD).
Construction and validation of prognostic gene signatures based on abnormally methylated DEGs
To screen genes with prognostic value in abnormally methylated DEGs, the TP53 wild-type samples obtained from TCGA were randomly divided into two unmatched and uncrossed groups. One group was used as the training set (n=180) and the other as the validation set (n=179). According to the gene expression and survival follow-up data of abnormally methylated DEGs in the training set, a regularized least absolute shrinkage and selection operator (LASSO) regression analysis was performed 1,000 times using 10-fold cross-validation. The results of each dimensionality reduction were summarized, the frequency of each probe was counted 100 times, and the gene combination with the maximum frequency was used as the component of gene signature construction. The gene signature was obtained by adding the product of the regression coefficient and the expression level of each gene. The survival rate of samples in different verification sets was evaluated by the Kaplan-Meier method and logarithmic rank test, and the prediction ability of the prognostic gene signature was evaluated using a time-dependent ROC curve. Sangerbox provided assistance with this article (33).
Statistical analysis
For survival analysis in different groups, log-rank test was conducted to compare their differences. All statistical analysis was performed in R software (v4.0; The R Foundation for Statistical Computing, Vienna, Austria).
ResultsOther Section
Identification of DMGs
Owing to the key role of the TP53 protein in the tumorigenesis of OC, we first analyzed and compared the expression of TP53 in OC and normal samples. We observed that the expression of TP53 in tumor samples was significantly higher than that in normal samples (Figure S1A). The difference in the tumor microenvironment (TME) between OC and normal samples was weighed by comparing the stromal score, immune score, and ESTIMATE score; it was found that compared with the normal samples, the stromal score of the OC samples decreased notably, while the immune score and ESTIMATE score increased markedly (Figure S1B). Given that TP53 mutation may be a confounding factor affecting OC research, only 359 TP53 wild-type samples out of 374 primary tumor samples were selected for this study.
CpG sites are distributed in different regions of specific genes. We checked the CpG methylation levels in the TSS200, TSS1500, and genebody regions using the DNA methylation comment file. Then, the DMGs in each region were identified. In the TSS200 region, 87 hypermethylated DMGs and 166 demethylated DMGs were identified; in the TSS1500 region, 129 hypermethylated DMGs and 693 demethylated DMGs were obtained; and in the genebody region, 439 hypermethylated DMGs and 337 demethylated DMGs were identified (Figure 1A). Hypomethylation tended to be located in the TSS1500 region, and hypermethylation tended to be located in the genebody region (Figure 1B). By cross-linking the hypermethylated DMGs or demethylated DMGs of the three regions, we found that most of the DMGs were region-specific, and 13 hypermethylated DMGs existed in three regions simultaneously (Figure 1C). The three regions also shared 15 demethylated DMGs (Figure 1D). The DMGs in the genebody region were introduced into ClusterProfiler to analyze the biological pathways and pathways they enriched. The main BPs they enriched included cornification, keratinization, skin development, epidermal cell differentiation, and keratinocyte differentiation. The significantly enriched CCs were high-density lipoprotein particles, intermediate filament, and intermediate filament cytoskeleton. The MFs involved included peptidase inhibitor activity, endopeptidase regulator activity, pattern recognition receptor activity, etc., which were significantly correlated with neuroactive ligand-receptor interaction (Figure 1E).
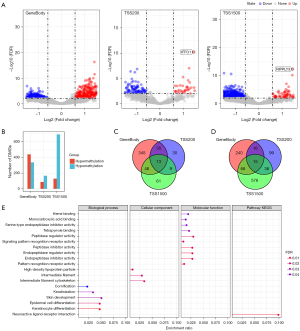
Screening of DEGs
A total of 6,841 DEGs were screened by differential analysis between the TP53 wild-type OC samples and the normal samples, including 3,502 significantly up-regulated DEGs and 3,339 significantly down-regulated DEGs in the TP53 wild-type OC samples (Figure 2A). Unsupervised hierarchical clustering analysis of these DEGs was then conducted, and the results showed that differential genes could clearly distinguish tumor samples from normal samples (Figure 2B). By analyzing the GO and KEGG terms involved in the DEGs, we detected 522 BPs, 83 CCs, 32 MFs, and 14 KEGG pathways that were significantly enriched in the DEGs. Among them, cell adhesion molecules (CAMs), systemic lupus erythematosus, the rap1 signaling pathway, the p53 signaling pathway, transcriptional misregulation in cancer, small cell lung cancer, Th17 cell differentiation, extracellular matrix (ECM)-receptor interaction, viral protein interaction with cytokines and cytokine receptors, and complement and coagulation cascades were the most significantly enriched GO terms (Figure 2C). KEGG pathways that were significantly enriched by DEGs also included cell adhesion molecule binding, extracellular matrix structural constituent, chemokine activity, etc. (Figure 2D).
