Expression analysis of human glioma susceptibility gene and P53 in human glioma and its clinical significance based on bioinformatics
Highlight box
Key findings
• The P53 expression can be an effective biological indicator of poor prognosis of glioma.
What is known and what is new?
• The inactivation of p53 gene plays an important role in the progress of brain glioma.
• KNL1 high expression and low expression were significantly different in OS, and the high expression group was associated with poor prognosis.
What is the implication, and what should change now?
• We confirmed that P53 expression can be an effective biological indicator of poor prognosis of glioma. The study of miRNA or lncRNA regulating the P53 was needed.
Introduction
Glioma is the most common primary intracranial tumor in adults, accounting for 46–70% of all central nervous system (CNS) tumors (1-3). Epidemiological investigation has shown that the incidence of glioma is increasing year by year, and males are more commonly affected than females. The prognosis of glioma varies with age and pathological type. The 5-year survival rate is low, especially in glioblastoma multiforme (GBM) and elderly patients. The World Health Organization (WHO) has classified gliomas of the CNS as low-grade and high-grade (4). According to this classification, low-grade gliomas are classified into grade I and grade II (5). Grade I tumors are benign and slow-growing gliomas, whereas grade II tumors have the potential to develop into high-grade gliomas. High-grade gliomas can be classified as grade III and IV. Grade III tumors have increased tumor cells and high rates of metastasis and recurrence (6-8). Grade IV glioma has the highest malignant degree, showing high proliferation, metastasis, vasculogenesis, and necrosis. The WHO tumor grade is one of the prognostic factors of treatment outcome. Other factors include the age of the patient, the location of the tumor, the extent of surgical resection, and the proliferation index. The survival rates of patients with grade II and III tumors is 5 years and 2–3 years, respectively (9). Patients with grade IV cancer die of the disease within one year, depending on the effective protocols available (9).
The pathogenesis of glioma is complex, involving risk factors such as ionizing radiation, hereditary syndromes, family history of brain tumors, and immunosuppression (10). However, genetic factors are thought to be the main cause of cancer. P53 gene is the most studied tumor suppressor gene at present, which has powerful functions of regulating cell cycle, repairing DNA after damage and inducing cell apoptosis. As one of the most common mutation genes in brain glioma, the inactivation of p53 gene plays an important role in the progress of brain glioma. A recent study had revealed several major molecular alterations typical for different types of tumors, such as IDH1/IDH2 mutations in diffuse invasive gliomas, TP53 and ATRX mutations in astrocytomas in oligodendrocytomas, mutations in the TERT promoter in oligodendroglioma and IDH wild-type, and BRAF mutations or fusions in astrocytomas, especially in children (10). Identifying those abnormalities in tumors and several other genetic abnormalities is therefore clinically important to help clinicians to determine the right treatment strategy and predict prognosis (11).
At present, the treatment of glioma is mainly surgical resection of the tumor, combined with radiotherapy, chemotherapy, and other comprehensive treatment methods (12). It is recommended that the operation should be safe and achieve the maximum extent of tumor resection. Radiotherapy can kill or inhibit the residual tumor cells and prolong the survival time of patients (13). Glioma has the characteristics of recurrence in situ, and 90% of the recurrence occurs within 2 cm from the primary lesion (14). Therefore, optimization of the local radiotherapy regimen is the focus of treatment. Chemotherapy is an effective treatment for glioma. Temozolomide (TMZ) combined with concurrent radiotherapy, followed by 6 cycles of TMZ adjuvant chemotherapy, can prolong the survival time of patients with GBM, increasing the median survival time to 14.6 months. TMZ concurrent radiotherapy combined with adjuvant chemotherapy has become the standard treatment for newly diagnosed GBM. At present, molecular biology, immunotherapy, molecular targeted therapy, and other new therapies are gradually applied in the treatment of glioma (15-17). Molecular targeted therapy is a new therapeutic concept proposed in recent years. Many targeted drugs have been developed for different target molecules. So far, only targeted therapies against angiogenesis have had some effect. For example, it is used alone or in combination for the treatment of lung cancer, esophageal cancer, breast cancer, and other malignant tumors. Among them, bevacizumab is the most common treatment at present. The efficacy of bevacizumab on recurrent GBM is limited to a few phase II clinical trials, but strong clinical data to validate the efficacy is still lacking (18).
