Regional peak flow as a novel approach to assess regional pulmonary mechanics by electrical impedance tomography: an observational validation study
Highlight box
Key findings
• Regional lung mechanics can be adequately quantified in spontaneous mechanical ventilation by a regional peak flow approach using electrical impedance tomography
What is known and what is new?
• Currently available electrical impedance tomography algorithms are not applicable for quantifying regional lung mechanics in patients on spontaneous mechanical ventilation;
• This manuscript presents the development and validation of the regional peak flow algorithm for quantifying regional lung mechanics by electrical impedance tomography applicable in patients on spontaneous mechanical ventilation.
What is the implication, and what should change now?
• The regional peak flow algorithm using electrical impedance tomography can be used to adequately quantify regional lung mechanics. This algorithm can now be used for titrating positive end-expiratory pressure settings in spontaneous mechanical ventilation modes.
Introduction
Spontaneous efforts during invasive mechanical ventilation in acute respiratory distress syndrome (ARDS) are widely recognized for improving oxygenation and preserving muscle function (1). Weaning from mechanical ventilation can be conducted through spontaneous mechanical ventilation (SMV), where spontaneous breathing efforts are allowed. These efforts may be gentle or vigorous depending on the severity of ARDS. Vigorous efforts are more likely to occur in moderate to severe ARDS and are linked to worsening of the lung and diaphragm injury (2). Higher positive end expiratory pressure (PEEP) levels can be used to decrease the magnitude of these vigorous efforts (3-5).
Electrical impedance tomography (EIT) is a non-invasive and radiation free technique which has the ability to quantify regional pulmonary mechanics in controlled mechanical ventilation (CMV) modes. EIT technology has been studied extensively for titrating PEEP in CMV (6-13). Its use is linked to increase in-hospital survival, decreased length of mechanical ventilation and improved weaning success (14,15). Based on the above-mentioned studies, it can be hypothesized that EIT-based PEEP titration is also clinically beneficial during weaning from mechanical ventilation.
The drawback of currently available algorithms is that their use is limited to CMV because it requires an equally distributed airway pressure phase which occurs during the plateau pressure phase after end-inspiration. In SMV, stable plateau phases are not always present, which would result in inaccurate quantification of regional pulmonary mechanics calculations (16). Therefore, a new EIT algorithm could be helpful for titrating PEEP in SMV if it can assess regional pulmonary mechanics (i.e., cumulative overdistension and cumulative collapse rates) without extra bedside actions. We, therefore, aim to present and validate an automatic EIT algorithm for quantifying regional pulmonary mechanics based on a regional peak flow (RPF) approach, which is independent of the plateau pressure phase and can be applied on a tidal-to-tidal basis. In this study, we use EIT data from patients on pressure-controlled mechanical ventilation from a prospective cohort study, which will provide evidence of whether a new EIT algorithm for SMV is possible and can be investigated in future studies. We present the following article in accordance with the STROBE reporting checklist (available at https://atm.amegroups.com/article/view/10.21037/atm-22-3420/rc).
Methods
Design
We used data from the prospective Maastricht Intensive Care Covid Cohort, a comprehensive clinical variable collection on mechanically ventilated coronavirus disease 2019 (COVID-19) patients. The study was approved by the local medical ethics review committee of Clinical Trial Center Maastricht (No. 2020-1565) and registered in the Netherlands Trial Register (No. NL8613). The study was conducted in accordance with the Declaration of Helsinki (as revised in 2013) (17). Informed consent was obtained from each patient or next of kin prior to inclusion in this study.
From March 2020 to May 2020, adults (≥18 years) with a COVID-19 Reporting and Data System (CO-RADS) score (a categorical assessment for pulmonary involvement of COVID-19 at unenhanced chest computed tomography) of 4 and 5 or PCR proven COVID-19 infection, who were mechanically ventilated using pressure-controlled ventilation and who were subjected to an EIT measurement at the intensive care unit (ICU) of the Maastricht University Medical Center+ were included in this study (18). Contra-indications for performing EIT within the cohort are: body mass index (BMI) >50 kg/m2, pneumothorax, cerebral lesions, pneumectomy, bronchial bleeding, pleural catheters, rib fractures, chest wounds with or without bandage, mobilization restrictions, internal or external pacemakers or implantable cardioverter-defibrillator, Richmond Agitation Sedation Scale >−5 or a bronchial alveolar lavage less than 24 hours before EIT.