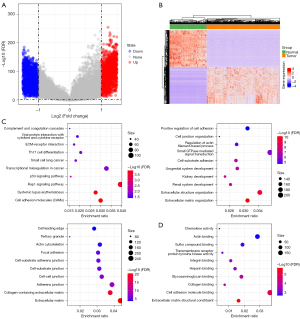
Identification and characterization of abnormally methylated DEGs
To determine the aberrant methylation-driven DEGs, we intersected DMGs and DEGs, and divided the intersecting genes into four groups: the hypo-methylated and upregulated group (HypoUp), the hyper-methylated and downregulated group (HypoDown), the hyper-methylated and upregulated group (HyperUp,) and the hypo-methylated and downregulated group (HyperDown). A total of 217 hypermethylated DEGs were obtained in the genebody region, 70 hypermethylated DEGs were obtained in the TSS200 region, and 243 hypermethylated DEGs were obtained in the TSS1500 region (Figure 3A-3C). Figure 3D depicts the differential methylation multiple and differential expression multiple of these aberrant methylated DEGs. It can be seen that some genes appear simultaneously in different regions, such as ammonium transport (AMT) and Cysteine dioxygenase 1(CDO1), which can be found in z in both TSS200 and TSS1500, and the orphan nuclear receptor steroidogenic factor 1 (NR5A1), which can be found in both TSS200 and genebody (Figure 3D). A total of 440 DMGs were identified, including 38 HyperDown, 132 HyperUp, 147 HypoDown, and 123 HypoUp, according to the levels of abnormal methylated DEGs in the three regions. The number distribution of four groups of abnormally methylated DEGs in each region is shown in Figure 3E.
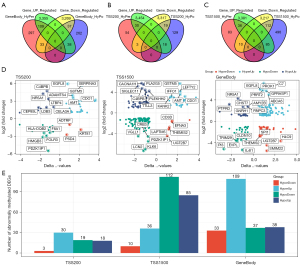
Next, we examined the distribution of 440 aberrant methylated DEGs in chromosomes and found that there were more than 10 aberrant methylated DEGs on each chromosome. Chromosome 1 was rich in the most aberrant methylated DEGs, 63 in total. In addition, the methylation patterns of abnormally methylated DEGs in similar gene regions were similar and consistent (Figure 4A). To explore the differences in gene expression and DNA methylation patterns between tumors and normal samples, we used the gene expression profiles of abnormally methylated DEGs as well as the methylation data of genebody, TSS200, and TSS1500 for discriminative pattern recognition analysis. Notable segregation between OC tumor tissue and healthy ovarian tissue could be observed both in the gene expression profile and the PCA results of methylation data from different regions (Figure 4B). The ROC curve confirmed the good performance of the classifier based on the abnormally methylated DEGs expression and methylation profiles (Figure 4C). GO analysis showed that 440 abnormally methylated DEGs were enriched into nine GO terms, including four BPs, three CCs, and two MFs: monocarboxylic acid binding, cell cortex part, extracellular matrix structural constituent, cell-matrix adhesion, cell-substrate adhesion, collagen-containing extracellular matrix, extracellular matrix organization, extracellular matrix, and extracellular structure organization, respectively (Figure 4D).
