Elevated ADAM-like Decysin-1 (ADAMDEC1) expression is associated with increased chemo-sensitivity and improved prognosis in breast cancer patients
Highlight box
Key findings
• We identified a novel indicator associated with the effect of chemotherapy as well as breast cancer prognosis.
What is known and what is new?
• The response to neoadjuvant chemotherapy is associated with breast cancer survival. The resistance to paclitaxel and anthracyclines is closely related to therapeutic failure and death.
• We identified that high expression of ADAMDEC1 was associated with the sensitivity of chemotherapy and favorable prognosis in breast cancer. The underlying mechanisms of chemo-response were also investigated
What is the implication, and what should change now?
• We can identify patients with chemosensitive tumors in advance by ADAMDEC1 expression, which provide useful information for therapeutic decision-making. We also provide novel insights into the mechanisms of chemo-response by exploring the regulatory mechanism of ADAMDEC1 expression. Experimental verification is needed to make the data more convincing.
Introduction
Breast cancer is the most common malignant tumor and the leading cause of cancer-related death in females worldwide. Over the past few decades, although its incidence has increased, the mortality rate of breast cancer has declined significantly, mainly due to early detection and the development of systemic treatments (1). Neoadjuvant chemotherapy is well-known for its importance in the treatment of high-grade, large-size, and advanced tumors. It can improve the operability of patients and make breast-conserving surgery more feasible (2). Neoadjuvant therapy has become one of the most recommended treatments, especially in triple-negative breast cancer (TNBC) (3).
TNBC, which is the most aggressive subtype of breast cancer, is characterized by the negative expression of estrogen receptor (ER), progesterone receptor (PR), and human epidermal growth factor receptor 2 (HER2) (4). It is associated with poor prognosis owing to its easy recurrence, easy metastasis, and lack of therapeutic targets (5). Neoadjuvant taxane and anthracycline-based regimens are still the common standard treatments for patients with TNBC (6). However, resistance to paclitaxel and anthracyclines is often observed in TNBC patients during chemotherapy, which is closely related to therapeutic failure and death (7). Additionally, it causes a high risk of recurrence and metastasis despite adjuvant treatment (8). Due to the adverse effects of chemotherapy and the limited survival time of TNBC patients, it is necessary to identify patients with chemosensitive tumors in advance using reliable biomarkers, which might provide useful information for therapeutic decision-making. Moreover, since taxane and anthracycline-based regimens are also the basic chemotherapeutic agents utilized in Luminal and HER2-enriched breast cancers, this investigation may lead to a better understanding of chemoresistance in all breast cancer subtypes.
In this study, we explored three datasets (GSE34138, GSE20271, and GSE32646) obtained from the Gene Expression Omnibus (GEO) database. Tumor samples were all derived from TNBC patients who were treated with doxorubicin/cyclophosphamide (AC), fluorouracil/doxorubicin/cyclophosphamide (FAC), fluorouracil/epirubicin/cyclophosphamide (FEC), or paclitaxel followed by 5-fluorouracil/epirubicin/cyclophosphamide (P-FEC). Differentially expressed genes (DEGs) between the chemosensitive and chemoresistant tumors were investigated using the GEO2R tool. Through exploring the overlaps of these DEGs, we finally found that ADAMDEC1 was upregulated among all three chemosensitive groups and there were no co-downregulated genes. Furthermore, we analyzed the potential role of ADAMDEC1 in breast cancer and identified a novel indicator associated with the effect of chemotherapy as well as breast cancer prognosis, and the underlying mechanisms were also investigated using meta-data analysis. We present the following article in accordance with the STREGA reporting checklist (available at https://atm.amegroups.com/article/view/10.21037/atm-22-6319/rc).
Methods
Data source
The gene expression datasets were obtained from the GEO database (https://www.ncbi.nlm.nih.gov/geo/) (9). In this study, we selected three datasets GSE34138, GSE20271, and GSE32646 for further analysis. All datasets contained TNBC samples receiving neoadjuvant taxane or anthracycline chemotherapy, and data about the efficacy of neoadjuvant therapy was available. We identified that pathological complete response (pCR) samples were associated with greater chemotherapy sensitivity, while non-pCR and residual disease (RD) samples were resistant to neoadjuvant chemotherapy. Also, we further investigated the DEGs by comparing chemosensitive and chemoresistant TNBC tumors using the GEO2R online analysis tool. DEGs were defined as genes that met the cut-off criteria of |log2 fold change| >1 (|logFC| >1) and P<0.05. Using the Venn online tool to investigate overlapping DEGs, we further explored the potential role of these genes in breast cancer (bioinformatics.psb.ugent.be/webtools/Venn/). The study was conducted in accordance with the Declaration of Helsinki (as revised in 2013).
The university of Alabama at Birmingham cancer data analysis portal (UALCAN)
UALCAN (http://ualcan.path.uab.edu/analysis.html) is a reliable website that provides a comprehensive analysis based on The Cancer Genome Atlas (TCGA) and MET500 data (10). It was applied to explore the expression level of ADAM-like Decysin-1 (ADAMDEC1) in breast cancer.