Co-expression analysis helps to identify genes in the network that lead to disruption of regulatory mechanisms. Gene expression data analysis is widely used in transcriptomic studies to understand the function and intermolecular interactions of intracellular molecules. Co-expression analysis investigated disease and phenotypic variation and found that the co-expression patterns of gene modules varied under different conditions (19). Over the past few years, numerous studies have been conducted to explore the underlying molecular mechanisms, genetics, and developmental pathways of GBM. However, the exact mechanism of GBM remains unclear. In recent years, bioinformatics analysis has attracted extensive attention and made consistent breakthroughs in the search for oncogenes (20). A variety of biomarkers have been discovered for cancer diagnosis and prognosis to better understand the underlying molecular mechanisms of GBM and to search for new potential therapeutic targets. Moreover, the correlation between P53 expression and clinicopathological parameters and prognosis was poorly studied. Therefore, this study provides more research evidence for GBM based on bioinformatics. Immunohistochemistry (IHC) was used to further observe the expression of P53 in glioma and its influence on clinical features, pathology, and prognosis. We present the following article in accordance with the STREGA reporting checklist (available at https://atm.amegroups.com/article/view/10.21037/atm-22-5646/rc).
Methods
Data download
We downloaded 2 groups of RNA-seq data and clinical data from The Cancer Genome Atlas (TCGA) database (https://cancergenome.nih.gov/). The dataset comprised a total of 173 samples which were categorized as patients with GBM (n=156) and normal samples (n=5), and other samples were grouped into unknown groups. In addition, a total of 523 samples were diagnosed as grade II and III gliomas. The data was downloaded in August 2022 (details at: http://www.cgga.org.cn/about.jsp). The study was conducted in accordance with the Declaration of Helsinki (as revised in 2013).
Data preprocessing
RNA-seq data for the disease in TCGA data were downloaded using the R software TCGAbiolinks in R (The R Foundation for Statistical Computing, Vienna, Austria). The ENSEMBL ID in RNA-seq is re-annotated using the GTF annotation file in GENCODE (https://www.gencodegenes.org/) to obtain the corresponding gene symbol. At the same time, messenger RNAs (mRNAs) in the data were screened out according to the information in the annotation file. Since multiple ENSEMBL IDs in the data may correspond to the same gene symbol, its average value was obtained as the unique expression value corresponding to the mRNA. Disease survival information and clinical data were obtained from cBioPortal (https://www.cbioportal.org/).
P53 expression and different cancer stages
The R software GGPUBR package was used to a draw violin map of P53 gene expression in glioma samples according to clinical information, and to mine the expression of P53 in different cancer stages of WHO grade (Grade II-IV). The glioma samples were grouped and plotted separately. If WHO grade clinical data of cancer stage are missing from a sample, the sample was not included in the figure for that stage type.
Gene-related survival analysis
The Kaplan-Meier survival curve is one of the promising methods for predicting survival and prognostic value. An online database containing microarray data sets. According to the expression level of P53 among samples, the total samples were divided into 2 groups: high expression samples and low expression samples. Secondly, according to the downloaded survival data, R and survival were used to analyze the survival of P53 gene and low-grade glioma (LGG) + GBM samples. The Kaplan-Meier survival curve was drawn, and the log-rank test was performed to determine whether there was any difference between groups.
Gene regulatory network
The Pathway Commons Network Visualizer (PCViz) is an efficient public network visualization tool online platform which can be used to link targets to interacting genes. We used the PCViz website (http://www.pathwaycommons.org/pcviz/) to construct a regulatory network including the P53 gene and the genes interacting with it. By inputting the name of the P53 gene, we could obtain all of its interacting genes. The top 50 genes were selected to construct the final gene regulatory network. The network also included the following types of functions: regulating protein state changes, regulating gene expression, and regulating phosphorylation in the same protein complex.
Gene co-expression analysis of the regulatory network
Based on the calculation method of Spearman’s correlation coefficient, the co-expression relationship between P53 and other genes in the regulatory network was analyzed. The correlation coefficient r>0.75 and P<0.05 were used as the threshold of the screened co-expression relationship. The ggpubr package (https://cran.rproject.org/web/packages/ggpubr/index.html) was applied to draw the correlation map of gene expression.