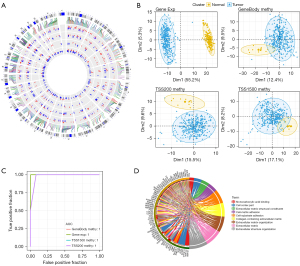
Potential agents and binding mechanisms targeting abnormally methylated DEGs
We attempted to identify the potential drugs targeting abnormally methylated DEGs by employing a network medicine framework. We obtained drug-protein interaction data from the DrugBank database and analyzed protein-drug interactions using the NetworkAnalyst 3.0 tool, and identified 32 genes that interact with the drugs (Table S1). Based on the drug-protein interaction data of DrugBank and the PPI information in the STRINGdb database, we calculated the proximity between drugs and abnormally methylated DEGs via network proximity analyses and constructed a random network against the background of stochastic simulation. We found that whether abnormally methylated DEGs or our randomly selected gene set were taken as samples, the Z score of network proximity degree was distributed in the range of 1–2; however, the Z score distribution of network proximity degree based on the abnormally methylated DEGs was more concentrated (Figure 5A). We conducted multiple hypothesis tests based on the random data obtained from the references and selected 126 drugs with small distances and FDR <0.01 as candidate drug sets related to the abnormally methylated DEGs gene sets.
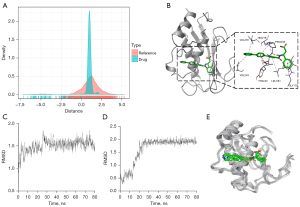
Taking the intersection of abnormally methylated DEGs and the 32 genes that interact with the drugs, six genes were obtained: cathepsin K (CTSK), 11beta-hydroxysteroid oxidoreductase type 1 (HSD11B1), matrix metalloproteinase 12 (MMP12), lipocalin 2 (LCN2), Integrin alpha L chain (ITGAL), and matrix metalloproteinase 1 (MMP1) (Table 1). The binding mode and free energy of each gene with the candidate drugs were obtained by molecular docking. Except for DB06367 and DB07556, 13 candidate compounds bound proteins expressed by six genes with affinities below −5 kcal/moL (Table 2). Since the binding free energy of DB03367 and MMP12 was the lowest, which indicated that their binding was very stable, the schematic diagram of their molecular docking conformation was constructed. When DB03367 binds to the MMP12 protein, it is embedded into the active site of the MMP12 protein, forms a hydrogen bond with PRO238 and LEU181, produces favorable hydrophobic interaction with IEU181, VAL235, TYR240, and VAL243, and produces favorable π-π interaction with HIS218 (Figure 5B). The MD of 80 ns illustrated the RMSD changes of the MMP12 protein bound to DB03367 and the RMSD value of DB03367 binding to the MMP12 protein. The conformation of the MMP12 protein was very stable during the 80 ns MD process (Figure 5C). The RMSD value of DB03367 fluctuated relatively significantly in the former 20 ns, exhibiting a notable upward trend. When it reached the 20 ns, it was stable and remained basically unchanged in the subsequent 60ns (Figure 5D). The dynamic binding pattern of DB03367 to the MMP protein during the MD process of 80ns is shown in Figure 5E. It was worth noting that because the molecular docking in this experiment was semi-flexible docking, it was understandable that the RMSD of the molecular skeleton fluctuated moderately in the initial stage of MD. During the entire process, DB03367 bonded to the MMP12 protein stably.
Table 1
Gene | Gene type | Drug count | Drug example |
---|---|---|---|
CTSK | HyperUp | 19 | MIV-711; MIV-701; Dibenzyl (carbonylbis{2,1-hydrazinediyl[(2S)-4-methyl-1-oxo-1, 2-pentanediyl]}) biscarbamate; Ilomastat |
HSD11B1 | HypoUp | 14 | (5S)-2-{[(1S)-1-(4-Fluorophenyl) ethyl]amino}-5-(2-hydroxy-2-propanyl)-5-methyl-1, 3-thiazol-4(5H)-one; 2-(2-CHLORO-4-FLUOROPHENOXY)-2-METHYL-N-[(1R,2S,3S,5S,7S)-5-(METHYLSULFONYL)-2-ADAMANTYL]PROPANAMIDE |
MMP12 | HypoDown | 12 | Acetohydroxamic acid; CGS-27023; CP-271485 |
LCN2 | HypoDown | 6 | Methyl nonanoate; 2,3-Dihydroxybenzoylserine; Sulpiride |
ITGAL | HypoDown | 7 | Methyl nonanoate; Trencam-3,2-Hopo; Miconazole |
MMP1 | HypoDown | 4 | CGS-27023; N-[3-(N’-HYDROXYCARBOXAMIDO)-2-(2-METHYLPROPYL)-PROPANOYL]-O-TYROSINE-N-METHYLAMIDE |
DEMGs, differential methylated genes.