The protocol used during an EIT measurement is described earlier in the supplemental material of Tas et al. and is summarized in Figure 1 (17). It comprises a stepwise incremental PEEP trial from baseline PEEP and a subsequent stepwise decremental PEEP trial from the upper limit in 2 cmH2O steps taking approximately 30 to 60 seconds, preferably starting at a PEEP of 24 cmH2O. Other mechanical ventilation settings such as the driving pressure, inspiratory and expiratory time, inspiratory rise time, respiratory rate and the fraction of inspired oxygen remained constant during the EIT measurement. Due to each inclusion’s individual clinical status, the number of steps within the PEEP trial may have varied. The upper limit of the incremental PEEP trial was reached at the PEEP level where either solely regional compliance loss (i.e., alveolar overdistension) and no further recruitment was measured, as assessed by EIT or when the peripheral oxygen saturation declined below 88%. The lower limit for the decremental trial was reached at the PEEP level where significant regional compliance loss (i.e., alveolar collapse) is measured, as assessed by EIT or when the respiratory system dynamic compliance declines or when the peripheral oxygen saturation declined below 88%. Although the MaastrICCht cohort included serial EIT measurements per patient, only the first EIT measurement of an included patient is used for validation analyses in the present investigation for simple and easy to interpret agreement analyses.
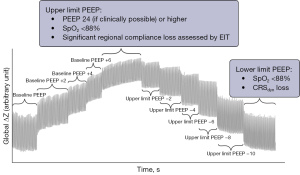
Data acquisition and pre-processing
EIT measurements are recorded with the Pulmovista 500 (Dräger Medical, Lübeck, Germany) with a sampling frequency of 20 Hz. First, the raw EIT data files from the Pulmovista 500 (continuous regional relative impedances with a spatial resolution of 32×32 pixels) are converted to MATLAB (The MathWorks Inc., Natick, MA, USA) readable files using Dräger’s “Data Analysis Tool 6.3”. Subsequently, the raw regional impedance data are filtered using a bi-directional fourth order 0.83 Hz low-pass Butterworth filter for removing cardiac activity as much as possible without phase shifting relevant ventilation information.
Automatic EIT algorithm for regional lung mechanics
In the RPF algorithm, cumulative overdistension and cumulative collapse rates are quantified, similar to Costa’s algorithm (6). However, instead of calculating these rates based on regional compliances, cumulative overdistension and cumulative collapse rates are calculated based on RPF (i.e., ODRPF and CLRPF). Under the assumption that regional peak airflow explains regional pulmonary compliance well, ODRPF and CLRPF may be used in SMV in the future. Analyses prior to calculating ODRPF and CLRPF, include: the determination of the aerated lung area, segmentation of each breath within all separate aerated pixels, calculation of the RPF within all separate aerated pixels per PEEP level, subsequent regional overdistension and collapse calculation and a factor four cubic interpolation of the regional overdistension and collapse data maps. The above-mentioned steps are illustrated in Figure 2 and full technical details of the algorithm are described in the supplemental material (Appendix 1). Last, the cumulative overdistension and collapse rate are calculated by a weighted average approach per PEEP level identically to Costa’s algorithm, which is also described in the supplemental material (6).
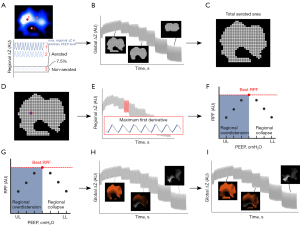
Validation
To validate the RPF approach, RPF-based cumulative overdistension and collapse outcomes are compared to the compliance-based cumulative overdistension and collapse outcomes by the Pulmovista 500 device (i.e., ODP500 & CLP500) in multiple PEEP levels (PEEP 10 to PEEP 24) during pressure-controlled mechanical ventilation (6). Although the MaastrICCht cohort included serial EIT measurements during pressure-controlled mechanical ventilation per patient, only the first EIT measurement of an included patient is used for validation analyses in the present investigation for simple and easy to interpret agreement analyses.