LinkedOmics
LinkedOmics (http://www.linkedomics.org/) is a web-based tool that provides multi-omics data analyses within and across 32 TCGA cancer types (11). It was applied in this study to analyze the association between ADAMDEC1 expression and clinical characteristics using the non-parametric test. Next, it was used to explore and visualize ADAMDEC1 and its neighboring molecules using the Pearson correlation test. Based on the above results, we further conducted a Gene Ontology (GO) functional analysis and a Kyoto Encyclopedia of Genes and Genomes (KEGG) pathway enrichment analysis. The thresholds were set as follows: Rank Criteria: P value; Sign: Positively correlated; Significance Level: 0.05.
Kaplan-Meier Plotter
Kaplan-Meier Plotter (http://kmplot.com/analysis/), a reliable portal, aims to evaluate the association between gene expression and survival in 21 cancer types (12). In this study, breast cancer patients were separated into high and low groups according to the median expression of ADAMDEC1 to assess overall survival (OS) and relapse-free survival (RFS). The Kaplan-Meier Plotter was also used to detect the association between hsa-miR-4534 expression and prognosis. The results were analyzed statistically using hazard ratios (HRs) with 95% confidence intervals (CIs) and log-rank P values.
GeneMANIA
GeneMANIA (http://www.genemania.org) is a reliable web resource that visualizes protein and genetic interactions and generates hypotheses about gene function (13). We used this resource to display a gene-gene interaction network of ADAMDEC1.
Search tool for recurring instances of neighboring genes (STRING)
STRING (https://string-db.org/) is a web-based tool for building physical and functional interaction networks using publicly available protein-protein interaction (PPI) data sources (14). In this study, we constructed a PPI network of ADAMDEC1.
Tumor Immune Estimation Resource (TIMER)
TIMER (https://cistrome.shinyapps.io/timer/) is a user-friendly portal that provides data for the molecular analysis of tumor-immune interactions (15). It was in this study used to investigate the relationship between infiltrating immune cells and ADAMDEC1 through the “Gene” module. This module was also used to detect the relationship between immune cells and hsa-miR-4534 expression. The relationship between immune cells and ADAMDEC1 copy number was explored using the “SCNA” module. The association between the expression of the long non-coding RNA (lncRNA) taurine up-regulated 1 (TUG1) and clinical outcomes was assessed using the “Exploration” module in TIMER 2.0.
An integrated repository portal for tumor-immune system interactions (TISIDB)
TISIDB (http://cis.hku.hk/TISIDB/), a dependable and user-friendly web server, can be used to conduct a comprehensive investigation of tumor-immune interactions (16). Our current analysis examined the immunomodulators regulated by ADAMDEC1 and investigated the relationship between ADAMDEC1 expression and immune cell infiltration levels using the Spearman correlation test.
Gene Set Cancer Analysis (GSCA)
GSCA (http://bioinfo.life.hust.edu.cn/GSCA/#/) is an established computational resource that integrates small-molecule drugs from the genomics of drug sensitivity in cancer (GDSC) and cancer therapeutics response portal (CTRP) databases as well as genomic and immunogenomic data from across 32 TCGA cancer types (17). In the present study, it was applied to explore the related pathways of ADAMDEC1 and its co-expression genes. We also evaluated the association between ADAMDEC1 expression and drug half maximal inhibitory concentration (IC50) from the CTRP database. The results were visualized as a bubble chart using the ggplot2 package (developer: Hadley Wickham; location: Houston, TX, USA) in R. To screen the related pathways and potentially effective drugs, the P value and false discovery rate (FDR) were calculated, and P<0.05 and FDR <0.05 were considered statistically significant.
StarBase v2.0
StarBase v2.0 (http://starbase.sysu.edu.cn/) is an open resource that provides an in-depth analysis of RNA-RNA and protein-RNA interaction networks from 108 crosslinking immunoprecipitation sequencing (CLIP-Seq) datasets (18). In this study, it was used to analyze the following relationships: microRNA (miRNA)-lncRNA, miRNA- messenger Ribonucleic Acids (mRNA), and mRNA-lncRNA.
DIANA-LncBase v2
DIANA-LncBase v2 (http://www.microrna.gr/LncBase) is a database for indexing miRNA targets on non-coding transcripts (19). The “Prediction module” provided a key clue regarding the upstream lncRNAs of hsa-mir-4534.
Candidate miRNA prediction
RNA22 (http://cm.jefferson.edu/rna22/Precomputed/) (20), miRWalk (http://mirwalk.umm.uni-heidelberg.de/) (21), and miRmap (http://mirmap.ezlab.org) (22) are publicly available comprehensive online tools. In this study, we used them to predict the upstream miRNAs of ADAMDEC1, and follow-up studies were performed only if the predicted miRNAs were included in all of the databases.
Statistical analysis
The UALCAN results were analyzed using a two-tailed Student’s t-test. A non-parametric test in LinkedOmics was used to analyze the relationship between ADAMDEC1 expression and clinical parameters. In TIMER and TISIDB, the relationships between ADAMDEC1 expression and immune infiltration were assessed using Spearman’s correlation and statistical significance. The Kaplan-Meier Plotter results were analyzed statistically using HRs with 95% CIs and log-rank P values. The results generated in UALCAN, LinkedOmics, TIMER, TISIDB, GSCA, StarBase v2.0, and RNA22 were displayed with P values, and P<0.05 was considered statistically significant.