Table 2
DrugBank ID | Compound | Target | Docking score | H-Bond interactions |
---|---|---|---|---|
DB01858 | [1-(4-Fluorobenzyl) Cyclobutyl]Methyl (1s)-1-[Oxo(1h-Pyrazol-5-Ylamino)Acetyl]Pentylcarbamate | CTSK | −6.231 | GLY66, ASN161 |
DB03405 | N2-[(Benzyloxy)carbonyl]-N-[(3R)-1-{N-[(benzyloxy)carbonyl]-L-leucyl}-4-oxo-3-pyrrolidinyl]-L-leucinamide | CTSK | −8.337 | GLN19, GLY66, ASN161 |
DB03456 | 2-[(benzyloxy)carbonyl]-n1-[(3S)-1-cyanopyrrolidin-3-yl]-l-leucinamide | CTSK | −6.843 | GLY66, ASN161 |
DB04234 | N2-({[(4-Bromophenyl) Methyl]Oxy}Carbonyl)-N1-[(1s)-1-Formylpentyl]-L-Leucinamide | CTSK | −6.379 | GLY66, ASN161 |
DB06367 | Relacatib | CTSK | −4.439 | GLN19, GLY64, TRP184 |
DB02118 | CP-271485 | MMP12 | −7.56 | LEU181, ALA182 |
DB03367 | PF-00356231 | MMP12 | −12.871 | LEU181, PRO238 |
DB04405 | 2-[2-(1,3-Dioxo-1,3-Dihydro-2h-Isoindol-2-Yl)Ethyl]-4-(4'-Ethoxy-1,1'-Biphenyl-4-Yl)-4-Oxobutanoic Acid | MMP12 | −11.83 | TYR240 |
DB07446 | N-(biphenyl-4-ylsulfonyl)-D-leucine | MMP12 | −9.932 | LEU181, GLU219 |
DB07556 | CGS-27023 | MMP12 | −2.798 | – |
DB02329 | Carbenoxolone | HSD11B1 | −8.091 | SER261 |
DB06992 | (3,3-dimethylpiperidin-1-yl)(6-(3-fluoro-4-methylphenyl)pyridin-2-yl)methanone | HSD11B1 | −8.524 | SER170 |
DB07017 | (5S)-2-{[(1S)-1-(4-Fluorophenyl) ethyl]amino}-5-(2-hydroxy-2-propanyl)-5-methyl-1,3-thiazol-4(5H)-one | HSD11B1 | −7.695 | SER170, TYR280 |
DB07049 | (2R)-1-[(4-tert-butylphenyl) sulfonyl]-2-methyl-4-(4-nitrophenyl)piperazine | HSD11B1 | −8.206 | – |
DB07310 | (5S)-2-{[(1S)-1-(2-fluorophenyl) ethyl]amino}-5-methyl-5-(trifluoromethyl)-1,3-thiazol-4(5H)-one | HSD11B1 | −7.987 | ALA172 |
DEG, differentially expressed gene.
Prognostic signatures were constructed by screening genes with prognostic value from abnormally methylated DEGs
LASSO regression analyses of the TP53 wild-type samples were carried out 1,000 times in the TCGA training set to identify the prognostic genes from 440 abnormally methylated DEGs. Ten-fold cross-validation was applied and the dimensionality reduction results were summarized. We found that five probe combinations exhibited the highest occurrence frequency, including AADAC, GCNT2, SACS, DACT3, and PI3 (Figure 6A-6C). LASSO regression also provided the coefficient of each gene, so the risk score was calculated according to the following formula: risk score = −0.17 × AADAC − 0.299 × GCNT2 + 0.542 × SACS + 0.291 × DACT3 + 0.152 × PI3. Based on this formula, the risk score of each patient in the training set was calculated and arranged from large to small, and a survival state scatter map and gene expression heat map were drawn.