Statistical analysis
Statistical analyses are performed in MATLAB. Study population characteristics and baseline ventilation variables prior to the EIT measurement are presented in mean [± standard deviation (SD)] for continuous variables, while categorical variables are presented in frequencies (%). Interrater associations of ODRPF & ODP500 rates and between CLRPF & CLP500 rates during a descending-PEEP trial are assessed by a two-level linear mixed effects model with a random intercept and random slope:
Where Yij represents ODP500 or CLP500, β0 the fixed effect intercept estimate for either cumulative overdistension and collapse with a random effect β0i, β1 the fixed effect estimate for either ODRPF or CLRPF with a random effect β1i, Xij either ODRPF or CLRPF and εij the model residuals (19). Both the random intercept and random slope are grouped by subject. A fixed effect coefficient for the input variable β0i and fixed effect coefficient for the intercept β0 close to 1.00 and 0.00 for both cumulative overdistension and collapse, respectively, is aimed for. Also, for both cumulative overdistension and collapse outcomes, the interrater agreement for each separate PEEP level is assessed by classic Bland-Altman analyses and intraclass correlation coefficient (ICC) using a single measurement-based two-way mixed absolute agreement model, as described by McGraw et al. (20-22). We considered a P value <0.05 statistically significant.
Results
Of all mechanically ventilated patients (n=98) admitted to the ICU during the given study period, 94 patients were mechanically ventilated primarily for COVID-19. Due to either the absence of ventilator data from the first EIT measurement or the absence of an EIT measurement or missing EIT data due to not recording, no RPF analysis was performed in 16 out of 94 patients, resulting in a total of 78 included patients for analysis (Figure 3). The average age and BMI were 63.9 years (±10.5 years) and 27.8 kg/m2 (±4.3 kg/m2), respectively. In total, 61 (78.2%) of the inclusions were male. In addition, 5.1% of the inclusions suffered from chronic lung disease prior to ICU admission. At ICU admission, the average APACHE II and SOFA score was 16.3 (±3.7) and 7.6 (±2.8) points, respectively. On average, the first EIT measurement was performed three days after intubation. Table 1 summarizes the patient characteristics, including their average clinical and mechanical ventilation status prior to the EIT measurement. The 16 excluded patients did not differ from the 78 included in terms of age, gender, APACHE II score and BMI except for the number of patients suffering from chronic lung disease prior to ICU admission (Table S1).
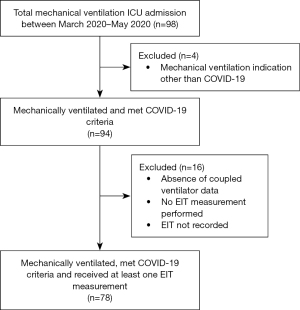
Table 1
Variables | Mean (± SD) or frequencies (%) |
---|---|
Patient characteristics (n=78) | |
Age, years | 63.9 (±10.5) |
Male | 61 (78.2%) |
Body mass index, kg/m2 | 27.8 (±4.3) |
Chronic lung disease | 4 (5.1%) |
APACHE II score, points | 16.3 (±3.7) |
SOFA score, points | 7.6 (±2.8) |
Day of EIT measurement†, days | 3.0 (±2.9) |
Baseline ventilation variables‡ | |
FiO2, % | 53.4 (±15.3) |
Respiratory rate, minute−1 | 22.2 (±4.0) |
Peak inspiratory pressure§, cmH2O | 25.4 (±3.6) |
Positive end-expiratory pressure, cmH2O | 13.8 (±2.4) |
Respiratory system dynamic compliance, mL/cmH2O | 44.9 (±16.3) |
PaO2/FiO2 ratio, mmHg | 171 (±60.0) |
Tidal volume, mL/kg PBW | 5.4 (±1.2) |
Arterial blood gas oxygen (PaO2), mmHg | 88.7 (±22.7) |
Arterial blood gas carbon dioxide (PaCO2), mmHg | 42.7 (±7.5) |
Arterial blood gas pH | 7.53 (±0.09) |
†, day 1 is considered the first day of intubation; ‡, latest prior to the first EIT measurement; §, peak inspiratory pressure is equal to the positive end expiratory pressure plus the driving pressure. APACHE, Acute Physiology and Chronic Health Evaluation; EIT, electrical impedance tomography; FiO2, fraction of inspired oxygen; kg, kilogram; mL, milliliters; N, total number of observations; PaCO2, arterial partial pressure of carbon dioxide; PaO2, arterial partial pressure of oxygen; PBW, predicted bodyweight; SD, standard deviation; SOFA, Sequential Organ Failure Assessment.