Results
Identification of DEGs between chemosensitive and chemoresistant TNBC samples
Three datasets from the GEO database were selected: GSE34138, GSE20271, and GSE32646. GSE34138 contained 58 TNBC samples treated with the AC regimen; 24 samples achieved a pathological complete response (pCR), while the rest showed only a partial response or no response (non-pCR). In the GSE32646 dataset, 26 TNBC tissues treated with neoadjuvant P-FEC comprised 10 pCR samples and 16 non-pCR samples. The GSE20271 dataset included 24 TNBC samples with residual disease (RD) and four pCR samples after FAC or FEC treatment.
The DEGs were investigated by comparing the chemosensitive and chemoresistant tumors, respectively. Subsequently, we explored the overlaps of these DEG profiles using the Venn online tool and found that ADAMDEC1 was upregulated among all three chemosensitive groups (Figure 1A). However, there were no co-downregulated genes.
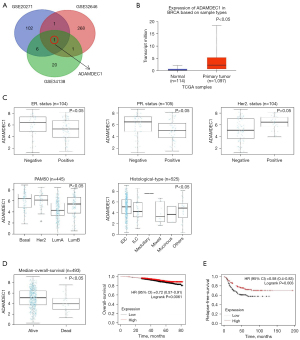
Expression and prognostic value of ADAMDEC1 in breast cancer patients
We explored the expression pattern of ADAMDEC1 to gain a comprehensive understanding of its role in breast cancer. Firstly, according to data from TCGA, an increased expression of ADAMDEC1 was observed in breast cancer tissues compared to normal tissues (Figure 1B). We then assessed the expression of ADAMDEC1 among patients with different clinical characteristics using the LinkedOmics web portal. The results indicated that ADAMDEC1 expression was significantly higher in patients who were ER-negative, PR-negative, or HER2-positive. Regarding the PAM50 subclasses, ADAMDEC1 expression was upregulated in basal-like and HER2-positive breast cancers compared to Luminal A and B breast cancers. In addition, higher mRNA levels of ADAMDEC1 were expressed in invasive ductal breast carcinoma (Figure 1C). However, no significant differences in ADAMDEC1 expression were found based on age, stage, or tumor, node, metastasis (TNM) stage stratification (Figure S1). These results illustrate that ADAMDEC1 expression is increased in breast cancer, especially in basal-like subtypes for which the commonly used systemic treatment is chemotherapy, indicating that the role of ADAMDEC1 in chemosensitivity requires further investigation.
To determine the prognostic value of ADAMDEC1 in breast cancer, we further investigated the relationship between ADAMDEC1 expression and clinical outcomes. In terms of OS, patients who were alive had higher ADAMDEC1 mRNA levels than those who died (Figure 1D). Moreover, we found that breast cancer patients with higher expression levels of ADAMDEC1 had a considerably longer OS and a longer RFS in TNBC based on data from the Kaplan-Meier database (Figure 1D,1E). These findings suggest that ADAMDEC1 mRNA expression is associated with a better prognosis in breast cancer.
PPI network and functional enrichment analysis of ADAMDEC1 in breast cancer
To explore the biological behavior of ADAMDEC1, we first constructed a PPI network of ADAMDEC1 and the interacted proteins (Figure 2A). A total of 19 edges and 11 nodes were predicted using STRING, including C-X-C motif chemokine ligand 9 (CXCL9) which may modulate the immunotherapeutic effect. Moreover, we also constructed a gene-gene interaction network using GeneMANIA (Figure 2B). The 20 most frequently altered genes were involved in the network, including Interleukin 18 (IL-18), C-C motif chemokine receptor 2 (CCR2), and leukocyte immunoglobulin like receptor B1 (LILRB1), which were closely correlated with the immune response. Furthermore, the 49 top genes that were most tightly and positively correlated with ADAMDEC1 were queried using LinkedOmics (Figure S2) and were used for further functional enrichment analysis. The related pathways of ADAMDEC1 and its co-expression genes were identified using GSCA (Figure 2C). These results showed that they were mainly enriched in apoptosis, which might explain why increased ADAMDEC1 expression was correlated to longer survival in breast cancer patients.
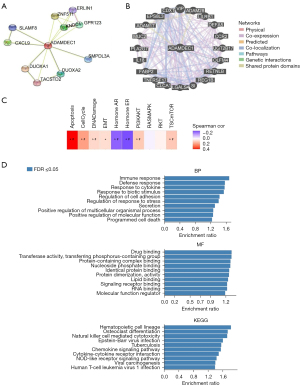
In addition, GO function and KEGG pathway enrichment analyses were carried out (Figure 2D). As expected, these results showed that the immune response was the most enriched signaling pathway in terms of biological process (BP), followed by the defense response, and the response to cytokines. In terms of molecular function (MF) analysis, ADAMDEC1 and the altered neighboring genes were significantly enriched in drug binding. Similarly, in terms of the top 10 KEGG pathways, ADAMDEC1 and its co-expression genes were found to be mostly involved in immune-related pathways, including natural killer cell-mediated cytotoxicity, the chemokine signaling pathway, cytokine-cytokine receptor interaction, and the NOD-like receptor signaling pathway. These findings indicate that ADAMDEC1 is significantly associated with the immune response in breast cancer, which may contribute to a better prognosis in ADAMDEC1high patients.
Correlation between ADAMDEC1 and immune cells
Based on the previous functional analysis, we explored the correlation between ADAMDEC1 and infiltrating immune cells. As shown in Figure 3A, ADAMDEC1 was uniformly and positively correlated with various types of immune cells, including B cells, cluster of differentiation (CD)8+ T cells, CD4+ T cells, macrophages, neutrophils, and dendritic cells, according to the TIMER database. Similarly, the expression of ADAMDEC1 was significantly associated with immune cells based on TISIDB (Figure S3).