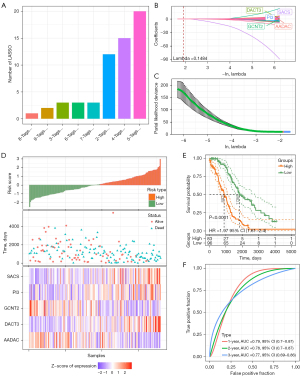
In the process of the risk score changing from low to high, the number of survival samples gradually decreased; the expression of SACS, PI3, and DACT3 increased; and the expressions of GCNT2 and AADAC decreased gradually (Figure 6D). According to the overall survival (OS) of the samples grouped by risk score, we found that the risk model could significantly distinguish the OS of high- and low-risk groups, and the low-risk group had an OS advantage (Figure 6E). The AUCs of the prognostic risk model were 0.79, 0.78, and 0.77 at 1, 2, and 3 years, respectively (Figure 6F).
Verification of the prognostic gene signature
In the same way, the risk scores of OC patients were calculated in the TCGA validation set containing 179 OC samples, the TCGA-OC dataset containing 359 samples, and the GSE32062 dataset containing 260 OC samples. For the TCGA verification set containing 179 OC samples, the survival trends of the samples with risk scores and the gene expression changes in the model were identical to those observed in the training set (Figure 7A). The survival status of the samples in the high-risk group was also significantly worse than that in the low-risk group (Figure 7B). The AUCs describing the accuracy of the risk model in identifying the prognosis of OC samples reached 0.64, 0.72, and 0.65 at 1, 2, and 3 years, respectively (Figure 7C).

For the TCGA-OC dataset containing 359 samples and the GSE32062 dataset containing 260 OC samples, the survival trends and prognostic differences between samples with distinct risk scores were no different from those of the training and TCGA validation sets. More specifically, the prognosis of the high-risk group in the two data sets was significantly worse than that of the low-risk group. The risk model exhibited effective predictive value in both cohorts, with a 1-year AUC =0.68, a 2-year AUC =0.72, and a 3-year AUC =0.67 of the ROC curves in TCGA-OC dataset, and a 1-year AUC =0.69, 2-year AUC =0.66, and 3-year AUC =0.67 of the ROC curves in the GSE32062 dataset (Figures 8,9).
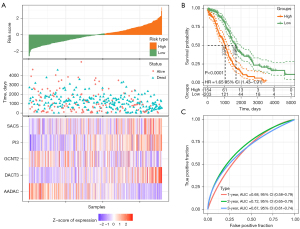
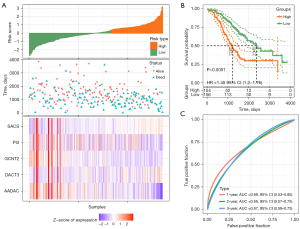
DiscussionOther Section
Owing to its sensitivity, specificity, and ease of analysis, DNA methylation has great potential to become a routine clinical cancer biomarker (10). Abnormal DNA methylation changes were also repeatedly observed in OC, which affected the activity of variable genes and led to variable tissue tumorigenesis (34). Although there are more than 14,000 scientific publications describing biomarkers based on DNA methylation as well as their clinical association in cancer, only 14 DNA methylation-based biomarker assays are currently commercially available and are designed to measure the methylation of only 13 genes in total, including GSTP1, APC, RASSF1, NDRG4, BMP3, SEPT9, SHOX2, TWIST1, OTX1, ONECUT2, MGMT, BCAT1, and IKZF1 (9). These markers are all monomethylated genes. Considering that the occurrence and progression of OC are the result of the common regulation of multiple genes, it is necessary to explore the characteristics of polymethylated genes with diagnostic or prognostic value in OC.
With the emergence of next-generation sequencing and large-scale joint research, it has become possible to analyze the genomes and epigenomes of thousands of primary tumors of almost every cancer type (35). Integrative omics analysis combining transcription, histone modification, and DNA methylation data will be a more reliable approach to better advance the identification of clinical biomarkers for precision cancer therapy (36). Promoter methylation has been established as the mechanism of tumor suppressor gene inactivation (37). In this study, we comprehensively analyzed the gene expression and DNA methylation data of OC and identified the DEGs and DMGs in different gene regions between TP53 wild-type OC and normal samples. A discriminative pattern recognition analysis model was constructed based on 440 abnormally methylated DEGs (i.e., overlapping genes between the DEGs and DMGs), which confirmed its superior performance in distinguishing normal samples from TP53 wild-type OC samples. Functionally, the abnormally methylated DEGs were closely connected with biological pathways related to cell metastasis, and thus, are likely to be potential targets for OC.