Differential analysis of co-expressed genes
Co-expression analysis is increasingly used to study the global transcriptional mechanisms underlying phenotypic changes. There were not enough blank samples in TCGA to test whether co-expressed genes were differentially expressed in GBM samples versus normal samples and LGG samples versus normal samples, so gene expression profiling interactive analysis (GEPIA) was used (http://gepia.cancer-pku.cn/detail.php). The database was used to screen the differentially co-expressed genes in the 2 comparison groups. GEPIA provides the normal sample data of TCGA as well as the normal samples in Genotype-Tissue Expression (GTEx). The process was as follows: select P53 gene, set |log2FC| =1.585 (fold change =3), P=0.05, select LGG and GBM for datasets, and obtain the corresponding box line diagram of P53. The genes were replaced with other genes co expressed with P53, and the corresponding box plots were drawn respectively.
Kyoto Encyclopedia of Genes and Genomes (KEGG) and Gene Ontology (GO) enrichment analysis
We used the following databases to extract the biological functions of many genes: Database for Annotation, Visualization and Integrated Discovery (David), GO, and the KEGG. The R software cluster profiler package was used to analyze the enrichment of KEGG and GO biological process (BP) of P53 and the above differentially co-expressed genes and a bubble chart was used to display the results. On the basis of KEGG pathway/GO terms, all GO terms involved in P53 were displayed. A P value <0.05 represented the significance of GO or pathway entries. The significantly enriched functional analysis results were visualized by David.
Statistical analysis
The statistical software SPSS 23.0 was used for data analysis. Chi square test was used for the expression of P53 in glioma and normal tissues; the correlation between P53 expression and clinicopathological parameters in glioma specimens was tested by chi square test; the correlation with Ki-67 in tumor tissue was analyzed by Spearman rank correlation analysis. Kaplan-Meier survival analysis was used to observe the effect of P53 expression on progression-free survival (PFS) and overall survival (OS) of malignant glioma patients during the follow-up period, and log rank analysis was used to compare the significant differences between the groups; Cox regression model was constructed to analyze the relationship between P53 protein expression level, general clinical factors, and patient prognosis (PFS and OS). The difference was considered statistically significant when P<0.05.
Results
P53 gene expression and different cancer stages
Among the data downloaded from TCGA, 60,483 probes were aligned to ensemblID. When the annotation file was used, a total of 19,600 mRNAs were found in the dataset, and a total of 19,579 different genes were screened. The amount of P53 expression was grouped using the glioma grade (grade II-IV, not included in grade I) in the clinical information (Figure 1).
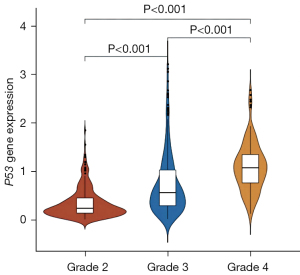
P53 gene-related survival analysis
The Kaplan-Meier plotter (http://www.kmplot.com) was used to evaluate the relationship between P53 gene expression and GBM clinical prognosis. LGG and GBM samples were divided into a high expression group and low expression group according to the median expression value of P53. From the survival curve, it can be seen that there was a significant difference between high expression P53 and low expression P53 in the OS period, and the high expression group was associated with poor prognosis (P<0.05) (Figure 2).
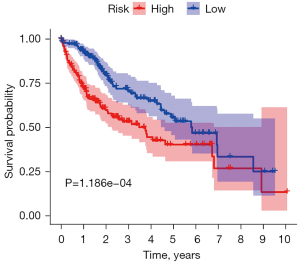
Gene regulatory network
A total of 172 genes and 8,422 interaction relationships were obtained by inputting the P53 gene into the PCViz website. The first 50 closely related genes were selected to display the P53 family related regulatory network. The gene regulatory network contains 3 relationships with knl1, for example, INS, CREB1, and other genes can regulate the expression of knl1 gene. It was shown that CDK1, CDC42, and CCNB1 can regulate the state change of P53 (Figure 3).