In the mixed model for cumulative overdistension (Table 2), the estimates for the intercept and the coefficient for ODRPF are 3.05% (95% CI: 2.31–3.78%, P<0.001) and 1.02 (95% CI: 0.98–1.07, P<0.001), respectively. The variance within ODP500 can be explained by ODRPF for 95.0%. ICC outcomes per PEEP level fall within the range of 0.78 and 0.86 (P<0.001) and the bias across all PEEP level ranges from 0.80% to 4.19% on average (Table 3).
Table 2
Fixed effects | Linear mixed effect model OD (R2=0.95) | ||
---|---|---|---|
Estimate | 95% CI | P value | |
Intercept (%) | 3.05 | 2.31–3.78 | <0.001 |
ODRPF | 1.02 | 0.98–1.07 | <0.001 |
CI, confidence interval; OD, overdistension; P500, Pulmovista-based algorithm; RPF, regional peak flow.
Table 3
PEEP (cmH2O) | N | Interrater analysis OD | ||
---|---|---|---|---|
ICC (95% CI) | P value | Bias (LoA) | ||
10 | 32 | 0.83 (0.67–0.92) | <0.001 | 0.80% (−3.63%; 5.23%) |
12 | 58 | 0.84 (0.71–0.91) | <0.001 | 1.33% (−4.57%; 7.24%) |
14 | 69 | 0.86 (0.71–0.92) | <0.001 | 1.92% (−5.12%; 8.98%) |
16 | 74 | 0.80 (0.58–0.90) | <0.001 | 3.15% (−7.10%; 13.40%) |
18 | 77 | 0.84 (0.57–0.93) | <0.001 | 3.81% (−6.55%; 14.19%) |
20 | 77 | 0.81 (0.68–0.89) | <0.001 | 3.17% (−11.10%; 17.43%) |
22 | 73 | 0.78 (0.59–0.87) | <0.001 | 3.80% (−10.62%; 18.22%) |
24 | 67 | 0.78 (0.57–0.88) | <0.001 | 4.19% (−10.80%; 19.18%) |
RPF, regional peak flow; P500, Pulmovista-based algorithm; PEEP, positive end expiratory pressure; N, total number of observations in specific PEEP level; ICC, intra-class correlation; CI, confidence interval; OD, cumulative overdistension rate; LoA, limits of agreement.
In the mixed model for cumulative collapse (Table 4), the estimates for the intercept and the coefficient for CLRPF are 0.29% (0.14–0.44%, P<0.001) and 0.93 (0.80–1.05, P<0.001), respectively. The variance within CLP500 can be explained by CLRPF for 92.0%. ICC values for CL fall within the range of 0.70 to 0.85 (P<0.001), and the bias ranges from −1.31% to 0.13% on average (Table 5). The supplemental material (Figures S1,S2) contains the Bland-Altman plots in all PEEP levels for both cumulative overdistension and cumulative collapse outcomes.
Table 4
Fixed effects | Linear mixed effect model CL (R2=0.92) | ||
---|---|---|---|
Estimate | 95% CI | P value | |
Intercept (%) | 0.29 | 0.14–0.44 | <0.001 |
CLRPF | 0.93 | 0.80–1.05 | <0.001 |
RPF, regional peak flow deviation; P500, Pulmovista-based algorithm; CL, cumulative collapse rate; CI, confidence interval.
Table 5
PEEP (cmH2O) | N | Interrater analysis CL | ||
---|---|---|---|---|
ICC (95% CI) | P value | Bias (LoA) | ||
10 | 32 | 0.84 (0.68–0.92) | <0.001 | −1.31% (−8.22%; 5.58%) |
12 | 58 | 0.85 (0.76–0.91) | <0.001 | −0.75% (−9.69%; 8.20%) |
14 | 69 | 0.82 (0.72–0.88) | <0.001 | −0.67% (−8.11%; 6.76%) |
16 | 74 | 0.71 (0.57–0.81) | <0.001 | 0.13% (−7.55%; 7.81%) |
18 | 77 | 0.80 (0.70–0.87) | <0.001 | −0.10% (−4.74%; 4.54%) |
20 | 77 | 0.81 (0.72–0.88) | <0.001 | −0.28% (−3.16%; 2.60%) |
22 | 73 | 0.78 (0.67–0.85) | <0.001 | −0.05% (−1.83%; 1.73%) |
24 | 67 | 0.70 (0.55–0.80) | <0.001 | 0.01% (−0.87%; 0.89%) |
RPF, regional peak flow; P500, Pulmovista-based algorithm; PEEP, positive end expiratory pressure; N, total number of observations in specific PEEP level; CL, cumulative collapse rate; ICC, intra-class correlation; CI, confidence interval; LoA, limits of agreement.