The development of network medical methods promotes the study and development of drug discovery (38). Advances in advanced “omics” and machine learning are providing new insights into drug discovery and the mechanisms of drug binding to targets (39). In this study, we created a machine learning-based network medicine screening framework that identifies potential targeted agents against abnormally methylated DEGs. Subsequently, the compound-protein target complex with the lowest binding energy was shown to demonstrate molecular docking, and MD simulation analysis was conducted to confirm the targeting effect of the selected drugs on the identified proteins.
Finally, based on LASSO regression analysis, we constructed a gene signature based on five abnormally methylated DEGs, which is reliable and accurate in evaluating the prognosis of OC patients. Some genes in abnormally methylated DEGs-driven gene signatures have been reported to drive cancer initiation and progression. For instance, arylacetamide deacetylase (AADAC) is a survival-related gene in patients with Borrmann III advanced gastric cancer; the higher the messenger RNA (mRNA) and protein expression levels, the longer the survival time of patients (40). AADAC inhibits the malignant progression of OC at the cellular level and promotes the therapeutic activity of cisplatin and imatinib against OC cells (41). Also, β1,6 N-acetylglucosaminyl transferase 2 (GCNT2) has been shown to promote the malignant progression of cancer as an oncogene in prostate cancer 26678556, breast cancer 21750175, esophageal cancer 30575058, and colon cancer 28542779, and as a tumor suppressor gene in melanoma cells. Furthermore, the Dapper antagonist of catenin-3 (DACT3) inhibits the malignant tumor phenotype in acute myeloid leukemia (42). Peptidase inhibitor 3 (PI3), often called elafin, is overexpressed and secreted in OC, and is related to the poor OS of OC and may be the determinant of the low survival rate of OC (43). To some extent, these studies revealed the feasibility of our gene signature driven by five abnormally methylated DEGs to predict the prognosis of OC.
However, the limitations of this study cannot be ignored. Firstly, all of the data were downloaded from public databases, and the sample size and clinical information were limited. Secondly, although a risk score system consisting of nine genes was developed, the regulatory network and biological effects between these genes still need to be explored.
ConclusionsOther Section
Through transcriptome data, DNA methylation data, the CpGs of different gene regions, and network medicine analysis based on machine learning, our study screened abnormally methylated DEGs and potential therapeutic drugs for OC and constructed a gene signature based on five abnormally methylated DEGs, which could better predict the risk of death. Analysis in this study may be used for clinical application in OC to help clinicians develop personalized treatment.
AcknowledgmentsOther Section
Funding: None.
FootnoteOther Section
Reporting Checklist: The authors have completed the STREGA reporting checklist. Available at https://atm.amegroups.com/article/view/10.21037/atm-22-5764/rc
Conflicts of Interest: All authors have completed the ICMJE uniform disclosure form (available at https://atm.amegroups.com/article/view/10.21037/atm-22-5764/coif). The authors have no conflicts of interest to declare.
Ethical Statement: The authors are accountable for all aspects of the work in ensuring that questions related to the accuracy or integrity of any part of the work are appropriately investigated and resolved. The study was conducted in accordance with the Declaration of Helsinki (as revised in 2013).
Open Access Statement: This is an Open Access article distributed in accordance with the Creative Commons Attribution-NonCommercial-NoDerivs 4.0 International License (CC BY-NC-ND 4.0), which permits the non-commercial replication and distribution of the article with the strict proviso that no changes or edits are made and the original work is properly cited (including links to both the formal publication through the relevant DOI and the license). See: https://creativecommons.org/licenses/by-nc-nd/4.0/.