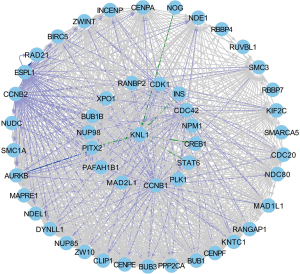
Gene co-expression analysis in the regulatory network
In order to confirm the relationship between P53 and the genes in the above regulatory network, Spearman’s test was performed to analyze the co-expression of 50 genes in all regulatory networks. With P<0.05, |R|>0.75 as the screening threshold, a total of 4 genes co-expressed with P53 were obtained: ZWIN, PLK1, NDC80, and MAD2L1, and it was found that the screened genes were positively correlated with the expression of P53 (Figure 4).
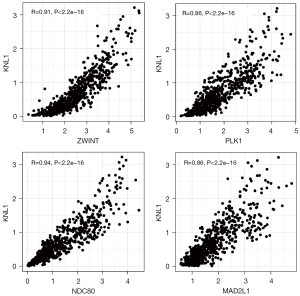
KEGG and GO enrichment analysis
In order to further learn the role of P53 in glioma, KEGG enrichment analysis and GO BP were performed on the 17 differentially expressed genes (DEGs). The most significant KEGG pathway was cell cycle and oocyte meiosis; however, knl1 was not enriched to any KEGG pathway. GO BP terms with significant enrichment of co-expressed DEGs included chromosome aggregation, mitotic sister chromatid aggregation. The mitotic sister chromatid aggregation term contained 14 proteins, including P53 (Figure 5).
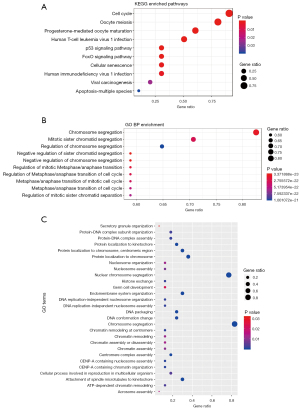
Heat map analysis
In the first part of the article, the authors systematically studied the relationship between the pathological characteristics of glioma such as WHO grade, IDH status, co-deletion status, and EMT, because previous studies had shown that P53 is closely related to the malignant degree of glioma. Relevant studies have considered the possible relationship between lymphocyte infiltration and tumor prognosis. Most of the cells infiltrating the tumor are T cells, and the degree of lymphocyte infiltration in the primary tumor is positively correlated with tumor metastasis (Figure 6).
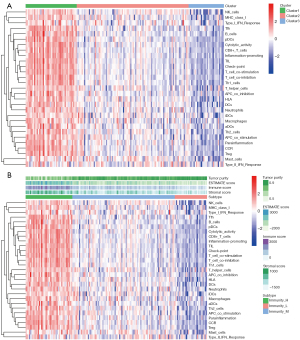
Correlation analysis of immune infiltrating cells
Tumor immune cell infiltration refers to the infiltration of immune cells from the blood to the tumor tissue, which can be separated from the tumor tissue. The infiltration of immune cells in tumors is closely related to clinical outcomes. The infiltrated immune cells in tumors are most likely to be used as drug targets to improve the survival rate of patients (Figure 7).
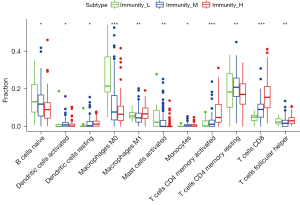
GSEA analysis
The input of GSEA is a gene expression matrix, in which the samples are divided into 2 groups: A and B. First, all genes are sorted. In a simple summary, GSEA is designed to sort from large to small according to the difference multiplier value after treatment, which is used to indicate the change trend of gene expression between the 2 groups. The top of the sorted gene list can be regarded as the up-regulated DEGs, and the bottom is the down-regulated DEGs (Figure 8).