Discussion
Taken together, the results of this study imply that the quantification of regional lung mechanics based on RPF in patients on CMV patients suffering from COVID-19 related ARDS is valid. This, in combination with the independence from a plateau pressure phase and the fact that no additional bedside actions are required, suggests that the RPF algorithm is applicable for testing in SMV. Eventually, this approach may quantify regional lung mechanics during SMV to optimize EIT-driven weaning strategies.
Monitoring regional lung mechanics
Several studies have investigated the effect of different pressure settings on ventilation homogeneity and lung inflation in SMV using EIT since EIT in CMV showed promising results. Blankman et al. and Mauri et al. found that increased PEEP during pressure support ventilation (PSV) results in more homogeneous ventilation and a better arterial partial pressure of oxygen (PaO2)/fraction of inspired oxygen (FiO2)-ratio in humans (23,24). However, these studies rather focussed on ventilation distributional parameters than on regional lung mechanics. Another study by Mauri et al. designed an algorithm describing regional overdistension for the dependent and non-dependent lung regions (25). They used the global tidal volume from the ventilator to calculate dorsal and ventral compliance under the assumption that the measured aerated area by EIT represents the complete lung, which may lead to an affected calculation of true regional compliances. Also, all the above-mentioned studies did not consider the collapse phenomenon, a fundamental concept in ARDS lungs and in SMV patients when end-expiratory transpulmonary pressures are negative (26). Becher et al. proposed an EIT-based algorithm to determine regional compliance in PSV (27). They used a low-flow ventilation approach during PSV to design a quasi-static pressure-volume (PV) loop and subsequently calculated the slope of the PV-loop (i.e., compliance). In some cases, this may be an unwise approach, as a patient may not tolerate such a low-flow procedure, especially during a PEEP trial. Inspiratory hold manoeuvres during SMV have been proposed earlier to monitor global pulmonary compliance and could be used to detect plateau pressure phases during SMV, indicating that Costa’s compliance-based EIT algorithm can be used to quantify regional pulmonary mechanics in SMV (28). However, this requires multiple inspiratory hold manoeuvres per PEEP level during a PEEP trial to adequately quantify regional pulmonary compliance. Moreover, the patient must be passive during the hold manoeuvre, which is not always possible in SMV (29). Apart from the above-mentioned studies, studies on quantifying regional pulmonary mechanics in SMV using EIT remain scarce.
Validation
The present results show that the association between the new RPF and the previous compliance approach for both cumulative overdistension and collapse is good. Also, the R2 of both overdistension and collapse linear mixed models are high, indicating the limited influence on the variance within ODRPF and CLRPF by other physiological processes. The ranking is similarly strong, with ICC values between 0.70 to 0.86 for PEEP 10 to PEEP 24, which is considered moderate to good agreement (21). The 95% confidence interval for the ICC is large in all PEEP levels and large in most Bland-Altman plots for both cumulative overdistension and collapse, possibly resulting from the relatively small sample size in this study.
As seen in the Bland-Altman plots in the supplemental material (Figures S1,S2), the limits of agreement of cumulative overdistension shrink as PEEP lowers, while this is the opposite for cumulative collapse. In parallel, the ICC value for both cumulative overdistension and collapse remain relatively equal, indicating that the difference in physiological principles of RPF and regional compliance plays a role in the shrinkage and expansion of the limits of agreement. We believe this has to do with the airway resistance, which increases when excessive or insufficient PEEP is applied, resulting in an altered relationship between regional airflow and regional lung compliance. Currently, regional airway resistance cannot be monitored, meaning no correction on its effects on ODRPF and CLRPF can be performed.
In clinical practice, EIT-based PEEP titration in CMV can be determined using a pre-defined threshold (i.e., maximum of 5% cumulative collapse rate or the intersection of the cumulative overdistension and collapse curve) using the Costa’s compliance-based approach (6). When using the RPF approach in SMV, the quantification of cumulative overdistension and collapse values may slightly deviate, resulting in different clinical decision making (i.e., higher PEEP or lower PEEP) at the bedside compared to Costa’s algorithm. However, for SMV, the RPF approach is an acceptable alternative since Costa’s algorithm is not applicable in SMV. Further research should point out the clinical added value of the RPF algorithm in daily clinical practice for patients on SMV.