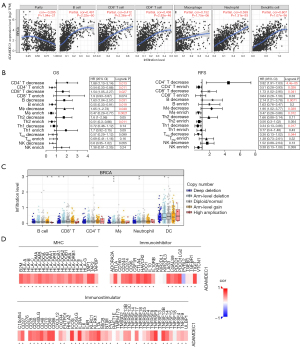
We then examined the relationship between ADAMDEC1 and the gene markers of immune cells. As expected, ADAMDEC1 was positively related to most immune markers (Table 1). These results further suggest that ADAMDEC1 is involved in the immune response. Moreover, we identified the impact of infiltrating immune cells on prognosis based on ADAMDEC1 expression in breast cancer patients (Figure 3B). In terms of the decreased infiltration of CD4+ T cells, CD8+ T cells, B cells, and macrophages, patients with high ADAMDEC1 had poor survival in OS and RFS. In contrast, a marked correlation between the upregulated expression of ADAMDEC1 and better OS and RFS was observed in patients with abundant immune cells. These findings reveal that enriched immune cells are favorable prognostic factors among patients with increased ADAMDEC1. In addition, we found that the infiltration levels of immune cells changed along with the TISIDB copy numbers (Figure 3C). Based on the TISIBD web portal, our analysis examined the immunomodulators that were regulated by ADAMDEC1 expression, and we found that ADAMDEC1 was positively associated with all major histocompatibility complex (MHC) molecules and most immunostimulators as well as immunoinhibitors (Figure 3D).
Table 1
Cell type | Gene marker | ADAMDEC1 | |
---|---|---|---|
cor | P | ||
B cell | |||
CD19 | 0.530 | *** | |
CD38 | 0.756 | *** | |
CD8+ T cell | |||
CD8A | 0.601 | *** | |
CD8B | 0.532 | *** | |
Tfh | |||
CXCR5 | 0.545 | *** | |
ICOS | 0.759 | *** | |
BCL-6 | −0.007 | 0.815 | |
Th1 | |||
IL12RB2 | 0.582 | *** | |
Th2 | |||
CCR3 | 0.346 | *** | |
STAT6 | −0.055 | 0.066 | |
GATA-3 | −0.324 | *** | |
Treg | |||
FOXP3 | 0.682 | *** | |
CCR8 | 0.694 | *** | |
Macrophage | |||
CD68 | 0.651 | *** | |
TAM | |||
CD80 | 0.692 | *** | |
CD86 | 0.703 | *** | |
NK | |||
XCL-1 | 0.588 | *** | |
CD7 | 0.615 | *** | |
KIR3DL1 | 0.438 | *** | |
DC | |||
CD1C | 0.308 | *** |
***, P<0.001. ADAMDEC1, ADAM-like Decysin-1; cor, correlation coefficient; TAM, tumor-associated macrophage; NK, natural killer; DC, dendritic cell.
Prediction and analysis of upstream non-coding RNAs (ncRNAs) of ADAMDEC1
To identify the upstream regulatory mechanism of ADAMDEC1 expression, we first predicted the possible miRNAs of ADAMDEC1. Seventeen miRNAs were obtained using the RNA22, miRWalk, and miRmap online tools (Figure 4A). Based on the competing endogenous RNA (ceRNA) hypothesis, miRNAs should be inversely correlated with targeted mRNA expression. As presented in Figure 4B, we finally observed a negative correlation between ADAMDEC1 and hsa-miR-4534. Next, we conducted a survival analysis of hsa-miR-4534 and found that it was associated with poor OS (Figure 4C). To assess the reason why hsa-miR-4534 was identified as an adverse survival biomarker, we investigated the correlation between hsa-miR-4534 and immune cells. As listed in Table 2, hsa-miR-4534 was negatively correlated with most immune cell gene markers. Taken together, these results suggest that hsa-miR-4534 might be a potential upstream miRNA of ADAMDEC1.