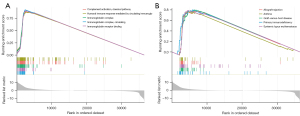
Discussion
Glioma is the most common brain tumor in adults, accounting for about 40–60% of primary intracranial tumors (21). The incidence rate shows a trend of further increase, with an annual growth rate of about 1–2%. Astrocytes, oligodendrocytes, and ependymal gliomas account for more than 70% of all brain tumors (22). Among all malignant gliomas, GBM is the most common and malignant tissue type, with a higher incidence in males than females. It is more common in the elderly, followed by diffuse astrocytes (23). At present, the main treatment for glioma is surgery combined with radiotherapy and chemotherapy, which can prolong the survival period to a certain extent. However, due to the characteristics of rapid recurrence and drug resistance of high-grade glioma, the one-year survival rate is about 30% (24). In recent years, some new therapies have been widely developed, such as immunotherapy, gene therapy, and targeted therapy, which have brought new hope to the treatment of glioma; however, the effect has not reached the expectation and the prognosis remains very poor. Therefore, it is of great significance to study the mechanism of glioma and explore the possible therapeutic targets for clinical treatment and improving prognosis (25). At present, the occurrence and development of glioma are driven by the dynamic changes of gene pathway activity and connectivity. Revealing these dynamic events is necessary for understanding the pathological mechanism of glioma and developing effective therapeutic methods (26). The mutation rate of p53 gene in malignant glioma is 35–60%. The aim of this study was to investigate the abnormal genes closely related to the occurrence of gliomas and to determine whether the expression of P53 is significantly different with different cancer stages (27).
Thanks to the rapid development of bioinformatics (28), a previous study has found that the high expression of P53 is closely related to the progression and prognosis of lung cancer, colorectal cancer, and gastric cancer (29). Through IHC, we found that the expression of P53 in glioma was higher than that in normal brain tissue. A total of 74 cases (62.18%) of glioma cells showed positive expression. However, in the control group, there were 2 cases (10%) of P53 positive expression. There was a significant difference in the expression between the glioma group and the control group. The expression of P53 was also different in different grades of gliomas (30). The positive expression of P53 in high grade gliomas was higher than that in low grade gliomas. Our results were consistent with these findings. However, the correlation between P53 expression and clinicopathological parameters and prognosis was not further studied. Therefore, P53 expression was also analyzed in this study. There were differences between the positive and negative expression of P53 in Karnofsky performance scale (KPS) score, WHO grade, and age of patients. This indicated that the positive expression of P53 was higher than the negative expression in KPS, WHO grade, and age (P<0.05) (31-33). In addition, we analyzed whether there was a correlation between the expression of P53 and the prognostic molecule Ki-67. The results showed that the poorly differentiated gliomas had a high expression of Ki-67, and the gliomas with P53 positive expression had a significant correlation with the high expression of Ki-67 (34). In the evaluation of prognosis, Kaplan-Meier was first applied to analyze the relationship between the expression of P53 and the PFS and OS of glioma patients. We found that the positive expression of P53 was closely related to the clinical prognosis of glioma patients (35). Glioma is one of the most immunosuppressive solid tumors. The ability of glioma to cause severe systemic T cell defects is one of its most prominent and earliest reported immune effects. We also found that the infiltration of immune cells in tumors was closely related to clinical outcomes.
Limitations
This study also had some limitations: (I) the sample size of this study is small, and the time to analysis of pathological tissue was long. The staining effect may have been distorted or flaked, leading to the possibility that the expression rate of P53 protein was low. It was a single center study, and the conclusion needs further confirmation; (II) the occurrence, development, and mechanism of P53 in gliomas were not studied in this study, which needs to be further explored in the future. Therefore, P53 as a prognostic indicator and potential immunotherapeutic target in glioma deserves further study; (III) we failed to study the miRNA or lncRNA regulating the P53; (IV) finally, in vivo and in vitro experimental were also needed to confirm these findings.
Conclusions
The innovation of this study is to propose the correlation between P53 expression level and clinicopathological parameters and prognosis of glioma for the first time, and confirm that P53 expression can be an effective biological indicator of poor prognosis of glioma. At the same time, P53 related indicators may also become new therapeutic targets.
Acknowledgments
Funding: None.
Footnote
Reporting Checklist: The authors have completed the STREGA reporting checklist. Available at https://atm.amegroups.com/article/view/10.21037/atm-22-5646/rc
Conflicts of Interest: All authors have completed the ICMJE uniform disclosure form (available at https://atm.amegroups.com/article/view/10.21037/atm-22-5646/coif). The authors have no conflicts of interest to declare.
Ethical Statement: The authors are accountable for all aspects of the work in ensuring that questions related to the accuracy or integrity of any part of the work are appropriately investigated and resolved. The study was conducted in accordance with the Declaration of Helsinki (as revised in 2013).