Limitations
At present, there is no golden standard for determining regional compliance of lung tissue. Right now, EIT is considered to be the best option for this purpose. Still, EIT comes with reoccurring limitations, such as regional mechanics using RPF-based regional relative impedance data instead of absolute flows as measured by the ventilator. Regional impedances and regional volumes are different physical concepts, but Mauri et al. described high correlations between EIT-derived peak flow and spirometry-based peak flow, meaning that the RPF approach is physiologically valid in terms of using air flow for determining regional lung mechanic parameters (30). Also, impedance changes occurring distally from the electrode band to a lesser extent contribute to the generation of EIT images which may lead to the over- or underestimation of regional airflow at the plane of the electrode band (8). Last, the spatial resolution of EIT (32×32 pixels) is low compared to other imaging modalities, possibly over- or under-estimating small regional differences in lung mechanics. At present, for clinical purposes, a spatial resolution of 32×32 is considered most feasible (31).
Costa et al. were the first to validate an EIT-based overdistension and collapse algorithm by comparing regional overdistension and collapse maps with computed tomography images (6). However, due to the observational study design and the critical setting of the patients, computed tomography imaging parallel to EIT was not performed in this study. Therefore, we assumed the EIT algorithm of Costa et al. to be the “gold standard” for the clinical validation of the RPF algorithm.
There are no clear rules for determining the aerated lung area in EIT, which may have led to overdistension and collapse outcome deviations compared to Costa’s algorithm. As a result, the confidence intervals for the mixed model estimates and Bland-Altman analyses may be overestimated. Furthermore, especially in lung-protective ventilation, where PEEP should be minimized as much as possible, a lack of consensus regarding a threshold for calculating the total aerated area in EIT may influence cumulative overdistension and collapse calculations and thus clinical decision-making regarding PEEP at the bedside significantly. Therefore, clear guidelines for determining the aerated lung area need to be established in the future to make the comparison of EIT research more reliable and the clinical use of EIT in mechanically ventilated patients more united.
Clinical implications
At present, a standardized approach for personalized PEEP in SMV is lacking. Current PEEP strategies are partially subjective and based on global lung parameters, such as the airway occlusion pressure (which is considered a surrogate for work of breathing), tidal volumes, and oxygenation status (32-35). These approaches may ultimately lead to suboptimal ventilatory strategies and potentially unnecessary lung injury. PEEP titration by EIT in ARDS in CMV has shown promising results regarding ventilator weaning success (14,15). The advantage of EIT in CMV and the results of the present study suggest that EIT-driven PEEP titration in SMV may be beneficial. However, this remains to be studied in the future.
Conclusions
In conclusion, we developed and validated a bedside EIT tool to quantify regional lung mechanics in patients on CMV that is independent from the plateau pressure phase. It showed good agreement with a currently commercially available tool. The RPF algorithm should therefore enable a valid quantification of regional lung mechanics in SMV and may help clinicians to optimize mechanical ventilator settings at the bedside in this population.
Acknowledgments
The authors thank all ICU personnel who helped us with performing EIT measurements.
Funding: None.
Footnote
Reporting Checklist: The authors have completed the STROBE reporting checklist. Available at https://atm.amegroups.com/article/view/10.21037/atm-22-3420/rc
Data Sharing Statement: Available at https://atm.amegroups.com/article/view/10.21037/atm-22-3420/dss
Peer Review File: Available at https://atm.amegroups.com/article/view/10.21037/atm-22-3420/prf
Conflicts of Interest: All authors have completed the ICMJE uniform disclosure form (Available at https://atm.amegroups.com/article/view/10.21037/atm-22-3420/coif). SAMdJ, SJHH and DCJJB are awaiting decision from the European patent office with regard to the algorithm, as described in the present manuscript. FHCdJ serves as an unpaid Assessment Director of the European Respiratory Society. The other authors have no conflicts of interest to declare.
Ethical Statement: The authors are accountable for all aspects of the work in ensuring that questions related to the accuracy or integrity of any part of the work are appropriately investigated and resolved. The study was conducted in accordance with the Declaration of Helsinki (as revised in 2013). The study was approved by local medical ethics committee of the Clinical Trial Center Maastricht (No. 2020-1565) and registered in the Netherlands Trial Register (No. NL8613). Informed consent was obtained from each patient or next of kin prior to inclusion in this study.