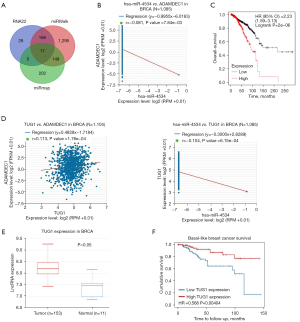
Table 2
Cell type | Gene marker | hsa-miR-4534 | |
---|---|---|---|
cor | P | ||
B cell | |||
CD19 | −0.041 | 0.175 | |
CD38 | −0.079 | ** | |
CD8+ T cell | |||
CD8A | −0.032 | 0.297 | |
CD8B | 0.043 | 0.154 | |
Tfh | |||
CXCR5 | −0.036 | 0.242 | |
ICOS | −0.070 | ** | |
BCL-6 | −0.121 | ** | |
Th1 | |||
IL12RB2 | 0.051 | 0.091 | |
Th2 | |||
CCR3 | 0.117 | ** | |
STAT6 | −0.034 | 0.262 | |
GATA-3 | −0.117 | ** | |
Treg | |||
FOXP3 | −0.075 | ** | |
CCR8 | −0.072 | ** | |
Macrophage | |||
CD68 | −0.076 | ** | |
TAM | |||
CD80 | −0.060 | ** | |
CD86 | −0.090 | ** | |
NK | |||
XCL-1 | −0.086 | ** | |
CD7 | 0.012 | 0.699 | |
KIR3DL1 | −0.030 | 0.330 | |
DC | |||
CD1C | −0.033 | 0.282 |
**, P<0.05. cor, correlation coefficient; TAM, tumor-associated macrophage; NK, natural killer; DC, dendritic cell.
It has been widely acknowledged that lncRNAs may boost mRNA expression by competitively binding to common miRNAs. The possible lncRNAs of hsa-miR-4534 were investigated utilizing the LncBase v2 database, and TUG1 was found to be positively linked to ADAMDEC1 and also negatively associated with hsa-miR-4534 (Figure 4D). Moreover, the expression of TUG1 was increased in breast tumors compared to normal tissues, which was consistent with that of ADAMDEC1 (Figure 4E). In addition, the high TUG1 expression indicated a favorable prognostic value in basal-like breast cancer (Figure 4F). These findings indicate that TUG1 might be the most likely upstream lncRNA of ADAMDEC1 and hsa-miR-4534. Finally, the TUG1/hsa-miR-4534/ADAMDEC1 axis was identified as a potential regulatory pathway in breast cancer.
Correlation between ADAMDEC1 expression and drug sensitivity
To further ascertain the effect of ADAMDEC1 on the efficacy of chemotherapy drugs, we evaluated the relationship between the expression of ADAMDEC1 and drug sensitivity from the CTRP database. ADAMDEC1 expression was negatively correlated with the IC50 value of paclitaxel, doxorubicin, docetaxel, and other chemotherapies, such as fluorouracil, gemcitabine, temsirolimus, olaparib, and dinaciclib, indicating a significant positive correlation with the sensitivity of these drugs. Likewise, the co-expression genes of ADAMDEC1 were positively correlated with the targeted and endocrine therapy drugs for HER2-positive and ER-positive breast cancer, respectively (Figure 5). These data imply that ADAMDEC1 plays a significant role in breast cancer treatment.
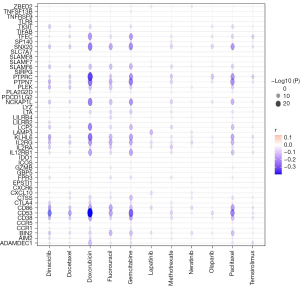
Discussion
Breast cancer has become the most common cause of cancer-related mortality among women worldwide (23). In recent years, breast cancer mortality has decreased significantly as a result of effective radiation and chemotherapies (24). According to previous reports, the response to neoadjuvant chemotherapy is associated with breast cancer survival (23). However, a large proportion of women still suffer drug resistance and recurrence, which is problematic and incurable following neoadjuvant systemic therapy. In addition, some patients with favorable prognostic features and in an early stage also have a significant risk of relapse due to treatment resistance (25). Although some novel markers have been demonstrated to predict the response to chemotherapy treatment, such as ACSL4 and GPX4 (26), the in-depth mechanism of chemoresistance is still unclear, and thus, more predictive markers are urgently required. It is crucial to explore reliable markers of the response to chemotherapy drugs in breast cancer to provide better therapy with high efficacy and low toxicity.
ADAMDEC1, a member of the ADAM family, has previously been shown to play a crucial role in immune response regulation. It is upregulated and associated with numerous inflammatory diseases, such as rosacea (27), systemic lupus erythematosus (28), and osteoarthritis (29), and is also involved in the regulation of tumors. A recent report has revealed that ADAMDEC1 is related to poor survival in glioblastomas and contributes to their invasiveness (30). In contrast, ADAMDEC1 is a positive regulator of epithelial defense against cancer (31). Also, ADAMDEC1 expression is significantly reduced in colon tumors compared with normal tissues. These findings highlight that the role of ADAMDEC1 in tumors has to be determined and reflect its unique characteristics in different tumors. However, the role of ADAMDEC1 in breast cancer has received little attention. In this study, increased ADAMDEC1 levels were observed in tumors that were sensitive to neoadjuvant chemotherapy, and the potential mechanism was explored.
We confirmed that ADAMDEC1 was a favorable survival biomarker, as patients with higher expression exhibited longer OS in breast cancer and better RFS in TNBC. To determine the details of the mechanisms affected by ADAMDEC1 in breast cancer, co-expression genes were detected using online tools. IL-18, CCR2, LILRB1, and CXCL9 are known key factors in immunotherapy. As expected, ADAMDEC1 and its neighboring genes were significantly enriched in immune response pathways and were positively related to the abundance of innate and adaptive immune cells, which played an important role in the maintenance of anti-tumor processes. Meanwhile, it was positive correlation with MHC molecules, which played the role of antigen presentation and immune stimulation. To our knowledge, the increased in-depth understanding of the anticancer process has revealed the activation of oncogenic pathways due to the failure of the immune response and decreased immune infiltration (32,33). The loss of antigenicity drives worse survival in breast cancer, even though the tumor is susceptible to conventional chemotherapeutics (34,35). These results confirm that the immune response contributes to better outcomes in patients with upregulated ADAMDEC1. We also found that the high expression of ADAMDEC1 was associated with some immunosuppressive cells, such as Treg, which might lead to the extrinsic immune escape. High levels of immunoinhibitors and immunostimulators were associated with the expression of ADAMDEC1, which affected the status of immune killing and detection by the immune system. Due to the interaction between immune microenvironment cells, it is not clear which of these plays a leading role. Moreover, it has been shown that the higher the infiltration of immune cells in TNBC, even with higher immunosuppressive cell infiltration, the better the prognosis compared with immune desert type tumors (36). It was worth noting that ADAMDEC1 was positively correlated with immune checkpoints, such as PDCD1 and CD274. Previous studies have demonstrated that PD-L1 might be an ideal biomarker for predicting the ICIs (immune checkpoint inhibitors) efficacy of TNBC (37), indicating that ADAMDEC1 might be associated with a good immunotherapy effect. Also, it has been shown that the DDFS (distant disease-free survival) and OS of TNBC patients are improved when ICIs added to neoadjuvant chemotherapy (38). This suggests that we can try ICIs combined with chemotherapy in TNBC patients with high ADAMDEC1 expression. However, further exploration and experimental support are needed.