Open Access Statement: This is an Open Access article distributed in accordance with the Creative Commons Attribution-NonCommercial-NoDerivs 4.0 International License (CC BY-NC-ND 4.0), which permits the non-commercial replication and distribution of the article with the strict proviso that no changes or edits are made and the original work is properly cited (including links to both the formal publication through the relevant DOI and the license). See: https://creativecommons.org/licenses/by-nc-nd/4.0/.
References
- Griveau A, Seano G, Shelton SJ, et al. A Glial Signature and Wnt7 Signaling Regulate Glioma-Vascular Interactions and Tumor Microenvironment. Cancer Cell 2018;33:874-889.e7. [Crossref] [PubMed]
- Sun X, Pang P, Lou L, et al. Radiomic prediction models for the level of Ki-67 and p53 in glioma. J Int Med Res 2020;48:300060520914466. [Crossref] [PubMed]
- Jiang L, Cheng C, Ji W, et al. LINC01116 promotes the proliferation and invasion of glioma by regulating the microRNA-744-5p-MDM2-p53 axis. Mol Med Rep 2021;23:366. [Crossref] [PubMed]
- Lou J, Hao Y, Lin K, et al. Circular RNA CDR1as disrupts the p53/MDM2 complex to inhibit Gliomagenesis. Mol Cancer 2020;19:138. [Crossref] [PubMed]
- Kadiyala P, Carney SV, Gauss JC, et al. Inhibition of 2-hydroxyglutarate elicits metabolic reprogramming and mutant IDH1 glioma immunity in mice. J Clin Invest 2021;131:139542. [Crossref] [PubMed]
- Wang Z, Ding Y, Wang X, et al. Pseudolaric acid B triggers ferroptosis in glioma cells via activation of Nox4 and inhibition of xCT. Cancer Lett 2018;428:21-33. [Crossref] [PubMed]
- Sheng J, He X, Yu W, et al. p53-targeted lncRNA ST7-AS1 acts as a tumour suppressor by interacting with PTBP1 to suppress the Wnt/β-catenin signalling pathway in glioma. Cancer Lett 2021;503:54-68. [Crossref] [PubMed]
- Cai Y, Gu WT, Cheng K, et al. Knockdown of TRIM32 inhibits tumor growth and increases the therapeutic sensitivity to temozolomide in glioma in a p53-dependent and -independent manner. Biochem Biophys Res Commun 2021;550:134-41. [Crossref] [PubMed]
- Larson JD, Kasper LH, Paugh BS, et al. Histone H3.3 K27M Accelerates Spontaneous Brainstem Glioma and Drives Restricted Changes in Bivalent Gene Expression. Cancer Cell 2019;35:140-155.e7. [Crossref] [PubMed]
- Wang C, Tu H, Yang L, et al. FOXN3 inhibits cell proliferation and invasion via modulating the AKT/MDM2/p53 axis in human glioma. Aging (Albany NY) 2021;13:21587-98. [Crossref] [PubMed]
- Chai C, Song LJ, Han SY, et al. MicroRNA-21 promotes glioma cell proliferation and inhibits senescence and apoptosis by targeting SPRY1 via the PTEN/PI3K/AKT signaling pathway. CNS Neurosci Ther 2018;24:369-80. [Crossref] [PubMed]
- Chen SR, Cai WP, Dai XJ, et al. Research on miR-126 in glioma targeted regulation of PTEN/PI3K/Akt and MDM2-p53 pathways. Eur Rev Med Pharmacol Sci 2019;23:3461-70. [PubMed]
- Buyandelger B, Bar EE, Hung KS, et al. Histone deacetylase inhibitor MPT0B291 suppresses Glioma Growth in vitro and in vivo partially through acetylation of p53. Int J Biol Sci 2020;16:3184-99. [Crossref] [PubMed]
- Sang W, Xue J, Su LP, et al. Expression of YAP1 and pSTAT3-S727 and their prognostic value in glioma. J Clin Pathol 2021;74:513-21. [Crossref] [PubMed]
- Luo X, Ge J, Chen T, et al. LHX9, a p53-binding protein, inhibits the progression of glioma by suppressing glycolysis. Aging (Albany NY) 2021;13:22109-19. [Crossref] [PubMed]