ReferencesOther Section
- Bray F, Ferlay J, Soerjomataram I, et al. Global cancer statistics 2018: GLOBOCAN estimates of incidence and mortality worldwide for 36 cancers in 185 countries. CA Cancer J Clin 2018;68:394-424. [Crossref] [PubMed]
- Ding H, Zhang J, Zhang F, et al. Role of Cancer-Associated fibroblast in the pathogenesis of ovarian Cancer: Focus on the latest therapeutic approaches. Int Immunopharmacol 2022;110:109052. [Crossref] [PubMed]
- Tan Z, Huang H, Sun W, et al. Current progress of ferroptosis study in ovarian cancer. Front Mol Biosci 2022;9:966007. [Crossref] [PubMed]
- O'Connell C, VandenHeuvel S, Kamat A, et al. The Proteolytic Landscape of Ovarian Cancer: Applications in Nanomedicine. Int J Mol Sci 2022;23:9981. [Crossref] [PubMed]
- Stewart C, Ralyea C, Lockwood S. Ovarian Cancer: An Integrated Review. Semin Oncol Nurs 2019;35:151-6. [Crossref] [PubMed]
- Caro AA, Deschoemaeker S, Allonsius L, et al. Dendritic Cell Vaccines: A Promising Approach in the Fight against Ovarian Cancer. Cancers (Basel) 2022;14:4037. [Crossref] [PubMed]
- Rosario R, Cui W, Anderson RA. Potential ovarian toxicity and infertility risk following targeted anti-cancer therapies. Reprod Fertil 2022;3:R147-62. [Crossref] [PubMed]
- Pan Y, Liu G, Zhou F, et al. DNA methylation profiles in cancer diagnosis and therapeutics. Clin Exp Med 2018;18:1-14. [Crossref] [PubMed]
- Koch A, Joosten SC, Feng Z, et al. Analysis of DNA methylation in cancer: location revisited. Nat Rev Clin Oncol 2018;15:459-66. [Crossref] [PubMed]
- Roy D, Tiirikainen M. Diagnostic Power of DNA Methylation Classifiers for Early Detection of Cancer. Trends Cancer 2020;6:78-81. [Crossref] [PubMed]
- Palomeras S, Diaz-Lagares Á, Viñas G, et al. Epigenetic silencing of TGFBI confers resistance to trastuzumab in human breast cancer. Breast Cancer Res 2019;21:79. [Crossref] [PubMed]
- Tian J, Xu YY, Li L, et al. MiR-490-3p sensitizes ovarian cancer cells to cisplatin by directly targeting ABCC2. Am J Transl Res 2017;9:1127-38. [PubMed]
- Ying Y, Tao Q. Epigenetic disruption of the WNT/ß-catenin signaling pathway in human cancers. Epigenetics 2009;4:307-12. [Crossref] [PubMed]
- Qian XJ, Li YT, Yu Y, et al. Inhibition of DNA methyltransferase as a novel therapeutic strategy to overcome acquired resistance to dual PI3K/mTOR inhibitors. Oncotarget 2015;6:5134-46. [Crossref] [PubMed]
- Romero-Garcia S, Prado-Garcia H, Carlos-Reyes A. Role of DNA Methylation in the Resistance to Therapy in Solid Tumors. Front Oncol 2020;10:1152. [Crossref] [PubMed]
- Jung H, Kim HS, Kim JY, et al. DNA methylation loss promotes immune evasion of tumours with high mutation and copy number load. Nat Commun 2019;10:4278. [Crossref] [PubMed]
- Lønning PE, Knappskog S. Mapping genetic alterations causing chemoresistance in cancer: identifying the roads by tracking the drivers. Oncogene 2013;32:5315-30. [Crossref] [PubMed]
- Knappskog S, Chrisanthar R, Løkkevik E, et al. Low expression levels of ATM may substitute for CHEK2 /TP53 mutations predicting resistance towards anthracycline and mitomycin chemotherapy in breast cancer. Breast Cancer Res 2012;14:R47. [Crossref] [PubMed]
- Lane DP, Cheok CF, Lain S. p53-based cancer therapy. Cold Spring Harb Perspect Biol 2010;2:a001222. [Crossref] [PubMed]
- Zafon C, Gil J, Pérez-González B, et al. DNA methylation in thyroid cancer. Endocr Relat Cancer 2019;26:R415-39. [Crossref] [PubMed]
- Papanicolau-Sengos A, Aldape K. DNA Methylation Profiling: An Emerging Paradigm for Cancer Diagnosis. Annu Rev Pathol 2022;17:295-321. [Crossref] [PubMed]
- Dong M, Yang Z, Li X, et al. Screening of Methylation Gene Sites as Prognostic Signature in Lung Adenocarcinoma. Yonsei Med J 2020;61:1013-23. [Crossref] [PubMed]