To overcome chemoresistance and prevent tumors from escaping during treatment, several genomic and non-genomic mechanisms associated with the chemotherapeutic response have been explained (39,40). Interestingly, several studies have focused on the correlation between the immune system and the clinical activity of conventional chemotherapeutics (41). The higher efficacy of chemotherapy has been demonstrated in immunocompetent mouse models compared with their immunodeficient counterparts based on anthracyclines, oxaliplatin, and taxanes (42). Similarly, the pCR, as well as RFS and OS, are associated with the abundance of immune effectors in patients treated with anthracycline- or taxane-based neoadjuvant chemotherapy (43,44). In constant, the increased degree of lymphocyte infiltration is associated with high probability of pCR (45). Additionally, chemotherapy is used to stimulate the antineoplastic immune response (46). These findings indicate that higher immune infiltration contributes to a better response to chemotherapy. In this study, we speculated that high immune infiltration improves the efficacy of various chemotherapies in patients with elevated ADAMDEC1 expression. Meanwhile, ADAMDEC1 was sensitive to many chemotherapeutic drugs, including paclitaxel, doxorubicin, docetaxel, fluorouracil, gemcitabine, temsirolimus, olaparib, and dinaciclib, which enhanced the pCR rate.
To better understand the role of ADAMDEC1, we further investigated the upstream regulatory pathways of ADAMDEC1. By conducting correlation and survival analyses, hsa-miR-4534 was identified as an upstream regulatory miRNA. Jima et al. found that hsa-miR-4534 exhibits differential expression in normal and malignant human β cells; however, its function is not clear (47). In our study, we detected that up-regulated hsa-miR-4534 indicated worse survival in breast cancer. Interestingly, it was negatively correlated with immune markers, which might explain its unfavorable prognostic value. Furthermore, it has been confirmed that TUG1 downregulation is associated with cell proliferation, migration, and invasion in breast cancer (48). Similarly, we found that it corresponded with better outcomes in basal-like breast cancer. Taken together, we identified the TUG1/hsa-miR-4534/ADAMDEC1 axis as a potential regulatory pathway in breast cancer.
The current research improves our understanding of the relationship between ADAMDEC1 and breast cancer. Based on ADAMDEC1 expression levels, we can predict sensitivity to neoadjuvant chemotherapy and prognosis in advance, and better help patients make treatment decisions to avoid overtreatment and undertreatment. However, some limitations should be noted. Firstly, we analyzed the potential role of ADAMDEC1 based on mRNA levels; further verification is needed to make the data more convincing in terms of protein levels. Secondly, although we discussed possible mechanisms through which ADAMDEC1 might affect the response to chemotherapy, there is a lack of experimental verification.
Conclusions
In summary, our study demonstrated that ADAMDEC1 is associated with the sensitivity of chemotherapy and the prognosis of breast cancer patients, thus providing novel insights into the mechanisms of chemo-response.
Acknowledgments
We thank the Gene Expression Omnibus (GEO) database for sharing the large amount of data.
Funding: None.
Footnote
Reporting Checklist: The authors have completed the STREGA reporting checklist. Available at https://atm.amegroups.com/article/view/10.21037/atm-22-6319/rc
Conflicts of Interest: All authors have completed the ICMJE uniform disclosure form (available at https://atm.amegroups.com/article/view/10.21037/atm-22-6319/coif). The authors have no conflicts of interest to declare.
Ethical Statement: The authors are accountable for all aspects of the work in ensuring that questions related to the accuracy or integrity of any part of the work are appropriately investigated and resolved. The study was conducted in accordance with the Declaration of Helsinki (as revised in 2013).
Open Access Statement: This is an Open Access article distributed in accordance with the Creative Commons Attribution-NonCommercial-NoDerivs 4.0 International License (CC BY-NC-ND 4.0), which permits the non-commercial replication and distribution of the article with the strict proviso that no changes or edits are made and the original work is properly cited (including links to both the formal publication through the relevant DOI and the license). See: https://creativecommons.org/licenses/by-nc-nd/4.0/.