Open Access Statement: This is an Open Access article distributed in accordance with the Creative Commons Attribution-NonCommercial-NoDerivs 4.0 International License (CC BY-NC-ND 4.0), which permits the non-commercial replication and distribution of the article with the strict proviso that no changes or edits are made and the original work is properly cited (including links to both the formal publication through the relevant DOI and the license). See: https://creativecommons.org/licenses/by-nc-nd/4.0/.
References
- Wawrzeniak IC, Regina Rios Vieira S, Almeida Victorino J. Weaning from Mechanical Ventilation in ARDS: Aspects to Think about for Better Understanding, Evaluation, and Management. Biomed Res Int 2018;2018:5423639. [Crossref] [PubMed]
- Yoshida T, Amato MBP, Kavanagh BP, et al. Impact of spontaneous breathing during mechanical ventilation in acute respiratory distress syndrome. Curr Opin Crit Care 2019;25:192-8. [Crossref] [PubMed]
- Yoshida T, Roldan R, Beraldo MA, et al. Spontaneous Effort During Mechanical Ventilation: Maximal Injury With Less Positive End-Expiratory Pressure. Crit Care Med 2016;44:e678-88. [Crossref] [PubMed]
- Morais CCA, Koyama Y, Yoshida T, et al. High Positive End-Expiratory Pressure Renders Spontaneous Effort Noninjurious. Am J Respir Crit Care Med 2018;197:1285-96. [Crossref] [PubMed]
- Magalhães PAF, Padilha GA, Moraes L, et al. Effects of pressure support ventilation on ventilator-induced lung injury in mild acute respiratory distress syndrome depend on level of positive end-expiratory pressure: A randomised animal study. Eur J Anaesthesiol 2018;35:298-306. [Crossref] [PubMed]
- Costa EL, Borges JB, Melo A, et al. Bedside estimation of recruitable alveolar collapse and hyperdistension by electrical impedance tomography. Intensive Care Med 2009;35:1132-7. [Crossref] [PubMed]
- Liu S, Tan L, Möller K, et al. Identification of regional overdistension, recruitment and cyclic alveolar collapse with electrical impedance tomography in an experimental ARDS model. Crit Care 2016;20:119. [Crossref] [PubMed]
- Frerichs I, Amato MB, van Kaam AH, et al. Chest electrical impedance tomography examination, data analysis, terminology, clinical use and recommendations: consensus statement of the TRanslational EIT developmeNt stuDy group. Thorax 2017;72:83-93. [Crossref] [PubMed]
- van der Zee P, Somhorst P, Endeman H, et al. Electrical Impedance Tomography for Positive End-Expiratory Pressure Titration in COVID-19-related Acute Respiratory Distress Syndrome. Am J Respir Crit Care Med 2020;202:280-4. [Crossref] [PubMed]
- Pereira SM, Tucci MR, Morais CCA, et al. Individual Positive End-expiratory Pressure Settings Optimize Intraoperative Mechanical Ventilation and Reduce Postoperative Atelectasis. Anesthesiology 2018;129:1070-81. [Crossref] [PubMed]
- Heines SJH, Strauch U, van de Poll MCG, et al. Clinical implementation of electric impedance tomography in the treatment of ARDS: a single centre experience. J Clin Monit Comput 2019;33:291-300. [Crossref] [PubMed]
- Karsten J, Grusnick C, Paarmann H, et al. Positive end-expiratory pressure titration at bedside using electrical impedance tomography in post-operative cardiac surgery patients. Acta Anaesthesiol Scand 2015;59:723-32. [Crossref] [PubMed]
- de Castro Martins T, Sato AK, de Moura FS, et al. A Review of Electrical Impedance Tomography in Lung Applications: Theory and Algorithms for Absolute Images. Annu Rev Control 2019;48:442-71. [Crossref] [PubMed]
- Hsu HJ, Chang HT, Zhao Z, et al. Positive end-expiratory pressure titration with electrical impedance tomography and pressure-volume curve: a randomized trial in moderate to severe ARDS. Physiol Meas 2021;42:014002. [Crossref] [PubMed]
- Zhao Z, Chang MY, Chang MY, et al. Positive end-expiratory pressure titration with electrical impedance tomography and pressure-volume curve in severe acute respiratory distress syndrome. Ann Intensive Care 2019;9:7. [Crossref] [PubMed]