- Bisarro Dos Reis M, Barros-Filho MC, Marchi FA, et al. Prognostic Classifier Based on Genome-Wide DNA Methylation Profiling in Well-Differentiated Thyroid Tumors. J Clin Endocrinol Metab 2017;102:4089-99. [Crossref] [PubMed]
- Ning W, Li S, Tsering J, et al. Protective Effect of Triphala against Oxidative Stress-Induced Neurotoxicity. Biomed Res Int 2021;2021:6674988. [Crossref] [PubMed]
- Ning W, Jiang X, Sun Z, et al. Identification of the Potential Biomarkers Involved in the Human Oral Mucosal Wound Healing: A Bioinformatic Study. BioMed Research International 2021;2021:6695245. [Crossref]
- Ritchie ME, Phipson B, Wu D, et al. limma powers differential expression analyses for RNA-sequencing and microarray studies. Nucleic Acids Res 2015;43:e47. [Crossref] [PubMed]
- Yu G, Wang LG, Han Y, et al. clusterProfiler: an R package for comparing biological themes among gene clusters. OMICS 2012;16:284-7. [Crossref] [PubMed]
- Zhou G, Soufan O, Ewald J, et al. NetworkAnalyst 3.0: a visual analytics platform for comprehensive gene expression profiling and meta-analysis. Nucleic Acids Res 2019;47:W234-41. [Crossref] [PubMed]
- Eberhardt J, Santos-Martins D, Tillack AF, et al. AutoDock Vina 1.2.0: New Docking Methods, Expanded Force Field, and Python Bindings. J Chem Inf Model 2021;61:3891-8. [Crossref] [PubMed]
- O'Boyle NM, Banck M, James CA, et al. Open Babel: An open chemical toolbox. J Cheminform 2011;3:33. [Crossref] [PubMed]
- Seeliger D, de Groot BL. Ligand docking and binding site analysis with PyMOL and Autodock/Vina. J Comput Aided Mol Des 2010;24:417-22. [Crossref] [PubMed]
- Vanommeslaeghe K, Hatcher E, Acharya C, et al. CHARMM general force field: A force field for drug-like molecules compatible with the CHARMM all-atom additive biological force fields. J Comput Chem 2010;31:671-90. [PubMed]
- Shen W, Song Z, Xiao Z, et al. Sangerbox: A comprehensive, interaction-friendly clinical bioinformatics analysis platform. iMeta 2022;1:e36. [Crossref]
- Dvorská D, Braný D, Nagy B, et al. Aberrant Methylation Status of Tumour Suppressor Genes in Ovarian Cancer Tissue and Paired Plasma Samples. Int J Mol Sci 2019;20:4119. [Crossref] [PubMed]
- Lakshminarasimhan R, Liang G. The Role of DNA Methylation in Cancer. Adv Exp Med Biol 2016;945:151-72. [Crossref] [PubMed]
- Fan S, Chi W. Methods for genome-wide DNA methylation analysis in human cancer. Brief Funct Genomics 2016;15:432-42. [Crossref] [PubMed]
- Poduval DB, Ognedal E, Sichmanova Z, et al. Assessment of tumor suppressor promoter methylation in healthy individuals. Clin Epigenetics 2020;12:131. [Crossref] [PubMed]
- Crunkhorn S. Deep learning framework for repurposing drugs. Nat Rev Drug Discov 2021;20:100. [PubMed]
- Issa NT, Stathias V, Schürer S, et al. Machine and deep learning approaches for cancer drug repurposing. Semin Cancer Biol 2021;68:132-42. [Crossref] [PubMed]
- Wang Y, Fang T, Wang Y, et al. Impact of AADAC gene expression on prognosis in patients with Borrmann type III advanced gastric cancer. BMC Cancer 2022;22:635. [Crossref] [PubMed]
- Wang H, Wang D, Gu T, et al. AADAC promotes therapeutic activity of cisplatin and imatinib against ovarian cancer cells. Histol Histopathol 2022;37:899-907. [PubMed]
- Gao Q, Hou L, Wang H, et al. DACT3 has a tumor-inhibiting role in acute myeloid leukemia via the suppression of Wnt/β-catenin signaling by DVL2. J Biochem Mol Toxicol 2022;36:e23014. [Crossref] [PubMed]
- Clauss A, Ng V, Liu J, et al. Overexpression of elafin in ovarian carcinoma is driven by genomic gains and activation of the nuclear factor kappaB pathway and is associated with poor overall survival. Neoplasia 2010;12:161-72. [Crossref] [PubMed]
(English Language Editor: A. Kassem)