References
- Britt KL, Cuzick J, Phillips KA. Key steps for effective breast cancer prevention. Nat Rev Cancer 2020;20:417-36. [Crossref] [PubMed]
- Long-term outcomes for neoadjuvant versus adjuvant chemotherapy in early breast cancer: meta-analysis of individual patient data from ten randomised trials. Lancet Oncol 2018;19:27-39. [Crossref] [PubMed]
- Korde LA, Somerfield MR, Carey LA, et al. Neoadjuvant Chemotherapy, Endocrine Therapy, and Targeted Therapy for Breast Cancer: ASCO Guideline. J Clin Oncol 2021;39:1485-505. [Crossref] [PubMed]
- Garrido-Castro AC, Lin NU, Polyak K. Insights into Molecular Classifications of Triple-Negative Breast Cancer: Improving Patient Selection for Treatment. Cancer Discov 2019;9:176-98. [Crossref] [PubMed]
- Waks AG, Winer EP. Breast Cancer Treatment: A Review. JAMA 2019;321:288-300. [Crossref] [PubMed]
- Bianchini G, Balko JM, Mayer IA, et al. Triple-negative breast cancer: challenges and opportunities of a heterogeneous disease. Nat Rev Clin Oncol 2016;13:674-90. [Crossref] [PubMed]
- Kim C, Gao R, Sei E, et al. Chemoresistance Evolution in Triple-Negative Breast Cancer Delineated by Single-Cell Sequencing. Cell 2018;173:879-893.e13. [Crossref] [PubMed]
- Kennedy WR, Tricarico C, Gabani P, et al. Predictors of Distant Metastases in Triple-Negative Breast Cancer Without Pathologic Complete Response After Neoadjuvant Chemotherapy. J Natl Compr Canc Netw 2020;18:288-96. [Crossref] [PubMed]
- Barrett T, Wilhite SE, Ledoux P, et al. NCBI GEO: archive for functional genomics data sets--update. Nucleic Acids Res 2013;41:D991-5. [Crossref] [PubMed]
- Chandrashekar DS, Bashel B, Balasubramanya SAH, et al. UALCAN: A Portal for Facilitating Tumor Subgroup Gene Expression and Survival Analyses. Neoplasia 2017;19:649-58. [Crossref] [PubMed]
- Vasaikar SV, Straub P, Wang J, et al. LinkedOmics: analyzing multi-omics data within and across 32 cancer types. Nucleic Acids Res 2018;46:D956-63. [Crossref] [PubMed]
- Lánczky A, Győrffy B. Web-Based Survival Analysis Tool Tailored for Medical Research (KMplot): Development and Implementation. J Med Internet Res 2021;23:e27633. [Crossref] [PubMed]
- Warde-Farley D, Donaldson SL, Comes O, et al. The GeneMANIA prediction server: biological network integration for gene prioritization and predicting gene function. Nucleic Acids Res 2010;38:W214-20. [Crossref] [PubMed]
- Szklarczyk D, Gable AL, Nastou KC, et al. The STRING database in 2021: customizable protein-protein networks, and functional characterization of user-uploaded gene/measurement sets. Nucleic Acids Res 2021;49:D605-12. [Crossref] [PubMed]
- Li T, Fan J, Wang B, et al. TIMER: A Web Server for Comprehensive Analysis of Tumor-Infiltrating Immune Cells. Cancer Res 2017;77:e108-10. [Crossref] [PubMed]
- Ru B, Wong CN, Tong Y, et al. TISIDB: an integrated repository portal for tumor-immune system interactions. Bioinformatics 2019;35:4200-2. [Crossref] [PubMed]
- Liu CJ, Hu FF, Xia MX, et al. GSCALite: a web server for gene set cancer analysis. Bioinformatics 2018;34:3771-2. [Crossref] [PubMed]
- Li JH, Liu S, Zhou H, et al. starBase v2.0: decoding miRNA-ceRNA, miRNA-ncRNA and protein-RNA interaction networks from large-scale CLIP-Seq data. Nucleic Acids Res 2014;42:D92-7. [Crossref] [PubMed]
- Paraskevopoulou MD, Vlachos IS, Karagkouni D, et al. DIANA-LncBase v2: indexing microRNA targets on non-coding transcripts. Nucleic Acids Res 2016;44:D231-8. [Crossref] [PubMed]
- Miranda KC, Huynh T, Tay Y, et al. A pattern-based method for the identification of MicroRNA binding sites and their corresponding heteroduplexes. Cell 2006;126:1203-17. [Crossref] [PubMed]
- Sticht C, De La Torre C, Parveen A, et al. miRWalk: An online resource for prediction of microRNA binding sites. PLoS One 2018;13:e0206239. [Crossref] [PubMed]
- Vejnar CE, Blum M, Zdobnov EM. miRmap web: Comprehensive microRNA target prediction online. Nucleic Acids Res 2013;41:W165-8. [Crossref] [PubMed]
- Liedtke C, Mazouni C, Hess KR, et al. Response to neoadjuvant therapy and long-term survival in patients with triple-negative breast cancer. J Clin Oncol 2008;26:1275-81. [Crossref] [PubMed]
- Sung H, Ferlay J, Siegel RL, et al. Global Cancer Statistics 2020: GLOBOCAN Estimates of Incidence and Mortality Worldwide for 36 Cancers in 185 Countries. CA Cancer J Clin 2021;71:209-49. [Crossref] [PubMed]
- Radovich M, Jiang G, Hancock BA, et al. Association of Circulating Tumor DNA and Circulating Tumor Cells After Neoadjuvant Chemotherapy With Disease Recurrence in Patients With Triple-Negative Breast Cancer: Preplanned Secondary Analysis of the BRE12-158 Randomized Clinical Trial. JAMA Oncol 2020;6:1410-5. [Crossref] [PubMed]