- Yang W, Wang H, Ju H, et al. A study on the correlation between STAT 1 and mutant p53 expression in glioma. Mol Med Rep 2018;17:7807-12. [Crossref] [PubMed]
- Gonzalez PP, Kim J, Galvao RP, et al. p53 and NF 1 loss plays distinct but complementary roles in glioma initiation and progression. Glia 2018;66:999-1015. [Crossref] [PubMed]
- Guo CF, Zhuang Y, Chen Y, et al. Significance of tumor protein p53 mutation in cellular process and drug selection in brain lower grade (WHO grades II and III) glioma. Biomark Med 2020;14:1139-50. [Crossref] [PubMed]
- Fischer NW, Prodeus A, Gariépy J. Survival in males with glioma and gastric adenocarcinoma correlates with mutant p53 residual transcriptional activity. JCI Insight 2018;3:e121364. [Crossref] [PubMed]
- Shao W, Hao ZY, Chen YF, et al. OIP5-AS1 specifies p53-driven POX transcription regulated by TRPC6 in glioma. J Mol Cell Biol 2021;13:409-21. [Crossref] [PubMed]
- Zhao QS, Ying JB, Jing JJ, et al. LncRNA FOXD2-AS1 stimulates glioma progression through inhibiting P53. Eur Rev Med Pharmacol Sci 2020;24:4382-8. [PubMed]
- Chen C, Hou J, Tanner JJ, et al. Bioinformatics Methods for Mass Spectrometry-Based Proteomics Data Analysis. Int J Mol Sci 2020;21:2873. [Crossref] [PubMed]
- Li K, Du Y, Li L, et al. Bioinformatics Approaches for Anti-cancer Drug Discovery. Curr Drug Targets 2020;21:3-17. [Crossref] [PubMed]
- Azad RK, Shulaev V. Metabolomics technology and bioinformatics for precision medicine. Brief Bioinform 2019;20:1957-71. [Crossref] [PubMed]
- Fu Y, Ling Z, Arabnia H, et al. Current trend and development in bioinformatics research. BMC Bioinformatics 2020;21:538. [Crossref] [PubMed]
- Huang X, Liu S, Wu L, et al. High Throughput Single Cell RNA Sequencing, Bioinformatics Analysis and Applications. Adv Exp Med Biol 2018;1068:33-43. [Crossref] [PubMed]
- Gong L, Zhang D, Dong Y, et al. Integrated Bioinformatics Analysis for Identificating the Therapeutic Targets of Aspirin in Small Cell Lung Cancer. J Biomed Inform 2018;88:20-8. [Crossref] [PubMed]
- van Gelder CWG, Hooft RWW, van Rijswijk MN, et al. Bioinformatics in the Netherlands: the value of a nationwide community. Brief Bioinform 2019;20:540-50. [Crossref] [PubMed]
- Ali MM, Hamid M, Saleem M, et al. Status of Bioinformatics Education in South Asia: Past and Present. Biomed Res Int 2021;2021:5568262. [Crossref] [PubMed]
- Pittard WS, Villaveces CK, Li S. A Bioinformatics Primer to Data Science, with Examples for Metabolomics. Methods Mol Biol 2020;2104:245-63. [Crossref] [PubMed]
- Canzoneri R, Lacunza E, Abba MC. Genomics and bioinformatics as pillars of precision medicine in oncology. Medicina (B Aires) 2019;79:587-92. [PubMed]
- Noor Z, Ranganathan S. Bioinformatics approaches for improving seminal plasma proteome analysis. Theriogenology 2019;137:43-9. [Crossref] [PubMed]
- Costa MC, Gabriel AF, Enguita FJ. Bioinformatics Research Methodology of Non-coding RNAs in Cardiovascular Diseases. Adv Exp Med Biol 2020;1229:49-64. [Crossref] [PubMed]
- Paul P, Antonydhason V, Gopal J, et al. Bioinformatics for Renal and Urinary Proteomics: Call for Aggrandization. Int J Mol Sci 2020;21:961. [Crossref] [PubMed]
- Auslander N, Gussow AB, Koonin EV. Incorporating Machine Learning into Established Bioinformatics Frameworks. Int J Mol Sci 2021;22:2903. [Crossref] [PubMed]
(English Language Editor: J. Jones)