- Becher T, Schädler D, Rostalski P, et al. Determination of respiratory system compliance during pressure support ventilation by small variations of pressure support. J Clin Monit Comput 2018;32:741-51. [Crossref] [PubMed]
- Tas J, van Gassel RJJ, Heines SJH, et al. Serial measurements in COVID-19-induced acute respiratory disease to unravel heterogeneity of the disease course: design of the Maastricht Intensive Care COVID cohort (MaastrICCht). BMJ Open 2020;10:e040175. [Crossref] [PubMed]
- Prokop M, van Everdingen W, van Rees Vellinga T, et al. CO-RADS: A Categorical CT Assessment Scheme for Patients Suspected of Having COVID-19-Definition and Evaluation. Radiology 2020;296:E97-104. [Crossref] [PubMed]
- Monsalves MJ, Bangdiwala AS, Thabane A, et al. LEVEL (Logical Explanations & Visualizations of Estimates in Linear mixed models): recommendations for reporting multilevel data and analyses. BMC Med Res Methodol 2020;20:3. [Crossref] [PubMed]
- McGraw KO, Wong SP. Forming Inferences about Some Intraclass Correlation Coefficients. Psychol Methods 1996;1:30-46. [Crossref]
- Koo TK, Li MY. A Guideline of Selecting and Reporting Intraclass Correlation Coefficients for Reliability Research. J Chiropr Med 2016;15:155-63. [Crossref] [PubMed]
- Altman DG, Bland JM. Measurement in Medicine: The Analysis of Method Comparison Studies. Journal of the Royal Statistical Society. Series D (The Statistician) 1983;32:307-17. [Crossref]
- Mauri T, Bellani G, Confalonieri A, et al. Topographic distribution of tidal ventilation in acute respiratory distress syndrome: effects of positive end-expiratory pressure and pressure support. Crit Care Med 2013;41:1664-73. [Crossref] [PubMed]
- Blankman P, Hasan D, van Mourik MS, et al. Ventilation distribution measured with EIT at varying levels of pressure support and Neurally Adjusted Ventilatory Assist in patients with ALI. Intensive Care Med 2013;39:1057-62. [Crossref] [PubMed]
- Mauri T, Eronia N, Abbruzzese C, et al. Effects of Sigh on Regional Lung Strain and Ventilation Heterogeneity in Acute Respiratory Failure Patients Undergoing Assisted Mechanical Ventilation. Crit Care Med 2015;43:1823-31. [Crossref] [PubMed]
- Umbrello M, Chiumello D. Interpretation of the transpulmonary pressure in the critically ill patient. Ann Transl Med 2018;6:383. [Crossref] [PubMed]
- Becher TH, Bui S, Zick G, et al. Assessment of respiratory system compliance with electrical impedance tomography using a positive end-expiratory pressure wave maneuver during pressure support ventilation: a pilot clinical study. Crit Care 2014;18:679. [Crossref] [PubMed]
- Bellani G, Grassi A, Sosio S, et al. Plateau and driving pressure in the presence of spontaneous breathing. Intensive Care Med 2019;45:97-8. [Crossref] [PubMed]
- Sajjad H, Schmidt GA, Brower RG, et al. Can the Plateau Be Higher Than the Peak Pressure? Ann Am Thorac Soc 2018;15:754-9. [Crossref] [PubMed]
- Mauri T, Spinelli E, Dalla Corte F, et al. Noninvasive assessment of airflows by electrical impedance tomography in intubated hypoxemic patients: an exploratory study. Ann Intensive Care 2019;9:83. [Crossref] [PubMed]
- Chitturi V, Farrukh N. Spatial resolution in electrical impedance tomography: A topical review. J Electr Bioimpedance 2017;8:66-78. [Crossref]
- Pérez J, Dorado JH, Papazian AC, et al. Titration and characteristics of pressure-support ventilation use in Argentina: an online cross-sectional survey study. Rev Bras Ter Intensiva 2020;32:81-91. [Crossref] [PubMed]
- Pletsch-Assuncao R, Caleffi Pereira M, Ferreira JG, et al. Accuracy of Invasive and Noninvasive Parameters for Diagnosing Ventilatory Overassistance During Pressure Support Ventilation. Crit Care Med 2018;46:411-7. [Crossref] [PubMed]
- Goligher EC, Ferguson ND, Brochard LJ. Clinical challenges in mechanical ventilation. Lancet 2016;387:1856-66. [Crossref] [PubMed]
- Madahar P, Beitler JR. Emerging concepts in ventilation-induced lung injury. F1000Res 2020;9:eF1000 Faculty Rev-222.