- Sha R, Xu Y, Yuan C, et al. Predictive and prognostic impact of ferroptosis-related genes ACSL4 and GPX4 on breast cancer treated with neoadjuvant chemotherapy. EBioMedicine 2021;71:103560. [Crossref] [PubMed]
- Liu T, Deng Z, Xie H, et al. ADAMDEC1 promotes skin inflammation in rosacea via modulating the polarization of M1 macrophages. Biochem Biophys Res Commun 2020;521:64-71. [Crossref] [PubMed]
- Shi L, Li S, Maurer K, et al. Enhancer RNA and NFκB-dependent P300 regulation of ADAMDEC1. Mol Immunol 2018;103:312-21. [Crossref] [PubMed]
- Balakrishnan L, Nirujogi RS, Ahmad S, et al. Proteomic analysis of human osteoarthritis synovial fluid. Clin Proteomics 2014;11:6. [Crossref] [PubMed]
- Jimenez-Pascual A, Hale JS, Kordowski A, et al. ADAMDEC1 Maintains a Growth Factor Signaling Loop in Cancer Stem Cells. Cancer Discov 2019;9:1574-89. [Crossref] [PubMed]
- Yako Y, Hayashi T, Takeuchi Y, et al. ADAM-like Decysin-1 (ADAMDEC1) is a positive regulator of Epithelial Defense Against Cancer (EDAC) that promotes apical extrusion of RasV12-transformed cells. Sci Rep 2018;8:9639. [Crossref] [PubMed]
- Schreiber RD, Old LJ, Smyth MJ. Cancer immunoediting: integrating immunity's roles in cancer suppression and promotion. Science 2011;331:1565-70. [Crossref] [PubMed]
- Wellenstein MD, de Visser KE. Cancer-Cell-Intrinsic Mechanisms Shaping the Tumor Immune Landscape. Immunity 2018;48:399-416. [Crossref] [PubMed]
- Noblejas-López MDM, Nieto-Jiménez C, Morcillo García S, et al. Expression of MHC class I, HLA-A and HLA-B identifies immune-activated breast tumors with favorable outcome. Oncoimmunology 2019;8:e1629780. [Crossref] [PubMed]
- Galluzzi L, Humeau J, Buqué A, et al. Immunostimulation with chemotherapy in the era of immune checkpoint inhibitors. Nat Rev Clin Oncol 2020;17:725-41. [Crossref] [PubMed]
- Xiao Y, Ma D, Zhao S, et al. Multi-Omics Profiling Reveals Distinct Microenvironment Characterization and Suggests Immune Escape Mechanisms of Triple-Negative Breast Cancer. Clin Cancer Res 2019;25:5002-14. [Crossref] [PubMed]
- Schmid P, Adams S, Rugo HS, et al. Atezolizumab and Nab-Paclitaxel in Advanced Triple-Negative Breast Cancer. N Engl J Med 2018;379:2108-21. [Crossref] [PubMed]
- Loibl S, Schneeweiss A, Huober J, et al. Neoadjuvant durvalumab improves survival in early triple-negative breast cancer independent of pathological complete response. Ann Oncol 2022;33:1149-58. [Crossref] [PubMed]
- Echeverria GV, Ge Z, Seth S, et al. Resistance to neoadjuvant chemotherapy in triple-negative breast cancer mediated by a reversible drug-tolerant state. Sci Transl Med 2019;11:eaav0936. [Crossref] [PubMed]
- Magbanua MJ, Wolf DM, Yau C, et al. Serial expression analysis of breast tumors during neoadjuvant chemotherapy reveals changes in cell cycle and immune pathways associated with recurrence and response. Breast Cancer Res 2015;17:73. [Crossref] [PubMed]
- Mesnage SJL, Auguste A, Genestie C, et al. Neoadjuvant chemotherapy (NACT) increases immune infiltration and programmed death-ligand 1 (PD-L1) expression in epithelial ovarian cancer (EOC). Ann Oncol 2017;28:651-7. [Crossref] [PubMed]
- Zitvogel L, Pitt JM, Daillère R, et al. Mouse models in oncoimmunology. Nat Rev Cancer 2016;16:759-73. [Crossref] [PubMed]
- Senovilla L, Vitale I, Martins I, et al. An immunosurveillance mechanism controls cancer cell ploidy. Science 2012;337:1678-84. [Crossref] [PubMed]
- Issa-Nummer Y, Darb-Esfahani S, Loibl S, et al. Prospective validation of immunological infiltrate for prediction of response to neoadjuvant chemotherapy in HER2-negative breast cancer--a substudy of the neoadjuvant GeparQuinto trial. PLoS One 2013;8:e79775. [Crossref] [PubMed]
- Savas P, Salgado R, Denkert C, et al. Clinical relevance of host immunity in breast cancer: from TILs to the clinic. Nat Rev Clin Oncol 2016;13:228-41. [Crossref] [PubMed]
- Chen G, Emens LA. Chemoimmunotherapy: reengineering tumor immunity. Cancer Immunol Immunother 2013;62:203-16. [Crossref] [PubMed]
- Jima DD, Zhang J, Jacobs C, et al. Deep sequencing of the small RNA transcriptome of normal and malignant human B cells identifies hundreds of novel microRNAs. Blood 2010;116:e118-27. [Crossref] [PubMed]
- Fan S, Yang Z, Ke Z, et al. Downregulation of the long non-coding RNA TUG1 is associated with cell proliferation, migration, and invasion in breast cancer. Biomed Pharmacother 2017;95:1636-43. [Crossref] [PubMed]
(English Language Editor: A. Kassem)